Panel: A Discussion on the Consequences of AI Bias
speakers
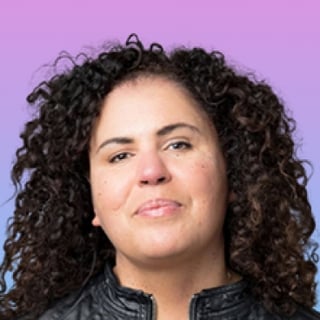
Dr. Safiya U. Noble is an Associate Professor of Gender Studies and African American Studies at the University of California, Los Angeles (UCLA) where she serves as the Co-Founder and Director of the UCLA Center for Critical Internet Inquiry (C2i2). She holds affiliations in the School of Education & Information Studies, and is a Research Associate at the Oxford Internet Institute at the University of Oxford where she is a Commissioner on the Oxford Commission on AI & Good Governance (OxCAIGG). Dr. Noble is a board member of the Cyber Civil Rights Initiative, serving those vulnerable to online harassment. She is the author of a best-selling book on racist and sexist algorithmic bias in commercial search engines, entitled Algorithms of Oppression: How Search Engines Reinforce Racism (NYU Press), which has been widely-reviewed in scholarly and popular publications. Dr. Noble is the recipient of a Hellman Fellowship and the UCLA Early Career Award. Her academic research focuses on the design of digital media platforms on the internet and their impact on society. Her work is both sociological and interdisciplinary, marking the ways that digital media impacts and intersects with issues of race, gender, culture, and technology. She is regularly quoted for her expertise on issues of algorithmic discrimination and technology bias by national and international press including The Guardian, the BBC, CNN International, USA Today, Wired, Time, Full Frontal with Samantha Bee, The New York Times, and a host of local news and podcasts. Her popular writing includes critiques on the loss of public goods to Big Tech companies, as featured in Noema magazine.
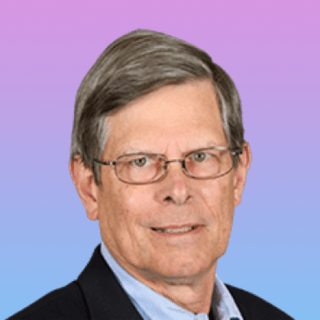
Mark MacCarthy is a Nonresident Senior Fellow in Governance Studies at the Center for Technology Innovation at Brookings. He is also adjunct professor at Georgetown University in the Graduate School’s Communication, Culture, & Technology Program and in the Philosophy Department. He teaches courses in the governance of emerging technology, AI ethics, privacy, competition policy for tech and the ethics of speech. He is also a Nonresident Senior Fellow in the Institute for Technology Law and Policy at Georgetown Law. He conducts research and publishes scholarly articles on privacy, competition policy, AI ethics and policy, algorithmic fairness, regulation of emerging technologies, platform responsibility, and content moderation. His regular commentaries on technology policy, privacy, AI regulation, content moderation and competition policy in tech appear in Forbes, Brookings TechTank, Lawfare, Project Syndicate, the Washington Post, and the Hill. He has extensive public policy experience in Washington D.C. He served as a professional staff member of the U.S. House of Representatives’ Committee on Energy and Commerce, where he handled telecommunications, broadcasting and cable issues, and as a regulatory analyst at the U.S. Occupational Safety and Health Administration. He was in charge of the Washington office for Capital Cities/ABC, served as senior vice president for Visa, Inc. and ran the public policy division of the Software & Information Industry Association. MacCarthy holds a B.A. from Fordham University, an M.A. in economics from Notre Dame and a Ph.D. in philosophy from Indiana University.
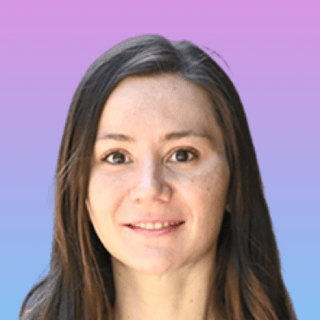
Aylin Caliskan is an assistant professor in the Information School at the University of Washington. Caliskan's research interests lie in artificial intelligence (AI) ethics, bias in AI, machine learning, and the implications of machine intelligence on privacy and equity. She investigates the reasoning behind biased AI representations and decisions by developing theoretically grounded statistical methods that uncover and quantify the biases of machines. Building these transparency enhancing algorithms involves the use of machine learning, natural language processing, and computer vision to interpret AI and gain insights about bias in machines as well as society. Caliskan's publication in Science demonstrated how semantics derived from language corpora contain human-like biases. Their work on machine learning's impact on individuals and society received the best talk and best paper awards. Caliskan was selected as a Rising Star in EECS at Stanford University. Caliskan holds a Ph.D. in Computer Science from Drexel University's College of Computing & Informatics and a Master of Science in Robotics from the University of Pennsylvania. Caliskan was a Postdoctoral Researcher and a Fellow at Princeton University's Center for Information Technology Policy.
