3 Areas Where AI Is Making a Difference in Healthcare Research
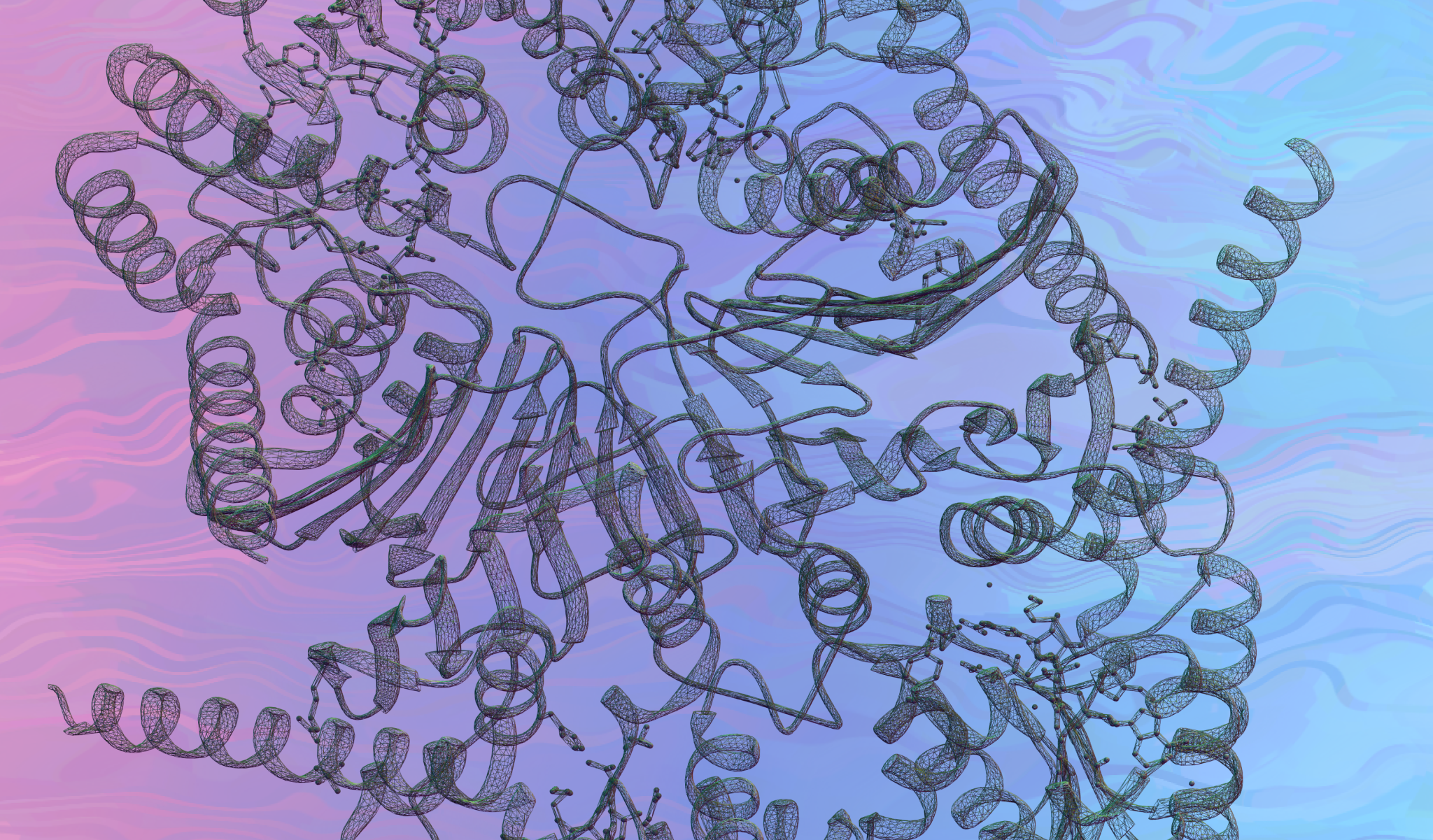
ML and AI are making scientific research in pharma, biology, and medicine more systematic, rigorous, and efficient.

