The Implications of AI Bias & Approaches to Operationalize Fairness
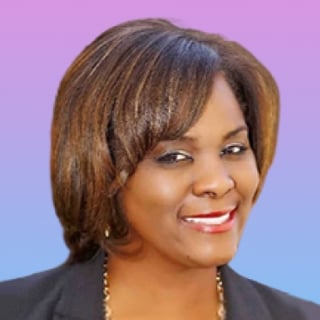
Dr. Nicol Turner Lee is a senior fellow in Governance Studies, the director of the Center for Technology Innovation, and serves as Co-Editor-In-Chief of TechTank. Dr. Turner Lee researches public policy designed to enable equitable access to technology across the U.S. and to harness its power to create change in communities across the world. Her work also explores global and domestic broadband deployment and internet governance issues. She is an expert on the intersection of race, wealth, and technology within the context of civic engagement, criminal justice, and economic development. Her current research portfolio also includes artificial intelligence (AI), particularly machine learning algorithms and their unintended consequences on marginalized communities. Her recent co-authored paper on the subject has made her a sought out speaker in the U.S. and around the world on the topics of digital futures, AI and ethics, algorithmic bias, and the intersection between technology and civil/human rights. She is also an expert on topics that include online privacy, 5G networks and the digital divide. Dr. Turner Lee has a forthcoming book on the U.S. digital divide titled Digitally Invisible: How the Internet is Creating the New Underclass (forthcoming 2021, Brookings Press). She sits on various U.S. federal agency and civil society boards. Dr. Turner Lee has a Ph.D. and M.A. from Northwestern University and graduated from Colgate University.
Join Nicol Turner-Lee, Senior Fellow at The Brookings Institution, as she explores the societal need for fair AI that is equitable to all. She describes the individual and enterprise consequences of bias in AI and the need for multi-disciplinary and multi-methodological approaches to preventing it. How does unintentional bias in AI occur? How do you self-regulate your AI algorithms, so they benefit everyone in the demographics you are trying to serve? How can the fields of data science, law, and sociology join forces to ensure that AI is unbiased? Join this session to hear a thoughtful and research-driven exploration about the risks, impacts, and approaches to mitigating unintended bias in AI.
Nika Carlson (00:15): Next up, we're excited to welcome Nicole Turner-Lee. Dr. Nicole Turner-Lee is a senior fellow in governance studies, the director of the Center for Technology Innovation and serves as co-editor in chief of Tech Tank. Dr. Turner-Lee researches public policy to enable equitable access to technology across the US and create change in communities across the world. She is an expert and well known speaker on the intersection of race, wealth and technology within the areas of civic engagement, criminal justice and economic development. Dr. Turner-Lee has a PhD and MA from Northwestern University and graduated from Colgate University, Nicole, over to you.
Dr. Nicole Turner-Lee (01:04): Hello, TransformX conference. I'm Dr. Nicole Turner-Lee. I am a senior fellow in governance studies and the director of the Center for Technology Innovation at the Brookings Institution and we're headquarter here in Washington, DC. If you haven't checked this out I'd encourage you to look at all the research that we generate both at the institution in general and at the center that I run. I'm excited to be here because my portfolio at Brookings is quite varied. I work on issues related to regulatory and legislative compliance, in addition to the digital divide, I've got a book coming out this year on the US digital divide that took me around the country before and after the pandemic to really understand people's digital access. And the third thing that I work on is artificial intelligence. Particularly I manage at Brookings our algorithmic bias portfolio. And what's so interesting about that is that I'm not a technologist, I'm actually a sociologist.
Dr. Nicole Turner-Lee (01:54): So when I think about machine learning algorithms, I really think about the sociological implications, the societal implications on how we actually create better AI that is not only more responsible as many of us talk about, but it's also lawful and it's equal. And it's also one of those things that's fair and that's something that I hope to talk about in my talk today. I want to use this time to really delve into why I, as a sociologist began to explore this area of algorithmic bias. It's no secret that all of us come to whatever situation we're in and with our values, our norms and assumptions about the world. We have certain upbringing, certain places that we grew up, certain norms that we have and habits and traditions that in many ways really influence how we design and develop things. I mean, I'm no computer scientists, but I can tell you this, that everything that I write about at Brookings comes from the angle of the disadvantaged or people who are more vulnerable in the digital ecology.
