Developing Realistic Approaches to Deploying ML in Federal Environments
speaker
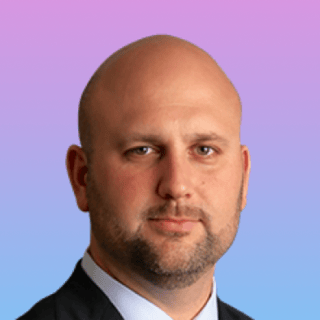
Steve Escaravage leads Booz Allen’s Analytics practice and Artificial Intelligence (AI) Services business, serving clients across the defense, civil, and intelligence sectors. As a leader in the Firm’s Strategic Innovation Group, Steve also leads the Firm’s investments in data science, machine learning, and AI. Areas of focus include machine learning operations, cognitive automation, and high-performance computing. He holds an M.S. in operations research from George Mason University and a B.A. in mathematics from Rutgers University.
SUMMARY
Steve Escaravage, Senior Vice President at Booz Allen Hamilton and Mark Valentine, Head of Federal at Scale AI, discuss how the Artificial Intelligence (AI) and Machine Learning (ML) community can help federal customers build, deploy and operationalize realistic AI/ML capabilities that bring tangible and measurable wins, within the strict compliance and governance limitations of federal IT environments.
TRANSCRIPT
Mark Valentine (00:00): Hello everyone, and good morning or good afternoon, depending on where you happen to be. Welcome back to TransformX, I am Mark Valentine, and I lead the Federal team here at Scale AI, and I'm really excited to continue our discussions focused on Artificial Intelligence and National Security. Now, in some of our previous sessions, we've looked at how government leaders look at AI and ML, and how policy affects their ability to achieve these goals, and I hope you found those sessions valuable so far. I know that I've enjoyed them, but I'm really looking forward to this next discussion. We're going to continue to live recording that we first started, but we're also going to start exploring some specifics on how to deploy innovation in the government market. So thankfully, we have a real expert with us today to help us with that task, and that is Mr. Steven Escaravage, who's the Senior Vice President of Analytics and AI at Booz Allen Hamilton.
Mark Valentine (01:12): So in this role, he drives the operational integration of data science, analytics in AI and ML across multiple market sectors in the public space, and he leads the firm's analytics vision, strategy, and technical delivery across all markets. Now, this is not his first rodeo, prior to this role, he's led strategy formulation and delivery across multiple sectors from Defense, Energy, Health, and International Business, and he's an expert in applied mathematics, as well as optimization and data management. He holds a Bachelor of Science in Mathematics from Rutgers University, and a Master of Science in Operations Research from George Mason. So Steve, thanks for your time today, and I'm already looking forward to this discussion.
Steven Escaravage (01:55): Mark, thanks for having me.
Mark Valentine (01:57): Yeah, we're really excited. So Steve, I want to start with the big picture, and I know that you've had a distinguished career helping governments and large corporations make better use of their data, even before this current craze about AI and ML, and you've been helping them focus on this. So can you give us just your high level assessment of where you think AI and ML are in the current federal marketplace?
Steven Escaravage (02:22): Yeah, I think it ranges from immature in some areas, to fairly mature in other areas, there's established programs in the public sector for Federal Government, that have been using these techniques for many years, for things like enterprise search, signal detection, control systems, so it's not a new capability, and I think that's a surprise maybe to some folks outside of the public sector area, but there's also a flurry of new start programs, a lot of those are centered in language modeling, computer vision, we've seen this rise over the last half decade to decade, and more recently, autonomy.
Steven Escaravage (03:04): And so the sophistication varies based on what I've seen, it depends on the mission, the customer and the agency, and just like anything else, the amount of investment that they've had, and also the stability of the nation, a lot of the organizations in the public sector, they have to change with the times, and so their mission has fundamentally changed, and they have to weigh investments in these new emerging technologies with continuity of mission on the day-to-day basis.
