How to Build an Effective AI Strategy for Business
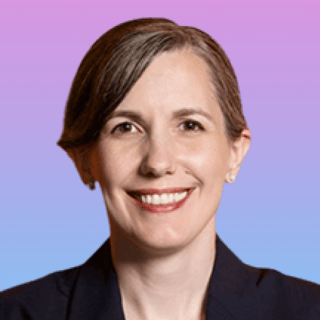
As the Global Head of iQ at Qualtrics, an experience management software platform, Dr. Catherine Williams leads the engineering and applied science teams that build advanced intelligent features into the Qualtrics experience management products and platform, leveraging cutting edge text analytics, predictive intelligence, and statistical analysis to help customers better understand and act on their data in real time. Catherine has extensive background and expertise in data science and beyond; prior to her work at Qualtrics, Catherine led data science, analytics, and product organizations at AppNexus and Xandr (part of AT&T) as Chief Data Scientist and Chief Data and Marketplace Officer. She received her BA from Grinnell College and her PhD in Mathematics at University of Washington and held postdoctoral fellowships at Stanford and Columbia Universities. Outside of work, Catherine enjoys exploring the outdoors, running, and spending quality time with her two boys.
Catherine Williams, Global Head of iQ at Qualtrics , discusses the key things that enterprises should think about, as they develop and grow an AI strategy for maximum business impact. She shares a framework for choosing which kinds of problems organizations should apply AI to, and the steps needed to operationalize AI successfully. Join this session to learn how you can best build an AI strategy for your own organization.
Nika Carlson (00:00): Next stop, we're thrilled to welcome Catherine Williams. Catherine Williams is the Global Head of iQ at Qualtrics. She leads the engineering and applied data science teams that build advanced, intelligent features into the Qualtrics platform. Prior, Catherine led data science, analytics, and product organizations at AppNexus and Xandr as Chief Data Scientist and Chief Data and Marketplace Officer. She received her BA from Grinnell College and her PhD in Mathematics at University of Washington and held post doctoral fellowships at Stanford and Columbia universities. Catherine, over to you.
Catherine Williams (00:56): Okay. Well, hello. My name is Catherine Williams. I'm the Global Head of iQ at Qualtrics. And I'm going to be speaking today about artificial intelligence strategy for business. Before I get started, let me say it is such a pleasure to be back at Transform X. I thoroughly enjoyed the inaugural one back this spring, and it's a treat to get to speak again this time.
Catherine Williams (01:18): So first let me say a note about the topic today. First of all, it's going to be a pretty high level sketch. Ordinarily, when you're thinking about strategy in business, you think about the total addressable market and the competitive landscape and so forth. But I'm planning to lift above that to about the 100,000 foot view and just try to focus on the elements that are common when you're thinking about artificial intelligence specifically. So, we can't be too precise with our applications, but we will stick to sort of a high level view. We're going to look at the forest instead of the trees. And the other note I want to make is that I am using the term artificial intelligence advisedly here. Yes, it's a jargon term and there's a lot of hype, especially in the VC world around artificial intelligence. But I also want to reflect more than just machine learning, more than just expert systems and data science. Really the super side of all of that, that comprises intelligence in software these days.
Catherine Williams (02:17): Okay. So let me get started. First a little bit about my background that helps to inform my thinking on this subject. I work at Qualtrics, which if you're not familiar, we're not small, but you may not have heard of us. We are a SaaS experience management company. So experience management is the business of designing and improving experiences for companies or institution's stakeholders. And we provide a SaaS platform and services to make that possible. Experience data means not just operational data or behavioral data, but how customers or employees or prospective customers feel right, which they may express in response to solicited feedback like surveys or unsolicited like reviews or social media, et cetera. And our platform helps our customers make sense of all of that data.
Catherine Williams (03:14): The three pillars that underpin our platform are sort of a directory customer experience directory and an actions platform and sitting in the center of it is our umbrella term iQ, which is the part of the company that I sit in, which enables us to process and understand all that data. So you can think of us as sort of the intelligent nervous system at the heart of all of that. And that helps to inform my thinking about strategy here today.
Catherine Williams (03:42): Before coming to Qualtrics, I worked at AppNexus, which is a programmatic advertising company in the business of buying and selling digital advertising. It was acquired by AT&T and then rebranded into the advertising wing of AT&T, which was Xandr. And there I built and led data science teams that built machine learning features into the platform. And before that, I was a professional mathematician, which is to say an academic and I studied general relativity and specifically the boundaries of black holes all under the gaze of differential geometry until ... And the reason why I'm bringing it up here, until I realized that I wanted to have more impact on the world. So that decision to jump into industry about a decade ago is related to what I'm talking about here today.
