Panel: Transforming FinTech with AI
speakers
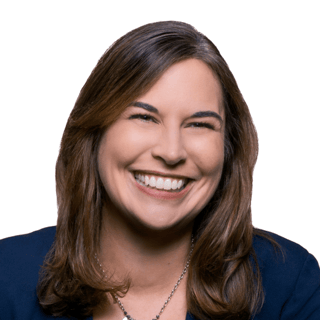
As Redfin’s Chief Technology Officer, Bridget leads the software engineering team. Her mission is to build technology that makes the process of buying and selling a home less complicated and less stressful. She is a leader on issues facing traditionally underrepresented people in technology, and 36% of Redfin’s technology team are women while 10% are Black or Latinx. Prior to Redfin, Frey was the director of analytics and business applications at Lithium Technologies. In addition, she has held management positions at IntrinsiQ Research, IMlogic and Plumtree Software. Since 2019, she has served on the board of directors for Premera Blue Cross. Bridget holds a bachelor's degree in computer science from Harvard University, where she graduated.
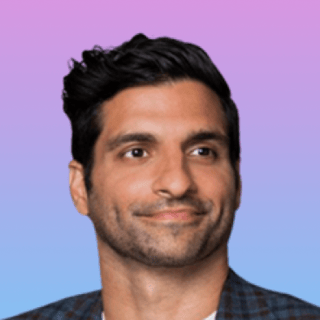
Nima Ghamsari is CEO and co-founder of Blend, a Silicon Valley technology company propelling consumer lending into the digital age. He leads the company's corporate and product strategy toward the vision of a more frictionless, compliant, and accessible consumer finance ecosystem. Nima is currently focused on expanding the Blend platform both in breadth and depth, bringing seamless processes to more consumers across more of their financial lives. Prior to founding Blend, Nima was one of the first employees of the commercial group at Palantir Technologies. He is a graduate of Stanford University where he earned a B.S. in computer science.
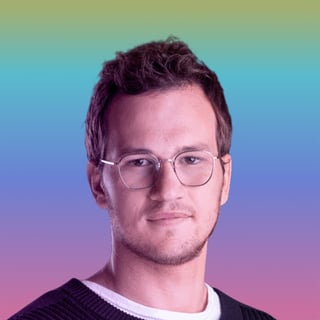
Henrique Dubugras is Co-Founder & Co-CEO of Brex — a corporate card and spend management solution that drives 100% compliance with 0 receipt chasing in 100+ countries. A Brazilian entrepreneur, Henrique built payments companyPagar.me — the Stripe of Brazil — when he was sixteen years old, with his co-founder Pedro Franceschi. In just three years, Pagar.me grew to $1.5 billion in volume of transactions processed. In the fall of 2016, Henrique and Pedro sold Pagar.me and enrolled at Stanford University. Henrique and Pedro founded Brex in 2017 to help startups more easily get access to corporate cards by rebuilding the financial stack and infrastructure behind them. Since then, Brex has evolved to help startups and scaled companies manage every aspect of spending, from the smallest purchases or the biggest procurements, no matter where employees live and work.
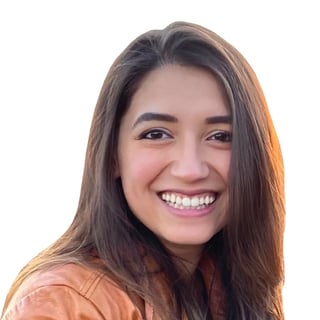
Melisa leads the Document Processing business unit at Scale that focuses on building fine-tuned ML models with human-in-the-loop annotation to automate handling of documents at a high quality. Previously, she was the Chief of Staff for the company and started the Government business unit. Before Scale, Melisa used to build products for new product monetization at Facebook. She graduated from Stanford University with a degree in Computer Science. Melisa is from a small town in Turkey.
SUMMARY
The panel discusses how advancements in AI technologies like document understanding are increasing efficiencies, lowering costs, and disrupting traditional processes in financial services.
TRANSCRIPT
Melisa Tokmak: Welcome everyone. It’s been a busy day today with a slate of amazing speakers since this morning. I’m not sure if you have been listening since 8:00 AM, but it’s been pretty awesome. I hope everyone enjoyed these sessions ranging from turning big data to good data, modernizing global trade, to making autonomous vehicles a reality. We have another exciting session here about how AI is transforming FinTech, an industry that’s deeply intertwined with our daily lives.
Melisa Tokmak: I’m Melisa Tokmak, Head Of Document at Scale. I have with me the leaders from pioneering companies in FinTech, Henrique Dubugras, Co-founder and Co-CEO of Brex, Bridget Frey, Chief Technology Officer of Redfin, and Nima Ghamsari, CEO and Co-founder of Blend. So FinTech covers a lot of ground. We’re not going to cover all of it today, but quite a bit with these speakers. Let’s dive in and start with a question that explains where your companies play in FinTech for the audience to see. Welcome all of you. Tell us a little bit about your companies and what makes your company unique. Nima, let’s kick it off with you.