Dr. Nicole Turner-Lee (02:49): My book is about that, it's about the people who are often digitally invisible. In fact, the name of the book, if you were really interested is digitally invisible, how the internet is creating the new underclass. And what I hope to do today in my few minutes remaining is really speak about why it's important that we look at algorithmic bias from a very multidisciplinary as well as multi methodological approach when it comes to solving it. A couple years ago our colleagues and I wrote a paper on identifying unmitigated bias within algorithms. And that paper was signature for us because it was done by three different types of people, a sociologist like myself, a lawyer, as well as an engineer. Now I'm not going to lie to you and say that it was the easiest paper to write because we all had different approaches, academic expertise that we brought to the table, but it was perhaps the best paper that I wrote because it allowed me to see what engineers actually think about the problem of algorithms, what lawyers think about when they philosophically frame the question of whether or not algorithms are fair.
Dr. Nicole Turner-Lee (03:52): And in my view, it's just so important that we have as many seats at the table if we're going to develop equitable, deployed, and inclusively designed models, particularly models that now make a difference in our everyday lives. It's no secret that in the last few months that our economy has been heavily reliant upon technology, from how we work and how we learn and how we live and how we earn, having access to tech is mattered. It's even mattered in how we've talked to our loved ones, people who have talked to their elderly family members through the windows of FaceTime or other tools that are available on our phones. These tools actually helped save our lives as we went through months of social isolation and despair. And it's not to say that we're out of it and it's not to say that the technology doesn't have a parallel side, but at least it was there to actually serve the purpose of keeping our humanity together.
Dr. Nicole Turner-Lee (04:52): And what I'd like to suggest in the research that I do that in the wake of this digitization, there are unattended consequences. And the consequences maybe ones that we see and they may be ones that are unforeseen when it comes to the subjects that are affected by them. In my definition of bias and particularly algorithmic bias as we outlined in our paper, it's when similarly situated people, places and things receive differential treatment. And for many of us who work in this space that differential treatment is not always a bad thing. I'm wearing a red dress right now and I do love it when the internet identifies the fact that I love to buy things that have the color red in it. But the challenges come based on the swarm of variables that the technology knows about me is that not only does the technology know that I actually like red dresses, but they can couple that with the fact that I also buy Barbie dolls who may be darker skin complexion, therefore suggesting that I may be a mother who has a child that is black, that I also may be black.
Dr. Nicole Turner-Lee (05:52): And then over time, what we know in the research circles is that that type of information lends itself to the inferential economy. That what started out as something that was an interest, a like, affinity group turns into something that has a potential to be discriminatory. Latanya Sweeney at Harvard University argues that when black or latina sounding names enter the financial services market place, that they tend to receive back predatory or higher interest credit card offerings. In fact, one of the ways that I got very interested in this space when she was the chief engineer at the Federal Trade Commission she put up a website that was promotion for a black fraternity. And she asked us for 30 seconds just to look at the screen. And in looking at that screen, we found that for this black male fraternity, that the higher interest loans were actually being marketed or getting your arrest record for free were some of the ads that actually popped up.
Dr. Nicole Turner-Lee (06:51): That was five years ago and we have got a lot more information about the implications of online behavioral advertising and the discrimination that it may admit. But my friends, it is so important for us to not only acknowledge that algorithmic bias can be a reality in this new digital ecosystem, but it has consequences and those consequences and I like to talk a little bit about them in my talk. They can foreclose on opportunities of populations that do not necessarily look like the people who develop them or people whose lived experiences are not reflected in the model design, where they are actually either invisible in this economy or they do not receive the same types of offerings that others do. And unfortunately in the opacity of the internet where we don't necessarily know if we're seeing the same thing, that can be problematic for groups of populations that have fought very hard for civil and human rights.
Dr. Nicole Turner-Lee (07:43): And so I like to think of it this way. We have come to acknowledgement over the course of time in these last five years, at least that I've been in this, that algorithmic bias is a reality. It's now up to us as scholars and engineers and philosophers and industry leaders to do something about it. And so I'd like to offer you something that I'm working on that might be of interest as we begin to think about how to identify and mitigate these problems. First and foremost, the work that I've been doing is really around what I call an energy star rating. I'll soon have a publication out just on this. I've been talking about it for years after interviewing several companies across the United States, really understanding what is their hygiene or their practices when it comes to developing machine learning algorithms, what are their tests when it comes to distinguishing between differential treatment?