Catherine Williams (04:32): I've always wanted to be able to figure out how to take the tools of the trade, mathematical tools, and have a bigger impact on people and the communities around me. You might even say that with that jump from academia to industry, that it became a little bit of a chip on my shoulder. I assumed rightly or wrongly that those around me would think that I was only interested in the abstract, interesting mathematical problems at hand, and not in the business. And so I've worked hard to prove the opposite and apply my thinking to how best to leverage math or really artificial intelligence and machine learning to drive impact strategically and for businesses. So with that starting point, how do we get started talking about strategy? Well, maybe we should start where we shouldn't be. Here. With all the hype.
Catherine Williams (05:22): Everyone knows you have to have machine learning in your story, or you're not sexy. I think, it captures the moment perfectly, obviously, artificial intelligence, the shiny new thing. And one might even say that people are treating it like vibranium. Generally, vibranium is that fictional metal from the Marvel comic universe that features in Black Panther and other places. And it basically, anything you make out of it has essentially magical properties. And moreover, it actually infuses the ground and the flora and fauna nearby it in Wakanda to give them magical properties as well. And I think that's some of the attitude that we sometimes hear generally in the business community about artificial intelligence. It's just magical. But we know that that's not real. We know that there is a reality to artificial intelligence. So how should we actually think about it? Well, I really love this simple but powerful statement from Andrew Ng paraphrased here saying the artificial intelligence is the new electricity.
Catherine Williams (06:30): And while that may just sound like a cute sound bite, I think it's actually pretty profound. If you think about how electricity transformed industries in the 20th century, one by one, changing the way that they manufactured and produced and just operated generally, you can see that in the 21st century we may well be poised to do the same with artificial intelligence. It's a pretty profound analogy. So this is much more realistic then in thinking about this technology. Now, what does this mean for business? Well, first of all, we know that a lot of new companies are AI first, they're machine learning first. Just like the light bulb didn't exist until there was electricity to put inside of it, there are many companies that are only made possible by advances in artificial intelligence. But there are many older companies that were not invented with artificial intelligence in mind. And those are the ones that are going to need to be retrofitted in order to keep up in the 21st century.
Catherine Williams (07:34): So how should we think about retrofitting those businesses and their strategies in order to accommodate this technology and accelerate? Well, maybe a simple framework for thinking about that is this triangle. First of all, in order to leverage AI, you're going to need the people in the right rules with the expertise and the know-how organized to be effective. And if you want to have ethical business impact, you're going to need to think about ethics with your people and how they operate as well. This could be a whole talk in its own right. The right people in org and structures, et cetera.
Catherine Williams (08:11): What else are you going to need? What you're going to need to be able to execute, you're going to need to have the data and the tools and the infrastructure and the governance in order to be able to leverage the technology and drive impact. And that is again a topic in its own right. But today what we'll be talking about is the third leg of the stool. If you will, that is to say a strategy. What exactly are you doing with these people and this data and these tools? What are you trying to do? And I think there's a little bit of a misconception here that one can just have a machine learning strategy and artificial intelligence strategy. You go into a room and you think about it for a while and it's one and done. But the reality is a little bit different. And so I like to think of it rather than as an electricity, I like to think of it as aluminum.
Catherine Williams (08:57): Okay. Why aluminum? Well, the parallel here is that no business, I think, ever went off and had an offsite and said, "What should our aluminum strategy be?" You know? No. On the contrary, businesses use aluminum to solve specific problems at hand. They may use it for building because it's lightweight and non-conductive, or they're unplentiful and cheap, or they may use it for aeronautics because of its properties. They may use it for cans, for whatever reasons. I don't know what reasons went into this, but in each case they're solving specific problems and aluminum happens to be the solution. It's not an all up one and done.
Catherine Williams (09:40): Now, a couple more notes about aluminum before I go on, first of all, I've used this metaphor many times before, but this is the first time that I put the chemical symbol of aluminum on a slide. And only now did I notice that it looks like AI, which is very appropriate, so that's kind of cool. The other note I'll make, the other interesting parallel is that aluminum when it was first discovered, I think in the 18th or 19th century was thought to be very rare and very precious, more so than gold, so much so that the French Royals had bars of it made, and they held it next to the crown jewels because it was so very, very precious. Until sometime in the 19th of injury, they figured out how to produce it industrially and then it became very, very cheap and plentiful as we know it today. So just like machine learning originally thought to be very unique and special and valuable, now increasingly ubiquitous.