Nima Ghamsari: Thanks, Melisa. Hello everyone. Blend is a software company. We’re a software platform used by about 300 banks, and credit unions, and lenders across the country. Our product is used to deliver seamless, frictionless end-to-end customer origination journeys of those products. So everything from mortgages, to auto loans, personal loans. The way we met Scale was through a lot of customers, consumers still provide income documentation, things like that via documents, via pay stubs, and being able to use AI in real-time to extract that information and process it is something that’s a real value to the whole industry.
Melisa Tokmak: Yeah. What about you, Henrique?
Henrique Dubugras: I’m the Co-founder, Co-CEO Brex. At Brex, we do corporate credit cards and corporate cash management for the next generation of businesses. We started a company in 2017 and we primarily focus on technology companies and e-commerce companies.
Melisa Tokmak: Yep.
Bridget Frey: I’m Bridget. I’m the CTO at Redfin. We are a real-estate brokerage that was founded by software developers. We use technology to make everything about buying or selling a house better and more efficient. We have actually saved our customers more than a billion dollars in fees over the years. So plenty of opportunity to use data and AI to make real estate better for everyone.
Melisa Tokmak: Yeah. That’s actually super interesting. From everything you have said, some of the words that stood out, the seamless experience, making it super easy for the user, bringing efficiency to them. We’ll talk a lot about that user experience and the whole journey and how you’re using AI for that. But just because it’s top of mind a little bit, obviously the pandemic has been in our minds pretty much for everyone, especially for FinTech. And because of the thing you have been mentioning, it’s the world is at a stand still.
Melisa Tokmak: People are turning to these online solutions, people like you who are making everything a lot easier. The pandemic left a lasting impact on consumers and businesses alike. For example, people are buying houses in new cities. Just before joining for this live session, we talked about LA and Miami a little bit. Online transactions are taking off as people purchase more online. Also, the current capital market environment is creating a new appetite for investing in startups.
Melisa Tokmak: So there’s some real interesting implications on the impact of COVID across both real estate for Redfin and Blend, and spending spending for Brex. What have been the biggest changes your companies have faced over the past year and how have you adapted to them? Bridget, you’re very relevant to this. You shared a lot about COVID-19 and its implications over the past year. So let’s start with you.
Bridget Frey: Yeah. I mean, the pandemic really made our year a wild ride. It seemed like at the beginning of the pandemic, everything just froze up and no one was looking to buy houses. But within a couple of weeks, it changed. People were more interested than ever and moving to new areas. Since the beginning of the pandemic, we’ve seen the number of our customers that are looking to move to places like Bend, or Boise, or Charleston has more than doubled that interest in smaller cities.
Bridget Frey: And so, we had to take a look at our business and say, people want to move, but they have to do that more virtually. We went from having 1% of our tours happen virtually, so over a chat or something like that, to a third just a few months into the pandemic. We just saw a sea shift and a sea change in people wanting to do things virtually.
Melisa Tokmak: Oh, well, Nima, what do you see in Blend?
Nima Ghamsari: Well, we’re definitely seeing what Redfin is seeing, but we’re also seeing that, not just for the home buying process, which is shifting and seeing we’re geographically disperse, but all transactions, all banking and financial services transactions seem to be moving more towards or accelerating towards digital. I believe that before the pandemic, people, banks, credit unions, lenders all understood the value of digital, but what we’re seeing now is all those things are being moved way up in priority. They’re being condensed into really tight timelines.
Nima Ghamsari: Making a digital transformation, the ability for us as a platform to make that possible for them to do it faster is a big priority for us. So how do we accelerate digital transformation? We think about digital agility, which is what we call it. I’m assuming it’s very important to us. That means that we’ve had to make a bunch of platform investments that almost roll out new products in days or weeks instead of months or quarters.
Henrique Dubugras: For us at Brex, it was quite interesting because our Brex Cash products, one of the things is we’re trying to replace people’s banks with Brex Cash. One of the pushbacks we got from a side of customers is like, “Hey, what about my branches? I may want to go to the branch.” After the pandemic, we saw the complete opposite effect, which is like, “Can I do stuff without going to a branch?” which I thought was quite interesting.
Henrique Dubugras: The other thing that was quite interesting was a lot of our credit modeling is pretty AI driven statistical. But we had to, I think, open the black box a little bit during the pandemic because we had to take into account everything that was happening in the world in order to make our decisions. We couldn’t just let models automate a lot of the decisioning like we had in the past.
Henrique Dubugras: I think having both the good judgments of humans plus the model is what got us to be in a great place and have ourselves and our customers do super well through the pandemic.
Melisa Tokmak: That’s super interesting because… What the pandemic really forced us, if I’m summarizing right, some of you really invested a lot in the infrastructure, being able to bring digital transformation. And with you, Henrique, thinking about not only models, but at the same time, the human judgment together to solve some of the problems. Also being able to provide a flock of people, trying to move to other places, with you, Bridget, and looking for those options, how to move there and have a great life in the places they move, it’s really interesting.
