Panel: The State of AI in eCommerce
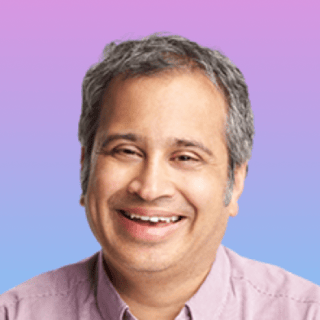
Minesh is Chief Operations Officer at Stitch Fix, where he is responsible for scaling the company's operations while consistently delivering delightful client experiences. His areas of focus include Styling, Client Experience, Warehouse Operations, Logistics and IT. Prior to Stitch Fix, Minesh has spent the majority of his career in high-growth, consumer-driven companies -- focusing on operations, customer experience, omnichannel programs and marketing. Most recently, Minesh led Delivery Operations at Tesla, rapidly scaling post-manufacturing infrastructure while delivering great post-purchase experiences. Prior to Tesla, Minesh was a leader in Global E-commerce at Uniqlo and spent 10 years at Walmart.com, where his experience ranged from being on the founding team to serving as interim COO. Minesh received both a B.S. in Chemical Engineering and MBA from Northwestern University.
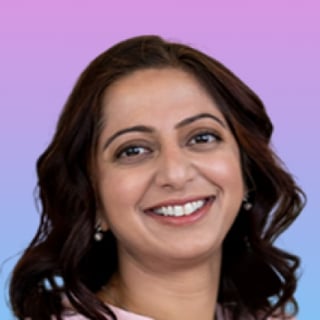
Kady Srinivasan is SVP of Global Marketing at Klaviyo, a leading email and SMS marketing automation platform. Prior to Klaviyo, Kady was Chief Marketing Officer at Owlet Baby Care. Kady has also held senior marketing roles at Dropbox, Electronic Arts, and Betable, and started her career as a software engineer at Intel. Kady, a mentor for Girls Who Code and an advisor to Relay Ventures, earned her bachelor’s degree in computer science and engineering from R.V. College of Engineering in Bangalore and a master’s degree in business administration from the University of North Carolina, Chapel Hill.
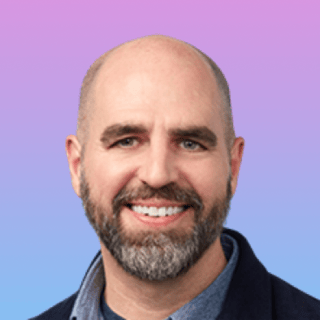
Greg Bowen is senior vice president of Digital Experience in Dell Digital, the IT organization that supports Dell Technologies. He is responsible for driving a single, personalized, consistent digital experience that delights all Dell Technologies customers — from browse to own. A pioneer in eCommerce, Dell revolutionized the configure-to-order experience for millions of PC customers. Thirty years later, Dell.com and Dell’s B2B eCommerce solutions serve 180 countries in 34 languages and are responsible for billions of dollars in revenue for the Fortune 50 organization. In addition to leading the teams responsible for these digital experiences, Greg oversees the strategic direction and tactical operations for Dell Digital’s customer satisfaction, experience design and analytics functions.
We are very familiar with the power of recommendation engines in eCommerce. The most dominant eCommerce companies in the world attribute much of their overall revenue to upselling and cross-selling powered by those recommendations. However, other AI use cases in personalization, logistics, and analytics are also transforming other parts of the eCommerce experience. In this panel, we will discuss the current state of AI in eCommerce. The panel will explore the upcoming use cases primed to disrupt the industry and share their experiences on how they have enabled their organizations to be AI-ready.
Ethan Choi (00:00): Hello. Hi there. My name's Ethan Choi, and I'm going to be your moderator for this panel today. I'm a partner at Accel. We're proud investors in scale of AI that are throwing this conference, but we're also investors in other companies such as Facebook, Atlassian, Slack, UIPath, Klaviyo, and many other great companies. And I'm going to be hosting this panel today that's focused on AI in eCommerce. So we're super excited to have some incredible guests join us today. Let me give a little bit of background on what the panels about, and then I'll have the panelists introduce themselves. And so we are very familiar with eCommerce and the role of AI. Many of us have dealt with that and experienced AI from product recommendations to personalized offerings that come through machine learning and AI.
Ethan Choi (01:26): But what we sometimes don't see is AI weave throughout the backend and the middle office operations of these incredible eCommerce companies that we have today, and many others. AI woven throughout logistics, throughout inventory management, throughout informing product development. So today we're going to dive in and learn more about how AI impacts every single piece of the eCommerce value chain and how it impacts us as consumers. And in this panel, we're going to talk about specifically with the panelists, some of the specific use cases relative to their own companies, and we're excited to have some of the best in the biz here with us today. And some of the most exciting companies that use machine learning AI every single day. We're joined by Minesh Shah, COO of Stitch Fix and Kady SVP of global marketing at Klaviyo and Greg Bowen CTO and SVP of Dell Digital. So I'm going to have each of the panelists quickly introduce themselves in that order, and then we'll dive right in.
