5 Ways AI Is Changing Healthcare
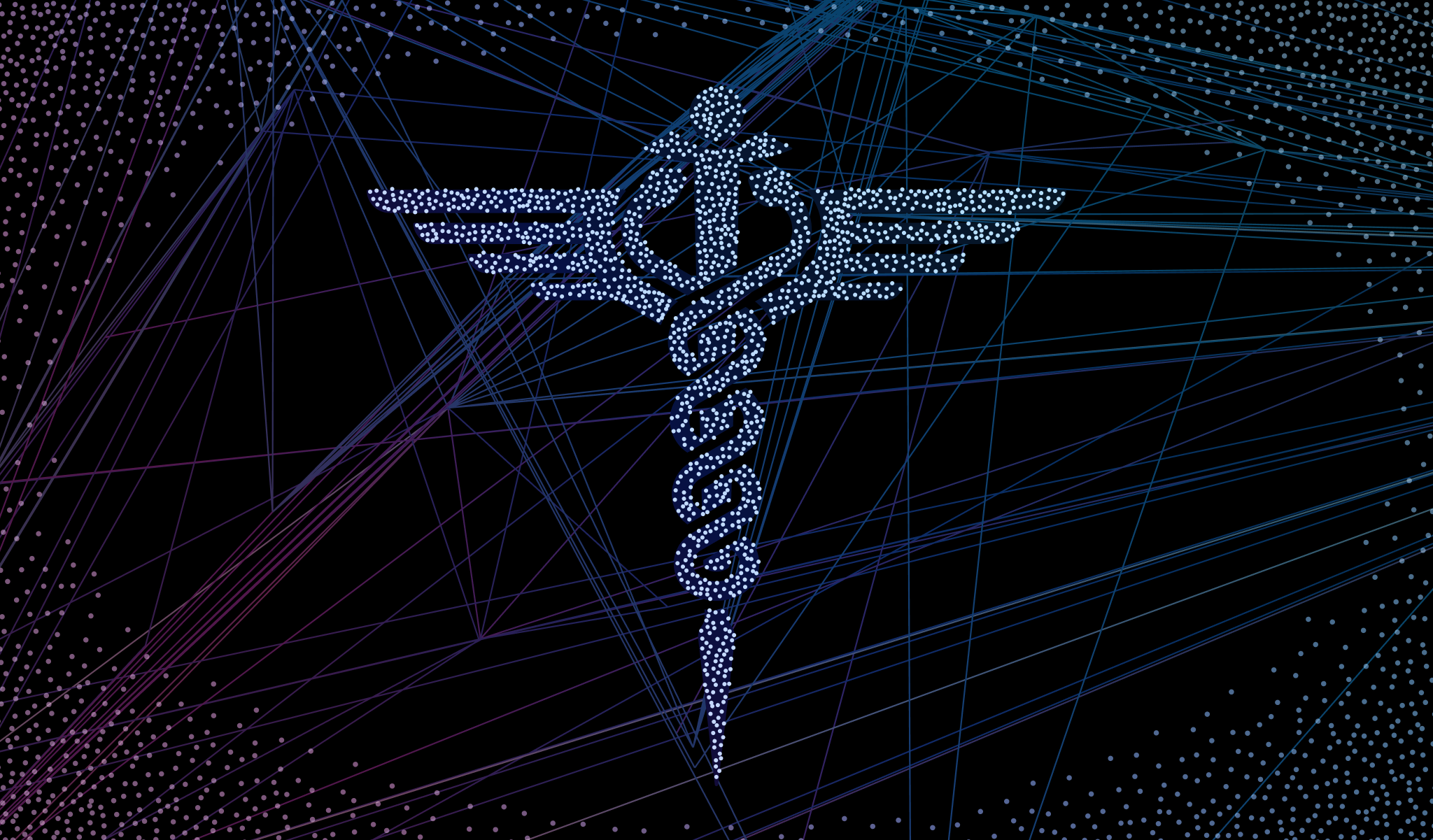
AI is driving drug discovery, fraud detection, and more in the healthcare industry.
