State of AI Report: Transformers Are Taking the AI World by Storm
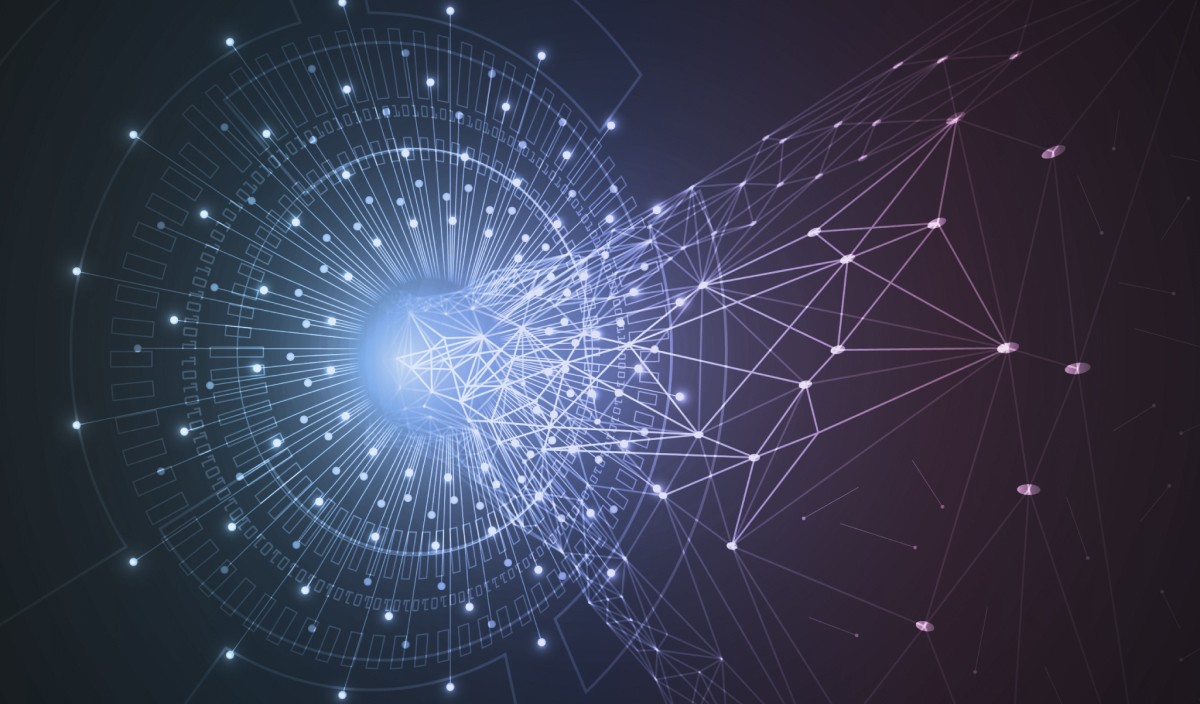
Self-attention technology is spreading beyond NLP to become a hot general-purpose architecture for machine learning.

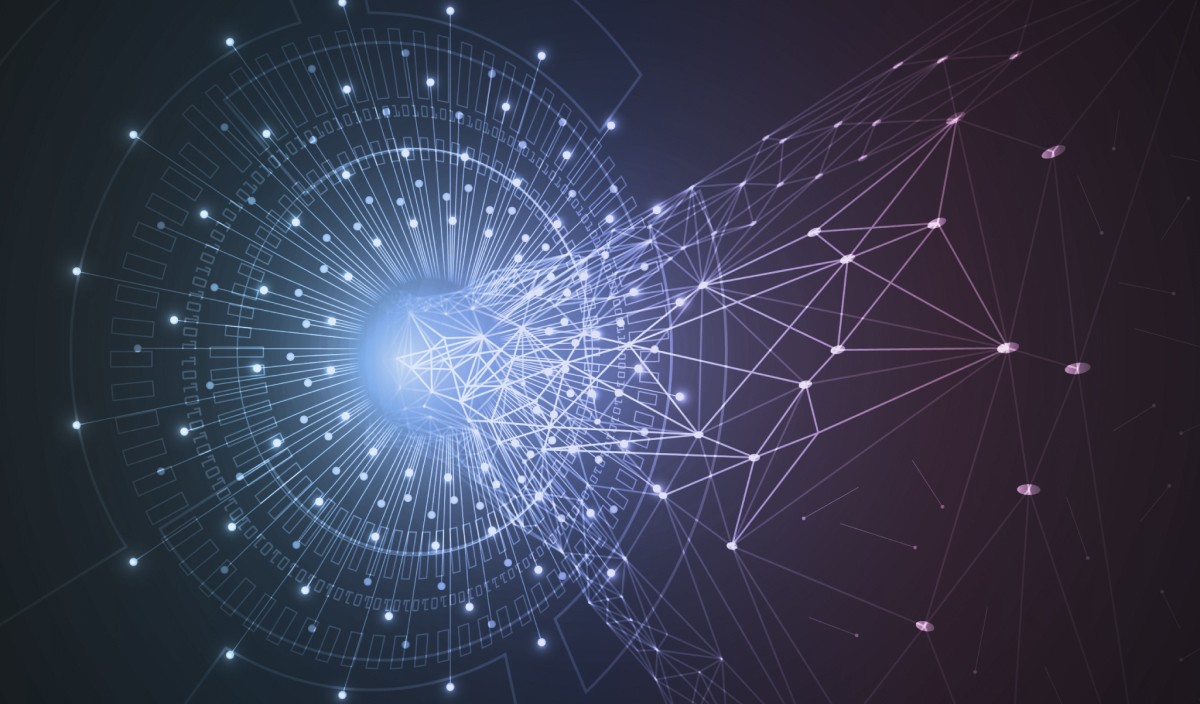
Transformers—not Megatron and Optimus Prime, but a neural network architecture based on a self-attention mechanism pioneered by Google—took the machine learning world by storm in 2021. Originally designed to work with natural language processing models, the technology has blasted out of NLP over the last 12 months to emerge as a general-purpose architecture for ML.
That was just one of the findings in the fourth annual State of AI Report recently released by Nathan Benaich, a general partner at Air Street Capital, a venture capital firm that focuses on AI-first and life science companies, and Ian Hogarth, an angel investor in more than 100 startups.
Benaich and Hogarth had predicted an upward trajectory for transformers in the 2020 edition of their report, but they were caught off guard by how rapidly the ML community embraced the technology. The most surprising outcome from last year was “the widespread expansion of transformers from NLP to almost every other machine learning task,” Benaich said during a recent talk about the 2021 report hosted by Elliot Branson, Director of ML and Engineering at Scale AI.
“We predicted that it would be applied in computer vision, but we didn't think it would expand into chemistry and biology. The transferability of these models to different domains is really remarkable." — Nathan Benaich
The report covers important developments in AI and ML in four key areas: research, AI talent supply and demand, areas of commercial application for AI and its business impact, and politics, including regulation of AI and its economic impact. The report also includes predictions for longer-term AI trends.
Predicting Proteins
The report called out Perceiver, a promising transformer by DeepMind. Its general-purpose architecture doesn’t use domain-specific assumptions and can handle arbitrary input types, including images, videos, and point clouds.