SUMMARY
Join Nicol Turner-Lee, Senior Fellow at The Brookings Institution leads a panel discussion with Safia Noble Associate Professor at UCLA, Aylin Caliskan Assistant Professor at University of Washington and Mark MacCarthy Senior Fellow and Adjunct Professor at Georgetown University. AI enables an incredibly broad set of new use cases that are moving businesses forward. However, there have been several high-profile companies that have elected to discontinue investing in AI use cases that are especially vulnerable to bias. Recruiting, criminal justice, and facial recognition are just some examples where relying on existing training data can amplify today's prevalent inequalities. While AI systems can be game-changing, how should we think about managing the bias within AI? Join this panel discussion to hear leading researchers dissect the impact of AI bias, discuss approaches to operationalize fairness and explore how the roles of government and private industry can support each other, when tackling bias.
TRANSCRIPT
Dr. Nicole Turner-Lee (00:33): Well welcome, I'm Dr. Nicole Turner-Lee, I'm a Senior Fellow in Governance Studies and the Director of the Center for Technology Innovation at the Brookings Institution. I'm really excited about this panel today. I'm excited for a variety of reasons, I get to hang out at the scale TransformX Conference, which I've heard so much about. I'm just honored and humbled to actually be esteem moderator as part of this and participate in other ways.
Dr. Nicole Turner-Lee (00:56): I'm also excited, because I get to hang out with people that I've worked with before. The three folks that are actually going to be part of this panel have done work with me in the past, whether in a professional manner, or the paper, or blog posts, and then a few of them, maybe one of them knows me really well, and if she tells any secrets, I'm going to be really upset. But I share that to say that these are folks who know what they're talking about when we begin to contextualize not just the sociological implications of AI bias, but also the legal implications, and the other economic or political implications that come with this. So I hope you walk away from this panel understanding a little bit more outside of the technical cadence of algorithmic bias. More so, the full picture of how, when we don't get this right, it actually impacts our ecology.
Dr. Nicole Turner-Lee (01:45): So today, I'm joined by Safiya Noble, who is the Associate Professor of Gender Studies and African American Studies at the University of California, Los Angeles. She's also serves as the co-founder, and co-director of the UCLA Center for Critical Internet Inquiry. She's the author of the best-selling book and titled, Algorithms Of Oppression, How Search Engines Reinforce Racism. If you've not read that book, I would actually encourage you to do so. For those of you that may not know, she's probably one of the pioneering folks in this area who actually started putting pen to paper.
Dr. Nicole Turner-Lee (02:15): We're also joined by Mark MacCarthy, who is a Non-Resident Senior Fellow in Governance Studies at Center for Technology Innovation, which I direct. He's an adjunct professor at Georgetown University, and a non-resident Senior Fellow in the Institute for Law and Policy at Georgetown. He conducts research on technology, policy, privacy, antitrust, and regulation. All I got to say, he's one of the most productive writers that we have at CTI.
Dr. Nicole Turner-Lee (02:38): Aylin Caliskan, who is a new friend, who has published for us at Brookings on AI Bias Stream, on Natural Language Processing and Bias. She does a whole lot of things around AI, ethics, bias, computer vision, and machine learning. She is now an Assistant Professor at the University of Washington in the Information Sciences Program. So first and foremost, I want to welcome all of you to this discussion today.
Dr. Safiya Noble (03:01): Thank you. Great to be here.
Dr. Nicole Turner-Lee (03:03): I want to start with this question. Over time, AI has had increasingly ubiquitous presence in all of our lives, I think it would be safe to say that most of the things that people touch today have to do with AI. It's either being controlled by AI, or the outputs are feeding AI, right, to make it even more optimized. What we're also seeing is that companies are using AI for hiring decisions, acceptance or rejection of loans, healthcare decisions, educational systems, cynicism to the like and even public benefits eligibility.
Dr. Nicole Turner-Lee (03:38): So we bring up the idea of AI bias, and when we start talking about it. I said this the other day to somebody. I don't think we have to prove that AI bias exists anymore. But I think it's something that we need to be clear about what we're talking about and why we're talking about it. So I'm going to start with you, Safiya, because you've done some interesting stuff, really, before this became public domain stuff on why AI bias is still an area that perhaps needs to be further explored from both the research and pragmatic perspective. But why should we care about it, right? Overall, particularly in an innovative economy, where we're going to make mistakes?
