Panel: Enhancing Online Commerce with AI
speakers
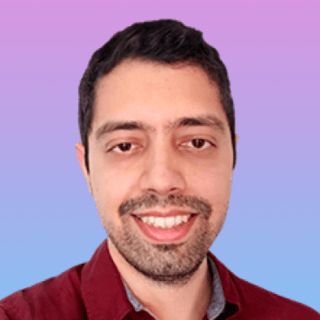
Akshat oversees the data engineering, data platforms and machine learning teams at Redfin. He leads a group of passionate scientists and engineers on a mission to make the process of buying and selling homes easy and fun by providing accurate data and valuable machine learning-derived insights. Akshat has helped build robust machine learning and data delivery infrastructure and large scale applications like recommendations, pricing and Hot Homes. Previously, he worked at Microsoft on Bing search and ads. Akshat graduated from the University of Michigan at Ann Arbor with a masters degree in computer science.
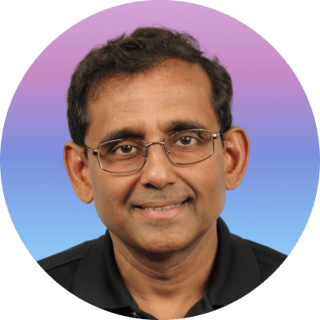
Dr. Ganapathy Krishnan is currently Vice President, Engineering of the US R&D Centre for Flipkart, India’s eCommerce market leader.
Prior to Flipkart, Dr. Krishnan spent several years working on Bing Search at Microsoft. He led teams to improve Bing Local Search quality and also launched the Bing Business Bot for Microsoft.
He then joined Amazon where he led a team of data and research scientists to help Amazon gain insights on its worldwide operations workforce. His team worked on novel approaches to reduce hourly worker attrition and improve the quality of life in Amazon’s warehouses.
He also built a data team at Sweetgreen, one of the hottest startups in the fast casual food industry.
Earlier in his career, he founded two companies with successful exits. He was also CTO at multiple startup companies. Prior to his career in industry, he was a tenured Computer Science faculty member.
In his career, he has published multiple papers on neural networks, Computer Vision and has two patents in eCommerce.
His current interests are in Conversational Commerce and Deep Learning for Natural language understanding and Computer Vision.
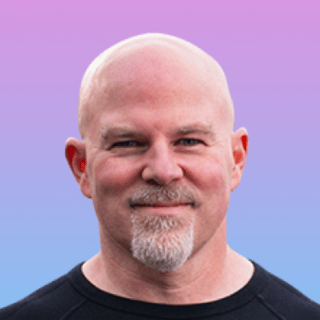
As CTO, David is responsible for the design and development of the FLEXE technology platform. Before FLEXE, he spent nearly 20 years at Amazon, including five years as the VP of Fulfillment Technology, where he oversaw the development and functionality of the technology within Amazon’s fulfillment centers, as well as the technology for Amazon's transportation systems for two of those years.
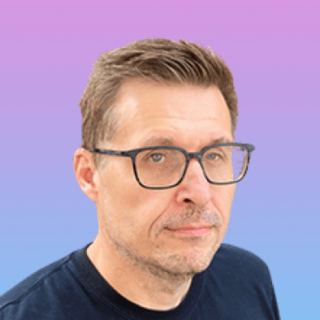
Jason is co-founder and CEO of Shipium where he guides the company's vision towards becoming the world’s best ecommerce supply chain platform. Prior to founding Shipium, he spent 19 years at Amazon in roles such as VP of Retail Systems and VP of Forecasting & Supply Chain. He owned the software and operations that power Prime, Subscribe & Save and Pricing. He is a University of Washington grad, and an engineer at heart who loves solving complex scaling problems.