Melisa Tokmak: It all comes to this application of AI in these different areas. So I want to go a little bit deeper there. This is not something that started with the pandemic, actually. FinTech has been really a booming space for the past couple of years. We have seen an explosion of new companies trying to reimagine the core elements of the financial services industry with technology. So it could be payment infrastructure, loan and mortgage origination, insurance, corporate banking, consumer banking, are just some of the areas that we see that have been exploding for the past five years.
Melisa Tokmak: Our audience today is heavily focused on AI, and you have done a great job on talking about how AI came into place. But digging deeper, what do you think are areas right now where technologies like AI are transforming financial services, if you can give details in depth? And more specifically, where does that tech part come into the picture, and how is it meaningfully changing the way things are done?
Henrique Dubugras: I can start. For us, making banking and credit cards and all that we do basically instant. I think traditionally, the experience is you go to a bank and you provide them a ton of documents and everything takes forever. They need to go and process those documents and they need to go and do fraud controls. All these things are done only by humans and batch processing and all these things. I think we need to move into a world in which all of it is instant.
Henrique Dubugras: You can go to Brex, sign up, and instantly get an account, instantly get a car, instantly get credit approved. I think that’s only possible with AI because it’s basically what allows you to have better judgment than just a traditional rules-based system, to actually make the right decisions. This is an allowable for just customer experience. If you look at a lot of the developments in FinTech, I think it has been FinTechs pushing the boundaries of what is not instant versus what could be instant as well. So I think that’s quite interesting.
Bridget Frey: Yeah. We’re really seeing a lot of innovation right now in this idea of how do you price a home? That’s something that was done by humans over the years. But now there’s a lot of technology entering that space. When you look at housing, it’s one of the largest asset classes in the United States. If you could figure out what a home is worth better than anyone else, there are all kinds of products and services that you can build.
Bridget Frey: At Redfin, we’ve really invested in this. We have the most online home value estimate, twice likely to be within 3% of the final list price as any of our competitors. And so, then we started looking at that and saying, “Okay, well, what can we do with that? What businesses can we build?” We built a business where we’re essentially an iBuyer. We can buy a home directly from a seller, fix it up, and put it back on the market. And it’s really only through pricing algorithms that you’re able to be competitive in those types of businesses.
Nima Ghamsari: Yeah. Building off that, I was thinking about what Henrique was saying, is everything’s moving to real-time or closer to it. Or the companies that win the customers are the ones that are able to serve the customers in real time. There’s a whole bunch of studies on this and analysis on this, where the ability to do things in real time is so much higher throughput for consumers. But the problem with financial services is that historically the decisions were very document heavy, like Henrique said.
Nima Ghamsari: And so, one thing that we talk about at Blend is this concept of data over documents. How do we get data? How do we then evaluate that data and in real time get the customer back, that commitment that we can do something for them, or our customers, the lenders and banks can do something for them? Which is amazing because there’s more consumer data available now than ever. There’s Plaid, there’s Finicity, there’s Equifax, there’s all these different data providers, which is amazing, but it’s only got some coverage.
Nima Ghamsari: What happens is, in the other areas where there’s not enough coverage, consumers still have to provide things in document format, and that’s where the real time breaks down. And so, where blend is looking to continue to add coverage, essentially, of real-time consumer financial data, is by using AI to when people do have to provide a document, be able to instantly get back to them and say, “Yeah, you’re good for this loan,” or, “You’re good for this new account,” or, “We think you’re a low fraud risk,” or whatever it is.
Nima Ghamsari: That’s a really hard problem. It hasn’t really been solved at scale before. Not Scale the company, but at scale before. But it hasn’t been solved. And so, solving that will be massively, massively impactful to millions and millions of consumers and something I’m very excited about.
Melisa Tokmak: No, I’m glad you brought that up. I mean, obviously, you know that it’s something dear to our hearts here at Scale. We’re exactly working to solve that problem. I like that you’re bringing that up, like real time data. How do you turn the problem from documents to data and do it instantaneously? And there’s always that trade-off, something that our team here deeply cares about. How do you think about that trade-off between real time and quality and push them to the edge?
Melisa Tokmak: Because especially in financial services, you do not want to be dropping quality while you’re becoming real time. They both have to go up, and it is a very difficult problem. It is a very difficult problem. It’s a real machine learning problem. It’s not only with a lot of data, like getting solved with a lot of data by training these algorithms. It’s also how you build these models and train them with data that becomes top of mind.
Melisa Tokmak: I like how the conversation is going, and I’m not sure if you guys listened to Andrew in session in the morning. But it was a lot about transitioning from big data to good data. You have already talked about this in the sense that… With your documents, Henrique, like it might be the receipts, every type of thing that you’re facing in terms of data, and Bridget, your pricing algorithms, the data that goes into it.
Melisa Tokmak: So we have seen this huge unlock in financial data about consumers and businesses, and ultimately really improving the customer experience in areas ranging from better underwriting, speeding up manual workflows, and recommending new services. But there is a difference between having this lots of data, whether it’s transaction reports or the documents people are bringing it to you and handing it to you, Nima, because they couldn’t find the digital versions, or you have to go get the title from an office, or consumer preferences, or any other types of documents, versus actually making use of it.