Dr. Safiya Noble (04:16): Well, let me just say, Dr. Turner-Lee, that it is such an honor to get to work with you again on this panel. I've known you since I was a graduate student working on this issues, which was just a couple years ago. When I was writing and doing this work while I was working on my PhD, it was not common sense that technologies could discriminate. In fact, 10 years ago, when I was trying to make a case, in my own work, that something as banal, a very banal kinds of technology, and AI, like search engines could produce very harmful results, and that we should look at these kinds of technologies, the everyday technologies, much more closely. We should be scrutinizing them much more carefully. That was screaming into the void. Do you know? I mean, no one really believed that.
Dr. Safiya Noble (05:17): In fact, I can remember how difficult that was, you being one of the people really early on to support these ideas and my work. So I just want to say thank you for that, because it really does make a difference when you're trying to... still a decade later, make these concepts legible. As you know, my esteemed panelists here, today are also doing and their work. There are so many taken-for-granted assumptions about technology that we're really still trying to break through. So one of those is that technology is simply a tool, and that where bias gets introduced, or where discrimination happens is in the implementation, the way humans misuse it or use it, right?
Dr. Safiya Noble (05:56): But what we know, of course, now, after unfortunately, so many years of amassing evidence, and studying that evidence is that at the level of code, at the level of logics that drive different kinds of pattern detection systems, the kinds of data that are used as inputs into large scale systems, whether it's a search engine, or a loan application software, a college admissions algorithm, that the kinds of data that go into these systems is also full of historical discriminatory patterns. There are so many ways. Of course, there's just the unsupervised machine learning kinds of projects where pattern detection is happening that might even be difficult for us to recognize and understand, and so we don't know about the harms until the harms have been enacted.
Dr. Safiya Noble (06:58): So there's still so much work to be done in dislodging the mythology of what AI is. Unfortunately, I live in Los Angeles. So I live in a city close to Hollywood that is been really profoundly implicated in creating a mythology about AI, that it's the Terminator. Of course, that might be one dimension, killer robots might be one dimension of AI, or the fantasy of AI being smarter than human beings. But most of the AI that we're talking about is kind of narrow, and it's transactional, and it is fraught with very kind of crude assumptions. So I think these are the kinds of things that we have to look at much more closely. I mean, we now have thousands of researchers around the world working on these problems, where a decade ago, we did not have that.
Dr. Safiya Noble (07:50): So I think it's really important that we have the resources, the funding, the time, and the attention of people who can help us intervene upon these systems, before they really become so commonplace, that it's difficult to dislodge them, and that we, again, take for granted the superiority of the logics of these kinds of systems over the logics of what human beings might be able to kind of implement.
Dr. Nicole Turner-Lee (08:19): Yeah, no, and I totally agree. Mark, no offense to you, when I started in this space early on, there were not a lot of women that were actually talking about these issues, let alone women of color. So the fact that this panel itself begins to represent the fact that we need more disciplines, right? We need more diversity in these conversations. I think it's really a step forward, but I do agree with you, Safiya, that we tend to still focus on sort of disproving that this cannot possibly be a reality, when in fact it is, and we have to get deeper instead. Mm-hmm (affirmative).
Dr. Safiya Noble (08:50): Let me just say one thing, one of the reasons why that we know what we know about the harms of technologies is also because people who have traditionally been in the crosshairs where these technologies are beta-tested, or where they're broken, have been women, women of color, LGBTQ communities, poor people. So we are the people who are also have been in the forefront of recognizing how these systems work, because many times they're broken when they're pointed toward us.
Dr. Nicole Turner-Lee (09:23): That's right. So Aylin, I want to pick up on you, because I know the work that you did with us at Brookings had a lot to do with specific types of AI that can be more fragmented than others, right? The paper you wrote was just fabulous. It was on natural language processing tools, and I know you do more, right, to really look at machine learning applications and the extent to which they do create some bias. Talk to us a little bit about your work in the NLP space, but also biases that you may see in other areas?
Dr. Aylin Caliskan (09:50): Thanks, Dr. Turner-Lee, for the introduction, and it's an honor to be among these panelists that have made significant contributions to AI bias. Dr. Noble made a great introduction to the problems we are facing with AI, and AI bias, the AI ecosystem and its scale that we are talking about. I've been looking at unsupervised language representations, and also natural language processing applications. I'll talk about the details of these representations and applications, and I'll also briefly mention the types of problems we are facing with other supervised machine learning algorithms, such as individualized price discrimination.
Dr. Aylin Caliskan (10:36): In 2016, I started looking into how representations of words for machines are embedding deterministic biases in them, and we didn't have principled methods to detect and measure the magnitude of these biases. Being inspired by sociology and social psychology, we develop theoretically grounded methods so that we can detect and quantify these biases in representations of language that are foundations of natural language processing applications. That means that when we have these word representations, that's basically how this machine perceives the world. We found that machines are replicating all the biases that have been documented in sociology, social psychology, including gender ratio, ability-related, class-related biases, sexuality and identity-related, discrimination.
