How to Build an Effective AI Business Strategy
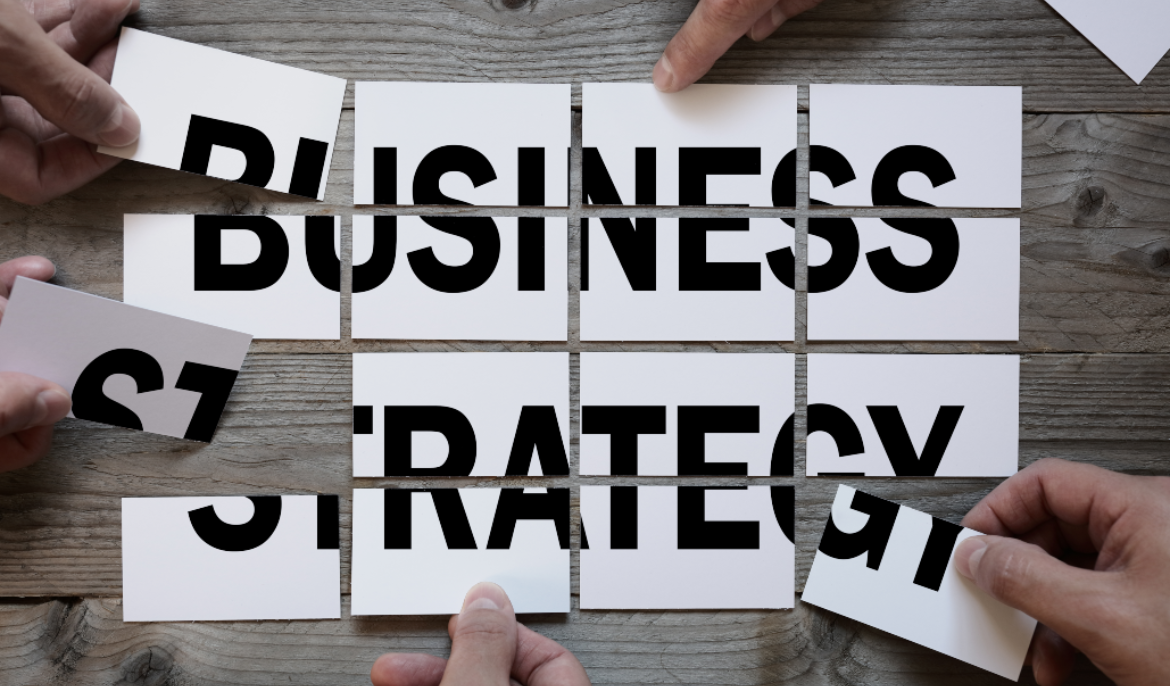
Focus on the problems you need to solve, not on the technology.
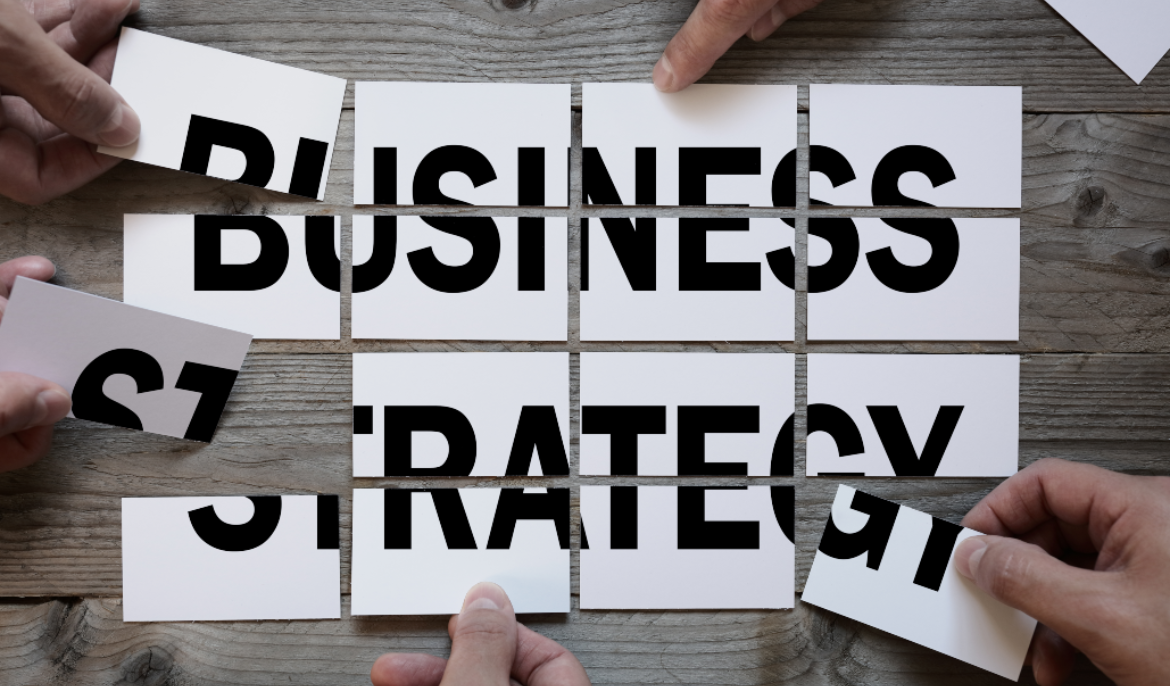
Think of machine learning and AI strategy in terms of what problems they will solve, not what technology you're going to bring to bear first. That was the advice that <a href="https://www.linkedin.com/in/catherine-williams-3aa74535/" target="_blank">”Catherine Williams”</a>, global head of iQ at Qualtrics, gave during a talk at <a href="https://exchange.scale.com/home/events/scale-transformx" target="_blank">”TransformX 2021”</a>.
