Transforming Drug Discovery Using Machine Learning
speaker
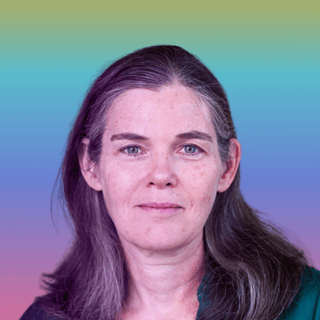
Daphne Koller is CEO and Founder of insitro, a machine learning-driven drug discovery and development company. Daphne is also co-founder of Engageli, was the Rajeev Motwani Professor of Computer Science at Stanford University, where she served on the faculty for 18 years, the co-CEO and President of Coursera, and the Chief Computing Officer of Calico Labs. She is the author of over 300 refereed publications appearing in venues such as Science, Cell, and Nature Genetics. Daphne was recognized as one of TIME Magazine’s 100 most influential people in 2012. She received the MacArthur Foundation Fellowship in 2004, the ACM Prize in Computing in 2008, and the IEEE CS Women of ENIAC Computer Pioneer award in 2022. She was inducted into the National Academy of Engineering in 2011 and elected a fellow of the American Association for Artificial Intelligence in 2004, the American Academy of Arts and Sciences in 2014, and the International Society of Computational Biology in 2017.
SUMMARY
Daphne Koller, Founder, and CEO of insitro, shares how recent advances in machine learning and medicine enables us to 'rethink' drug discovery and reverse a long-standing trend of declining drug research effectiveness. She also shares how AI can make high-quality clinical predictions that are as good as human beings, if not better, leading to better patient outcomes.
TRANSCRIPT
Nika Carlson (00:16): I hope you're enjoying TransformX so far. For our last session of the day, we're honored to welcome Daphne Koller. Daphne Koller is the CEO and founder of Insitro, A machine learning enabled drug discovery company. Daphne is also co-founder of Engageli, was the Rajeev Motwani professor of computer science at Stanford University, the co-CEO and president of Coursera, and the Chief Computing Officer of Calico, an alphabet company in the healthcare space. She is the author of over 200 publications and was recognized as one of Time Magazine's 100 most influential people. She has received the MacArthur Foundation Fellowship and the ACM Prize in Computing. She has been inducted into the National Academy of Engineering and elected a Fellow of the American Association for Artificial Intelligence, the American Academy of Arts and Sciences and the International Society of Computational Biology. Please enjoy this insightful keynote from Daphne Koller.
Daphne Koller (01:31): Hello, everyone. I'm Daphne Koller, the founder and CEO of Insitro, And it's a great pleasure to be here and tell you about our work on transforming drug discovery using machine learning. So drug discovery is a tale of the glass half empty and the glass half full. The glass half full is a series of tremendous advances that have happened over the last 50 years or more where different medicines have transformed the care of different therapeutic indications, training what has been a death sentence or a sentence of lifelong illness to something that is either cure or manageable disease. And there is a lot of examples of that, not least of which is what we've currently seen with the COVID vaccines. But there's many others that have transformed cancer care, the cure of immunological disorders, genetic disorders, such as cystic fibrosis and more. The glass half empty is what come to be known as Eroom's Law.
Daphne Koller (02:25): Eroom being the inverse of more Moore. Moore's Laws, we all know, is the exponential increase in productivity of technology. Eroom's Law is the inverse of that, which is an exponential decrease in productivity of pharmaceutical R&D steadily for the past 70 years. Where if you look at the graph, which is on a logarithmic on the Y axis, you can see that the number of drugs approved per billion US dollars is actually decreasing exponentially year on year to the point that it takes about $2.5 billion today to approve a drug when you amortize in all the cost of the failures. And that's because the aggregate R&D success rate is about five to 10%, depending on how you look. So what is driving this phenomenon? If you think about the journey that the drug makes between the time that you want to work on a project to the time that the drug may be approved at the end administered to humans, there's multiple forks along the road.