Dr. Aylin Caliskan (11:40): As machines are learning from historical data, that also includes historical injustices. Accordingly, when machines are learning these unsupervised models, they figure out these patterns of injustice and discrimination, and then, they start replicating them, and eventually amplifying them both for AI models and in society. So it's turning into the snowball effect that is then impacting all social processes and the structure of our society. Because right now, AI is so light scale being used in domains ranging from education, for example, college admissions, PhD admissions, essay grading, or health, and resource allocation. These have all been shown to be biased applications of AI. Immigration decisions, job candidate assessment, employment, surveillance, law enforcement, and many other critical domains that are making consequential decisions about individuals lives.
Dr. Aylin Caliskan (12:48): When these natural language processing, or AI systems learned biased representations or models, they end up replicating these for the individuals at light scale which causes an unprecedented speed, accelerated speed of replicating bias, both in AI and society. One other example that is not related to natural language processing is for example, ride-sharing applications. We focused on data from the city of Chicago because of transparent mandates, they were making data about ride-hailing public, and studying large scale data from ride-hailing applications, we saw that this advantage neighborhoods in Chicago were further disadvantaged with higher fair pricing as individualized price discrimination algorithms are learning to avoid certain areas, and the relative demand compared to supply seems higher, disadvantaging certain populations further. These are some of the examples, and I will be happy to go into more details as this panel progresses.
Dr. Nicole Turner-Lee (14:00): Well, you look, I'm just going to say we should end the panel now, Mark, [inaudible 00:14:03] because, I think we've actually discovered [inaudible 00:14:06] show it up. I think what's so interesting about it, we've heard so far a perspective of sociologists. Aylin sits within the technical as well as a social psychological space. Mark, you're a lawyer, right? You sit squarely, also, in addition to all that you do in this public policy space. Speak to us in terms of just again your perspective on this legal framework. Why is it that these examples that we're hearing become concerning from a policy perspective.
Dr. Mark MacCarthy (14:35): So thanks very much, Dr. Turner-Lee for having me. It's good to be on this panel with you. I have to correct a slight misunderstanding. I'm a philosopher and an economist, but I'm not a lawyer. I just play one in panels like this.
Dr. Nicole Turner-Lee (14:51): You're right.
Dr. Mark MacCarthy (14:52): So far, I've been able to get away with it, but I think full disclosure requires me to confess, this is being recorded. So someone may be able to hold it against me in the future. I'm also actually delighted to be in the minority in this particular panel, it's a sign of progress, that when White guys don't take the lead in discussions like this. Let me start off by giving a couple of examples of the kind of problems that we've run across. These are all famous ones. So I'm not going into any technical detail in explaining this.
Dr. Mark MacCarthy (15:30): Let's think about the allocation of medical care. There was a statistical program that was developed for allocating the need for extra medical care. It was put into the field by the software developer without conducting any disparate impact analysis. After it had been out there for a while, and hundreds of thousands of people had been affected by it. An independent researcher found for disparity, the racial disparity using a pretty routine test, and is now working with the software company to improve both the accuracy and the fairness of the statistical program.
Dr. Mark MacCarthy (16:11): A second example, facial recognition is notorious for disparate levels of accuracy. It's nevertheless used extensively in law enforcement and other private sector uses, despite that known disparate impact that it has. This leads, of course, to wasted police resources and dangerous to the civil liberties of innocent people. Employment, the famous example there, is derived from Amazon, where they went to their own internal employment records and said, "We're pretty good at algorithms, let's take the employment records that we've got and try to develop an algorithm that will allow us to hire the really qualified people and not hire the less qualified people." They couldn't get it, despite tweaking it for a long time to do anything, but return their predominance of White guys, which was, of course, a function of what the company had been doing for years, and years, and years in their hiring practice. The good news is that they didn't put it into use and just deep-six the entire project.
Dr. Mark MacCarthy (17:21): The last example, is a Propublica Study of recidivism scores, which are heavily used in decisions involving parole and sentencing, and they showed that it involved substantial amounts of bias, which obviously affects people's life chances. So what do all these examples really show? Among other things, they show that very simple statistical tests can reveal when an algorithm has a potential disparate impact, and might be adversely affecting people's lives in a way that when we think about it, we really don't want to do. The initial measure that this suggests for a legal requirement is that when an algorithm, AI algorithm, or even a less sophisticated version of them. If they're going to be used for these areas where the use has a significant impact on people's lives, when it's consequential for their life chances, the minimum that should be done is a thorough, disparate impact analysis.