Mark Valentine (03:36): Got it. Now, Steve, we've talked to several military leaders as well as some elected officials from the US here at TransformX, and we've talked about how, perhaps in the past, the government-led research and development was leading the market, whereas it appears that in this area AIML, that perhaps the private sector is leading the research and development innovation, and the government is following. Do you think that's an accurate assessment?
Steven Escaravage (04:07): Yeah. I think the one thing that is a little bit interesting about the federal government is the large research enterprise that they have, and it's one of the ways that the public sector is a little bit different, and the private sector reserves large, basic and applied research organizations like DARPA, NIH, the National Science Foundation. But I do think it's fair, and many of the leaders of those organizations will readily admit that, over the past few decades, based on insufficient research in RnD funding, an incredible investment in the private sector, that for maybe the first time in generations, we're seeing, especially for this technology, the innovation is coming from outside, it's coming from private sector, from commercial use cases, and for the public sector, for the government to be successful, we simply cannot ignore that investment and we need to channel it back into the public sector.
Mark Valentine (05:08): Right. Yeah. That's great. So Steve let's move to this next topic I like to get to. So if it is true that government is not necessarily building or innovating in this area, that implies that they are adopting innovations from the outside, and in your role in Booz Allen, you guys have led in helping the government integrate and adopt these capabilities, into all sorts of government operations, including tons of AI and ML capabilities. So in that role, I'm sure that you were used to, I would be polite and say, wrestling with large formidable bureaucracies and complex acquisition systems. So can you give us a sense of the big picture, what's going well in this area of government adoption of AI and ML, and then alternatively, what is not going so well, what some of the friction points are?
Steven Escaravage (06:00): Yeah. And just for context, I've seen about 150 programs, up to now across the public sector.
Mark Valentine (06:09): Only 150?
Steven Escaravage (06:10): Yeah. There's a lot of programs out there. I mean, in some of these organizations, they have been at it for some time, and it's always difficult given the nature of some of the work that we do to broadcast exactly what's being done, but maybe I'll take that question in a couple parts.
Mark Valentine (06:30): Okay.
Steven Escaravage (06:30): I think overall at the top line level what's going well, is that there's progress, and there's real momentum, it was just, probably back in 2017, when the Pathfinder Initiatives, the DoD, and some of the executive departments, really started re-energizing interests and directing funds and activity back towards AI and Machine Learning, and then followed by the executive orders, and then the National Security Commission on Artificial Intelligence.
Mark Valentine (07:04): Right.
Steven Escaravage (07:04): In my view, it's probably the most important document that we'll see in this area for at least a decade, and it has really codified the activity and the call-to-action around this. So I think most importantly, top line that's what's going well. You mentioned, what are some of friction points or what are some of the challenges that we're seeing in the space? And it's really three key things, and people won't know these, the audience won't know these, but it is these three things in order, which is, it's access to real world data assets and data sets that can be used to train AI systems, it's access to approved computing environments that are configurable, scalable to support the systems that are being built, and its accreditation ATO, and ability to deploy systems in real world operations, I mean, those are the sources of friction in the process today, but there is reason for optimism because of all the activity, because of the foundational investments being made, and so I'm excited about the future.
Mark Valentine (08:19): Awesome. So Steve, you mentioned three things there, and I will summarize them as data, compute, and policy, just at a high level. So those to me seem like three things that drive successful projects, but can you give us... are there any other themes that you see because I'm assuming you've seen some successful projects, and you've seen some that have been less than successful, or as we'd like to say, opportunities to improve. You mentioned those three things, so I understand that those are probably there in all of the successful projects, but are there any other themes that you've come across and say, "Yeah, in all successful projects I've seen, here are some common themes." And then again, conversely in things that haven't gone so well, are there any common themes?
Steven Escaravage (09:06): Yeah. Thank you for that question. As I mentioned Mark when we first talked, this is like occupational therapy getting to share some of this feedback and, I do see a pretty clear recipe or blueprint for success. I tend to work in three years, and so I think it's three things again, it's focusing on discreet challenges, and discreet use cases versus these open-ended, we're going to introduce AI and ML and we could achieve great things, it's the discreet use case, it's really understanding, what does success look like? What is the actual measure of success that the user cares about? Or, how is it articulated in terms of the mission impact? And then finally, probably the hardest one is, the technological approach, the integration approach, the integration pathway is achievable in today's environment.
