How to Build an Enterprise Data Framework
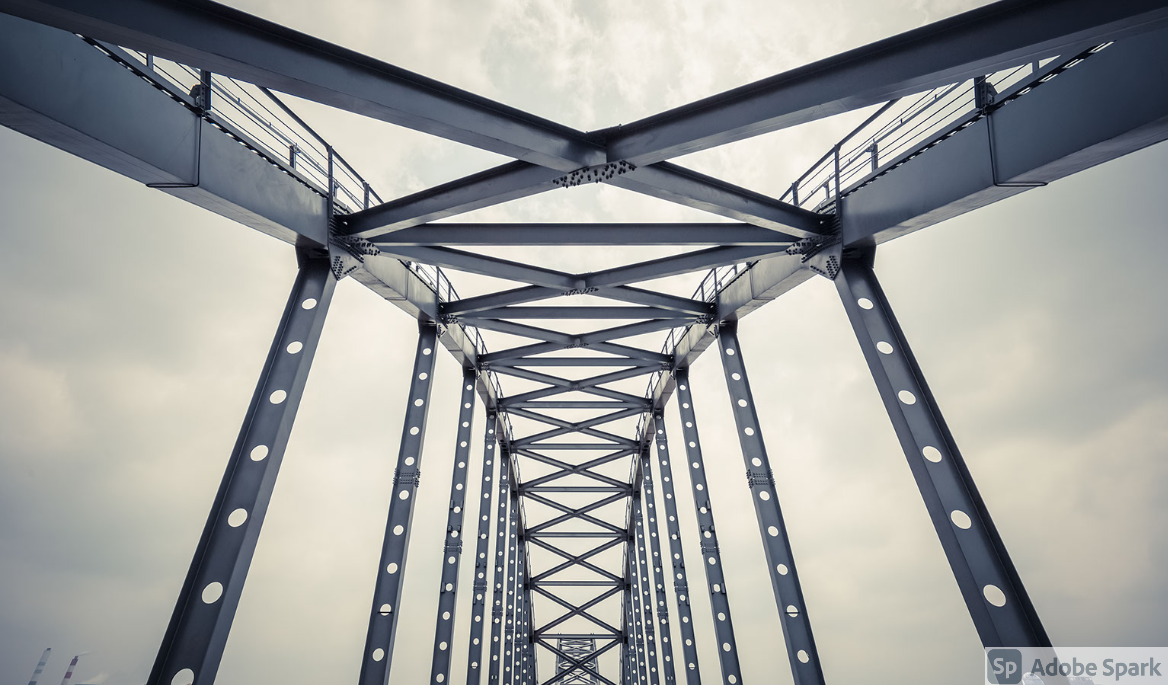
To achieve actionable insights from AI, you need a solid data framework, says Etsy chief data officer Chu-Cheng Hsieh
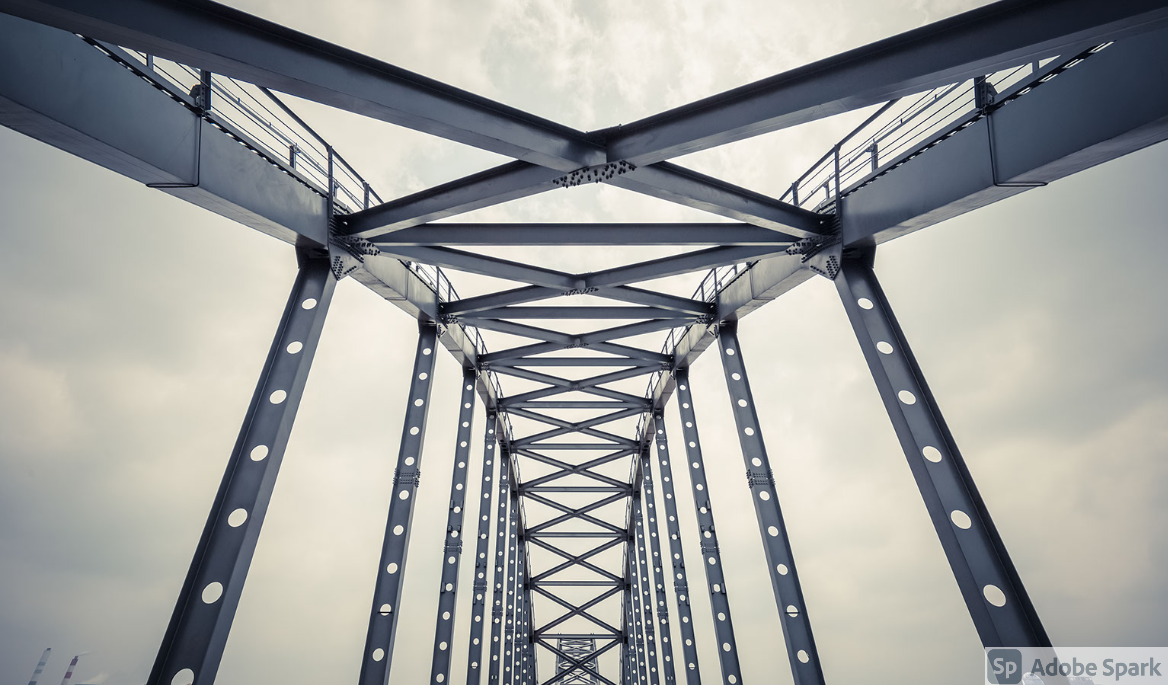
Over the last two decades, enterprises have learned to deal with ever-increasing amounts of data. Using “big data” involves building or acquiring the tools, technologies, processes, and skills required for large-scale historical or retroactive enterprise reporting on large datasets.