Dr. Mark MacCarthy (18:37): We can go beyond that, there's more that needs to be done. There's a lot more protection that needs to be put in place to make sure that people's civil liberties and civil rights are protected. But let's start with the low hanging fruit. Let's go look and see if we have a problem, and if we have a problem, we can have informed discussion about what to do about it. As the management consultants always say, "You can't manage what you don't measure." So let's go measure this, and then have a conversation about how we set about fixing the problem.
Dr. Nicole Turner-Lee (19:08): Well, Mark, I want to stay on you for a moment, because at Brookings, we're going to be coming out with a paper shortly, and a series of papers around Privacy As a Civil Right, and this whole idea of applying disparate impact tests to the algorithmic economy. The question I have for you, though, is when I did the paper that we worked on at Brookings a few years ago, we sort of defined bias around similarly situated people, places and objects having differential treatment.
Dr. Nicole Turner-Lee (19:33): As I've thought about that model over the years, I begin to think about a lot of the work that Safiya has even put out there where some of this differential treatment is, perhaps the likes interests preferred preferences of people, where the micro targeting or micro surveillance may be a little more innocuous than others, when it becomes more difficult is when we see the disparate treatment where collective groups in the case of which you gave women at this particular company would have been denied access to better paying jobs or rides that are being diverted from communities of color.
Dr. Nicole Turner-Lee (20:08): The problem here, and I'll start with all of you all, I want all of you to answer this, and I'll be [inaudible 00:20:11] to get to Safiya first, and then go with Mark, is that no one can do that type of comparison on the internet. Because in some ways, it's so opaque and the inferential economy has become so strong, that they don't even need to know whether I'm a Black woman that loves blue dresses. They may actually find that I'm a Black woman by my purchasing behavior, my reading material. The type of time that I get on the internet to do certain things. How do we begin to sort of address and remedy those concerns, because this is what I find so interesting, that it's not the clear linear pathway to a civil right or disparate impact violation, because many people don't even know they're being discriminated against. Safiya, I'll go to you, Aylin, and then Mark.
Dr. Safiya Noble (20:56): Yeah, I mean, I regret that I spend all of my waking time thinking about the answer to that question. I will say that I think part of the challenge here is that you have really like six companies who dominate the internet, six US-based companies. Let's keep this confined to the internet, we experience the United States, because I think that's probably most relevant right now. When you have kind of six monopolies who control the information stack. Let's say, that kind of the software platform interface that you're dealing with, but they also, in many ways control the infrastructure, the digital infrastructure. They're able to amass and collect data about you, in many, many ways, far beyond just to kind of your own smartphone applications that you're engaging with, right?
Dr. Safiya Noble (21:53): So this, I think, raises a number of challenges around remedy, how do you address the kinds of discriminatory harms that can come when there are really no competitors in each of the verticals that these companies sit in, from social media to search to shopping, like Amazon and other kinds of infrastructure, Amazon and Google? So I think these are places where we need very robust policy. I mean, I appreciate so much, Mark, your work around antitrust, because I think those are the conversations that also need to be out in the forefront. When we're talking about harm, part of that is a monopoly control of markets. Part of that is the blocking of new entrants, who might be able to, let's say, create other kinds of products that would protect... let's say, create some modicum of privacy, although, you can't ever do anything privately on the internet, that's just like a misnomer, as well. But that's another panel for another day.
Dr. Safiya Noble (22:59): So I think, we have to think about the kinds of supply chain effects of, again, all kinds of companies that can't participate in the digital economy, and who also provide some of the kinds of resources that we need to not have us be just kind of sequestered into whatever the dominant players serve us up. So it's a complex environment. I think antitrust is one of the really important remedies that we have at the heart of some of these conversations. I really argued, at the time that I was writing the book Algorithms Of Oppression, I wrote a lot about the Federal Trade Commission, and why I thought that was such an important site, while everybody else was writing about the FCC, and Section 230. I was like, "No, but when you have monopoly control, that's part of the reason why it's very difficult to intervene here in these spaces."
Dr. Safiya Noble (23:54): We have almost no oversight in the tech industry. I think this is another thing that when we think about the way in which so many digital products are part of now, the way we think about a public good, or we think about the way the economy works, or we think about access, and inclusion. Well, some of the kinds of projects that my colleagues have talked about here today are matters of life and death. They're matters of well-being. They matter in terms of quality of life. They're really important, fundamental kinds of human and civil rights that we think should be protected.
Dr. Safiya Noble (24:34): Many of these technologies really have no interface or oversight with civil rights, human rights, laws and paradigms. So I think we have so much work to do, if we hadn't... there are many different proposals. I mean, people think about things like, "Should we have the equivalent of a Food and Drug Administration over the tech industry?" "Should there be a duty of care?" This is Danielle Citron's work, right? Around executives of these companies, "Should they go to prison, if their technologies harm people?" We don't have any of these kinds of models the way we might have in big pharma. The way we thought about Big Tobacco in the past and other kinds of large scale industries that have a huge effect over the public health and well-being of the public.