Mark Valentine (10:09): Got it.
Steven Escaravage (10:09): I'll maybe just pull those apart a little bit if you will.
Mark Valentine (10:13): Sure.
Steven Escaravage (10:14): And I think the first piece is, around defining the challenge and defining what success looks like? What I've seen is, most of the pure capability-based programs, we've seen some innovation that we're going to deploy it, and great things are going come from that, most of those programs are failing to launch, they get stuck because they are too focused on what can be built versus what the user needs today, and it's hard to get their bandwidth, they're executing existing missions. And so they get stuck in this pilot purgatory where there's good technology, developed by good organizations, implemented correctly, but it is difficult for the user to make the trade off between incremental value today, and the disruption to continuity of mission today.
Steven Escaravage (11:05): And everybody wants... everybody's saying, "Well, but the future benefits are going to be great." It's harder when the mission today has so many demands and there's technical debt today that's not being met, so that's one piece of it. And then I think the second piece around that achievable integration pathways, this is, I find the most confusing, especially when folks are looking into the public sector, who have mostly private sector, or research experience is that, fortunately and unfortunately, the information architectures in environments that we work in, in the public sector space, are some of the most complex controlled, and in many cases, secure environments that have ever been developed, and there's just no easy pathway to rapid modernization and cut over.
Steven Escaravage (11:59): There's lead time that's needed to accomplish that, and so I find that the teams, the companies, the vendors who are working backwards from today's constraints, and trying to determine, how I can take my innovation, how I could take the value that I can provide to the end-user and deploy it against the constraints of today, while providing a pathway for modernization, those are the ones that I see having more success, and also the ones who bring their information security leads to the first meeting. A lot of times folks will want to bring their amazing chief scientists and chief technologists, the hard question is, how do we integrate again into the available infrastructure? Bring the [inaudible 00:12:43] folks along to meetings.
Mark Valentine (12:45): Absolutely. I couldn't agree more Steve. And by the way, I'm going to steal your phrase that you coined, "Pilot purgatory." I've seen that happen quite frequently, and I'm also struck by what you were saying in successful projects, it reminds me a little bit about some basic management principles, I know in the flying world, when we used to develop objectives for a training operation, we used to use the acronym SMART, specific, measurable, achievable, realistic, and time-bound goals. And it sounds like that's your prescription for successful projects in this area as well.
Steven Escaravage (13:22): Yeah, definitely, a good old fashioned system engineering, and it applies in this space. And I think that the more that we can engineer back from the problem, instead of just throwing technology a problem, the more success we're going to have.
Mark Valentine (13:38): So we've talked a lot here at TransformX, both in our federal track, as well as our commercial track, about the ethical use of Artificial Intelligence, and especially when we start talking about national security context, it's a very important topic. I know that you personally, have been vocal in the past about the need to focus on ethics in this domain. So can you give us a sense of how you think about ethical AI development when developing solutions for your customers?
Steven Escaravage (14:08): Absolutely. And Mark, thanks for bringing the question up so early in the conversation, I think that's the right place to have the conversation. Look, I think it's incredibly important, I think it's table stakes for our field, our industry, it's the only way to build trust in the systems that we're designing and integrating and building, and trust is the only way to adoption. So I think that, again, this is something that everyone needs to take seriously. And for Booz Allen, it's not a new topic, we've been discussing and implementing controls probably all the way back to 2015, at least since 2015. And in many ways over the last few years, it's transitioned from a proactive exercise, and in some cases it's theoretical exercise too applied. We have the first contract in the history of the Department of Defense to require formal alignment with the DoD ethical principles, and so that was awarded in May, 2020. And so when you get a contract that says, "You will adhere and align to these principles," and as the signatory you've got to sign it, it takes it up to an even higher level.
Mark Valentine (15:24): Yeah. Great.