Another development demonstrating the flexibility of transformers was made by researchers at UC Berkeley, Facebook AI, and Google, who showed you don’t have to fine-tune the core parameters of a pre-trained language transformer to obtain very strong performance on a different task.
While transformers have become wildly popular in the ML world, the report noted that two technologies—convolutional neural networks and multi-layered perceptrons—can provide benefits competitive with transformers on several NLP and computer video tasks.
In biology, the report said, AI-first approaches could simulate both proteins and RNA with high fidelity. “The two coolest applications in biology that I found both involved NLP,” Benaich said.
“A group at Salesforce research made use of these models applicable for translating a sentence from one language to another and applied it to a large body of proteins,” he explained. “From that, they figured out the ‘language’ of proteins.
“The coolest bit of that is you can get the model to generate a protein that has never existed in nature” and has new properties, he said, “that are of industrial importance and interest.”
Some have wondered how a research group at Salesforce, which makes enterprise software, could have “a big impact in a field that presumably they have no direct experience in,” Benaich said.
"The fact that that’s possible is a hat tip to the generalizability of these models.”
Forecasting COVID Mutations
Another breakthrough during the year was a discovery by researchers at MIT that NLP models could be used to predict the evolution of the spike protein on the COVID-19 virus. Using the models to collectively learn the “grammar” of the spike protein could open the door to identifying mutations before they occur and give vaccines the ability to counter them when they do appear.
The report also flagged JAX as gaining popularity as an ML framework. While the framework isn't used in production yet, the report predicted that the research-to-production gap would be closed eventually.