Melisa Tokmak: So what kinds of data do your companies typically deal with for these? Please go in depth a little, and how are you leveraging AI to get more value, unlock more value from those to improve your products? Exactly what Nima was saying, turn docs to data. Right.
Nima Ghamsari: Well, I’ll start. I think offering a loan to a customer, one thing that we want to make sure that our customers are able to do, our customers, the banks and lenders are able to do, is they want to be able to make sure the consumer’s able to pay back that loan, and the small business is able to pay back that loan. One of the considerations there is income. This is just one example.
Nima Ghamsari: But income verification is a surprisingly hard problem because, one, consumers, most of their income data is locked into payroll providers. And not all payroll providers make their data available digitally. And so, people provide pay stubs, and pay stubs come in many different formats. The fields are named differently on the actual physical pay stub. Ends up being a very dirty problem.
Nima Ghamsari: Some pay stubs, like early days, Blend, our pay stubs were handwritten. Some problems are unsolvable. Not everything has to be solved on day one is nothing I would say. Pay stubs are one example. But then small businesses have a different kind of way they track their income. People who have a lot of investment income have a different way that they track their income or we collect their income information. That comes with a different type of form.
Nima Ghamsari: And so, all of those kinds of things, coming up with a unified income solution that can help the consumer, A, get it digitally if they can. But then waterfall down to a more manual approach is something that we built into Blend or been building into Blend, which is with the concept of data first if we can get the data digitally. If not, get the data via documents, then try to process it. If we can’t process it, then we’ll have somebody manually review it.
Nima Ghamsari: That’s a pretty important thing for the industry because, frankly, so much time is spent manually underwriting and reviewing how much income somebody has on their forms, and then verifying it and checking that the number you calculated over was right or tracked over was right. And that’s a problem that eventually will be solved, and we’re helping drive some of the solutions forward.
Melisa Tokmak: Actually before like maybe Henrique, you’re going to go next, but before, I want to double click onto that. At the same time, you are dependent on other organizations as well when solving this problem, whether it’s in compliance and other… And it’s difficult. So I’m curious, are you seeing any challenges as well with that side of the market, while you’re pushing for that digital transformation and efficiency?
Nima Ghamsari: Well, not necessarily challenges in the sense of I think people use data already. So it’s not about, can we use data in a lot of cases? It’s, one, do we trust the data, that it’s accurate? Some of the data providers that we use, for example, we do our own QA before we hand it over. Spot checking of some subset of loans are QC, I should say, where we’re allowed. And then, they still do manual review to ensure, in a lot of cases, some subset of the loans as well.
Nima Ghamsari: And so, it’s not like it’s an overnight thing where people just suddenly trust the data. But they do want to use data. There’s not an unwillingness to use data. I think there’s just more of a, “Hey, this is new. Let’s make sure we’re not doing the wrong thing here, making mistakes here, as much as there’s a compliance issue.”
Melisa Tokmak: I mean, it’s good to hear because we see hopefully the whole industry is moving there and everyone’s getting on the train. But as you said, how do you make sure it’s trusted data? What do you think, Henrique?
Henrique Dubugras: Yeah. I’ll give two examples. The first one is around… We call it in Brex categorization and vendorization. I don’t know if vendorization is a word that exists, but it’s what I call it.
Melisa Tokmak: Now it is.
Henrique Dubugras: Now it is. Which is when you look at your credit card statement, if you just look at it, it’s a bunch of weird gibberish stuff. It’s really hard to get reporting, very, very simple reporting of like how much did I spend on Uber this month? And how much should I spend on Uber Eats this month? Because they’re two different things, and you want to look at it separately. We do a lot of work to basically re-categorize and re-vendorize every single transaction that comes to a customer because doing that infrastructure layer of it enables a lot of things later.
Henrique Dubugras: Reporting is one example. We can report to you where you spend your money, in which categories or which vendors. But it also helps around control. So if you want to make a card that only works for that vendor, for example, it’s only possible if we actually have that concept, and we did a lot of work to make sure that that’s clean and safe and secure. I think that’s one of the things where I would say cleaning up the data that you get is extremely important because otherwise it doesn’t work.
Henrique Dubugras: Another example is then we can use AI for fraud controls, because we know exactly what this vendor you’re trying to spend on is, versus not knowing. So it makes fraud controls much easier because we clean up the data. I think that’s one example that matters a lot. The second thing is, how do we get context out of things that didn’t exist before in our industry?
Henrique Dubugras: For example, we have all these people submitting receipts for Brex. Those receipts, they have a lot of interesting information. What did you buy? When did you buy it? And things like that, that we don’t get just with transaction data. So processing those receipts and actually getting the real data and what’s the real context behind that so we can make it available to customers, and even make inferences and decisions based on it is super important.
Henrique Dubugras: I think that one thing that was really interesting about working with Scale has been this part of, hey, there is the model, which is obviously quite good. But it also gets better with humans over time. Because like in our industry, if the customer can’t trust what you’re telling them, they’re just going to completely ignore whatever you do and they’re going to review it manually. If they can’t trust 100% that you do a good job, so if you just automate 100% of it, like what Nima was saying, then you just lose trust because you’re never going to get 100%. Some stuff was handwritten.