Catherine Williams (10:31): Okay. So maybe by now, I've convinced you to think of your machine learning and artificial intelligence strategy in terms of aluminum and what are the problems it's going to solve, not what is the technology that you're going to bring to bear first? Well, how do you get your head wrapped around? What kinds of problems to even be thinking about? Where do you start? I've discovered over the last few years, this helpful framework that I think provides the two key dimensions for thinking about how you might be able to leverage this technology. And these dimensions are: one, are you seeking to create knowledge for humans or are you seeking to create an action, some sort of a task? That's one that's a horizontal dimension here and are you intending to have a human in the loop or are you intending to be automatic?
Catherine Williams (11:22): And of course these are both spectra and there are different points in between. But let's talk about what this means in terms of what your applications might look like. In the top left corner, if you want to generate knowledge and you want to have a human in the loop, you're basically talking about analysis tools. What machine learning technologies can humans use in order to generate more knowledge, that's a toolkit. Bottom left, if you want to automatically create knowledge, that's where you're going to be creating insights, right? Insight generation. Over on the right hand side, when we're talking about tasks or we're focused on a task or a next step of some kind, up on the top right, we can suggest tasks or suggest actions or make recommendations. On the bottom right, we can be talking about completely automating the tasks entirely. And somewhere in between there's task assistance.
Catherine Williams (12:14): So the vertical spectrum on the right hand side is about how much the human has to do in the loop. Whether they just click one button or whether they maybe make, do a lot more in terms of what taking that action. But either way, it's focused on at specific action. So this is the frame work that I would post it is useful for organizing thinking about where and how you might be able to leverage artificial intelligence in your business. And by the way, let me just say this framework can apply both internal to a business, thinking about it in terms of like decision science, potentially enabling internal people, internal to your company, to do their jobs better, or be more effective. Or if you are in a SaaS company like ours, providing software to customers, all these same pieces can apply in the software that you're providing. And that's the case for Qualtrics.
Catherine Williams (13:09): So let me start here then by giving a few examples of how this lights up in practice. I'll start with some examples from Qualtrics and the iQ platform. First off is our Stats iQ product. This is a stat, statistical tool built into our platform. Think of it as a user friendly SPSS to enable our clients to make better sense of the data that they're collecting. Their experience data. So, from very simple things like regressions to correlations and cluster analysis, et cetera, it's a machine learning toolkit and statistical toolkit to help generate knowledge.
Catherine Williams (13:47): We also have a text analytics feature set called Text iQ, which similarly is a tool, but it leverages machine learning to help understand the unstructured text. Let's say, survey verbatims that are coming in or whatever else that might be classifying sentiment and topics, and potentially emotion and actionability and other things in service of creating knowledge, but certainly with a human in the loop to help define what those topics should be, et cetera. Over on the bottom left, mind you, we also provide some insights automatically to our customers. One such is anomaly detection. Our customers who maybe are running big survey programs, who want to know if response rates have dropped precipitously or are spiking for some reason. And that's something that we can detect and let them know right away automatically. Or if they're measuring a business outcome like a customer satisfaction or net promoter score, and that precipitously drops, they may want to dig in to understand. And so that's an insight that we can generate automatically on their behalf.
Catherine Williams (14:52): Similarly, we provide services under the umbrella of Driver iQ, helping our customers to find insights into what's causing their net promoter scores, to be what they are, et cetera. So providing those insights to our customers. And we do similar things internally. What's driving our customer behavior? We dug through in our own platform here. So this is the kind of automatic insight, automatic knowledge that machine learning can help to generate.
Catherine Williams (15:21): Now over on the right hand side, I mentioned that Qualtrics has a workflow orchestration part of its platform. And we have wired that up to connect to our Text iQ feature set. So portmanteau of X flow, our action platform with Text iQ TextFlow so that our system can understand when say, a survey response comes in, for instance, that has maybe a keyword that's legally problematic, or a negative sentiment or some other aspect that we're able to extract with natural language processing, and then tie it to maybe creating a system of some kind. I mean, a ticket in some system, or maybe it sends an email or maybe it flags in some other way, causing an action to happen based on this machine learning system.