Dr. Safiya Noble (25:29): So I think these are some of the ways in to think about different kinds of remedies, certainly greater oversight, greater civil rights protections for people. Price surging and discriminatory pricing, that's just like a 101. I mean, right out of the gate, we have laws on the books to protect people, but we don't really have a lot of enforcement mechanisms right now.
Dr. Nicole Turner-Lee (25:53): Aylin, did you want to chime in?
Dr. Aylin Caliskan (25:58): Yes, these are great points, especially about big tech, and how they are monopolizing the entire domain. Since big tech has access to all the data, and citizens, internet users think that the services big tech provides is free, except that their data is what is making this possible in their optimization process. As they are trying to optimize for targeted advertisements, internet users are consuming social media, all kinds of applications that are powered by AI. And then, this in turn affects our values, potentially, our cognition, the way we behave, and so on. We don't have any regulation about the harmful side effects of big tech and these AI systems.
Dr. Aylin Caliskan (26:48): So regulation is one of the things that can help in the short term. But in the long term, society needs to be aware of these problems, understand the privacy and fairness trade-off. As we reveal more sensitive information about our lives, our behaviors, our ideas, as that these are being used, then these are also impacting social groups causing disparate impact, in certain cases, disparate treatment. Regulation is one way to remedy this, raising awareness about these issues would help us as a society, understand what might be going wrong here. We can also try to change our practices so that they don't keep reflecting discriminatory actions, behaviors that end up in these AI datasets.
Dr. Aylin Caliskan (27:41): As for example, hate speech is propagated on the internet, or all kinds of bias language, or images are shared on the internet that end up as part of large-scale natural language corpora, or computer vision datasets, these are being used by big tech to generate or train these models. As we are more aware of our practices that might be harmful in the long run, we can also try to change these things. It will be a small contribution from each one of us, but at large scale, it will have an impact. Other than that, we need a diverse set of AI developers.
Dr. Aylin Caliskan (28:24): As Dr. Nobel earlier mentioned, people that have lived experience with discrimination and bias know what to test for, because these systems, especially don't work with the social groups, that are in summary, basically, anyone that belongs to a social group that is not White men in general. And based on this value-sensitive design with a diverse set of AI developers, interdisciplinary researchers ranging from philosophy for AI ethics to computer scientists, information scientists, sociologists, psychologists. If we come together, we can analyze this AI ecosystem that is very complex and large-scale better.
Dr. Aylin Caliskan (29:08): As Dr. MacCarthy mentioned, as interdisciplinary researchers come together, we can first develop methods to detect and quantify these harmful side effects of AI. Once we are detecting them, then we can start doing something about it. One way to mitigate these problems would be developing methods that also promote more equitable fair systems. So there's a lot of research that needs to be done in this area. These are just some of the few things we can start doing now, because we are facing an immediate light scale problem.
Dr. Nicole Turner-Lee (29:49): Yeah, no, and I agree. I want to actually get back to what some of what Aylin has said, because Aylin, you're reading my book, okay. You're sitting here saying a lot of stuff that I think has been very dominant in the public sphere. But Mark, before we go into really unpacking trading data, and our made of time, as well as some of the implications going forward. Antitrust, I mean, this is an audience that is watching this that may not be fully entrenched in the DC space, but has heard about the attempts to sort of regulate really in tech. Going back to Safiya's comment, though, do you think that antitrust regulation is the answer to this, or going back to your prior comment? Is it really having a deep dive on civil rights advocacy so that we can ensure that we're creating not just responsible AI, but lawful AI?
Dr. Mark MacCarthy (30:38): So thanks pretty much for that question. It really goes to the heart of a lot of these issues. But first, I want to acknowledge the shout-out to philosophers that just made these. It's a field that I trained in, and I still love. But these issues are not technical issues, and they're not really just legal issues. They're normative issues. The people who tend to be pretty good at analyzing those kinds of questions are philosophers. So I'm a great believer in having them involved in these discussions of AI fairness and discrimination, and what to do about it.
Dr. Mark MacCarthy (31:15): On the question of antitrust, I think it's an important thing to do to promote competition in these industries. In fact, the book that I'm working on for Brookings on Regulation of Digital Industries, calls for a sectoral regulator, who will have, among other responsibilities, the promotion of competition. They would also be responsible for promoting privacy, and encouraging good content moderation in the sector, and while we're at it, why not take on this issue of fairness, and discrimination as well. The reason why you have to have these different and separate policy missions is that just promoting competition won't really do it. It won't solve the problems of privacy. It won't solve the problems of content moderation, and it won't solve the problems of discrimination.