Minesh Shah (02:35): Thanks, Ethan. Appreciate it. It's great to be here with everyone. Thanks everyone for joining. My name is Minesh Shah. I'm COO at Stitch Fix and have been more broadly working in eCommerce and in retail for most of the last 20 years of which the last three years have been at Stitch Fix. For those not familiar with Stitch Fix, we're an online apparel retailer that leverages AI immensely in the core business model of Stitch Fix itself, as well as in how we run different functions within the business.
Minesh Shah (03:10): Ethan's point whether that's logistics or inventory management or marketing spend optimization. We leverage AI in just about every facet of our business. At our core in many ways, Stitch Fix is a matchmaker of sorts. We're a matchmaker where on one hand we learn what our client's preferences are, what they're looking for in their apparel size, fit, design, and then the other hand matching it with what's available in the market that will really meet their needs. And in cases where we can't find what will meet their needs. And we try to manufacture the product ourselves. So a very data intensive business. Excited to be here and look forward to the conversation. Thank you.
Ethan Choi (03:53): Awesome. We'll jump to Kady.
Kady Srinivasan (03:54): All right. Hey Ethan, thank you so much for giving me the opportunity to be here. This is super exciting. Before I start Minesh I want to tell you that I was a big time customer of Stitch Fix. I still have so many of those pieces and I was so passionate about Stitch Fix at one point I wanted to go be the person who, I forget what you guys call it, the stylist, I wanted to be the stylist, but I didn't have enough-
Minesh Shah (04:19): The stylist. I well, thank you. We appreciate that.
Kady Srinivasan (04:25): Absolutely. Plus one for Stitch Fix. I run marketing at Klaviyo and for those of you who don't know what Klaviyo is, we call ourselves a marketing automation software company, but for eCommerce in reality, we do much more than that. We do more than email and SMS. We are actually a predictive personalization kind of a platform where we allow eCommerce businesses to be able to see revenue from emails as quickly as 60 minutes. And there's like Ethan mentioned, AI is actually core to almost everything that we do within the company.
Kady Srinivasan (05:03): And it kind of goes towards our founders vision of democratizing access to technology for a lot of our eCommerce businesses who are traditionally not very sophisticated, who want technology to do the things without them having to jump in and do stuff. And so I believe we offer a pretty competitively differentiated product called Benchmarks that actually helps a lot of our eCommerce businesses. Prior to Klaviyo, I've done a lot of other stuff in marketing. I've been at Dropbox, Electronic Arts, a bunch of startups, and then CPG before that, if you can believe it, Johnson and Johnson places like that. So being across the board in terms of all kinds of business models, premium transaction, physical goods is just fun to be in tech.
Ethan Choi (05:58): Awesome. Thanks Kady. Greg.
Greg Bowen (06:00): Well thank you, Ethan. And, and thanks for having me. I look forward to the conversation and feel honored to be on the panel with Minesh and Kady. My name's Greg Bowen, and I am the senior vice president of Dell Digital experience. So I'm really happy that I get to lead a team that runs our entire online experiences. Dell technologies is fortunate in that we are not only a manufacturer of infrastructure that allows our customers to run their AI workloads, but we're also one of the top 10 eCommerce destinations from a B2B and a B2C point of view. So we have to kind of balance those two things, the products that we take to market being AI enabled and also AI enabling our business so that we're more relevant to customers. So I have those online experiences, also our design organization and an analytics and AI team that actually helps build a lot of the AI capabilities that we're putting in front of customers. So look forward to the conversation. Thanks for having me.
Ethan Choi (07:06): Awesome. Well, what incredible backgrounds and excited for this discussion. So why don't we dive right in? So I'm going to start with if you Minesh, if you don't mind putting you on the hotspot. You've seen so much across your time at Walmart, Tesla, and now it's Stitch Fix. Where do you see us in terms of the evolution of AI today? How far along are we, how much more do we have to go to really fully realize AI's potential in eCommerce and your own company?
Minesh Shah (07:39): Yeah. I'll share a couple thoughts top of mind. First is that, to answer your question directly, I think from an AI perspective. I continue to see us all in our infancy, right? As much as we talk about AI, and as much as we talk about the potential and all the progress made to use a baseball analogy, it's still feels like the first inning. And what I mean by that is that, to your point earlier on so much of where AI has been focused from a customer benefit thus far has been the form of product recommendations or customer service, chat bots, great benefits to be very clear, but tend to be pretty transactional, tend to help with the problem that's in the moment.
Minesh Shah (08:30): And what I'm excited about seeing is how can AI be leveraged in the future for more, especially in eCommerce, more sustained richer relationships with between businesses and their customers especially in a world where everyone's one click away from buying something somewhere else. How does it compliment the human touch, not replace, but compliment the human touch in a manner that makes that experience that much richer. At Stitch Fix, that's what we strive to do. We strive to have, like how Kady was saying our stylists compliment what our algorithms generate to look for that combination, but even as much progress as we've made there in the 10 years where we've had that model, we still see a lot more upside in terms of how those two channels interact. So there are a couple thoughts there in terms of where I feel we are in the long journey ahead.