In the talent department, the report said that China continues to build its AI capabilities. Chinese universities have gone from publishing no AI research in 1980 to producing the largest volume of quality AI research today, the report said. Meanwhile, it projected that China will have double the number of STEM Ph.D. students of the United States by 2025.
Other nations are also stepping up their AI efforts, the report added. Brazil and India are hiring three times more AI talent today than they were in 2017, matching or surpassing the hiring growth of both Canada and the United States.
The report warned of a growing trend in the AI field of big tech companies collaborating with elite universities at the expense of middle- and lower-tier schools. That results in the “de-democratization” of AI research, with a small set of actors creating the majority of high-impact research.
It added that academic funding and depletion of faculties are ongoing problems. Government funding cuts threaten STEM students, who cost more to educate. That stands in stark contrast to China, where elementary and secondary students have been taking AI courses since 2018.
Trust in High-Risk Scenarios
In the industry realm, the report said, the AI company ecosystem continued to mature during the year. IPOs by three companies alone—UiPath, Snowflake, and Confluent—created $38 billion in public market value in 2021. In addition, startups in the United States, Canada, and Europe raised $375 million in the last 12 months to bring large-language model APIs and vertical software solutions to customers that cannot afford to directly compete with Big Tech.
Two AI-first drug companies also floated IPOs during the year, the report said. One of them, Anagenex, has developed a method using graph neural networks to improve the accuracy of DELs—DNA-encoded chemical libraries—which are used for synthesizing and screening large collections of small-molecule compounds. The other company, LabGenius, significantly improved protein designs used to treat inflammatory bowel diseases, the report said.
AI-first products are also starting to be trusted in more high-risk scenarios, the report explained. It cited computer vision models developed by Intenseye that can detect more than 35 types of employee health and safety situations that humans could not detect in real time. Meanwhile, Connecterra has developed a system for monitoring the health of dairy cows by collecting data from a sensor worn around each animal’s neck. The system can identify health problems days before they would be discovered through human observation.
Greater Focus on Data Issues
Data issues have started to raise greater concern in the ML community, the report said.
Although ML models are growing in power and availability, model improvements have been marginal. That’s awakened the ML community to the importance of needing better data practices and MLOps to build better products.
Greater focus is being placed on data issues, such as bias, drift, labels, and specification. Under-specification, in particular, can be a thorny problem in industrial settings. “Models can perform slightly differently depending on how you initialize them, which is scary,” Benaich said.
The report added that benchmarks for models also need improvement. The rapid besting of benchmarks—typically in a matter of months—has become commonplace, but the benchmarks often don’t reflect how a model will perform in the real world. The report called for more dynamic benchmarking, where datasets are continuously updated by human users, which will make the benchmarks more useful.
The report also touched on the current semiconductor shortage. It noted that interest in homegrown semiconductor production has rapidly accelerated among nations smarting from shortages caused by the pandemic.
That will be a daunting task, Benaich explained. “Despite the U.S. and Europe earmarking $200 billion for onshore semiconductor capabilities, achieving sovereignty over the whole value chain would cost over $1 trillion,” he said. That’s almost six times the combined R&D investment in the capital expenditure of the entire semiconductor value chain in 2019. “It’s an incredibly uphill battle,” he added.
More AI Safety Needed
Awareness is growing for the need for AI safety—making sure that AI isn’t deployed in ways to hurt humanity. Citing a survey of 524 AI researchers conducted by Cornell, Oxford, and the University of Pennsylvania, the report said that 68% of them felt safety should receive greater prioritization. That compares to 49% just five years ago. Nevertheless, the domain remains understaffed, with fewer than 100 full-time researchers working in the field of AI alignment—in other words, how to ensure that AI systems' goals are aligned with humanity.
Meanwhile, some nations have AI in their regulatory crosshairs. The European Union has a proposed law on the table that prohibits AI practices that use “subliminal techniques” to distort a person’s behavior or target vulnerable groups.
Predictions for 2022
Transformers, the report said, will replace recurrent neural networks to learn real-world models. That will allow the creation of reinforced learning agents that can best humans in large and rich game environments.
The report’s authors also see a wave of consolidation in AI semiconductors and predict DeepMind will release a major research breakthrough in the physical sciences.
Science will be one of the main beneficiaries of AI developments in the coming year, Benaich said.
“I look forward to more fundamental science problems being solved with machine learning.”