Steven Escaravage (15:26): Yeah. And so we've had some real run time, looking at different projects, working on some of the most sensitive projects in the space. And I would say, just a few opinions, personal opinions based on my experience is that, in general, the use of the AI in an effort doesn't change my opinion on whether or not something is ethical or not. I think that any emerging technology can be used inappropriately, if it's not guided by values. And so what we've had to do, is review work on a case-by-case basis to ensure that it does align with our values. However though, there are unique aspects of the AI, especially large machine learning-based implementation that complicate the risk assessment, and management, and governance of these efforts. You have to sign appropriate goals when you're building systems, the sheer speed and scale can lead to unintended consequences, and some of the methods are difficult to understand, and we've talked about this as an industry, and I think that everyone recognizes the engineering challenges.
Steven Escaravage (16:41): And so what we've done is we've implemented a process for all programs across our firm, that we're delivering on behalf of our customers that use AI or ML, we have a process that every program goes through to make sure, a, it aligns with our values and b, that we have a continuous process to understand, to continue to make sure it aligns with these values, and implement the engineering controls, by design into the process, so that we can avoid some of the risks that have been well socialized and published. And I can give you two examples on that, let me take a break. Does that make sense to you Mark?
Mark Valentine (17:24): It completely does Steve, and what I really love about your approach is that you highlight the continuous nature of this, because I think we all recognize that whether it's an algorithm, or a model, or whether [inaudible 00:17:36] somebody is building, it's not just a fire and forget, or one-time-use type activity. And I think that requires all of us, whether from industry, from government, to make sure that this is a continuous process. That's great. And so if you have examples? Yeah, please continue.
Steven Escaravage (17:53): Yeah, I'll give you two examples back on that same line is, when we started down this pathway, what we realized is, we need a pretty detailed risk ontology, and it needs to be mapped into first and second order, and higher order effects, not just to the nature of the work that we're doing, but to the people. To people working on the project, to people that this might impact if it's used in the real world, and even beyond that, and to less direct influences. And so having folks sit down and develop an ontology, I find in most organizations that we support, when we come in day one, and we say, "Can we please have your risk ontology and your access to the repository where you've cataloged known risks related to AI implementations?" That's still an area that people are investing in.
Mark Valentine (18:46): Yep.
Steven Escaravage (18:46): And it's an area that we take pretty seriously. And I think building that repository of knowledge over time, as this becomes a more mature field and area of technology, I think it will benefit everyone.
Mark Valentine (19:01): Excellent.
Steven Escaravage (19:01): And then I think, second, some of the first projects, where we used Machine Learning-based methods, years later, I would check in on those programs for customers that maybe we weren't supporting anymore to find out that they were still using some of those systems that we had trained years before. And that very different environmental conditions, and so we put together this concept of a delivery specification. So when we build models and systems, when we help integrate them on behalf, working with other partners in the space, we make sure that we document, what's the purpose of the system? The intended use, any dependencies and limitations, and provide the context so that someone later, who's reviewing this system, as people change, and organizations, and new people come in, they have that specification that defines why the system was being built and how it was intended to be used. I think this is something that we should try to standardize across the community.
Mark Valentine (20:08): Yeah. So you're channeling a little [inaudible 00:20:11] there with, start with why? That's great. I think that's fabulous, Steve. So I think I know the answer to this question beforehand, and maybe it's elementary, but who is responsible for AI ethics?
Steven Escaravage (20:27): Yeah. So we've been going through this for a very long time, and I think that there's accountability and responsibility that's shared from both the developers of the system, and then what we call transition partners or whoever is responsible for deploying and monitoring that solution, but it is a shared responsibility.
Mark Valentine (20:50): Indeed.
Steven Escaravage (20:51): And in some of the areas that we work, in the Defense and National Security space, I think this is an area where again, given how fluid those environments are, and how far from the developer or the original program, these capabilities can be put in place, we have to find a way to couple that accountability, and almost the chain of custody around how these systems are built and implemented, so that we don't lose that as these systems scale.
