Insitro Is Using Machine Learning to Make Drug Discovery Faster and Less Costly
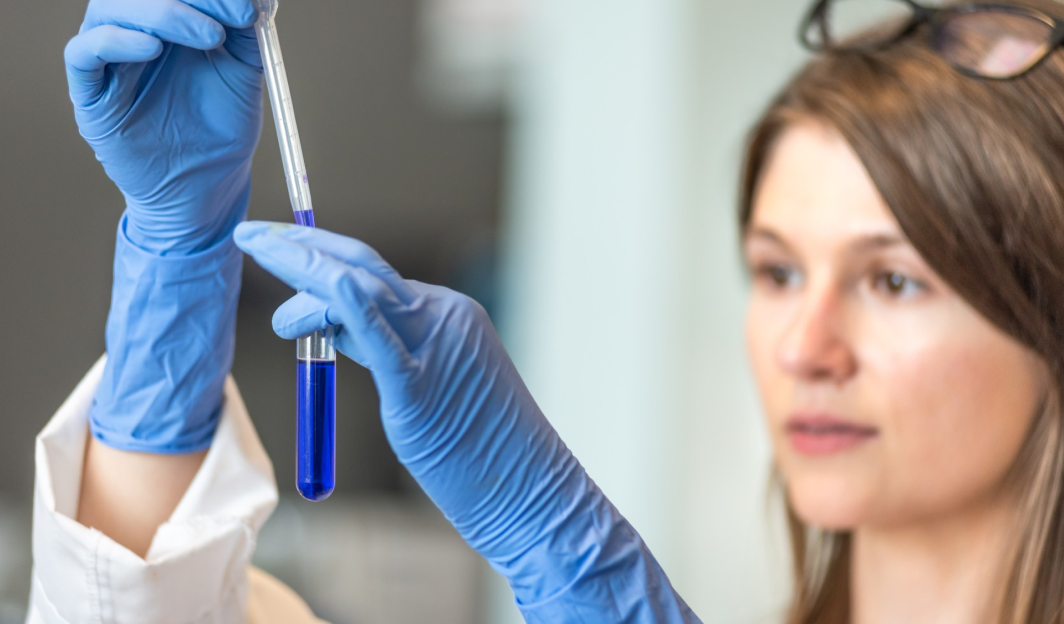
The success rate of drug-related R&D has declined, but machine learning is helping to reverse the trend, says CEO and founder Daphne Koller.

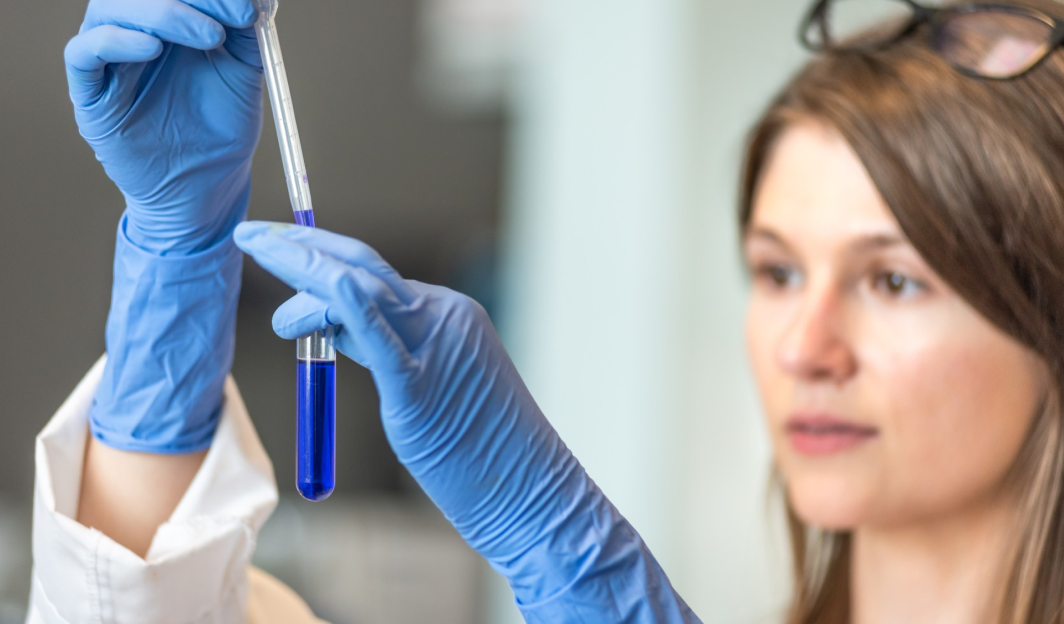
Traditional drug discovery often involves a series of failures before a successful product is created, and each failure can cost hundreds of millions of dollars. It's not unusual for the development of a successful drug to cost $2.5 billion or more, said Daphne Koller, CEO and founder of Insitro, a company focused on drug discovery through ML.