Ethan Choi (09:29): Kady or Greg, I'm curious to get your take on how far along you think we are.
Greg Bowen (09:34): Yeah, I think... Go ahead.
Kady Srinivasan (09:38): Sorry, Greg. I was just going to actually, plus one, what Minesh said about that rich relationships aspect of it, the ability to help businesses develop that one-on-one relationships and then be able to make it complimentary to your human touch. I think that is so important. And I don't think we've cracked that nut. I think we are making some progress towards creating personalized experiences at a certain scale, but that scale is also pretty subscale. We have so much more to go in terms of how we can truly get personalized. And I am excited about what the future can bring as we bring more data together, more technologies talk to each other, and then we start to apply more intelligent algorithms to how we can parse that data to think about putting our customers at the center, not necessarily businesses at the center.
Greg Bowen (10:35): Yeah, that's right. And one of the things that we've been striving to do is really trying to democratize AI within our digital group. Too often it has been siloed from a customer experience perspective. It's a recommender and that recommender gets popped in front of the customer. It sometimes in opportune times. When you get more product teams involved in the creation of AI capabilities, and then you're able to stitch it together across the journey, that's one of the ambitions that we have. So we call it personalization at scale. It's not to simply provide a better recommender, although the machine learning allows us to do that and the feedback loops get better and better, but because we have customers that range from the individual going off to college and buying their first laptop on their own, all the way to CTOs that are trying to figure out am I going to go on-prem for my next infrastructure spend?
Greg Bowen (11:33): We have to be able to really try to put the right experience in front of the right customer for every time. And that's why we're trying to get more teams and more business units involved in the creation of AI capabilities and take it out of the IT department. So I think you'll see more and more of that as AI matures, because as Kady and Minesh said, we're day one as well, we're early on. We have over 2,000 data scientists within the organization. But we haven't just scratched the service on what they can do.
Ethan Choi (12:10): I like what you said, Greg, about getting access to AI for the general population of your employee base. And we all know how important data is in terms of enabling better feedback loops and informing the machine learning. Besides that, what other hurdles stand in the way of us making more progress in AI?
Greg Bowen (12:33): Well, I think it's talent. This is a relatively new field. I mean, AI's been around since the concept from the '50s, but it's still a relatively new field and there are not enough skilled practitioners to meet the demand that we all have a for it. So, talent's number one. Organizational structure, I'd say for us was number two, we realized early on you couldn't centralize this in one function, in one team and hope to meet the needs. And so we had to really change the way we were organized and embed it in the teams as I was talking about out earlier. And then finally we talked about data, but content, that is a data type, but you can have the best algorithm in the world. And if your content isn't tagged correctly, you'll never present the right experience in front of the customer that you're interacting with. So those are three pretty desperate hurdles, but I think key ones that we have to overcome.
Ethan Choi (13:42): Yeah. I like that a lot. Sorry, go ahead Kady.
Kady Srinivasan (13:45): No, I was going to say, I love that part about content. I think it's one of the things that we've been working on for the past one year or so is we call this Benchmarks. Basically, it's we collect data from a lot of different companies and we kind of organize that data by if you are a company that's growing at this size with this size of revenue, here are all the funnel metrics. So here's your average open rate, clickthrough rate, here's average add value that kind of stuff. And what we've seen more frequently is people have that information, the people who can access that information, understand it and absorb it in their organization. They love it. They're big, big fans, but a lot of people actually don't adopt it because they're like, I don't quite know how to use this information.
Kady Srinivasan (14:32): I'm not structurally set up to use this information. So for us, I think we see some barriers towards changing behavior of customers to adopt AI related insights that actually makes their work better. And that goes to similar to the content side is a big one. So once I know I have to do all of this stuff, do I have the bandwidth to create all of this content that I can power like a hundred different customer micro segments at these levels? That ends up becoming a barrier too.
Minesh Shah (15:06): Yeah. I really appreciate what you both are sharing, Ethan with asking the question. I had very similar thoughts go through my head. One thing you bring up about content just as an example in the space that Stitch Fix is in in apparel. It absolutely requires us to understand the measurements of the product from manufacturer so that we can understand can we match up that product with the client and their body type? And that's just one example across all of our industries of the accuracy required in that data. And then the scalability of building that content infrastructure. So it remains accurate because if you don't have the right content and the right data, it's like garbage in, garbage out a little bit.