Henrique Dubugras: But then if you do everything manually, then it’s super expensive. So this mix and getting better over time is probably the right way. And obviously, Scale, has been super helpful with that to us.
Melisa Tokmak: Thank you.
Bridget Frey: At Redfin, we have a really unique dataset to work with. We have the number one real-estate brokerage website. We’ve got more than a billion sessions a year where people are coming and looking at houses and giving us all kinds of data signals as part of their visit to the site. But since we are a national brokerage as well, we also know, are people touring those homes? Are they making offers? Are they winning those offers? And so, you can imagine there’s all kinds of machine learning that we can do across all of this.
Bridget Frey: But I think in the early days when I started at Redfin 10 years ago, I thought, “Oh yeah, we’re going to take all this data and we’re going to crunch it and we’re going to spit out the answer and that’s going to be great.” And that’s what our customers want. But we also have real-estate agents who work for our company and they have to go into someone’s living room and say, “Here’s what I think your home is worth,” and if you just spit out a number, the listing agent isn’t going to use your data because there’s no way that they can interrogate it or understand how it arrived at that conclusion.
Bridget Frey: Also, some of the things Henrique was talking about, that you need to leave some space for human beings to tell you whether your algorithms are working or not. So we really designed a lot of our algorithms to show their work, to show their homework along the way, and have opportunities for humans like agents and customers to give us feedback on that so that we can constantly make them better and look for opportunities to improve the quality.
Melisa Tokmak: Let’s double click on that, Bridget. For you, it’s in general the algorithm build, because you’re dealing with pricing and various other workflows, and I think we should hear more about that. For Nima’s and Henrique’s side, from the examples they have given, it focused more on the document processing or automating the manual workflows side. Both are very interesting. And it came up, like you want to apply AI to make it a lot more accelerated and efficient, while all the while keeping the QA element as well.
Melisa Tokmak: This is what Henrique was mentioning, now modeling is not something static, it’s dynamic. You have to continuously improve the outcomes with more training and tweaking, by making sure you’re covering those edge cases and you have enough representative data and you know how is your model structure, to make any changes when necessary? I want to dive deeper in this, and especially, Bridget, with you. You’re leading all of the engineering organization on the Redfin side.
Melisa Tokmak: What does that workflow look like when you talk about algorithms for you, or how does it work at incorporating these elements that we spoke about?
Bridget Frey: Yeah. There are many places where we’ve inserted these algorithms into an agent’s workflow, and those are probably the most interesting cases to work to talk about. As with Nima and Henrique, we do have quite a lot of documents and workflow and processing that are happening. So we have a document intelligence team, and they’re essentially helping to reduce the amount of time that one of our coordinators has to spend on getting gigantic PDF documents and splitting them and merging them back together, and changing data in them.
Bridget Frey: It’s really quite a lot of work. And so, we’ve worked on algorithms that can extract meaning from those documents and help save them time. But in developing the algorithms, you really have to crawl, walk, run. First, we would show the coordinator who was working on these documents, “Here’s what the algorithm thinks is happening. Do you want to accept that you should split or merge these documents in a certain way?” And they could say yes or no.
Bridget Frey: And then that feeds back into the learning and the way the model is built over time, so you can get better. Then eventually you get to a point where there’s enough trust that you can really roll it out. But I think it’s that idea of thinking about phased deployment. I think as an engineer, again, you really want to get the answer. But most of that interesting work and machine learning is about developing the algorithm, figuring out how it works. It’s really not about this single answer.
Melisa Tokmak: Definitely. One thing Andrew Ng said this morning really stuck with me, I really enjoyed it, which is talking about this. When you deploy a model, that doesn’t mean it’s the end. It’s about 50% done. I really enjoyed it. Like how he said, it’s halfway done, you have to go back to it again and again. I see Henrique and Nima smiling here, so they’ll tell us a little bit more about their workflows and how they really incorporate AI into these systems in their own companies.
Henrique Dubugras: I can go first. I was smiling because we go through so many of the same situations, and we think that we deploy the model and then it’s all going to work. And then, there’s so much more work after that. I think that the way we try to do it, though, is we try to make it like the first version of it, just be super simple so we can start getting data, and just making it as simple as possible.
Henrique Dubugras: I think a lot of times we try to over-complicate things and then it never works and we have to go back. So I think getting something out there launching, getting some data, getting some traction, I think helps iterate on the development of everything. I think that’s super important within our company and our processes to keep doing that. Then the second thing is to understand which problems require 100% accuracy and which problems is 90% fine. You know?
Melisa Tokmak: Yeah.
Henrique Dubugras: I think clearly identifying those, because they require different solutions and they require different amounts of time that you invest. It’s just completely different. I’ll get an example, it’s like fraud. It’s impossible to get 100% right on fraud. You’re going to flag some stuff that’s not fraud. You’re going to not flag some stuff that’s fraud, something you just want to continuously improve and get better over time.