In her session at the recent TransformX conference, Koller discussed the difficulties of developing new drugs using traditional approaches and described how it uses ML to optimize drug discovery, speeding the process while lowering costs. The company uses ML at many decision points to answer the key question: “Which path is most likely to lead to success?” Here are the key takeaways from Koller's presentation.
Insitro Uses ML Throughout the Drug Discovery Process
Over the past 70 years, the success rate of drug R&D has declined significantly. This inverse performance of drug discovery is now established as Eroom’s law.
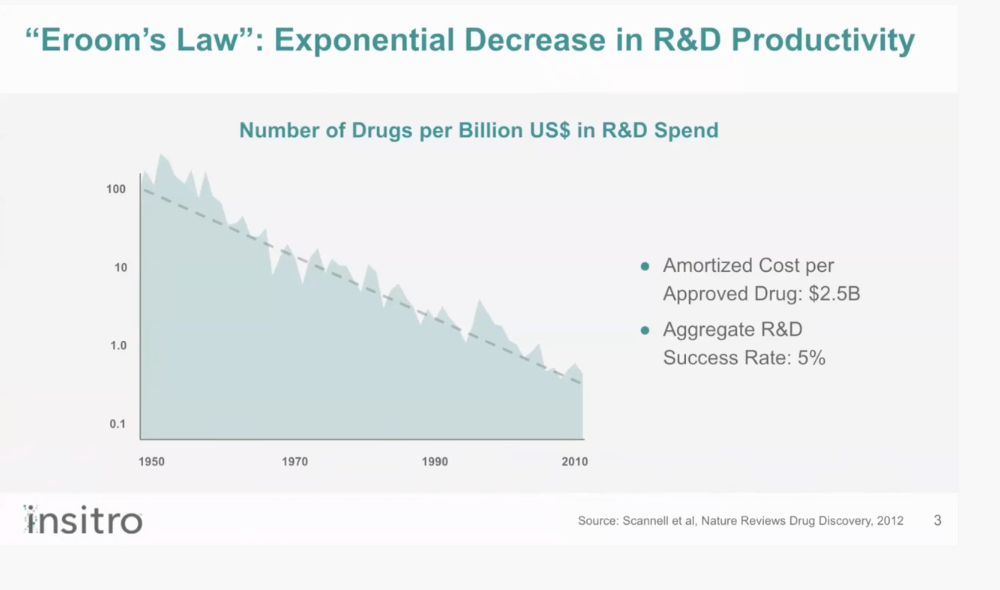
Figure. The "glass half empty" law, known as Eroom's law, is the inverse of Moore's law, which describes the exponential increase of technology. Eroom's law, which describes the exponential decrease in productivity for pharmaceutical R&D over time. Over the last 70 years, the number of drugs approved per billion US dollars has been decreasing exponentially.
To help counter this, Insitro applies ML at multiple steps in the value chain, Koller said, with the final goal being to predict which therapeutic drug will be effective and safe for a specific patient population. Other objectives include reducing costs and time to market.
Before ML is applied, Koller said, Insitro uses statistical and stem-cell models to define a target (a gene or a protein). After that, molecule development, usually a multi-year process, benefits from using ML and active learning.