Dr. Nicole Turner-Lee (08:33): Meaning I like a red dress and that's something that I don't mind you micro surveilling my data around to disparate impact. Where that red dress and the compilation of other variables seems to point out that I actually am more vulnerable to be exploited. And what I've tried to come up with in this energy star rating, really rest upon the laurels of the simple thing that many of us who are watching me today have experienced. You ever have an appliance go out? My dish washer went out a couple years ago and I found myself in a store trying to figure out which appliance to purchase. And I landed my eyeballs on this big yellow sticker called the energy star rating for appliances that essentially told me how much water the dishwasher was going to consume, how much energy it was going to take, the durability of the plastic, et cetera.
Dr. Nicole Turner-Lee (09:22): And it dawned on me as I've been doing this research that we need a better housekeeping seal or some type of assessment that allows us to give the confidence back to the consumer, that this algorithm is actually not going to necessarily embed the type of biases that we have seen in the marketplace. It's also helpful my friends, just for those of you who are watching from industry, is that it allows us to keep you off the front page of the newspaper because a reputational risk of having an algorithm that goes bad, like facial recognition technology that may not recognize me because of my darker skin complexion or changing of my hair, that it may not pick up based on the lighting that I use. Those types of reputational risk or blind spots have implications in the long run.
Dr. Nicole Turner-Lee (10:09): So my energy star rating has three components and I'll just short of share that with you and hope that it will elicit a response as you're watching me. And if you want to follow up the conversation, I am easily accessible on all social media platforms. But it starts out with this one pillar, which is what is the self-regulatory action to optimize the interaction of the algorithm with the populations or context in which is being deployed. What does that mean? In simple language it takes it out of the laboratory and into a context where we begin to think about it socio technically, which is what is it that we're trying to solve and do we have the right people at the table in designing and executing the right questions around the model and the model itself? Oftentimes when we are testing things in the algorithmic economy, we don't have the right people at the table nor do we have a seat pushed up to the table to include them.
Dr. Nicole Turner-Lee (11:03): Where I work in this space, like I said, I'm a sociologist, I always tell my engineer friends that they are lucky to have a sociologist as a friend and vice versa. But even for people of color who are often misrepresented or lack representation in the tech sector, thinking about the consequences of an algorithm that goes bad when it comes to financial eligibility, healthcare education, and hiring, again lands you on the front page of the newspaper, but it also suggests that you may not have all the people at the table to better understand how proxies actually show up when you actually deploy the algorithm from the lab into the world. And so my energy star rating assumes that we're going to put some technical cadence to these bottles. When I think about the training data that we use, we're going to ask questions on whether or not the training data is inclusive and representative, whether or not the trading data itself will actually generate a disparate impact in terms of the collective discrimination versus something that's more differential treatment.
Dr. Nicole Turner-Lee (12:02): We're going to use that data and we're going to use those people and resources around the table to test this algorithm in secondary markets or third tertiary markets. We may even find ways to bring more people in to help us think about what could potentially go wrong. In my view, this self-regulatory model is the same thing as a person going into a bank and giving their money to the teller, they're expecting that the money will go into the vault. And oftentimes a lot of the confusion with algorithms that go wrong is that it's not so much that the developer is creating malfeasance, it's the fact that the developer in and of itself brings their own values, norms, and assumptions to the table and they forget about what potentially is around them and the ecology of which is being deployed. Give you a great example of that.
Dr. Nicole Turner-Lee (12:48): There was a company, Amazon is public that wanted to hire more engineer, more women engineers at that. And they trained the data for this new hiring algorithm off of the people who were already employed. Well, 10 years of hiring data were men which meant that every woman that applied or every woman who listed a woman's college or an extracurricular activity that had the word woman in it was kicked out of the system. I'm glad they recognized it before versus later when it became a front page news story that Amazon wasn't hiring women. The second part of my model which I think is particularly important and interesting, it goes to the public policy part of it. I work in public policy, so I always find this part interesting. And here my energy star rating says, okay, we've got the self-regulatory model terms of what are we doing to ensure the best technical cadence, the best monitoring, the best input, the best inclusiveness of voices at the table, the public policy side says, what are we doing to ensure that the outcome of the algorithm is fair, ethical and lawful?