Minesh Shah (15:57): So I fully agree. And then the other thing that you both mentioned that for what it's worth really resonates with us at Stitch Fix is this notion of... We like a lot of organizations have a big data science team, right? However, if they're organizationally set up to work in a silo and they're only impacting product recommendations or inventory management, the sum of the parts is definitely less than the whole. And so what we've been really try to work on is how do we make it more of an ecosystem that permeates throughout our organization? And to Greg's point, I think where we all sometimes run into a little bit of a speed bump is that change management and that talent of those folks that can understand the data. But in addition to understanding it, be able to determine, well when do I use data and do I use intuition? Because just because you're an AI centric organization, doesn't mean there's not room for judgment, experience and intuition to move fast. And if anything, I think that's one of those Ethan to your question, one of those headwinds that we face at times is how do we find that balance?
Ethan Choi (17:10): Well, I love this. So we're going to come back to getting your guys' advice and thoughts on how to continue to move an organization closer towards being really AI capable and forward leaning. But I'd love to hear actually, just a little more detail within your organizations, maybe some really cutting edge use cases of AI today. For example, Minesh I was looking at algorithms Stitch Fix, and it was getting pretty deep into the machine learning techniques and the different applications through inventory management and product development and consumer behavior and matchmaking. Are there some new techniques or new use cases that have come up for you guys that you're finding you're pushing the envelope?
Minesh Shah (17:59): I can probably share maybe an example of a use case. It's actually a bunch of use cases, but I'll simplify it to one use case in terms of how we think about our data. So at Stitch Fix, we have something that we call style shuffle and it's available within our app. And it simply allows our customers to swipe through different styles and give a thumbs up or thumbs down to is this a style that resonates with them? And of course we can use that data to understand what are the styles and the design and the types of apparel that a given client is looking for. That's the primary reason that we have this feature to allow us to serve our clients better. But what it also allows us to do is serve up images of product that we may not have yet that and not have manufactured yet.
Minesh Shah (18:51): And what's to me at least as an operator and as a logistician need about that application is now we're using AI to go way up the supply chain and go upstream into determining, hey, what do we need to provide to clients that we don't have yet or we can't find from any of our brand partners yet? And we want to build private label, and then it goes upstream into actually impacting our manufacturing strategy, our sourcing strategy. And that's a use case set. I think the more upstream you go, it's almost like the downstream client experience is more set up for success. So that's one I'd share with you. That is something that we're continuing to innovate on.
Ethan Choi (19:35): Yeah. Greg, I'd love to hear from you as you're trying to drive, holistic organizational transformation some new use cases you're finding that's helping Dell become more digital as an organization and how it's impact your end customers as well.
Greg Bowen (19:52): Yeah. So we have the concept of AI in and AI on. So AI in are products take Alien ware laptops, for example. Creating algorithms that are going to optimize frame rates per second, to match the games that are being played on the laptop, or to try to better understand that gamer so that we can optimize the settings in real time to optimize the gameplay. So that's an example of AI in a product. And then AI on.
Greg Bowen (20:27): So for us in digital, when we're trying to speak and engage with customers, real time sentiment analysis, that's not only looking at when customers fill out a survey telling us what their satisfaction is, but how can we scan the immense amount of call logs, chat logs, in order to anticipate situations and problems in the experience before they start to snowball, and then we can fix it. Another example is that for our customers, we analyze, petabytes of data coming into the data center so that we can go out and fix a problem in a storage array before it becomes a major issue for customers. So avoiding an outage, for instance, for them, those are examples of how we're both using AI to make our products better, but also to make our processes better for our customers.
Ethan Choi (21:28): That's fantastic. And Kady would love to hear from you, Klaviyo is near indeed my heart, because with my partner, I helped lead the investment. And we invested because at its core, it's a data machine learning company, more so than a marketing automation company, as you said, Kady and there's so many ways you guys are using your data across the thousands and thousands of merchants you have to benefit the individual merchant at the end of the day, that wants to speak the end customer. So what are some of the new things that Klaviyo is rolling out? You mentioned Benchmarks, but we love some other product offerings and things that you guys are doing.
Kady Srinivasan (22:04): Like Minesh said, I can actually share one use case as well. We have a customer, a merchant who's selling sports, apparel, and today they can actually collect data from Klaviyo and a few other places that basically dump data into Klaviyo, create a profile of their customer that says this gentleman, Joe he bought a pair of shorts back in July from this particular catalog in the store in LA. And based on his history, we expect that his next purchase is going to be two weeks from now. And we believe that he's going to be interested in the same catalog, which we are going to introduce. And he's going to shop in this region. So we are able to get our merchants into this idea of predictive personalization, which is a pretty powerful concept.
Kady Srinivasan (22:59): I run eCommerce for a long time if I had the ability to be able to say, I know when this next set of cohort of users is going to make this purchase, I would've done everything I could to keep the catalog ready, keep the merchandise ready so that they purchased up from it. So a lot of our customers are seeing tremendous value from not only the data which gets aggregated in the Klaviyo platform, but also what we are able to do with that data that provides them insights for they can do with that information. And so that's one way that we are driving AI and Andrew, our founder, he talks about this idea of personalization at scale, but his idea is for most of our eCommerce merchants and they range from a person who starts from the garage all the way to billions of dollars in revenue and majority of our customers, they don't want to mess around with technology.