Dr. Mark MacCarthy (32:11): The best example, can be found by thinking about, "What would happen if you had 50 Facebooks?" Would they suddenly invent new ways of protecting consumers privacy? Almost certainly not, they would still have an interest in targeted advertising, because that's how you make money from social media. Charging people, a cash amount in the face of the free service offered by Facebook would simply not be a good market proposition. So all the competitors are going to be in the business of exploiting consumer data, but with the result that more competition leads to more privacy risks, not the availability of better privacy alternatives. So you need a separate measure to enforce good privacy, not just the promotion of competition, and the same is true in the protection of people against unfair and discriminatory treatment.
Dr. Mark MacCarthy (33:07): Last comment on this issue of, what about discrimination that seemed to take place behind people's back? That's really related to the issue that's been studied for many, many years of statistical discrimination where you're actually not using a variable for race, or gender, or some other protected class, in the way you're analyzing data, or the way you're making decisions. We use other variables that seem to be correlated with that, and you're trying to do it to achieve some sort of corporate, or institutional purpose. But in the process of using variables that are correlated with race or gender, you wind up making decisions that have a disparate impact on people.
Dr. Mark MacCarthy (33:57): Now, you can't solve that problem by simply looking at the interior of the algorithm and say, "Where's that variable for race?" It's not there. The way you get a handle on that kind of issue is through analysis of the outcomes. That's what's been done in employment law, where people have been living with the rule of thumb of 80% for many, many, many years, which says, "If you hire 10% of the White applicants, then you really shouldn't be hiring less than 8% of the African American applicants." If you're below that, and you're an employer, you know enough now to look at your employment practices and your hiring practices and saying, "What am I doing wrong?" And then taking steps to see if your employment criteria are skewed in ways that continue to have that disparate impact.
Dr. Mark MacCarthy (34:55): Again, that's just a minimum of stuff that needs to be done, but it's the kind of thing that right now is enforced in areas where there are anti-discrimination laws, protecting certain categories of people, for an agency like the Federal Trade Commission, that could be authorized to extend those kind of protections to new classes of people, not the current classes that are protected under current law. That's where issues like price discrimination might come in. We've got different classes that would be adversely affected.
Dr. Mark MacCarthy (35:27): Last point, the good news is that our friends in Congress are looking at increasing the resources available to the Federal Trade Commission. The proposal that's being introduced in the Congressional Budget discussion is for the FTC to get an additional $1 billion, roughly tripling its current budget. No new statutory authority to extend what they do, but plenty of new bodies to go forth, and do good things, we put that together with the FTCs authority to promote competition, their current authority under consumer protection, to protect privacy, and their likely authority under content, moderation bills, to promote transparency and other forms of due process protection. You've got an agency that can cover many of the ills that face us in the algorithmic discrimination area. One with enough resources to actually get the job done.
Dr. Nicole Turner-Lee (36:29): I like the way that all three of you have sort of outlined the public policy perspective, because this invitation to speak at this conference, and to work with scale AI around this content really it matters because I think this is where many of the mistakes happen, because sometimes developers don't know that there's this whole ecosystem that's concurrently happening around them as they develop. But I want to be sure, because we're going have a lot of developers who are watching this panel, that we give them some good etiquette, I call it some good algorithmic, or machine learning hygiene, so that they can actually avoid some of the missteps that actually result in poor close opportunities on various populations.
Dr. Nicole Turner-Lee (37:06): So Aylin, I want to come to you, and then I'll go to Safiya, who has spent a lot of time with technologists, if you were able to give sort of like the Good Housekeeping Seal of what folks should be focusing on first, what would it be? Would it be understanding the compliance measures? Would it be focusing on the training data? Or would it be making sure that you're continuously evaluating, that you're not creating any type of harm for the user? So I'll start with you, Aylin, and I'll go to you, Safiya.
Dr. Aylin Caliskan (37:35): That's a great and complex question. You already hinted to some of the potential solutions. But looking at the AI lifecycle, first of all, why is an AI system being developed? Who is it being developed for? As these processes are taking place, is everything compliant? For that, we need to have some kind of a standard so that people know what kinds of things they should be watching for. After that, we are developing methods for these systems to test disparate impact or bias related to any social group that is represented in society, especially underrepresented groups and marginalized groups, because those are the ones that are most inaccurately, and in a biased way that are represented in these datasets, and systems.
Dr. Aylin Caliskan (38:34): After that, we need more experts that need to understand datasets. We cannot just use whatever data is out there without having consent from individuals that are contributing to these datasets. After that, we need to be able to measure the representation of social groups and try to do something about underrepresented groups. Because, as you mentioned, this is a dynamic problem. As we create more biases, underrepresented groups will still be smaller in size. Accordingly, we need some kind of a technical solution or other types of remedies to deal with this as well.