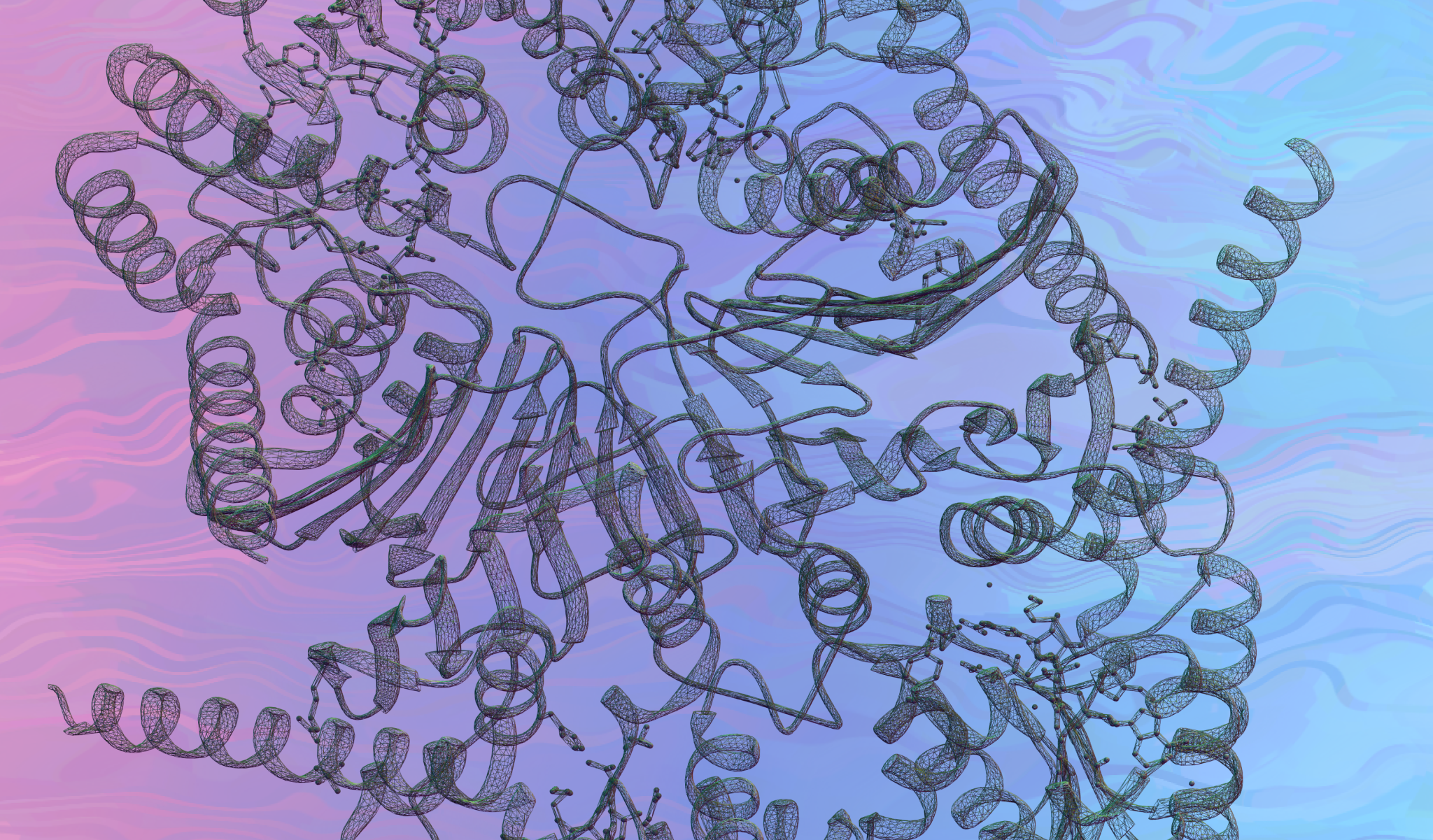
Artificial intelligence has already affected research in the health sciences, but several key scientific areas, including medicine and pharmaceuticals, stand to benefit from AI and machine learning (ML) even more than they already have.
That’s just one of the many takeaways put forward by Nathan Benaich and Ian Hogarth in the State of AI Report. Benaich is a general partner at Air Street Capital, a venture capital firm investing in AI-first technology and life science companies, and Hogarth is an angel investor.
AI will become part of the fundamental toolkit for scientific discovery, Benaich predicted. “All the workflow in science—generating an idea, designing an experiment, running the experiment, analyzing the data, figuring out how that changes your hypothesis, rerunning the experiment—all that can be rethought to work with very powerful assistive technology,” he said.
Many scientific domains require the application of rules to large amounts of data. That makes them ideal candidates to reap the benefits of ML and AI, which are helping scientists discover and innovate faster and more economically than ever before.
“AI more and more is viewed as a tool that amplifies the scientist, as opposed to a tool that replaces or does the job of the scientist,” said Elliot Branson, director of ML and engineering at Scale AI. (Branson recently discussed the impact of AI on healthcare research with Benaich during a fireside chat on the State of AI Report.)