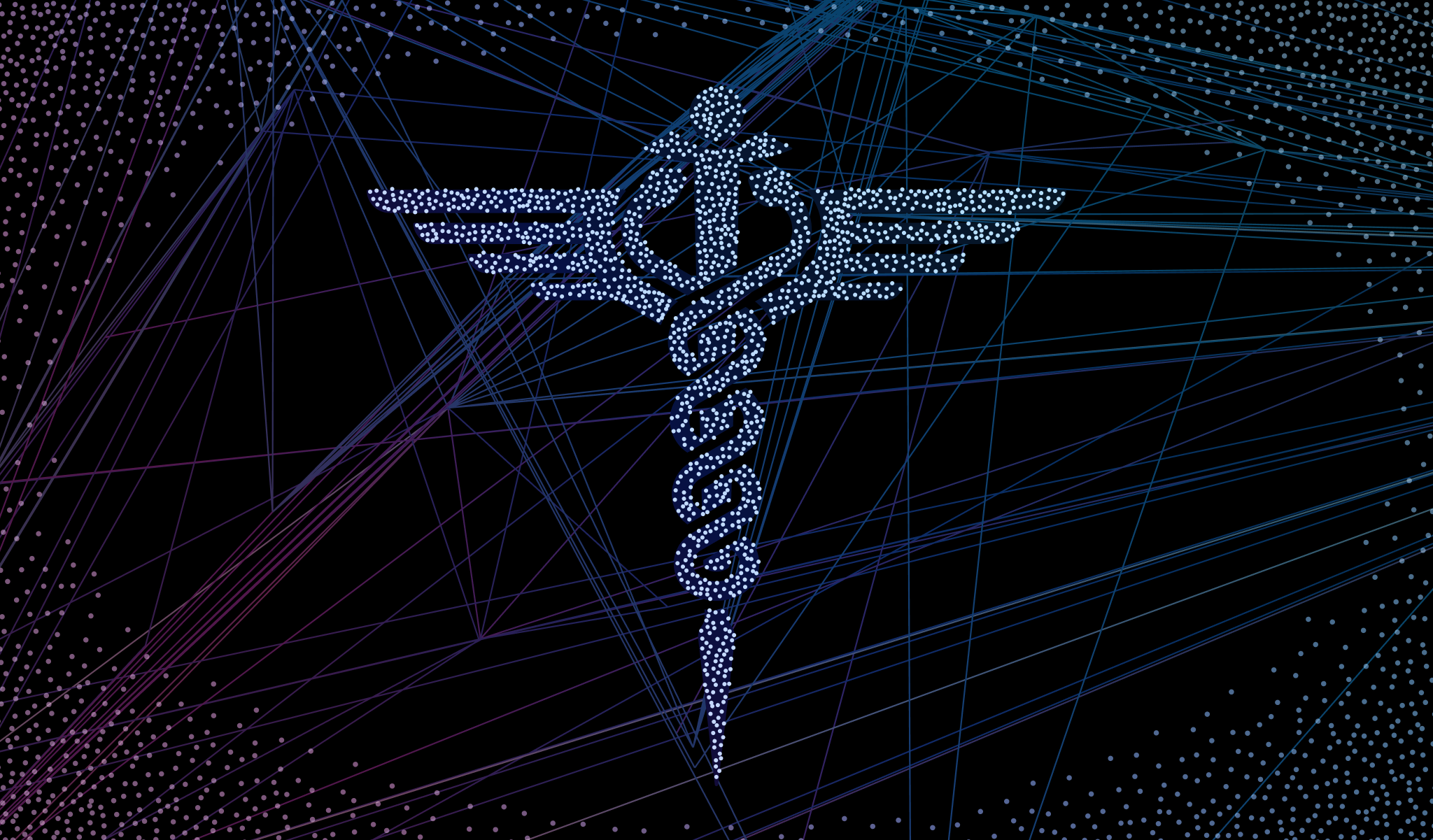
Every step in the healthcare process—from the development of new treatments to assuring proper payments to tracking patient outcomes—involves massive amounts of data. When that data can be properly gathered and analyzed, artificial intelligence can help reduce the time and cost of drug development, cut red tape, detect fraud, and supplement human therapists. Here are five examples of how AI is changing healthcare now, and what the future holds.