Mark Valentine (21:23): Got it. That's awesome. Well, Steve, I want to transition a little bit now, and by the way, thanks for the discussion about AI ethics, I find it a fascinating topic, as well as a supremely important topic. But now I want to transition a bit to talk about aligning with the operational environment, and the previous answer you mentioned working with partners, and I know that you at Booz Allen Hamilton are delivering tons of AI projects to our federal government market, and almost all the time you're doing so with partners, to integrate different technologies and deliver those capabilities. So can you share with me and the audience some of the best practices that you've seen and transitioning that innovation from the, "All right, here's the pilot purgatory phase into an actual operational capability?"
Steven Escaravage (22:16): Yeah. Yeah. Great question. Thanks. I think, again, it goes back to understanding the incentives and understanding what is going to result in an actual change. Deploying a capability into a production, that's not success, right? It's adoption and use, and real impact to the mission, and so I think understanding those incentives is really important. It's the biggest gap that I see in research today, where probably every week, somebody sends me a paper that has an incredible accomplishment around some new method or technique largely in the training space, but then I look back to the programs we support, and the users we support today, and it is tough to figure out how that accomplishment is going to add value. And I think the reason is we have to meet the user, the customer in this case, talking about the public sector, our government organizations [inaudible 00:23:17] their expectations, and it's things like latency, power consumption in the environments, and devices that they want to use, it's making sure that they can access the results or the system itself in their environments on those devices.
Steven Escaravage (23:37): I think that really becomes the best practice, just like any other market driven activity is, make sure that we're making the customer happy or the user happy. I think the second thing that I would say that is not unique to the public sector, but worth reinforcing is that, you have to understand the mission, it's difficult to solve a problem if you don't understand the problem and the environment in which that problem manifests, and I think that a lot of the criticism that has been levied on all of industry is that sometimes we're coming with a hammer looking for a nail instead of really working backwards from the problem.
Mark Valentine (24:22): Absolutely. Well Steve, I think you've almost partially answered the next question I want to ask, and getting back to this idea of partnering, so we at TransformX, a lot of folks in our audience represent new entrants to this market, and is there any advice that you can provide them on how to partner with firms like yours, or how to partner with the federal government to achieve the government's goals?
Steven Escaravage (24:49): Yeah, so I think the conversation that we had around... We understand that you have invented incredible capability, and you understand how it's going to move the needle around the value from AI and ML. I think taking that constraints-based approach and understanding that we're not going be able to cut over to idealized environments in the near term, and we have to work to some degree within the constraints of today, and show value as a community, as industry that will lead us to additional investments where we could change the underlying foundation, I think that's one key thing. The second thing which is a trend we see coming out of the innovation corridors, which is good, but I would say to reinforce it is that, in many cases, organizations, vendors, companies are focused on building really the robust solutions, and they have a number of dependencies because of that. They need a certain type of computing infrastructure, they need access to certain open internet data sources, and that might make a lot of sense.
Steven Escaravage (26:10): The programs that we've seen that are most successful though, are working on how to start solving the problem, and then focus on really fast easy updates with new capabilities, versus really robust solutions right out of the gate, and so in some cases less is proving to be more, and I guess the final thing that I'll say is, don't wait to discuss intellectual property, there's a few different camps across the public sector space around, desire to use and adopt commercial off the shelf technology, and then adopt first mentality, which makes a lot of sense, and then there's a legacy around traditional IP rights for governments and data use agreements, and so I think that's something to address upfront, and not wait.
Mark Valentine (27:11): Now, that's great. So that coupled with your advice to focus on actually solving a problem for an end-user, I think is fabulous. So thank you for that. So, Steve, I think we've established that working with the US government in particular, I mean, it's tough, right? It's a large, complex, loosely affiliated confederation of different departments and agencies, all with different missions, many with different authorities, and as such, each one of those entities can vary widely in it's technical, sophistication, and maturity. So based on your experience in working across this space, can you give us a sense of what some of the technical barriers that you've witnessed inside the government that can limit their ability to adopt these technologies?