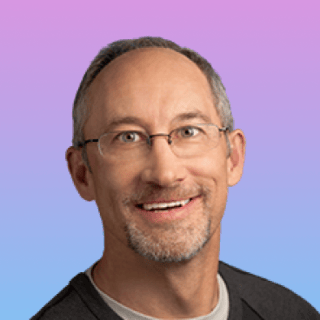
Jim is a former CEO, CTO, Google executive, and Amazon executive. Jim is currently the Chief Technology Officer, Wayfair (he was on the Board of Directors for 3+ years before leaving to take this role).
Jim is currently on the Board of Directors of ITRenew, Brambles Limited, and The RealReal. He is a member of the MIT Corporation, the Board of Trustees of MIT. Additionally, he is an Advisor and multiple early-stage, mid-stage and public companies, venture capital firms, and private equity firms.
Jim’s expertise is helping early and mid-stage companies scale their operations through hyper-growth phases.
As the CTO of Wayfair, he has responsibility for a team of approx. 3500 employees consisting of software engineers, product managers, UX/UI design, and data scientists. He has functional responsibility for all of the technology and applications that power Wayfair. Additionally, he has responsibility for a multi-hundred person data science team.
Jim was Chief Executive Officer of Arevo Inc. In this role, he was responsible for commercializing and ramping Arevo’s continuous carbon fiber 3D deposition technology and fabrication processes.
Prior to this role, Jim was Vice President of Worldwide Operations at Google. In this role, he had responsibility for global operations, planning, supply chain, and new product introduction for Google’s Cloud infrastructure and Google Fiber. Additionally, he was responsible for Google Energy, LLC and Corporate & Social Responsibility. He served as an Advisor for many of Google’s new initiatives in GoogleX, Consumer Hardware, and Verily/Google Life Sciences.
Prior to joining Google in 2010, Jim worked with leading companies in electronics, networking, cleantech, communications technology, manufacturing, and consumer services. His expertise in supply chain was honed at Amazon.com, Cisco, First Solar, Sanmina-SCI Corporation, IBM Corporation, Intel, and Sierra Crest Consulting.
Jim has a bachelor’s degree in Aerospace Engineering from Purdue University, a Master’s Degree in Mechanical Engineering from the Massachusetts Institute of Technology, and a Master’s Degree in Management from the Sloan School of Management at the Massachusetts Institute of Technology where he was a “MIT Leaders for Manufacturing” Fellow.
SUMMARY
Online commerce marketplaces, from groceries to furniture to real-estate and everything in between, are seeking to leverage artificial intelligence and machine learning to enhance buyer and seller experiences. In this panel, learn from executives with experience at Wayfair, Amazon, Flipkart, Flexe, Shipium, and Redfin about how they approach their AI/ML development to improve the customer experiences on their platforms.
TRANSCRIPT
Aatish Nayak (00:38): Welcome everyone. I'm Aatish Nayak the head of product for eCommerce at Scale. And I'm really excited to moderate this panel today. As a reminder for our audience, this panel is about how online marketplaces from groceries to furniture to real estate and everything in between are seeking to leverage artificial intelligence and machine learning to enhance the buyer and seller journeys. Thank you to the five experts on this panel for joining us today. I'm really excited to learn from them. To kick things off. I'd love to ask our panelists to briefly introduce themselves and describe each of their backgrounds, companies, and roles. Jim, do you want to kick us off?
Jim Miller (01:20): Yeah. I'm Jim Miller, I'm the chief technology officer of Wayfair, which is one of the leading online home retail and furniture eCommerce sites. I've got a long history in tech. I was a former Googler and a former Amazonian among other things. So great to be here.
Aatish Nayak (01:38): Dave, over to you.
Dave Glick (01:40): Hi, this is Dave Glick. I'm the CTO at FLEXE and we help shippers get their products to customers faster. Before that I spent 20 years at Amazon. I ran Amazon fulfillment technologies and Amazon logistics technologies for several years before I left.
Aatish Nayak (01:59): Very cool. Akshat over to you.