Daphne Koller (03:21): And at each one of those forks, we have to pick which way to go. And most of the paths lead to a failure that often takes months or years and millions and millions of dollars before we realize that we've taken the wrong path. So this is what makes the journey long, expensive, and often ultimately unsuccessful. So the question that we asked ourselves is can we use machine learning to make better predictions at each of those forks in the road on which path is somewhat more likely to get us to success? We don't expect perfect predictions. Biology is really hard, but given how unsuccessful these efforts are today, even a small difference can make a very big difference at the end. So why is this the right time to take on this effort? We are at the convergence of two revolutions. One of which has happened in life sciences and the other in data science and machine learning.
Daphne Koller (04:13): On the left hand side is a suite of technologies, each of which is incredibly impressive and transformative in its own right DNA sequencing, the ability to take cells from any one of us and create a neuron with our genetics, but with a right cell lineage, the ability to perturb those cells using technology, such as CRISPR to change the genetic composition of those cells, the ability to measure those cells at unprecedented scale and fidelity, and do all that in a way that allows us to scale with automation microfluidics. You take these and you put them together, it's an incredible opportunity to generate massive amounts of data that can help us elucidate human biology, human disease, and suggest potential therapeutic interventions. The challenge, of course, is that this data is far beyond what a human can encompass. And so on the right hand side, fortunately, we're in the midst of another revolution, which is that of machine learning where if you look back even a decade, there are many tasks that we were able to perform via machine learning at performance that is barely above that of random.
Daphne Koller (05:19): And today we're performing many of those tasks at a level that is at or beyond that of a human being. And what is remarkable is that difference, the delta between what a human can do and what a machine can do exists, even at tasks that people are good at, like recognizing and captioning natural images. In tasks that people are not so good at like, for example, the interpretation of really high content biological data, the gap between the machine and the human can be even larger assuming that we feed the machine with the right data to learn on. So I'm going to start with a very brief introduction to machine learning. Some of you might be familiar, certainly with the panel on the left, but we find that this way of looking at things really helps explain what it is that we're doing in applying these ideas to biology.
Daphne Koller (06:06): So on the left hand side is the paradigm shift that's happened in machine learning over the past decade or so. Traditionally when we had to train a machine learning to tackle a particular problem, then a human being would need to go into the data set and basically define features slowly and manually that would be the input to the machine when looking at those data instances. So, for example, in images a person would have to say, well, we're looking for certain kinds of textures in the image, certain kinds of edges, maybe little circles that will look like noses of a dog, and that was the feature representation of those images. And the machine learning on top of that was actually relatively simplistic. Turns out the people are just not very good at defining predictive salient features. And that's even in problems that they're actually good at performing themselves like labeling natural images.
Daphne Koller (06:54): And so what happened was that the machines actually plateaued at a relatively mediocre level of performance because their input just wasn't very good. Today we don't try and have people do this task. Rather, the machine gets input, gets its input, the raw data, for example, pixels in the context of images, and then the machine creates for itself an increasingly complex hierarchy of features that are built on top of other features that are all trained in order to optimize the abilities of the machine to perform the task. And this hierarchy of features turns out to be much better than what a person could come up with to the point that the machine's able to make really subtle distinctions like between an Arctic Fox and an Eskimo dog, where most people are unable to make this distinction far us to explain how it should be done.
Daphne Koller (07:41): So that's one standard perspective of what's called end to end learning or deep learning. The other way of thinking about this though, which people don't always think about, is that this is also a way of reducing the dimensionality of the problem, creating a new feature representation of the domain. So for example, if you think about images initially as say a thousand by a thousand, they reside in a million dimensional space. That final layer of features just before the prediction is made is usually about a hundred or a couple hundred features. So in some sense, what we've done is we've created a hundred dimensional representation in a million dimensional space, which from a mathematical perspective, illustrated in the right panel is kind of a manifold or a surface of low dimension that resides in a higher dimensional space. And what's important to understand is that the geometry of the surface or manifold is very different from the geometry of the original space in that objects that are far away from each other in the original space are potentially close by in the manifold and vice versa.