Henrique Dubugras: But to give another example, like bank statement processing, when you get a bank statement, there’s no room for getting a zero wrong there and giving the customer 10 times more, 10 times less credit. That’s a big problem and a big no-no. I think some things that require 100% accuracy, you need to just run differently. I think that you’re just going to have to improve over time.
Melisa Tokmak: Yeah. I actually really like what you said, Henrique, because many times we work with a lot of different companies, yourself included. It really becomes a really good outcome for both companies when we work too, when we can talk about that. Let’s understand the problem. In this problem, what’s important? What do you really need? As you said, in some of them, you cannot really let go of any accuracy. You have to be close to 100%.
Melisa Tokmak: In some others, for example, some of the products that you’re building, to be super fast and instantaneous, so important because you care about customer experience. But it really depends on the problem, and where does that system live in your product? And being able to talk about this with the companies or internal teams, or it might be either, that you’re working with to solve the actual problem and not try to solve everything at once. But-
Henrique Dubugras: Yeah. Maybe I’m overgeneralizing, but I think a lot of problems in financial services, you want the instantaneous for 80%, and then the other accuracy for the 20%, that is harder. I think that that’s the nature of most problems in financial services, if that makes sense.
Melisa Tokmak: Yeah. Especially now, people have… I mean, thanks to all of you, a lot of it now expectations for better and faster. They’re not going to settle down for anything less than that.
Henrique Dubugras: Yeah. Yeah. It’s just rare the situation in financial services where you can give a wrong answer, but there’s some situations in which you can ask for another 24 hours to look at it for 10 or 20% of the cases.
Melisa Tokmak: Nima, tell us a little bit about how your workflows work in this case.
Nima Ghamsari: Yeah. I think one thing that happens a lot, and it’s a common thing with our customers, is they’ll initially want to solve for everything, including all the edge cases. Henrique said 80% being automated and 20% having a manual fallback is good. I would almost even say, when we start, we just push them to say, ""Look, don’t even worry about getting to 80%, get started with 20%. Make 20% of your customers’ lives better. And then we’ll gradually increase from there.
Nima Ghamsari: One, the value can be incrementally added as we learn more about the problem space, as we learn more about the dataset, as we learn more about the customer set. But two, creating that incremental value is meaningful value to those 20%. So why hold them back while you wait to figure out the rest of this problem domain? It’s unnecessary waste in the system. It’s a natural problem in finance… Because they’re used to growing out software in this huge waterfall methodology where everything takes two years.
Nima Ghamsari: And so, the next project’s not for another two years. And you’re like, ""Well, if you only solve 20%, then it’s going to be 2024 before we solve 40%. That’s not how modern software… We do releases almost every day at Blend to our customers, and it’s one code base and all that stuff. And so, that allows them to continually improve and get towards that end goal faster than they would otherwise.
Nima Ghamsari: It’s just a mindset shift that we’ve had to help work with our partners, the 300 or so banks and lenders across the country, to do, is just think about solving part of the problem, expanding, as opposed to trying to solve for everything on day one. The reality is the technology to solve 100% might take a hundred times as long as the technology to solve 80%. And so, it’s not linear either. And so, that’s something that we tell our customers, like get started and keep innovating and everything will take care of itself over time. They’re all on board with that. It’s just a mindset shift, and something that we’ve been helping push within the industry.
Melisa Tokmak: That actually trade-off is also relevant to what you solve versus what you outsource, because you guys gave a little bit different answers. So I find that very interesting. Bridget talked about creating more teams internally as well to really attack this problem. I think Nima and Henrique, you talked a little bit about working with different companies in the space as well, Scale included.
Melisa Tokmak: Right before this, actually, there was a session on modernizing global trade where we hosted Flexport’s CTO, James. He talked about the same thing, that they care about being that domain knowledge, like in this case, trade. That’s their core competency. And everything else, especially involving AI, that they are looking for the experts in that area, in the infrastructure area. How do you really weigh those options internally as the leaders of your company?
Melisa Tokmak: I think many companies are looking at this trade-off and thinking about it. Once we have different opinions, I want to hear more, one works with the other.
Bridget Frey: Yeah. I mean, I can jump in on that. I think at Redfin… This may be a little bit one of the differences between Redfin and the other businesses here today, at Brex and Blend. But we’re basically trying to give a real-estate agent more time to spend with their customer. We’re taking something that’s a very human-intensive business, real estate, where you want someone on your side who’s walking through every part of the transaction with you. And we’re saying, “What can we do as engineers to offload pieces of that so that the agent can spend more time on the human side with that customer and deepen that relationship?”
Bridget Frey: And so, I think that what we’re primarily doing is taking things that humans do and moving them to machines, which machines can do a lot of things better. But because of that, we’ve had to have our engineers work very closely with real estate agents. And in fact, we hire engineers who have that empathy and want to work side by side with real estate agents who feel like there’s a lot to learn on both sides about that, because we found that unless we do that, we don’t attack the problem in the right way. We attack it in this very engineering machine-led way and we miss the parts of that human interaction that we’re trying to automate.
Bridget Frey: So that’s been really important in our business, and I think it’s why we have built a lot of our AI and machine learning in-house.