Steven Escaravage (27:59): Yeah, I'll focus in two areas. I'll focus on the maintainability of solutions, and then I'll just focus on the expertise. Let me do that in reverse order. Some of the experts that I've worked with in the federal agencies, become many of the most successful CTOs and chief engineers and chief scientists, those are some of the most amazing companies coming out of the innovation corridors. There is a ton of talent, but there's never enough.
Mark Valentine (28:33): Right.
Steven Escaravage (28:33): And I think, especially the folks who exist today within those government agencies, they're stretched thin, and so, a technical barrier that inhibits adoption is just the availability to take that capability along the entire journey, and I think the responsibility, if the customer can't do it, or the client can't do it in this case, it falls on industry to make sure that we're providing all of the insights and the justifications, and we're talking about outcomes in the language that makes sense in those environments, but I find a lot of organizations, they want to focus on building a software product for instance, or building a data product, and throw it over the fence, that is most likely going to be insufficient, to build a foundation or adoption for the solution.
Steven Escaravage (29:35): And then the technical barrier, and not to get too deep at the engineering level, but the maintainability of solutions in production environments is I think, fundamentally different in the public sector, at least from my private sector experience where, given the regulated, controlled, secured environments that we operate in, it's difficult to do some very basic things that you need to drive AI~ML solutions. For example, I'm working in a secure computing environment, but my research development environment is in an unclassified, or open internet environment.
Mark Valentine (30:18): Right.
Steven Escaravage (30:19): I might not be able to pass back weight files, I might not be able to identify why I'm having errors in certain classes of models, and so, that just requires a different approach to how we're going to improve and maintain these solutions, and then similarly, the government is working in many cases, because these environments are so controlled, they might not be ready to do updates every two weeks or four weeks or eight weeks, depending on whatever schedule you're on as a commercial vendor. And so then you get this baseline challenge of different baselines for different customers, and I find that that is one of the biggest areas you get a friction where, if we're building momentum, building adoption on a public sector mission, and to get to the next level of capability, there's an upgrade involved to a new version, that can get pretty challenging both on behalf of the government and the companies, especially smaller companies and startups, to have to manage multiple baselines is challenging.
Mark Valentine (31:26): Absolutely. I couldn't agree more with you, Steve. So those are some of the technical barriers, are there any non-technical barriers out there? I keep hearing this idea of culture getting in the way, is that a real thing or any other non-technical barriers you think that exists to this adoption?
Steven Escaravage (31:43): Yeah, there's been a lot of investment in the infrastructure to try to change acquisition, to change the way that innovation can come into the different executive departments and government as a whole, there's been a lot of dialogue over the last few years around creating AI marketplaces, which I think is very exciting, where companies that have capability that's relevant to a public sector mission can announce the supply of those capabilities to meet the demand that might exist, but I do think that for all the efforts and the change in the regulation and policy, and opening up new pathways around other transaction authorities and accelerated procurement, time tends to be the one dimension that is most different, where it just seems to take longer, it is more complex, let's not forget that the US government and the organizations within it are some of the largest organizations in the world, I mean, the Department of Defense is the largest player in the world, right? And so sometimes that type of scale creates challenges when you're trying to acquire enterprise capability.
Mark Valentine (33:09): Absolutely.
Steven Escaravage (33:15): There's reason for optimism around the new procurement methods. I look forward to three to five years from now, I think they'll be a lot more fluid than they are today.
Mark Valentine (33:27): Yeah. I hope you're right, Steve. I really do. So Steve, do you think there's anything... I know in industry we tend to focus or at least for the most part, it seems like we focus on helping the governments solve some of those technical barriers, do you think we have a role to play in helping solve some of those non-technical barriers that you've discussed?
Steven Escaravage (33:46): Yeah, I do. I think that we have to... They are the customer and we need to meet them halfway, or probably further than that. The government leaders will be the first ones to acknowledge that they are working to change, and that there's more work to do, but back to what we've said, there's a lot of inertia that needs to change first, there's budgeting cycle, there's a number of legacy investments, there's technical debt as we've been focused on other things for a long time. And so I think that the industry needs to meet the customer more than halfway, by being flexible to the existing constraints, which we've talked about, and instead of putting the onus on the government or the agencies to figure out how to integrate your solutions, and what are those pathways? But really investing time and money to figure out what exists today and proposing the solutions, so that we can deliver your capabilities in today's environment. I think that's important.