Kady Srinivasan (23:58): They're not technologists, they're business owners, they're business operators, and their primary thing is they want to run a business. They want to see results, and he wants to make it as easy as possible to use that technology. So we talk about this idea of giving people a path to success. We want our software to guide our technology users to get to a certain point. We don't want them to worry about what they have to do in technology. So we are spending a lot of stuff and investment in that area. We are coming out with some new products around how to make it easier for people to take decisions based on the data that they're seeing. So it becomes more intuitive all that kind of stuff. And then I really liked what Greg said about AI in and AI on. One of the things I'm trying to do within marketing is take some of the concepts of AI and say, how do we within marketing help drive this idea of right message, right channel, right time to the right person, but do it in a more scalable fashion. And so I'm trying to bring that thinking to marketing, which I think Greg would call AI on to my team. It's still construction, work in progress, but a year from now, we should make some progress there.
Ethan Choi (25:12): I love this whole theme of making AI more accessible to everyone. And to even those who are not really engineers by nature. I certainly could do with all the help machines could do in my life. And so hopefully we all execute on that vision and find ways to apply AI in all sorts of different ways. Maybe we'll transition here a little bit to getting some advice from our three panelists on how organizations can become more AI ready and what you guys and your leaders have done to get your organizations set up that way. So maybe some thoughts around what did you have to do, or what did your company do to set up to be an AI forward company from a cultural and structural standpoint? And then we'd love to get into what your teams look like around moving forward those objectives, but let's start with the first question. What did your organizations have to do? Maybe we'll start with you Greg, this time.
Greg Bowen (26:10): Yeah. So for us, the first thing was trying to understand what was actually happening in the organization. We were 140,000 people. So we just started doing a survey to understand how many data scientists were out there, how many data science projects were going on. It sounds trivial, but we uncovered hundreds and hundreds of them and they were happening in silos. So the first thing was just understanding what's going on. Everyone a few years ago, AI was hyped. It was buzzy. And so if you were a business analyst, you wanted to be a data scientist and have that next to your title.
Greg Bowen (26:49): So we had to level set what did it mean to be a data scientist once we understood the scope of the skill that was in the organization, and then we created an AI COE center of excellence, which was a loosely coupled group of people that could share ideas. And there would be a monthly meetup where we would present, a best in class AI example of either an algorithm. How did you do the right data engineering to prep the data, whatever, just to get a community of practice going. And those were instrumental in us to start building a culture and level setting a large organization on what it meant to be thinking AI forward. So those are a couple examples that we used and definitely we can go into team structure later on after others pitch in here.
Ethan Choi (27:46): Minesh, we want to hear from you. If Stitch Fix ever comes up with a way to make me dress better, I'd love to hear about that too. But yeah, as a company, I mean, you guys were born that way, right? The whole premise of the company was around using data to inform, but what did Katrina and the founders have to do with it all?
Minesh Shah (28:06): Yeah. Stitch Fix it's so different than candidly other companies I've worked at, I spent a lot of my career at Walmart and I remember at walmart.com when we hired our first data scientist, that was a big deal, right. And, and then, you know, quite a few years later, I joined Stitch Fix. And to your point, the premise of Stitch Fix has all always been around having, you know we call the, the marriage of art and science or the marriage of retail and technology. And so we haven't gone through some of the change management like Greg mentions. I'd say more of what we've thought about it organizationally, and even in the early days of which I wasn't there, but I'm well familiar with it is how do we start with, what are the most critical problems we're trying to solve?
Minesh Shah (28:56): Before we talked about, do we leverage AI? Do we not? What are the most critical challenges that we had to solve as a company? And then how do we then determine what is the role of AI in solving that challenge? So, obviously at the beginning of Stitch Fix, a key problem to solve the customer promise is taking on one side, a lot of data that customers have given us that they've like very proactively shared with us about themselves. Some of it's structured some of it unstructured and then matching up with what we know of the apparel that we have in supply on the other end. So I'm oversimplifying it, but we focus there because that started to create some organizational momentum for what can AI do. What's the benefit that it can drive? And almost a little bit of an element of how do you get some quick wins so that the organization understands the capabilities and those that are either unfamiliar with AI, or maybe even skeptical of AI are able to see like, ah, now I see what the role of that is in our strategy.
Minesh Shah (30:07): And then that was the first in a way, probably the first several years, maybe called first three to four years of Stitch Fix. And then beyond that, once we have momentum in there, that area, that's when we started to leverage data more as an ecosystem. And we brought in those similar ideas into inventory management, logistics like I was mentioning earlier, marketing spend optimization. But I really feel like, you have to start with one to three, three tops, key problems that you're trying to solve, not force AI to be the solution, but figure out where does it naturally come in with other processes and then use that as evidence to have it propagate throughout the rest of the organization.