Daphne Koller (08:39): So as an illustration, the two trucks that one sees at the left side of that panel are, in pixel space, as far from each other as any two random images. They share no similarity of color, texture, anything else at the pixel level, but at the high level representation that encompasses tires and windshields, they are quite close to each other. Now, in some sense that's not surprising because in order for the machine to label both of them the same way, they have to be close to each other in the manifold, but what's maybe less obvious is that objects in other classes that aren't labeled the same way, like cars and tractors, still share some of the same underlying features and therefore are placed in an adjacent region of this manifold. And because of that, we can effectively infer that those are related classes, despite the fact that that was not part of our input.
Daphne Koller (09:28): And that is going to be a critically important capability when we're doing biology discovery. So what we're doing in our work is we're taking these ideas and we're applying those, as I said, to multiple steps in the value chain with the goal of better predicting what therapeutic will be effective and safe and in which patient population. So we can think of this, by and large, as encompassing three major steps, although there's a lot more granularity that one could come up with. On the left side is the target. What is the gene or protein or metabolite that our drug is going to target? And to tackle that question, we're bringing together two techniques, machine learning enables statistical genetics on human cohorts and what are called IPC, or Induced Pluripotent Stem Cells, which are these cellular disease models that are derived from human patients.
Daphne Koller (10:16): Given a target, we have to turn it into a molecule. That is often a very complex multi-year process. We think that process can be accelerated and de-risked using ideas for machine learning and active learning to create a molecular entity, which can then be administered to a patient. And the last then piece of this is, okay, how do we administer this? To which patients do we administer? And how do we know that our drug is working? And so for that, we're using machine learning enabled segmentation of patients, as well as biomarkers for patient state, to get us better powered and more successful clinical trials. So I'm going to start with our biology discovery engine, which really focuses both on the first and last chevrons in this diagram, and then we'll talk about the middle one. So the goal here is first and foremost to answer what is the clinical impact of an intervention in a human being?
Daphne Koller (11:07): So we don't want to cure mice. We don't want to cure even non-human primates. We want to cure people. So how do you get in data about the intervention in human without actually being able to do interventions in human until you do the clinical trial. So here what we're doing is we're basically taking a high content representation of human beings that gives insight about their underlying biology. So in the case of images, we were representing images via pixels. Here we're taking human beings and we're representing them using high content data from both human cohorts, as well as from cellular models taken from those humans. So for example, you can take a human and measure their biology using histopathology, using brain MRI, using electrocardiograms. It gives you a very dense representation of patient state. We can also take those humans, take cells from those humans, measure those cells, using microscopy and transcriptomics and get insight about what their phenotypic representation of cells from those humans look like.
Daphne Koller (12:13): And so with that we can then take genetics that we know give rise to disease, or we know do not give rise to disease. That gives us labels for our machine learning model, and then allows us to create the manifold representation, which basically shows us which patient cluster together and what biological processes do they share. This is basically a discovery engine for patient segments, a discovery engine for causal drivers of disease that can then be therapeutic intervention targets. So in order to get this kind of data, we rely on, as I said, the revolution in bioengineering and cell biology. One of the most important part of that has been the understanding of the human genome. This is a very compelling graph because it is actually a Moore's Law graph. You can see that Y axis is on a logarithmic scale. It measures number of human genomes that have been sequenced from the very beginning of the Human Genome Project in around 2000.
Daphne Koller (13:10): And you can see that not only is the number of human genomes sequenced growing exponentially, it's actually growing twice as fast as Moore's Law. So we would expect by the end of this decade to have hundreds of millions, if not billions, of genomes sequenced. Genomes on their own are useful in themselves, but they're even more useful when you juxtapose them with phenotypes. Phenotypes are not, there's not quite as many of those to be had as there are genomes, but it is growing rapidly. One of the earliest of those efforts is the UK biobank, which took 500,000 individuals and measured them in many, many, many different ways, including blood biomarkers, urine biomarkers, imaging, predisposing factors, and many others. And has continued to follow them for what is now out over a decade since the data were collected so we can see how phenotype correlates the ultimate clinical outcome.