Henrique Dubugras: I think, for us, it depends a little bit on the problem set. I’ll give an example. There’s some problem sets that are very unique to our business and they’re very specific. Credit, how do we use AI to give credit? I think that’s something that has to… It’s the entire organization. It’s not only a team in data that does it. It’s interacting with our capital markets team. It’s interacting with the product team, the marketing team.
Henrique Dubugras: There’s a lot of things there that’s a little bit of a core competency. We do work on those in-house. But there’s a lot of other problems that are very important to our customers, but it’s not a core technology that we need to invest our own resources in, that we’re very happy to use someone like Scale to help us solve.
Henrique Dubugras: The receipt processing is an example that… It’s just like, great, we could be best at receipt processing, and that’s going to be awesome, but it’s probably better that we focus a lot of our resources trying to be your best at the credit side or the fraud side, etc. That’s the part that’s quite unique because, when we look at other vendors, you either have the outsourcing firms that are just doing manual stuff, or you have the APIs for OCR that’s just like doing some ML model. It’s very hard that we can find a vendor that can do both with us and can help with both the things.
Henrique Dubugras: Especially in financial services, as I was saying, you do need both. So having both, being highly integrated, I think it’s something that has been very hard to find. But I was very happy that we work with you guys.
Melisa Tokmak: That’s great. Nima, anything from you? Do you make decisions differently or…
Nima Ghamsari: Well, I would say, I am so focused on speed that if a platform… Of speed of innovation, I guess, or speed of whatever we’re able to deliver to the market, because I think speed is the thing that ultimately allows us to create the most value. If something exists out there and it’s something we can buy, but also can deliver that amazing experience, that our customers want to be able to deliver to their customers. That’s the key thing.
Nima Ghamsari: I think most things, unfortunately, there’s a lot of… Because there’s some really good tech companies that we can partner with, but there are a lot of spaces that are still pretty green. And so, we’re not always sure that we can bet on those things. Until we can prove that we can deliver that experience, it’s a little bit tough to put all our eggs in a basket on something.
Nima Ghamsari: And so, as long as we can deliver that experience, we will always outsource it because then we have so many… Our roadmap, the amount of things that have to change in the banking and lending ecosystem before it’s a truly digital, one-tap, proactive, personalized experience, it’s on the order of hundreds of different products that have to be developed and created and innovated on over time.
Nima Ghamsari: There’s a lot to do, and I would say, almost always, we aim to not do things in-house if we can. But we end up building more things than I’d like to, partially because I think some of the spaces are pretty nascent and there hasn’t been heavy investment in them. But we do find good vendors, good partners. We get really excited.
Melisa Tokmak: Yeah. I think definitely you’re saying speed, obviously. So if there’s something fast, go after it. But at the same time, prove the value a little bit. You’re not going to put everything in at once, but you want to see value quickly and then expand from there. I think it’s definitely interesting. I think, across the board, I have been picking this up from some of the examples you guys talked about as well, whether with Henrique’s credit. And obviously, on the mortgage side, or with Bridget, pricing.
Melisa Tokmak: We talked a lot about risk mitigation or where to risk, where not to take risk. I’m very interested in that because it’s an AI problem. It gets better, risk mitigation gets better, with having more data, and more importantly having better understanding of your data. So tell us a little bit more about where it’s important in your businesses to understand risk, but you already gave some examples, especially how would you assess the risk in your businesses?
Bridget Frey: I guess, for us, the closer that it is to the actual transaction where you’re actually affecting the customer transferring dollars, that’s where you have to be very serious about how you’re detecting fraud or making sure that those calculations are really correct. Often, there is where you end up in the end you are sometimes pairing a machine with a human to make sure that that’s really all squared away.
Bridget Frey: I think as you go earlier, though, when you’re recommending homes or really anything where you’re showing like, “Hey, this is what the machine thinks, but the human can still override it,” there you have a little more wiggle room and all of those things. We also have a mortgage business, a loan origination system that the algorithms and the code that we’re doing there, it really has to be, as with Nima at Blend, I’m sure, that’s where we really have to make sure that we’re not making mistakes because the cost of a mistake is really high.
Henrique Dubugras: Yeah. I would say that, for us, there’s two types of risks. One is, I would say, economic risk, and the other one is compliance risk. It’s two different approaches for each of them. Things that are like compliance risk, for example, making sure that we don’t onboard anyone associated with a sanctioned country or something like that, or send money to like sanctioned countries like… That’s zero tolerance risk, where you cannot have any of that happen. And so, we treat it that way.
Henrique Dubugras: Then there’s transaction risk. I think the important thing there is being a little bit more profit-driven than just risk-driven. What I mean by that is if you’re… I’ll give you an example in e-commerce that I think is easier to grasp. Let’s say you have a hundred e-commerce transactions, that people are trying to buy a hundred products. What you’re trying to maximize is the profit for the customer, even if there’s one or two or three fraudulent charges that are more there.
Henrique Dubugras: But it’s better to approve 95 of them, and then maybe you have five of them that are fraud, than approve only 70 of them and have three of them that are fraud. So it’s important to take into account like, what is the cost of blocking something? In our case, what is the cost of someone going to a restaurant and us thinking that it’s a fraud and their card unblocking that transaction. It’s a really high cost. It really sucks. Well, you need to take that into consideration and look at it more from a risk return perspective than just from a purely risk perspective of trying to block fraud as much as you can.