Ethan Choi (30:54): I love that it's a little bit of a land and expand motion if you will, with AI. So Kady same thing with Klaviyo, from the get go, it was all about, housing data, using data, leveraging it. But what has it looked like now and how has it evolved in terms of really maintaining what is it a really data first, AI first company?
Kady Srinivasan (31:16): Yeah, that's right. I think that that's the key is it is a data first, AI first company, just because the way that Andrew, our CEO started to think about how to solve problems for eCommerce merchants. He started with the idea of it has to be about data and it has to be about technology that's fast and intuitive and does not require technologists to operate. And so when you have those kind of principles in mind, you end up with a system that's really easy for any size of business to use, and it puts the data at the center of it. And then once you have all of that data, then you start thinking about how do I use that data to make it really easy for our merchants to get the results that they want. And so that is the core DNA of the company.
Kady Srinivasan (32:02): We all operate from that perspective. If you sit in any of our leadership meetings, the conversations all revolve around, how do we make this more scalable? How do we make this more programmatic? And so it just kind of seeps into the character of the company in terms of how do we use data to basically put ourselves out of work at the limit.? And so with the specific focus on data science, we have an amazing data science leader and kind of his mission is to expose the right sets of insights to our customers based on all of the data that we are collecting from across the ecosystem. And so his whole team is focused on how do we bring the right data in, organize it, synthesize it, and make it accessible to our customers in a way that they can use. And so it's a part of the DNA of the company and started kind of become much more prevalent in other areas like CS. I've had conversations with our head of CS of okay, how do we use technology to make things more efficient? And that's sort of the evolving nature of how Klaviyo is building up.
Ethan Choi (33:17): I'm going to open this up to anyone, any misconceptions or boogieman we should shoot down in terms of trying to move forward, your company as an AI company?
Kady Srinivasan (33:33): I'll jump in here. Actually I'm going back to something Greg said about or Minesh, maybe you said this around balancing intuition with AI. I think that's the hardest one to get right. And I think that's where we all have to be a little bit careful because I have seen cases where we get so immersed in the data, there's so much analysis paralysis happening that we don't get to move forward. And frequently, we kind of dismiss the idea of a gut feel about what this needs to be, particularly when you think about what a product vision should be or how we should be thinking about strategy and other things. So that's probably the only thing I'll say as a caveat or a thing to keep in mind as we move forward.
Minesh Shah (34:22): Yeah. I meant mentioned it earlier, so I definitely agree with you there, there are times where in my mind, this is more me where I'm in different discussions. And I almost like the science fiction movie quote, going through my head of, are we letting the machines take over? And that is something that I think is really important to be mindful of. To Kady's point, because sometimes, especially as companies are developing AI capability, it's still the capabilities in development and it often is optimizing for just one metric. And generally when we're all running a business, we're optimizing for a dozen metric. And if you let that machine take over for that one metric, it looks like a virtuous cycle for that one metric and a vicious cycle for the other ones. And that's just something I think, to be careful of. It doesn't diminish the promise of AI to be clear or the need to focus on it. I think it's that element of just, how do you make sure it's thoughtful balance.
Greg Bowen (35:27): And maybe just riffing on that for a little bit. This isn't to replace humans, this is a augment, and this makes every employee better at what they do. AI for the beginning stages can be used to just eliminate repetitive redundant tasks and let your teams focus on the much more critical thinking skills that are necessary to drive business growth and customer engagement. So don't believe that this is going to solve all your problems. You still need really smart people to work with AI and not let it replace everything that you're doing.
Kady Srinivasan (36:11): You probably likely create new problems.
Ethan Choi (36:15): Yeah. It's a great point, Kady. Maybe to the theme of trying to make AI more accessible for the masses and an organization, those who aren't maybe so familiar with data techniques or data itself, or in quantitative roles are there things that your organizations are doing to better educate them or bring them up the curve in terms of how to think about data? What are some that other organizations should be thinking about to continue to make AI more accessible for the rest of the organization?
Greg Bowen (36:48): I can start. And this was when I referenced talking maybe about team structure and organizational structure earlier. Yes, AI should be more accessible. We also think that we should bring data scientists and machine learning engineers to every team. And so we created a product team structure. This isn't novel or new. I was at Amazon for 15, 16 years prior with Dell, and they called it two pizza teams. We call it the Dell Digital way or the product model, but it's a way of bringing design, engineering and product management together. And a data scientist is often part of that team. And that data scientist can help inform the rest of that team on best practices for data management and use, how to write a great machine learning algorithm.
Greg Bowen (37:41): And so we believe that it's bringing the practice of data science, machine learning, AI, engineering into each one of your teams. It might not hit your point about in the business, but we also have a model where the business comes into the product team as well. And so there'll often be a business process or a subject matter expert that sits with that product team and understands how you can use machine learning to solve a problem better than potentially another engineering practice. So it's really how we've tried to infuse it into everything we do, and to make us, AI native in a way going forward into the future.