Daphne Koller (14:02): And so if you take genotypes and phenotypes and put them together, you can now start to basically create associations between them and because the genetics precedes the phenotypes then to a first cut approximation, associations are generally causal, which means that you can now look at the genetics of the genetic variants that are associated with the given disease and understand something about the causal mechanisms that underlie that disease. And that's been done for multiple different phenotypes. Some of them are disease phenotypes Alzheimer's, type one diabetes, Crohn's Disease. Others are more biomarkers like red blood cell traits or bone mineral density.
Daphne Koller (14:39): And some are not even clinical at all, like for example, height or educational attainment. And so you would think that with this understanding of the genetics of human disease, that gives us all the drug targets we can handle. And the truth is the problem is it gives us way more drug targets than what we can handle, because for most of these complex traits, there are many, many variants that, at least at the population level, have relatively small effect sizes and as such, it's really hard to know which of those you want to prosecute as a drug target, which given that one of those efforts cost hundreds of millions of dollars, is a pretty high stakes decision so it's hard to make it in an intelligent way.
Daphne Koller (15:17): So what we're doing is we're actually creating this intermediate layer that allows us to better interpret the effect of genetic variation on biological processes that are much closer to what is going on genetically. And it is by looking at that high content data and associating with those genetics that we can now understand how genetic variation drives change in human clinical outcome. So I'm going to give you a couple of vignettes that show how we've done this in different ways. So the disease that we've spent the most time focusing on, because it was our first project and collaboration with our colleague at Gilead is a disease called NASH, or Nonalcoholic Steatohepatitis. It's a disease of basically liver failure that is driven by what's called non-alcoholic fatty liver disease, whose prevalence unfortunately is about a quarter of the world's population and increasing because of the increase in obesity and metabolic disease.
Daphne Koller (16:10): So NASH is what happens when the liver starts to fail where the hepatocytes, or the liver cells, start to die. It causes the overall liver to inflame. That leads to scarring and ultimately to liver failure and carcinoma. And it is currently one of the most common reasons for liver transplantation in Western countries and will soon and be the leading cause as we start to cure viral hepatitis. So the challenge that we had when we started working on NASH is to try and understand the genetic drivers of NASH and specifically NASH fibrotic progression. Luckily we had at our disposal a really great data set that was taken from a NASH clinical trial done by our colleagues at Gilead where we had biopsy data that was taken at the beginning, and the end of the trial. The pathologist then interpreted in terms of the level of fibrotic progression.
Daphne Koller (17:03): There's also other data from these biopsy samples like RNA sequencing data, which gives us the transcriptional profiles of those samples as well as multiple others. And so we asked, are there any genetic drivers that give rise to someone progressing or regressing in terms of their fibrotic state. And at the pathologist's score level, there was nothing because it's not a very large data set. It's only a few hundred patients and it's only about a year long. So there's not that much time for a patient to progress in terms of the fairly coarse grained scores that the pathologist describes.
Daphne Koller (17:35): And so what we did is we said surely there is more information in these biopsy samples than just the four scores that the pathologist gives. There's 20,000 by 80,000 pixels. Let's really dig into those biopsy samples and understand the patient state. And so we basically devised a machine learning model that even though had labels only at the level of the entire slide, so only four ordinal labels per one of those large images really dug in and understood where it is that the pathologist was seeing fibrosis within the slide. Notably this was done without the pathologist ever telling the machine where they saw fibrosis. The machine figured it out for themselves using clever machine learning.
Daphne Koller (18:24): And so what you see here is that just from these slide level scores, the machine was able to recapitulate on a held out test cohort from a completely different set of clinical sites, a machine learned score that was very highly correlated with the pathologist score as highly correlated as inter pathologist agreement. And the reason it was able to do that was because it figured out exactly what it is that, say fibrosis or [inaudible 00:18:53] or inflammation looks like, again, without being taught. What's more interesting is that it really uncovered notion of score that was much better correlated with the underlying biology. So you can see here, the correlation with both blood biomarkers and transcriptional profiles from those patients, none of that were ever given to the machine learning, even for training. And you can see that the machine learned scores are better correlated with those biological processes than the pathologist score. But maybe the most important aspect of this is really coming back to the original question of what are genetic drivers of fibrotic progression?