Thanks to advances in AI, it is now easier than ever for many enterprises to gain predictive insights through models trained on their own data, said <a href="https://twitter.com/chuchenghsieh" target="_blank">”Chu-Cheng Hsieh</a>, chief data officer at Etsy. However, the volume, velocity, and variety of many enterprise data systems makes them increasingly complex to manage.
Hsieh spoke on the topic recently at the <a href="https://scale.com/events/transformx" target="_blank">”TransformX 2021 conference”.</a>
<br> <div style="position: relative; padding-bottom: 56.25%; height: 0;"><iframe src="https://fast.wistia.net/embed/iframe/vuwryy5pzx" frameborder="0" webkitallowfullscreen mozallowfullscreen allowfullscreen style="position: absolute; top: 0; left: 0; width: 100%; height: 100%;"></iframe></div> </br>AI models can be highly susceptible to changes in the data used to train them, he said, so a multitude of controls and automation are often needed to ensure that AI models are trained with correct and relevant data. A clear and prescriptive framework is often required to implement those controls and ensure that any insights derived through AI are timely, auditable, and high quality.
Etsy uses data to create a more personalized experience for shoppers. Real-time data collection about each customer interaction allows for personalized product recommendations. It also provides insights about how the Etsy website can be optimized to increase customer engagement.
Detect and fix poor data quality
High-quality ML predictions require high-quality data, so enterprises must prepare processes and mechanisms to measure and manage data quality. According to the <a href="https://hbr.org/2016/09/bad-data-costs-the-u-s-3-trillion-per-year" target="_blank">”Harvard Business Review”</a> poor-quality data cost enterprises $3.1 trillion in 2016. Quality checks are of the utmost importance when collecting raw data and should be a key part of any data framework.
Let your models create useful (and reusable) datasets
ML can also enhance the reusability of data, said Hsieh. Neural networks can be used to create embeddings, which are low-dimensional representations of input data.
Embeddings are useful representations of enterprise data that can be reused and are often stored in feature stores, but creating embeddings at scale is often computationally intensive. Feature stores serve as a central enterprise repository, so embeddings are readily available for reuse in new model development.
What should your data be telling you?
Before establishing a data framework, an enterprise should understand what kind of insights it expects from its data, and those should be closely related to broader business objectives. One of Etsy’s business objectives, said Hsieh, was to boost customer engagement and sales through a recommendation system.
An insight to support this objective could provide an understanding of customers’ individual preferences. What item is a customer most likely to engage with or purchase? What data does Etsy have that could help predict a future customer choice?
Automate testing in your framework
If your enterprise is collecting data, you probably already have a framework of some sort in place. Optimizing this framework is key to maximizing the value an enterprise can get from its data.
However, the ecosystem of tools, processes, and integrated partners may frequently change. As a best practice, enterprises should revisit each layer of their framework to find opportunities to optimize it further.
Another business objective at Etsy is to continuously improve the way it processes data. <a href="https://en.wikipedia.org/wiki/A/B_testing" target="_blank">”A/B testing”</a> can help analyze whether a new feature has any predictive qualities. For example, you might want an answer to the question, “Does this feature increase the number of people who sign up?”
You can use ML to recognize and respond to important patterns such as back-office operations or customer behavior, said Hsieh.
Build a layered framework
In his presentation, Hsieh described how the foundational layers of the data framework contribute to data preparation. The practice of data preparation is not about just collecting data—not all data is immediately useful. Raw data often has limited value. It requires manipulation, filtering, and often enrichment to be useful.
Extracting value and insights from data often hinges on thorough, automated, and clearly defined data preparation, which itself requires a rigorous framework.
Furthermore, in a data framework, all data should be considered in the context of its useful life. A dataset may be retired if it becomes outdated or is no longer needed; you can archive or even delete it.
Involve your stakeholders
You need an understanding of the data framework across all levels of your organization, including by business executives and other stakeholders. Having business executives understand this framework creates trust in the process. This fosters an understanding of how patterns are recognized and insights are formed through data and ML.
Data frameworks also need to be flexible by design, to maintain compliance with ever-changing regulatory and compliance requirements.
Learn more
For more details how to build a useful data framework, watch Hsieh’s talk, “Rethinking The Framework for Data,” and read the full transcript here.