Catherine Williams (16:12): So those are some examples from Qualtrics, but it's sort of a fun game to think about what other examples might be from industry more broadly. And I've picked a few that I think are illustrative. First of all, back up in the top left quadrant, just any kind of toolkit that leverages machine learning sits over here. So think SPSS or Mathematica, or what's now Wolfram I believe, or any kind of tools that enable a human to dig into data and generate insights. There's a whole broad category there, and I've just reflected it with one dot. Over on the right, however, this is a little bit more, maybe a little newer thinking about next best action. So these models recommend real time actions for say, sales or service folks when they're interacting with a customer or a potential customer, like recommending that here's a troubleshooting step or now might be a good time to offer a gift certificate or whatever it might be. The next best action for that customer relationship.
Catherine Williams (17:12): So it's action oriented, but there's a human in the loop who's ultimately going to make that decision. And more broadly you can think about the whole category of personalized recommendations, like the original Netflix movie recommendation engine, or anytime you're using personal data or some sort of collaborative filtering to recommend to someone an action that you think will be well suited for them. Moving down a little bit to a little bit more towards the automatic, because there's a little bit less human involvement is say, auto complete. This is maybe a simple example, but it's an interesting one. You know, if you're typing along, sometimes a machine learning system can say what they think how you should complete that thought or how you should finish the sentence. And it's just a one key stroke to actually do that completion, which is why it's a little further down at my spectrum. I should say, by the way, that all of the circle placement in these quadrants is that definitely more art than science and I think reasonable people could disagree about exactly where they're placed on these two dimensions.
Catherine Williams (18:22): Moving down further is autocorrect. So think about this. This is where the system does something for you automatically, but you as the human do have an opportunity to go back and undo. And I undo autocorrect all the time. So there's a opportunity for the human to join the loop, but they don't have to, it just ho happens automatically. But maybe some more interesting examples of automatic actions come from programmatic marketplaces. This is my background where we built algorithms that would do real time bidding based on machine learning to determine which ad impressions to buy on behalf of a given advertiser or similarly how to set floor prices if you're a publisher wanting to monetize your advertising, your inventory, your digital inventory. And there are examples of other programmatic marketplaces, obviously financial ones. Think about Wall Street and all the programmatic bidding that happens there as well. And maybe others where there's not even an opportunity for the human to be in the loop. The bidding and the buying just happens autonomously. And maybe you can go back and correct it or audit it later.
Catherine Williams (19:29): Another example from my background is load shedding. Now this is usually meant to refer to power systems like electrical grids, that shed load so as not to take down the whole power supply. That is to say they cut out demand for electricity so as to protect the power supply, when there's an imbalance. We use to it in the programmatic marketplace, because by the time I left, we were seeing well over a hundred billion impression requests per day, and our systems could not run that many auctions concurrently in real time. And so we would use machine learning to figure out which ones of these impressions are not likely to get any bids whatsoever or are not likely to generate revenue for their publishers. And we would just cut them out of priority and protect our system that way.
Catherine Williams (20:18): And finally, moving back over to the left hand quadrant to automatic insights, automatic knowledge generation, there's lots of applications here. The whole branch of say, decision science can be thought of sitting in this category. For example, risk management think insurance agents or credit card companies that want to be able to get deep insights into how credit worthy someone is or what an insurance premium should be. This is all knowledge that's informed by machine learning largely without a human in the loop. And generally all kinds of forecasts can fall into this category as well. Whether it's revenue forecasts, we had a marketplace model that forecasted the supply and demand matching at AppNexus for our marketplace that was machine learning based. And there are all kinds of other things you might want to forecast like consumer demand or weather and all of these things can be powered by machine learning.
Catherine Williams (21:16): And finally, I've placed one dot right in the center, the digital marketing and advertising world, which has numerous applications that are email powered. And they sit in different parts of this picture. So I've located the dot right in the middle. On the one hand, a lot of times advertisers or their proxies will mine customer data to understand what segment, what's their behavior, what segments should I put them in? How should I think of how customers are responding to my advertising? They're generating knowledge using machine learning. But then they turn around and plug those straight back into buying platforms like the one that we had at AppNexus to do programmatic buying. So there's both knowledge and action bouncing back and forth sometimes with humans in the loop and sometimes less so.
Catherine Williams (22:02): And that's a paradigm, I think, you'll see lots of times in more complex businesses that there's not just one application of artificial intelligence, but multiple ones in multiple quadrants that bounce back and forth and inform each other. And maybe that's a good way of thinking about sort of a desired end state is to have pieces in each quadrant that can inform each other. If you think about maybe one of the most exciting applications of artificial intelligence in the last 10 or 20 years, think about autonomous vehicles, right? Obviously they're about automatic action, but if you look under the hood, so to speak, there are subsystems that are generating knowledge in their environment, that they then feed back to different parts of the models that decide on the actions. And I think that's a good sort of paradigm for thinking about complex business use of artificial intelligence.