Daphne Koller (19:30): And what you can see here is again, because the data set is so coarse grained, over 54 weeks there's not a lot of events in which the fibrosis score changes from F3 to F4 or vice versa. Whereas with our much better powered and precise machine learned scores, there is way more events in which we can see a change in for fibrotic progression. And that allowed us to basically uncover two novel genome-wide variants of fibrotic progression in NASH that have very compelling biology and that we're now prosecuting in collaboration with our colleagues at Gilead in terms of potential novel NASH targets. So that's one of the aspects of what we do. And if you remember at the beginning of the talk, I mentioned that a big component of what we do is also devise cell-based models of disease. And you can think of those as beginning where the statistical genetics leaves off.
Daphne Koller (20:22): So given a genetic architecture of disease, the first thing that we do is we recreate that genetic architecture in an in-vitro population of cells. We can do that by collecting cells from multiple patients with different genetics of the disease. And we can also create artificial patients by introducing variants that we know to be disease causing using technology such as CRISPR to create basically a fairly broad landscape of different genetic patients with different levels of disease burden that we can then differentiate into the appropriate cellular lineage, so say hepatocytes, if we're doing NASH or neurons, if we're doing CNS disease. And then we can measure those cells in all sorts of different high content readouts, like imaging with microscopy or transcriptional profiles to get a sense of how disease appears at the phenotypic level in those cells.
Daphne Koller (21:16): And based on that, we get novel insights on the disease processes. We get the ability to understand what genetics drives those disease. And we also get what we do not have in a human, the ability to take those cells, to perturb them, to basically screen for interventions that revert the disease state into more of a healthy state. So this is, again, work that we've done on NASH. We've also done a lot of work on neuroscience that I don't have time to show you here. But this is just to show you that the machine is able to also identify novel phenotypes at the cellular level. So this is basically nine patients and nine matched controls. Human biologists cannot distinguish NASH from control by eye, but a machine learning model was able to very nicely separate them on the training set, as well as on a separate validation set of three new patients and three new matched controls.
Daphne Koller (22:07): And we can use techniques from the interpretability of machine learning models in order to basically try and understand what it is that the machine was looking at in distinguishing between the different levels of disease burden. And what turns out to be the case is that if you look at these images, and sorry I forgot say, the pink is nucleus, the blue is cell membrane, and the green are lipid droplets, which are characteristic of fatty liver disease. It turns out that the really big difference is not the total amount of lipid in the hepatocytes. It's not the number of droplets. It's having large lipid droplets at the nuclear membrane. And that actually turns out to align very well with the biology that came out of the genetics. And so now we're using this as a screening platform for therapeutic interventions that basically try and revert that unhealthy phenotype to one that is healthier.
Daphne Koller (22:58): And so, as I mentioned, this really gives you a screening platform that allows you to basically look for genetic interventions via CRISPR screening that reverts from an unhealthy to a healthy state, allows you to also take molecules that are potential drug candidates, and evaluate their efficacy in this disease model. And really once you can evaluate efficacy, you can also, in fact, do a closed loop optimization of a molecule towards this phenotypic output so that you can do what we call phenotypic SAR or active learning in order to optimize molecules towards a reversion of a phenotype in a human cell. So the last piece of what I'm going to talk about is the middle piece, which is it's great to have targets. It's great to have patient populations. What we are also looking to do is to devise molecules. And the question is how do you generate better drugs faster?
Daphne Koller (23:54): So here in the same spirit that we had with all of the rest of our work at Insitro, which is machine learning, is at least as much about the data as it is about the machine learning model. The question was, how do we get chemistry data at high fidelity in high scale? And so what we ended up doing is adopting a technology called DNA encoded libraries, that I'll talk about a little bit on the next slide. It creates a massive data set of binding of small molecules to a protein target. And that, in turn, is used as the input to a machine learning model that basically predicts what molecules are likely to be good binders to that target. With that predictive machine learning model, along with other predictive machine learning models, for example, on properties, such as cell permeability, one can take the vastness of chemical space, which is about 10th of the 80 and quickly narrow down on regions that are likely to be good binders.