Melisa Tokmak: How do you make that decision with AI versus… I think audiences have been also curious about these rule-based systems versus actually applying AI. It’s definitely interesting to make that decision and it has to be the right decision. How do you do that?
Henrique Dubugras: I think the important thing is just setting the right metric for the teams. If you set the metric that you set is just a fraud metric, they’re going to do everything in their power to just reduce that. Then you’re going to end up with a bad experience because you’re focused on fraud. You need to set a metric that balances out. That’s why I call it profit, or maybe some metric that involves both the things that you’re blocking, but also the things you’re accepting. Maintaining balance between the indicators is super important. So I think that’s super important.
Henrique Dubugras: In terms of AI versus rule-based, depends on the problem, honestly. If it’s something that has a lot of external inputs… I’ll give an example. We do a lot more AI and fraud for transaction fraud than we do for credit. For fraud, because the cost of a fraud is much lower than the cost of bad credit… If we give someone a hundred thousand dollar limit and they default on us, that’s a pretty expensive mistake for an AI to make, to learn. So we definitely want to have someone there making sure that those things don’t happen, having some sort of intuition.
Henrique Dubugras: Versus in fraud, if there’s a $50 transaction that’s fraud, we need to charge back and the customer gets their money back. It’s all good, so we can have the AI and get better at the model, etc.
Melisa Tokmak: Anything from you, Nima?
Nima Ghamsari: No, I think they pretty much covered it.
Melisa Tokmak: Yep.
Nima Ghamsari: Our customers have tons of people who are willing to look at documents when needed. So we play a lot of this on the safe side when we’re not sure. If we’re sure about something, like we’re pulling directly from a payroll provider, then we’ll just populate that data directly. And if we’re not sure, we’ll always lean towards having their ops teams evaluated, just because it is so important to underwrite these loans appropriately.
Nima Ghamsari: The one thing I’d add about fraud or AI versus rules, like the pay stub example, going back to that one, if there’s 400 different permutations of pay stubs and they all use different types of language around gross pay, and what that field is called. It’s just building a rules-based model for that. It’s not super tenable to build that and maintain that. And so, that’s one example where it might make more sense to have AI manage it, versus maybe something like a W2, which is a standardized government form, as it’s a little different of an approach that you might not want to take there.
Nima Ghamsari: And so, we’re definitely open-minded to the right approach for the right problem. We’re not really just about any of those things.
Melisa Tokmak: Definitely, especially when there are just so many permutations to look. How do you build the right robust models that learn, and that also won’t be a high barrier for your entry that you have to set up something for each permutation. It really is about really balancing that. We’re coming up on time, but I do want to end with hearing from all of you on how you think about using data as a moat for your companies. You talked a lot about data, clearly. You’re really leveraging it in your businesses. So let’s hear that a little bit. Bridget, let’s start with you.
Bridget Frey: Yeah. I mean, I think we’re just very inquisitive about data because every piece of data that we get can help our customers find their dream home and really get into the right home. And so, if we can build algorithms in data that are more open and transparent, if we can hire a diverse engineering team that helps to make sure those algorithms are fair and the data that they’re presenting to the world, and then we can get that into the hands of our customers, that’s a huge competitive weapon because the more we can help people get into the right house, the more they’re likely to work with Redfin.
Henrique Dubugras: Yeah. I think for us, the way we think about it is we want the data to work for our customers. Today in financial services, most software providers or banks, they’re trying to say, “Hey, don’t worry about your data. Let us handle everything.” I think the way we approach is, how do you learn from your data? I don’t want to forget about what I’m spending. I want to learn from what I’m spending to decide if I want to spend it again next month for… If I’m getting return on my spend.
Henrique Dubugras: I think like being able to get the data from the customer, process it, and give it back to them in a way that’s meaningful and not just gibberish like most financial services, if you open your bank or credit cards, I think it’s something that’s super important for us.
Nima Ghamsari: Yeah. I’d say our approach is to make our data and our customer’s data a moat for our customers, the banks, lenders of the world. So how do we use the fact that we have so much scale now? We process millions and millions of consumers a year through our platform, to then turn that back into a moat for our customers so that they can become better banks and lenders.
Nima Ghamsari: And so, because we’re able to see how people go through these lending funnels, and these lending flows, and in real time understand where are there opportunities to make things more real time, for example, to drive higher conversion, higher pull-through, higher success with that consumer, we want to turn that insight back into something of value for our customers. So we spend a lot of time on that and creating moats for our customers really because our customers are the ones who are ultimately delivering these products to consumers.
Melisa Tokmak: Awesome. Thank you so much. Henrique, Bridget, Nima, thank you for joining us and sharing how you’re operationalizing AI in FinTech. Thank you for everyone listening in. Enjoy the rest of the sessions.
Henrique Dubugras: Thank you so much.
Nima Ghamsari: Thank you.
Bridget Frey: Bye.