Drug Development
Development costs for a single drug can mount into the billions of dollars—and more than 90% of such development efforts fail. That is why identifying new drugs, pinpointing potential targets for them, and predicting their effectiveness is one of the leading uses of AI in healthcare.
Drug development is a “very complex process” that spans the identification of the proper patients and target treatments through creation of the drug, clinical trials, manufacturing, and marketing, said Daphne Koller, CEO and founder of Insitro, a company focused on drug discovery through machine learning (ML).
Large pharmaceutical companies have primarily used AI in the later stages of the process, she said, such as for improving manufacturing processes or for better targeting of information to clinicians about a treatment. Recently, the focus has turned toward the use of AI in creating and securing approval for medications, Koller added.
Among the many uses of AI in drug development cited by Nathan Benaich, coauthor of the State of AI Report and general partner of Air Street Capital, are using language models to predict how viruses may mutate, analyzing electron microscopic images of molecules or proteins to aid drug development, and prioritizing which drugs are most likely to bind with a protein to help treat a disease.
Another emerging use of AI is to understand differences among patients. For example, Koller said, “breast cancer is a collection of different causes that cause the tumor to grow. This creates a tremendous opportunity to understand the different causes and create treatments that will effectively target each.”
Mental Health
Looking to ease the effects of a global shortage of mental health counselors and the cost of such care, AI-enabled wellness app developer Kai has created an AI-powered chatbot for teens and young adults that acts as a 24/7 companion designed to help them ease conditions such as anxiety, depression, and sleeping disorders.
The chatbot encourages users to take an active role in their well-being, engages them with personalized questions, and provides insights and feedback based on their interactions with it. Rather than replacing a human therapist, Kai is best at providing ongoing, easily accessible support and assessing a user’s psychological well-being, said co-founder and CEO Alex Frenkel. It can also detect extreme distress and suicidal thoughts and direct users to humans who can help, he said.
The chatbot prompts users, “Tell me how you’re feeling. Tell me something good that happened today,” or asks what they have learned about their responses to the issues they are facing. It responds with comments such as “I’m so sorry to hear that,” or “You sound angry now—am I understanding that right?” Over time, it asks users to identify which areas they want to improve in, such as relationships, communication, or becoming more positive.
It also provides a history of their progress toward their goals and summaries of the topics, such as relationships or communications, they have mentioned most often. Based on its training on gigabytes of conversations, Kai personalizes its responses to each user.
While its developers expected that the more humanlike Kai is, the more its users would trust it, they found that the young users who are its target audience trust Kai more because they know it’s not a person and will not judge them. Some respond to it, Frenkel said, with comments such as “You are the best bot. You really care about me” and “You are like a real friend.”
Kai has not yet conducted full clinical trials of the chatbot’s effectiveness, Frenkel said, but self-assessments by users show “impressive levels of ongoing engagement and improvements.”
Fraud Detection
In 2021, fraud, waste, and abuse cost U.S. healthcare systems $380 billion, a figure that’s projected to rise to $600 billion in the next few years, said Musheer Ahmed, CEO and founder of Codoxo. Reducing those losses by proactively identifying questionable billing is his company’s mission.
The company aims to use AI to move beyond current rule-based fraud-detection methods that rely on knowledge of existing fraud schemes, he said. People who want to defraud the system are resourceful and creative enough to circumvent such systems, he said, and can cost a health plan “millions or tens of millions of dollars until someone stumbles on the scheme and mitigates it.”
Codoxo’s proprietary technology combines rule-based pattern recognition with AI to analyze data from a wide range of healthcare providers and identify behavior patterns from individuals, providers, provider networks, and health plans, said Ahmed. This analysis can identify unusual behavior and potential fraud, waste, and abuse earlier and more accurately than can traditional techniques, he said.