“It’s not going to tell you an answer that’s perfectly correct,” he added. “It works best when you have a strong set of intuitions, a strong knowledge of the field.”
Here are the key areas where AI and ML will have the greatest impact.
The Development of Artificial Proteins
In biology, proteins are the product of evolution, but with AI, researchers can generate artificial proteins with useful functionality beyond evolution’s design. AI can generate proteins unseen in nature by predicting the next amino acid for more than 280 million protein sequences from thousands of protein sequences.
Proteins are composed of amino acids, Benaich said. Each amino acid has a role to play in a sequence, “much in the same way letters play a role in words.” But instead of feeding strings of letters, machine learning is feeding strings of amino acids into similar natural language processing models, he added.
Because there are “relatively simple rules” that describe how proteins and molecules form and interact with one another, you can turn it into a deep learning problem, “where they can try to come up with things that satisfy those rules but haven't been seen before," Branson added.
For example, to discover a protein with XYZ properties, data on how proteins work can be fed into a model that is then told to fit the proteins together until it has one that fits those properties. “Anytime you can turn a scientific process into a numerical, rules-based process, there's a high chance that you can apply deep learning to it," Branson said.
Using Convolutional Neural Networks to Enhance Protein Therapy
AI is a better way to run simulations in biology than how the process traditionally works, Benaich said. You can run an increasing number of experiments in the computer that you would have had to run in the real world, so “by the time you actually do real-world experiments, you’re doing them on ideas much more robust and substantiated by evidence. You’re doing less trial and error in the real world and more trial and error in the computer."
AI can be used not only to identify novel proteins, but to design protein therapy, too. For instance, treatments for inflammatory bowel diseases, such as Crohn’s disease and ulcerative colitis, need to both inhibit inflammation and survive traveling through the GI tract. That requires increased potency and stability in the presence of protease.
Using convolutional neural networks, researchers have designed proteins that increase potency 400-fold and improve protease stability 100 times compared to molecules designed by traditional methods.
Medical science has also been an important domain for ML and AI. Natural language models are predicting the evolution and emergence of escape mutants in viruses. A virus develops escape mutants to evade neutralizing antibodies in a host’s immune system. As seen by the emergence of the delta variant of COVID-19, that can impede the development and effectiveness of vaccines. By using language models to better understand how a virus generates sequence diversity, researchers can reduce its ability to neutralize therapeutic antibodies.
"Instead of waiting until a mutation happens and then designing a vaccine for it, if you know what mutations might occur based on those that haven’t occurred, you can start designing an mRNA [vaccine] for it," Benaich explained.
Better Testing, Better Treatments
Reinforcement learning has been used in another medical area: testing. One of the few real-world deployments of AI to address the current pandemic is a system called Eva, which was created in Greece. Using reinforcement learning, the system chose from among the travelers entering the country those who should be screened for COVID-19. Eva identified up to four times as many positive infections at a given testing facility than random selection did.
Computer vision also has proved to be a valuable technology for medical science. For example, it’s being used to select the most potent drug to improve survival rates of cancer patients.
However, AI-based microscopy can be used to measure how live cancer cells respond to 140 clinically approved drugs at the single-cell level. In a prospective clinical trial of 56 blood cancer patients, those patients who received AI-guided therapy achieved a 55% overall response rate and a statistically significant improvement in progression-free survival over their prior therapy treatments.
In the same vein, AI can also enhance the efficiency of predicting and prioritizing novel drug combinations, dosages, and timing for therapy. While combining drugs can improve cancer patient outcomes, empirically testing large numbers of combinations is not feasible in a lab setting. By using an ML technique called self-supervised learning, the behavior of cells treated with a finite number of drug combinations can be leveraged to predict the effects of unseen combinations.
“AI can help pharma companies ultimately synthesize 10 times fewer drugs compared to the industry average before selecting one that they’re happy with to move into clinical studies," Benaich said. Not only does that save time and money, but it allows them to “advance candidates that they’re more confident will work.”
A Fundamental Component of Scientists’ Discovery Toolkit
Research, generally, can be improved through AI, Benaich added. A few years ago, he recalled, a study showed that 70% of researchers have tried, but failed, to reproduce another team’s experiments. More than half couldn’t even reproduce their own work.
ML is a good solution to that problem because it makes scientific discovery and development “much more systematic,” Benaich said. "We're at the point where running these experiments is incredibly expensive so you have to have a strong thesis for what you're going to get before you run an experiment," he added.
AI can be used to find pockets of knowledge that science doesn’t know much about but that have promise that something interesting is there, he explained. Then humans will need to confirm what’s in that pocket.
All told, Benaich said, AI and ML will help address scientific problems “in much more systematic and rigorous ways."