Minesh Shah (38:27): Yeah. I mean, Greg building off of what you said, I do think the organizational structure is a key piece of that puzzle. If you look at an org structure of Stitch Fix, you see the data science team altogether, but they're really quite decentralized. They're centralized on an org chart, but decentralized in how they work. And a couple of examples very similar to what Greg said in two different directions one of my areas of responsibilities is all of our logistics and warehousing, and sometimes the best progress we make in solving a warehouse issue is when you're literally in a warehouse and you have a team of data scientists with a team of engineers, with a team of folks from warehouse ops. And it happened a lot more pre-COVID.
Minesh Shah (39:16): But it's a beautiful thing because everyone is seeing the problem live in the same way at the same time. And the operator maybe thinks differently than somebody from data science and vice versa. And it's very old school, but it really drives towards getting the entire org educated about what AI can do. And then on the flip side, also to what Greg shared, one of the key focus areas that we have right now at Stitch Fix is how do we take all the data that we get from when our clients contact us? Something has confused them. Something has happened that hasn't made the experience as delightful as we'd like, and then how do we feed it back into the data science team? So we're really trying to figure out how do you have this multi directional flow of information, but not just even the data itself, but the why behind it that educates everyone about how can we use this data.
Kady Srinivasan (40:19): Yeah. I love that. I love those examples. I think for us, what I'm seeing actually is less about educating our internal teams, but more about in educating our customers and partners to some extent about how beneficial AI can be for them. A lot of our customers today they know about the stuff that they have to do to get a business running and go online. But a large part of them are saying, I really don't want to deal with all of that stuff. What is marketing automation? I really don't want to know about that stuff. And so for me, my challenge is I don't want to go to them and say, look at this beautiful piece of AI software I have, because it's just not going to track. And so what we are spending a lot of time doing is thinking about where do our customers stand in their kind of mindset in their learning journey of what they can do with AI, and then helping influence them to just kind of graduate to the next level and to the next level.
Kady Srinivasan (41:24): Similarly with partners too, it's interesting Klaviyo can absorb reviews data and shipping data and all of this, and then put it in one place. But when we go to partners and talk to them about, look at all of the stuff Klaviyo can do, it's like light bulbs go, whoa, I had no idea that you guys can do this stuff. And so the, there's the spectrum of understanding that we are trying to get a push all the time.
Ethan Choi (41:51): Love that. Is there a critical mass of certain number of data scientists or AI machine learning focused experts that you need to have in an organization to have a base level of capabilities? Or how do you guys think about that question in particular?
Greg Bowen (42:14): Well, I maybe would say you just need to get started. Hire the first one or first define a problem. It's just not just hiring a data scientist, but what's a customer issue that you're dealing with and start with that customer problem and work backwards. Do you need AI? Do you need a data scientist? Get started. Scale helps, there's as much intelligence human intelligence in defining AI algorithms as the machine, the algorithm and the model itself. So it definitely helps to have scale but like I said, they can operate independently and decentralized if you have good infrastructure, if you you've got good tooling. And I think that's as important as the number of data scientists. If you put them out there in the wild and they're operating on their own, and they don't have good tooling, it's going to be very difficult to engineer the data correctly to create the right algorithm and then deploy it into production. That can be very difficult without scale. So it just depends on the size of your organization and the customer problems you're trying to solve is my recommendation.
Ethan Choi (43:27): That's great. Maybe I think a few more questions, I think we have some time for. As you think about your organizations and eCommerce in general, what does the future of AI applications internally and externally look like for you? I don't know if your organizations have dreamed up new ways of interacting with consumers using machine learning. I'd be curious to hear that, but if there's any broad statements on, hey for us, we believe machine learning is going to be in every single department. I'm just curious what do you think the future holds in for AI in eCommerce?
Minesh Shah (44:15): I'll share a couple thoughts. We talked a lot today and especially about this idea of matchmaking and we talk about product recommendations. And of course that's going to continue to be a key part of AI is understanding what do customers look for and what should companies deliver to them. And either on their website or through marketing channels or, or what have you, one of the big focus areas I should say that we have is to get to that level of sophistication in all of the other functions where AI can really have a strong benefit in sourcing, in inventory management, logistics, customer service. I think it's a big one. That's very much in its infancy, still. Chatbots are just the start.
Minesh Shah (45:03): So that's Ethan an admittedly general answer to your question, but I share it with you because there's a lot of what's not directly customer facing or backend. That really is at the top, the very top of the first inning when it comes to AI. And that's where I see more and more focus being required. Otherwise you can argue that in at least in the world of eCommerce AI effectiveness and benefit to the customer will start to plateau, right? It'll start to plateau if it just starts and ends with recommendations and chatbots.