Codoxo’s AI technology combines supervised learning, which relies on known examples of fraud, waste, and abuse, with unsupervised learning that can identify new suspicious patterns but cannot explain what is unusual about them, said Ahmed. The technology, while based on unsupervised learning, adds the ability to explain itself. “If you can’t understand the decision process for AI, it’s very hard to take action on its recommendations,” Ahmed said.
Codoxo uses this information to proactively reach out to providers filing questionable claims with guidance on proper techniques. “The bad folks get a quick signal that we are on to you,” Ahmed said.
Ahmed claims his clients have seen a greater than 20-to-1 return on investment, with Codoxo’s technology identifying problematic claims that other techniques missed.
Outcome Tracking
Clinicians and pharmaceutical companies need to know which treatments work best to guide their care. But “healthcare now uses only a fraction of the data at its disposal,” said Dan Riskin, founder and CEO of Verantos, which uses AI and other techniques to provide “real-world evidence” gathered during routine care for clinical, regulatory, reimbursement, and other purposes.
Data on outcomes is limited, he said, because documentation of patients’ conditions, their treatments, and their outcomes is fragmented between unstructured data, such as physicians’ notes, and structured data, such as claims from providers and death registries. Verantos uses AI to scan these varied data sources for a more complete understanding of each patient’s medical history, treatment, and outcomes.
For example, one study required tracking only patients who were suffering their first heart attack. In more than half the cases, the only records that indicated that a patient had suffered an earlier attack were doctors’ notes, Riskin said. AI techniques examine those records for abbreviations such as “MI,” which stands for “myocardial infarction” (the medical term for a heart attack), as well as pattern recognition to identify terms such as chest pain and EKG, which might suggest a previous heart attack.
“That’s a lot of very important content,” he said, but technology such as AI is required to accurately draw the required information out of very large quantities of records. “This has been done manually for almost a decade in oncology studies, but these dealt with smaller volumes of cases,” Riskin said. “If you have 1,000 patients with 1,000 pages of records each, asking people to read 1 million pages is infeasible and not very accurate.”
Appointment Scheduling
Anyone who has struggled to make an appointment for medical care knows how frustrating it can be to call and be put on hold. The process becomes only more stressful when a patient with a serious condition is trying to coordinate schedules and appointments among multiple caregivers.
Notable’s web-based Intelligent Scheduling platform aims to eliminate the need for phone calls to make appointments, notify patients of changes, and perform other scheduling issues. As well as allowing patients to schedule appointments based on their needs or referrals, it automatically reminds them about details such as the need for a referral or prior authorization before requesting an appointment, said brand and communication lead Gregory Kennedy.
Its use of ML, optical character recognition, and natural language processing helps Intelligent Scheduling automate complex tasks, such as interpreting medical records, routing patients to the right type of visit with the provider in the right location, and scanning insurance cards and clinical documents into an electronic health record, Kennedy said.
Data Is the Challenge
As in other industries, the availability of high-quality, unbiased data is a significant challenge for its use in drug discovery and healthcare.
“You’re never going to be in the same position as machine learning from images on the web, where you have 100 million images to train the system on,” Insitro’s Koller said. Medical data is “often in little silos,” she said, and even when you do have access, the data is often not curated with ML in mind.
Large portions of the U.S. population “have been excluded from medical AI training data sets in radiology, ophthalmology, dermatology, pathology, gastroenterology, and cardiology,” said the 2021 State of AI report co-written by Benaich. As a result, AI-generated models used to develop treatments may be less effective for those groups.
In addition, the report said, missing or biased biomedical data used in medical AI systems “can potentially cause discriminatory harm and reproduce or exacerbate the racial disparities that already exist in medical practice.”
The report also cited cases where AI screens for conditions were less accurate than those made by humans and of shortcomings in studies of the accuracy of AI.
While AI-based medical research “is a really important opportunity and could provide even more value,” Koller said, more work and funding are needed to realize that value. Even then, she added, some “overhyped” promises of the value of AI “are never going to come true.”