"Everyone knows you have to have machine learning in your story, or you're not sexy," she said. But while a lot of new companies are AI first, many older companies that were not invented with artificial intelligence in mind. "Those are the ones that are going to need to be retrofitted in order to keep up in the 21st century."
<br> <div style="position: relative; padding-bottom: 56.25%; height: 0;"><iframe src="https://fast.wistia.net/embed/iframe/7wri03u55r" frameborder="0" webkitallowfullscreen mozallowfullscreen allowfullscreen style="position: absolute; top: 0; left: 0; width: 100%; height: 100%;"></iframe></div> </br> So what kinds of problems should you be thinking about, and where should you start? Williams shared a simple framework (see figure below) with two key dimensions that can help:-
**Dimension 1: **Do you want to create knowledge for humans, or do you want to create an action or some sort of a task?
-
**Dimension 2: **Do you intend to have a human in the loop, or do you intend the system to be automatic?
How it works in the real world
Williams discussed how this works in practice, providing many examples.
“If you want to generate knowledge and you want to have a human in the loop, you're basically talking about analysis tools,” she said. For example, consider SPSS or similar analytics tools that enable people to dig into data and generate insights (see the figure below). The models might recommend the next-best action for service people when they're interacting with a customer, such as recommending a troubleshooting step. “It's action oriented, but there's a human in the loop who's ultimately going to make that decision,” Williams said.
Auto complete is a simple example of something more automatic that suggests actions. Machine learning can suggest how you might complete a thought or finish a sentence. “It's just one keystroke to actually do that completion, which is why it's a little further down on my spectrum.”
Autocorrect moves even further down into automation. So think about this. “This is where the system does something for you automatically, but you as the human do have an opportunity to go back and undo it. So there's an opportunity for a human to join the loop, but they don't have to, it just happens automatically.”
Programmatic marketplaces provide other examples of automatic actions. “We built algorithms that would do real time bidding based on machine learning to determine which ad impressions to buy on behalf of a given advertiser, or similarly how to set floor prices if you're a publisher wanting to monetize your advertising, your inventory, your digital inventory,” Williams said. And there are other examples where there's not even an opportunity for the human to be in the loop. “The bidding and the buying just happens autonomously. And maybe you can go back and correct it or audit it later.”
Figure 1. This framework helps to identify problems that AI could potentially solve. Blue dots are examples from Qualtrics and its iQ platform. Black dots are other industry examples. Image credit: Qualtrics
Your next steps
Once you have a framework to help identify problems to solve, what’s next? There are many ways to maximize the business impact of AI, said Williams. Here are a few to get you started.
-
Get traction on the problem. Execute rigorously and turn the data into something of value. You can’t fake it, said Williams.
-
**Acquire more data. **You have plenty of options to do this. You can improve the instrumentation for collecting data from your environment, or you can buy it, partner for it, or create it. Think creatively.
-
**Extract more signals from your existing data. **Deep neural networks, for example, help companies get more signal out of images, audio, video, and natural language than before. Creating ensembles of models also helps generate insight.
-
**Contextualize. **Look for elements of the specific problem to fine-tune and make a more effective solution. At a very high level, this could take the form of something as simple as developing a schema or ontology for the problem. Or you could augment deep neural networks with knowledge so that the system has some facts at its disposal even before it goes live.
Now put the pieces together
Leveraging AI and driving impact require many things: the right people, data, tools, infrastructure, and governance. How you use these things to accomplish what you want is the strategy. But don't expect it to be “one and done,” added Williams. Reality is a bit different.
Learn More
For more details on how to build an AI strategy for your business and more real-world examples, watch Williams’ talk, “How to Build an Effective AI Strategy for Business,” and <a href="https://exchange.scale.com/home/videos/how-to-build-an-effective-ai-strategy-for-business" target="_blank">”read the full transcript here”</a>. You’ll also hear about the history of aluminum, visit a fictional comic book universe, and meet up with blue-footed boobies.