Dr. Nicole Turner-Lee (13:50): And what does that mean? I've been thinking that many of the algorithm that go wrong, particularly that generate bias, where similarly situated people, places and things get different treatment and potentially disparate impact is largely because we have not applied the existing civil right's framework to these models. So we don't really understand that creating models that allow advertisers to check people off, actually violate the Fair Housing Act or other models that allow us to exclude people, though may be efficient in design they actually land up having unforeseen and unintended consequences. And in the end being unlawful because that's not how our civil rights frameworks were negotiated. We're working on a paper right now, Brookings around algorithm bias and whether or not the existing civil right's framework of fair housing and credit and access to financial services are actually the appropriate gap stops to understand civil rights.
Dr. Nicole Turner-Lee (14:46): But my friends in my research what I found is that highly regulated industries like banking or housing who develop and deploy algorithms, keep that in mind when they're actually designing their products. I would like to ask all of you who are developers when you are putting together that next best hiring algorithm, have you looked at the Equal Employment Opportunity Act? When you are actually thinking about ways to harness the data that comes with healthcare information, have you thought clearly about what are some of the sectoral guidance when it comes to privacy and health data? We could get beyond some of these challenges if we came up with some foundational and fundamental principles for what we know we don't want algorithms to do. And that is to strip people of their ability to participate fully in this digital economy. And I think policy makers could also do something that they do best, which is to help industry in the funding of inclusive and more representative data sets.
Dr. Nicole Turner-Lee (15:47): We know that most of these data sets are not necessarily generated with a pony in this sky, but they're based on the human behavior, what we actually do as individuals who are online. I always tell my friends, you can't be mad that you were just marketed that red dress because that's all you buy. In the end of the day it's important for us to realize that we may need help from Congress to be able to get money, to create more over represented or data sets through the National Science Foundation or other partnerships where universities can help us to really over sample some of those populations that we care about. And I think public policy makers was part of my under star rating, could also set up what they did with FinTech, which are regulatory safe harbors and other sand boxes so we can ensure in the absence of collecting specific demographic data, that we're not harming people. Maybe we need to over represent people like me who change their hair for facial recognition technology.
Dr. Nicole Turner-Lee (16:44): Maybe we need to disclose that sister your phone is not going to open when you're in bad lighting, but the bottom line is that public policy makers need to work in tandem with industry and developers to make sure we create a more inclusive and fair, responsible ecology. And I would say the last thing of my energy star rating which I think is so interesting and I'm still working through it for my article is where do consumers fit? When we think about algorithm bias and we think about the algorithmic economy, there's really not a place for us as consumers to wage what our perception is, how the computer is actually seeing me. I think about all of the other tools that I use and of course this is problematic for somewhere I constantly rely upon a consumer's evaluation of a product, a hotel or another service to make the judgment as to whether or not I should actually do business there. In my model of an energy star rating which is why I don't call it a new regulatory gap stop or something that the government has to control.
Dr. Nicole Turner-Lee (17:47): It's almost like that better housekeeping seal, it reminds me of many respects when we move from HTTP to HTTPS, where we knew that when we engaged in secure transactions and in some way that data was encrypted. Friends, we as developers, we as social scientists, we as industry leaders, we as government, we need to allow consumers, the users of our products to give us feedback and input. And I would share with any company that has not opened up a feedback trail, not just an audit, not just something that is post after the fact, but really engages the audience, your consumers in ways that help you build better products with less reputational risk, we're missing out on something. Now you may have heard this and said, okay, Dr. Lee, you've given us something that is a pragmatic way to look at this and I'm going to tell you, I'm going to write about it, you should read it, I think it's interesting and I think that there's been models that are sort of being talked about, which are like labels or nutrition labels, like certifications of algorithms.