Catherine Williams (23:03): Okay. So having explored this quadrant, these quadrants a little bit and provided a framework for thinking about what problems artificial intelligence could potentially help with in your business, whether internally or on behalf of customers, is that it? Is this all we need to think about? Well, no. Surely we're just scratching the surface. But I want to show you a few more dimensions of opportunity to maximize the business impact of artificial intelligence. And so I'll land here in biology, right? We started off in a fictional comic book universe with vibranium, moved to physics with electricity, went to material science with aluminum, and now we're in the natural world with biology. And I'll use these as my metaphors. So the first dimension for maximizing impact is kind of obvious, but you really do have to get traction in your specific problem and you have to do so rigorously.
Catherine Williams (24:02): You can't fake it. Here in this xkcd comic, they're just stirring the pile until the answers start looking right. But long term, that is not what's going to drive strategic value. Kind of obvious, but feels like it's important to say anyway, don't do this. The reason I'm using this picture here is because my metaphor is that no matter what, you're going to have to photosynthesize. You're going to have to do the business of turning the data into something of value.
Catherine Williams (24:31): Okay. So you've gotten started. What's the next dimension that you could potentially maximize? Well, it's always useful to acquire more data. Here, you see a blue whale, who's eaten up a lot of krill, krill like the data here, it's always good to have more. We've learned that from watching ImageNet in computer vision and the large language models like GPT-3 more data is good.
Catherine Williams (24:56): So how can you acquire more data? Well, an obvious answer is to instrument to collect more. Can you just collect more data from whatever environment you're in? Or be creative. Can you buy your partner for additional data that could help accelerate your impact. Or even can you create or create synthetic data or run around and do something by hand. These are all options for sort of maximizing the data that you have available to drive. Whatever problem it is. What else? Well, another dimension is to extract more signal from your existing data. And my image here is of a Panda. Pandas are genetically and evolutionarily carnivores actually, but somehow they have now evolved, eat bamboo exclusively, and their guts have special flora and fauna in them to be able to consume that bamboo, to digest the cellulose. And they extract every last bit of nutrition out of that bamboo to replace what they're not getting in terms of meat.
Catherine Williams (26:06): So like the panda, I argue that we should try to extract every last bit that we can from the data that we do have. This is where the deep neural network revolution has been so powerful lately, I think. Enabling us to get much more signal out of say, images and audio and video and natural language than we had previously. So lots of interesting alignment with research there. But it's not just about deep neural networks. Also think ensembles in our programmatic marketplace at AppNexus. One of the things we needed to do was try to root out bots and fake publishers and bad advertisers, all of whose incentives were different than ours, and they wanted to scam our systems. And there's no way to get a supervised system there. There's no way to get a smoking gun, but we learned over time that by generating a whole lot of sort of weak models, an ensemble of weak models about the different say, user or advertiser behavior, we could generate a lot of insight and knowledge and actually keep the platform pretty fraud free.
Catherine Williams (27:12): And I've included an ellipses here just to reflect that, I think, there's lots of ways and lots of creativity that you can bring to bear, to extracting more signal from existing data to accelerate impact. And finally, and maybe most excitingly is this dimension, to contextualize. Now, what I mean here is to really look for the elements of the specific problem at hand, to be able to find tune and make more precise or more impactful the solution. I'm speaking very high level here, of course, but this could take the form of something as simple as like developing a schema or an anthology for the specific problem at hand. Or in the context of deep neural networks, perhaps to augment with knowledge so that the deep neural network system has some facts already at its disposal. Or if you're talking about say, something in the physical world, there's physics-informed machine learning that can take the best of both PDE-type physical system modeling and machine learning and pull them together.
Catherine Williams (28:23): So, all these to say, you want to be like the blue footed booby of Galapagos Island that adapted to its very specific context in order to thrive and figure out what elements from the specific problems that you've identified, you can bring to bear as well to create the best product market fit or the best value or whatever that is. And this too becomes a strategic moat, that very, very tight and fine adaptation. And once again, I've included the ellipses because I think this is a probably a very long list of interesting techniques for adapting to a specific problem and contextualizing. And so with that, I will just say, I hope if you've learned nothing else today, it's that you can think of artificial intelligence's strategy for business as not vibranium, but electricity or aluminum or photosynthesis or blue whales eating krill, or pandas eating bamboo or blue footed boobies, adapting to their environment. Thank you so much for your time.