Greg Bowen (45:42): Yeah. I agree with you Minesh. At Dell technologies, we are really trying to drive this into every facet of the business from forecasting demand, from a total marketplace to a sorting product for customers, making the right recommendations. But we even look at it as our CTO, John Rose talks about there has historically been human users. They run applications on infrastructure and they need compute and storage. Then there were machines and machines were in the environment. Now AI is a user in and of itself. And AI is going to be one of the biggest users of your infrastructure. And it's going to create immense amounts of data, not only using it, but creating the data. And so when you start to think about how AI permeates every aspect of the business, when AI becomes a user of your infrastructure and your ecosystem, you've kind of gone full circle and it can really be a cutting edge capability for your organization. So it's just something to keep in mind as you start that first step and you don't know where you're actually going to end up at the end.
Kady Srinivasan (47:02): For me, having run an eCommerce business before and being now in deep into the heart of eCommerce, I think the biggest challenge for eCommerce businesses is going to be growth, hands down. There's going to be so many problems with how do I grow when I'm not going to be easily discovered? How do I grow when there are so many people competing for my customers? How do I grow when data privacy issues are happening? That single thing is going to be such a big problem that the way I foresee it is the best way for you to kind of bend that curve a little bit is to focus on your customers and to give them that the best personalized experience possible to hang onto them. I mean, the Stitch Face fixed thing. It's been 10 years since I bought a Stitch Fix thing.
Kady Srinivasan (47:52): I still remember it as the best experience I had. It doesn't get more personalized than that. And so for me this is about how do we ensure that we are using AI to help merchants or eCommerce businesses to move forward on that growth path with that underlying philosophy of it's all about personalized experiences. How do we help AI drive personalized experiences at scale for those people so that you can create those experiences, hang on to those loyal customers, which then hopefully will create a virtuous loop for that eCommerce business? So I think we are maybe 10% of the way there with moving target. So it'll be exciting to see how much more we can push it.
Minesh Shah (48:42): If I can jump in Kady on one thing you said. For me, it's such a critical point. It's this notion of in a world where there's going to be more data and Greg alluded to it too, that AI's going to create even more data. The best data is the data that's given to you by your customer, right? That requires minimal interpretation and machining of, and how to create those cycles and that virtuous cycle with the customer of, hey, look, the more that we learn about you, the more we can improve the quality of experience that you get from us. That's so powerful and being completely above board about it, right.
Minesh Shah (49:20): Being above board transparent, and then letting the customer decide, do I still want to keep opting in and giving you information about myself or do I not? And as we think about the future of AI, that aspect, in my opinion is going to have an out sized role when we think about data privacy and the such, is that how are we really transparent about the collection, the use and the benefit, and then give the customer the choice? Do I want to keep playing this game or do I not?
Kady Srinivasan (49:53): Yeah, that's such a great point. I actually want to put a plug in for something that we are calling customer first data. The customer first data is essentially the combination of zero party data, which is basically what Minesh, like you said, what customers give us and the first party data, which is what you observe when they come to your site. And the plug is as an industry, let's stop calling it ex-party data because it's about customers. I will stop there.
Ethan Choi (50:23): I love that. That's a great framework for us to think about, and we could talk about data privacy for days and how to make sure we do that in the right way and bias and all kinds of things. But this has been wonderful. Maybe the last question we'll end on here is, maybe a recommendation from you, word of advice for a company just starting out in their customer journey. I think we've heard some great ones, which is the theme around just get started with one single problem. But if there's any other great advice you might have for a company just starting out in their AI journey, what would that be? And Greg we'll start with you.
Greg Bowen (51:04): Yeah. So I said it, just get started, but take an iterative approach. If you fail the first time or the second time, don't worry about it. If you're starting small, it allows you to learn. So just be curious and open-minded and learn from each and every implementation that you take on.
Ethan Choi (51:24): Minesh.
Minesh Shah (51:29): I talked a lot about, first identify the problem that you're trying to solve and then figure out the role of AI, but I'll add another one. And that's some of what we covered today, bring the organization along, bring them along, right. As you're just getting started, don't let it be just a project that one team works on because they are the data scientists, but involve the organization in defining what do you want that problem to be? Why, what are people's hypothesis on where we can go. And then as you start to leverage the data, a transparency around what are we learning and what aren't we, which ultimately hopefully gets to that balance of human experience and intervention with the science of the data.
Ethan Choi (52:16): Awesome.
Kady Srinivasan (52:17): Awesome. Yeah. And for me, I think it's hiring, I think in addition to everything that both Greg and Minesh said, I am a strong believer that if you can find the right people and create the right ecosystem, that lot of problems get solved .you start focusing on high quality problems because you have high quality people solving them, and it becomes a virtuous cycle.
Ethan Choi (52:42): That's wonderful. Well, I love our discussion today. We've heard, make AI more accessible, just start somewhere, be thoughtful around the culture and the communication and how you infuse the data scientists throughout your teams. It's been a wonderful learning experience, and I hope those in the audience took some things away and a big thank you to our panelists, who spending the time and shared their wonderful perspectives and their experience with us today. So with that, we'll move on to the next panel, but thank you.
Greg Bowen (53:14): Thank you.
Kady Srinivasan (53:14): Thank you.
Minesh Shah (53:15): Thank you very much.