Dr. Aylin Caliskan (39:10): We need to be aware of the fact that bias is not some kind of a bug that we are observing. It's the default. These systems are learning from us, learning from the noisy data that is provided to them on internet platforms. Keeping that in mind that bias is the default and we need to do something about it otherwise, the system developers are creating are going to perpetuate harmful effects, it should be at the forefront of our principles. Otherwise, it's going to keep advantaging certain groups, and we won't be able to solve the problems created by AI in an accelerated manner, if we keep doing the same things we have been doing. These are some of the recommendations.
Dr. Nicole Turner-Lee (40:01): No, that's excellent. Safiya, how would you add? I mean, if you got developer audience here? What would you say to them? I think Aylin has pointed out some really good points.
Dr. Safiya Noble (40:09): Listen everything Dr. Caliskan says is correct. I think I would only add to this that developers... I know many of them and I have worked with programmers for a long time, oftentimes that work is so compartmentalized in particular, that thinking about the broader effects or broader impacts of how that work comes together, and whether some of that work should be done is really also part of the work that developers can do, and that we need them to do. So if it's Microsoft workers walking out and saying, they won't put their labor in services of certain types of projects. If it's Google workers, Facebook. I mean, many technology workers have said, "I've reached my own personal moral limit to what I'm willing to do." This, of course, is why having an understanding of kind of the politics of our work, all of us, is really important.
Dr. Safiya Noble (41:12): I mean, think about what 100 years of working on technologies like cars, and airplanes, and trains that run on fossil fuels has done, and now the effects that we are dealing with in terms of the climate crisis. I mean, at some point, everyone's just working on their knob. But there's also the bigger picture of, "Do we need this? What will be the long-term effects for the planet?" I mean, we haven't even talked about the environmental impacts of large-scale data modeling. This research is here, I mean, we think about our colleague, Dr. [Timini 00:41:51] Jabiru being fired and working with our colleagues at the University of Washington, on these various issues of the environmental consequences of the work and what the limits should be.
Dr. Safiya Noble (42:05): So even when developers get fired, for doing that work, or computer scientists get fired for trying to put the brakes on, that matters. We have now, at least, in California, I can say, for people working in Silicon Valley, thanks to the work of a remarkable Black woman. We have whistleblower protections now, for developers and for people working in the tech sector, who can speak up and speak to the public and share out. Think of all the people who did that in terms of the shattering through the falsehoods of what the tobacco industries sold us as a bill of goods. Now, we know, so many people know that we're kind of on the wrong side of history in terms of public health. These are the kinds of conscientious objectors we also need in the development community. Those will be people who will, I think, be leaders in helping us think about where the limits should be, and what alternatives could be.
Dr. Nicole Turner-Lee (43:13): Yeah. Mark you've got one minute, and being in the minority, you also have the last word. What would you say to developers, as sort of a hint of suggestion that combines what we talked about with them?
Dr. Mark MacCarthy (43:28): My fundamental message would be, you have agency. You're not a victim here. You're not powerless. Technology is not something that happens to us. It's something that we do, and we do it largely through the institutional roles that we play as part of our work. You do have agency to object, to complain, to focus attention, and largely you have the knowledge that many of the people outside of your institutional setting don't have, which is enormously valuable for the public to know about. If there ever gets to be a good regulatory agency, something that a good regulatory agency would need to know about as well.
Dr. Nicole Turner-Lee (44:07): I have to say first of all, thank you to three of you, Dr. Noble, Dr. Caliskan, and Dr. MacCarthy, who is not a lawyer, but since he's a philosopher on this panel. I think for those of you who are listening to us, I think the key thing that you took away that I definitely took away is that this push poll when it comes to tech and civil society could also find itself in collaboration, and collaboration around interdisciplinary perspectives at the table, demographic perspectives around the table, and really having the conscious as Mark said, the agency to determine whether or not the tools that you're putting out are fair, ethical and lawful.
Dr. Nicole Turner-Lee (44:46): I think the other thing I would like to just point out as people wrap up their hearing of this panel is at the end of the day, our technologies are put in context, and they've deployed among real people who have lived experiences outside of our labs. So it's important that we think about that as well. Finally, I love what you said, Mark, about agency. We do have the right to ensure that this technology is actually done for the public good, and not necessarily for our profits.
Dr. Nicole Turner-Lee (45:10): With that being the case, I want to thank everybody for joining this panel. I'm Dr. Nicole Turner-Lee from the Brookings Institution. I too, have some ideas on algorithmic bias that will come out soon as part of an [inaudible 00:45:21] rating that I'll be putting together pragmatically, then I also have a book coming out on the US Digital Divide. Thank you again for joining us, and you can find all these three wonderful people in the public domain. They're online. Thank you.