Daphne Koller (24:49): And so that you can basically purchase those off the shelf, test them quickly, and then iterate around this loop in a way that doesn't require years and years of searching through the iteratively and slowly through the vastness of chemical space. So DNA encoded libraries, I'm not going to go through the specifics of the technology, but basically you can think of it as a very clever way of doing combinatorial chemistry, where different building blocks are put together to create larger molecules in a way that also keeps track of which building blocks were put together so that we have at the end of the day in a single test tube a hundred million chemical moieties, each with a distinct DNA barcode that keeps track of what that compound is. You can then use that pooled collection of compounds with a given protein target to basically incubate the target with those compounds, pull it out, and use DNA sequencing to figure out what compounds actually bound to the target and which ones did not.
Daphne Koller (25:47): So you get basically a hundred million binding experiments in one test tube, which is an amazing amount of data. So we experimented, inspired by work that came out of McCloskey et all, but also in our own work, with different types of machine learning models on this type of chemical data. We experimented with both classification models using traditional random forests, as well as more sophisticated graph neural networks. And then we really came up with a new model that uses graph neural network regression on the actual count data that came out of this experiment rather than just dichotomizing the compounds into the binders and non binders. And that turned out, as we'll see on the next slide, to give much better results. So in fact, and I will only show you one of those results, this is for a protein target, a very challenging one as it happens, where there was fortunately an experimentally derived estimate of this coefficient of binding called PKD.
Daphne Koller (26:50): And so this experimentally derived estimate was what we used as our validation of the performance of these models. And we were able to show that the graph neural network regression has a much, much higher correlation with this binding affinity as you can see in the graph, both on the left and in the middle, than any of the other models. And when you looked at it from the perspective of retrieving high affinity compounds from the collection, and again this is on a completely held out test set, you can see that the regression model retrieved a lot more of the compounds that had binding the affinity than the more traditional classification models. So in order to further drive our work in this area, we actually acquired a company that has a technology called Indexer that gives not just this relatively simple readout of binding affinity with something that is much, much more quantitative.
Daphne Koller (27:45): I'm not going to go into the technology, but it is really an incredible synergy with our more quantitative models in that it allows you to make predictions using much more quantitative data. So putting this all together, our aspiration at Insitro really is threefold. First of all, it's to discover and develop transformative medicines. All of biopharma really wants to do that. But we want to do it in a way that de-risks and accelerates this R&D process by building these predictive models that are based both on cutting edge machine learning and on the ability to generate data at unprecedented scale. And then finally, because machine learning does that, it allows us to build a flywheel that just keeps getting better and better as you give it more data, therefore providing us with a durable advantage in how we discover and develop medicines. I'd like to end this talk with a very philosophical note that I think is just interesting to think about from the perspective of why I'm doing this, of all the many things that I could be doing.
Daphne Koller (28:45): If you think about the history of science, there is you can think of science as progressing at a set of epochs where in different times there has been a given field that has evolved really quickly because of a new insight or a new way of measuring things. In the late 1800s, that was chemistry because of the realization regarding the periodic table. And we are no longer trying to turn lead into gold. Early 1900s, that was physics with the connection between matter and energy and space and time. 1950s computing, where an artificial entity was suddenly able to perform computational tasks up until that point only a person, if and maybe not even that, was able to do. Then in the 1990s, there was an interesting bifurcation. Two fields started to move forward really quickly. One of those is the field of data, which is related to computing, but isn't quite. It also relies on elements from statistics, from optimization, and from neuroscience.
Daphne Koller (29:46): That's the field that gave rise to machine learning. On the other side is what I would call quantitative biology, which are all of these tools to measure biology at unprecedented fidelity and scale that have given us so many insights about human biology, human disease and even some therapeutic interventions. But by and large, these two fields evolved in parallel with relatively little interaction between them. The field that I think is emerging next is the field of digital biology, which is really the synthesis of those two disciplines in a way that enables us to measure biology in entirely new ways and new scales, interpret what we measure using machine learning and data science, and then take that insight and turn it into interventions that make biology do something wouldn't normally otherwise do. That field has repercussions in human health, as I showed you today, but also repercussions in agriculture and in biomaterials and in the energy and the environment. And I think it's an incredible field to be in because I think it will be the field that shapes the next 30 years of science. Thank you very much.