Dr. Nicole Turner-Lee (18:50): My challenge is I'm a sociologist, I'm not a technical person and so even if you made the open code available to me, I wouldn't know what it says. But what I do know as a person who lives in our society and as a member of a civil society at that, that an algorithm that doesn't optimize its values, it doesn't optimize its performance for me means that it's excluding me in that ecosystem. And that really my friends is a purpose of why I do this work. How many people and this is something again, I hate to get on the sidebar, but I feel like I have to do it because I already feel like a preacher. How many of us are quick to band products versus optimizing them to be much more diverse and inclusive. Friends, that's where I stand in this debate, how can we work together to develop better technical cadence, find the right points for policy intervention and guidance and ensure that everyday people have a right or say to what is actually being developed around them.
Dr. Nicole Turner-Lee (19:54): When we think about these bigger rock issues of misinformation and disinformation, when we think about algorithms that have misidentified African Americans as primates or are very poor at accurately defining or understanding various languages and accents and natural language processing models. When we think about the fact that one wrong decision in the algorithm on credit verification or eligibility may actually mean that that individual may never have another chance to participate fully in our economy. These to me are reasons why we're having this conversation. And I would suggest to anyone there who is out in this business of permission less innovation to avoid the tendency to fall into the block of permission less forgiveness, having to apologize that you forgot about a populations, of the effect of an algorithm on a particular population or that your products and services were more discriminatory and exclusive than you thought.
Dr. Nicole Turner-Lee (21:00): I've tried to say to technologists and companies as I begin to close that this almost reminds me of how we develop the learning that is before the machine. Machine learning algorithms don't often and always mean that they should be static nor doesn't often and always mean that it should be transactional. My friends in my view, when we've developed tools that actually are more representative and able to be accessed by more people, we actually create an economy of efficiency. When I was a little girl and I'm not going to tell my age because I just had a birthday. I used to watch three cartoons, one was The Jetsons where George Jetson used to fly around in autonomous vehicles. The other one was The Flintstones where Fred Flintstone's wife used to drive a car that had rocks as wheels. And because I was like every other African American kid at that time and others who really enjoyed Fat Albert, I used to come home and watch that.
Dr. Nicole Turner-Lee (21:57): But when I think about which of these cartoons and I'm going to exclude Fat Albert, I just had to say it because it just made me think about it, that moment that had the greatest impact on my research today is the fact that Fred Flintstone has become archaic and pretty much obliterated in terms of this analog society in which we live. And George Jackson's realities, the idea of the use of artificial intelligence, AR, VR, autonomous vehicles and robots has become more realized in my very short tenure as a child to an adult. It suggests to me that technology will always be there to help us solve some of the most critical global problems that we have. But friends just like in my book, which I entitled digitally visible, how the internet is creating the new underclass, which tends to argue that we have this flippable coin where technology can become an opportunity or a peril. We need to do the same thing when it comes to artificial intelligence systems.
Dr. Nicole Turner-Lee (22:56): And I'm happy to say that there's a lot of people who have joined the party to actually talk about ways to make AI more responsible, but we need more folks to be cognizant that the AI in which we're developing may have these unintended consequences on people who are not necessarily sitting at the table in its design or whose experiences are not necessarily understood when it's executed. We can solve this and that's why I say to all of you, as we think about this conference and the transformational aspect of AI and what scale AI is, the organization has done even in their development of their own tools to advance this type of progressive view of how we look at algorithms. Remember my voice and remember my name because I think at the end of the day, if we don't stand up and have these conversations in conferences like this, we're not going to have those conversations anywhere else. Technology is only made better, not only by the human agency that's involved, but it's also made better when we're deliberate, strategic and aware that those blind spots do exist.
Dr. Nicole Turner-Lee (24:07): So let's make these algorithms better and let's make this world in which we all live when we geek out on technology, a place that is more inclusive into disciplinary, responsible, lawful, and at the end of the day, fair. Despite fairness being a trade off, somebody's going to lose and you have to determine as a developer who is that going to be. So thank you very much. I'm Dr. Nicole Turner-Lee from the Brookings Institution. This is the work that I do, I manage our AI bias portfolio at Brookings. You can find me on our website. I'm also on Twitter and other social media platforms. I'd love to hear from you. Look out for my new book coming out and also look out for the stuff that I'm writing about this energy star rating. I can use as much advice as possible and if you got things you want to share, I'm always open to read it. Thank you so much for having me and enjoy the rest of the conference.