Steven Escaravage (34:53): The second thing, and we all own this, is we have to [inaudible 00:35:00] in the marketing machines and really focus on helping our government understand what is the current state of the art? What is the total cost and timeline to get to outcomes?
Mark Valentine (35:12): Right.
Steven Escaravage (35:13): And what we need from them, in terms of foundational investments to get there.
Mark Valentine (35:18): Well, that's awesome advice, Steve. Thank you so much. Well, Steve, I know we're getting towards the end of our time, and I want to be respectful of your time, and thank you for spending it with us. So I like to get to a final question, and we're talking about operationalizing these concepts and capabilities like Artificial Intelligence and Machine Learning, and we've talked a lot about different incentive structures and things like that, but when I look at what's actually being done in government, it seems like most of the spending right now is still on the research, development, testing and evaluation type of accounts. So if the government is trying to operationalize these technologies, are they aligning with their incentives correctly with focusing on RnD at this point?
Steven Escaravage (36:02): Yeah. Yeah, it's a great question. One of the questions that I've seen come up, similar question is, when are we ready? When is the research and development done? And if you look at the standard model that's used for RDT&E within the government for the six years, it's like, "When is an AI system moving from development into operations? And is it..." And I think that's one of the questions, we focus on two milestones, and I think you have seen some momentum, there is some momentum. If you look at the Pathfinder programs where in the last budget cycles, there is recognition that O&M dollars and procurement dollars are needed, in addition to just pure RDT&E.
Mark Valentine (36:50): Right.
Steven Escaravage (36:51): But I guess the challenge becomes understanding what that milestone is, for me and my portfolio, milestone one is, have we built a system that can operate in a production environment with real world data that can improve over time through just a learning process? And then once we achieve the performance, the outcome, we're meeting those success criteria, then we're ready to move into operational use, and so that second phase is where it requires field service engineers and engineers, AI systems is not like traditional software, requires very different footprint in terms of operations and maintenance as you're continually trying to deal with changing environments and improving performance.
Steven Escaravage (37:44): So I do think we've seen a little bit of change where there is a recognition, and again, if you look at the Joint Artificial Intelligence Center, fairly substantial O&M budget, as they look to scale up their impact across, I do Mark, think that you'll see appreciation, recognition for more of that, and then as industry, we can really help in that area, try to educate on what's needed through our government affairs options.
Mark Valentine (38:13): Absolutely. And hopefully events like this as well. So Steve, I really want to thank you for your time, I don't want to offer you any parting shots that you might have, because I'm sure there are 1000 questions, and important bits of wisdom that you have that you can help the audience with. So I'd like to give you an opportunity now, in case I've forgotten something, or any final thoughts that you might have.
Steven Escaravage (38:34): Yep. Thank you, Mark. Earlier this year we had the final report for the National Security Commission on Artificial Intelligence, and in the report, it talked about the importance of maintaining the link, and expanding the link between the private sector and the intervention of corridors in the country, and the public sector missions, and so I started on the top line that there is progress, there is momentum. I've worked with a large number of companies who are seeing great results from working in the public sector, and I think we all have a responsibility as citizens of our governments to try to get them to better outcomes, and so what I would just ask the audience is, there's real opportunity in the public sector, always happy to have conversations with folks interested in it, but just thank you for the focus on that, and it's going to need more effort from all of us as innovation comes from the outside.
Mark Valentine (39:37): Indeed. Well, Steve, thank you again for your time, and your wisdom in this session, and I also want to thank the audience for your time, for your attention. I hope you've enjoyed this session, and I hope you've enjoyed TransformX at large. I know it's been an awesome experience for me, and I hope we all get a chance to meet in person soon. Thanks, Steve.