Rich Data Connects Human Genetics with Biology
Insitro creates data that’s based on patients’ specific biological features and other data sources. By using brain MRIs, ECGs, or histopathology, for example, researchers can create a “dense” representation of humans. This data is combined with cellular samples using microscopy and transcriptomics, Koller explained.
This data helps identify genetic features that could cause disease and generates labels for training a model. These labels can be used to cluster patients and discover which biological properties they share.
Other sources of data include genomes -- an organism’s complete set of genetic instructions -- and phenotypes -- an individual's observable traits, such as height, eye color, and blood type. The number of genomes available has grown at an exponential rate since the start of the Human Genome Project in the early 2000s. Phenotypes are not yet available at this scale, but availability is increasing at a rapid rate, Koller explained.
By combining genotype and phenotype data, researchers can find causal relationships. But there are many different possibilities that can result from this data, and “it’s expensive to explore” each of them, Koller said.
How Useful Is ML in Medicine?
Nonalcoholic steatohepatitis (NASH) is a liver disease prevalent in approximately 25% of the world’s population and is a leading cause of liver transplantation in Western countries. Insitro applied ML to the question of whether there are any genetic drivers to signal whether a given patient’s NASH disease is progressing or regressing.
Insitro sought an answer by using biopsy samples as raw input, creating a convolutional neural network to predict pathology scores, and building an attention mechanism to identify where the disease was progressing, Koller said. The model also learned to predict scores that were more correlated with blood biomarkers than were the pathologists’ scores.
These finer-grained scores of a patient’s disease state also allowed researchers to identify novel, significant genetic drivers of fibrotic progression, the key process in NASH morbidity and mortality.
ML also helped Insitro devise a cellular model of the disease. Researchers recreated a diverse subset of NASH-relevant genetics using cells collected from multiple patients and from artificial samples made with the CRISPR gene splicing tool.
Researchers then measured and analyzed these cells to create high-content profiles. This allows for something that can’t be reproduced within humans: manipulation of cells to revert them back to a disease-free state.
“The NASH disease models are often indistinguishable to the human eye, but not to ML models,” Koller said. “Additionally, Insitro was able to apply interpretable ML techniques to figure out how the ML model made its decision, thus adding revealing insight that might not have been observed otherwise.”
The task for the ML models was to distinguish between cells taken from NASH patients and those taken from matched controls. They were matched for age, gender, body mass index, other diseases, and other factors.
This revelation allowed the company to create a NASH screening platform for therapeutic interventions intended to revert unhealthy phenotypes to ones that are healthier, she added. The goal is to make the "sick" cells look more like "healthy" cells. And these are real cells, not computational models.
“This allows for the identification of potential drug candidate molecules, and their efficacy can be evaluated on the disease model by optimizing on a specific phenotypic output,” Koller said.
Can You Speed Up Molecule Development?
Researchers generate large-scale chemistry data using a technology called DNA-encoded libraries and use this data as input for novel machine learning methods. This, in turn, helps build models that aim to predict what molecules are likely to be good binders to a protein target, Koller said. The goal is to identify plausible compounds and quickly test them, to see which regions of chemical space are worth additional exploration.
This reduces molecule development time because you can purchase these compounds off the shelf, test them quickly, and then iterate to make them better.
“Insitro aims to discover and develop transformative medicines in a way that lowers the risks and accelerates the R&D process by building predictive models based both on cutting-edge ML and on the ability to generate data at an unprecedented scale,” Koller said.
Is Digital Biology the Next Epoch?
Since the rise of the scientific method in the 17th century, various disciplines of science have reigned supreme. In the 1990s, data science and quantitative biology started coming to the fore. These fields developed separately, but now a combined field of digital biology seems to be emerging, Koller said.
Digital biology will allow scientists to measure biology in entirely new ways, to interpret the measurements by using ML and data science, and to turn the resulting insights into interventions that make biology behave in ways it normally would not. Digital biology is the field to watch for the next 30 years, Koller said.
Learn More
For more details about how Insitro uses ML in its work, watch Koller’s talk, “Transforming Drug Discovery Using Machine Learning,” and read the full transcript here.