Akshat Kaul (02:01): All right My name is Akshat Kaul and I'm the head of data and machine learning at Redfin. Redfin is a real estate brokerage founded by software developers. We use technology to make everything about buying or selling a house better and more efficient. And we have saved our customers more than a billion dollars in fees over the years. And we have tons of unique and interesting data, and we rely quite heavily on AI in many parts of our business. Before Redfin, I was at Microsoft and before that in a different life, I used to work at a hedge fund based out of New York.
Aatish Nayak (02:35): Cool. Krish.
Dr. Ganapathy Krishnan (02:38): Hi, my name is Krish and I'm the vice president of engineering and north American operations for Flipkart. Flipkart is the largest eCommerce provider in India. And my focus is really on conversational commerce. Prior to this, I worked at both Microsoft and Amazon. And Microsoft I worked in the search team and before that, I actually worked in a number of companies where I started. And I had several successfully exerts in my career.
Aatish Nayak (03:11): Last but not least, Jason.
Jason Murray (03:13): Hi, my name's Jason Murray. I'm the co-founder and CEO of Shipium. We are a technology driven supply chain platform for eCommerce. I spent almost 20 years at Amazon, mostly focused on the merger of logistics and technology. My biggest accomplishment there by far was building out Amazon supply chain technology to support prime when prime was growing from 2010 to 2016 so rapidly.
Aatish Nayak (03:39): Very cool. Thanks everyone. So taking a step back and looking at the bigger picture initially, online commerce, has a share of global retail sales has gone from 13.5 pre-pandemic to 19.1 this year. In the US alone, it's gone from 11% pre-pandemic to 14% this year, and a lot of accelerated growth from COVID, especially. But even though this represents hundreds of billions of dollars, I was surprised it's relatively so low as a share of total retail sales. So I'd love to kick off and learn from each of you. How do you see the share continue to increase? What's holding back to growth? And then what role AI plays in each of basis to increase that growth? Jim we'll get started with you. Go for it.
Jim Miller (04:25): Sure. Like many of the people here we saw a pretty significant increase in traditional eCommerce and I think it was driven by one, the acquisition of a lot of new customers driven by the necessity during the pandemic to acquire home goods and furnishings and so on. We acquired many, many new customers. I think that trend will continue. Obviously, the pandemic was probably a one-time step event and we'll continue to see organic growth from here. But I think that one of the things that'll continue to accelerate or at least maintain the growth is the necessity to drive the perfect order and the perfect fulfillment concept. Relative to your question around AI, I think, we certainly have seen where the digital first companies had a significant advantage over traditional retailers who probably for variety of reasons were lagging in their investment in technology. And we see ML and AI being everywhere in the business today, whether it's with search and recommendations to fraud prevention, to optimizing logistics, so on and so forth. It's a competitive weapon that facilitates every aspect of our business and will continue to do so. (silence).
Dave Glick (05:58): Yeah, for sure. This trend has been a secular trend for the last 20 years. We went from 0% market segment share of eCommerce to now, whatever you say, 14% or 20%. And we did see a step up last year. And so what's driving that is customers love low prices. They love huge selection and they love getting their products conveniently at their doorsteps. And I don't think that's going to change. Where we've seen the bottleneck, especially in the last few years is I guess this is no surprise that I'm going to say in logistics, right? There's a labor shortage out there. There's a carrier shortage. And we had ship again in part last year, and it's going to be even worse this year, where you've got UPS and FedEx already over capacity.
Dave Glick (06:44): And the only people who can scale to meet the needs of last mile is AMZL as far as I can tell. And so I see that as like the big bottleneck probably for the next two or three years, and let's not even get started on the first mile where you've got ships sitting in Long Beach. I'm actually somewhat of an AI skeptic. So I like to be on these panels because you hear people talk about robots and talk about AI, they're going to save everything.
Dave Glick (07:14): Jim and Jason were with me in 1999, when we put in all the wrong automation at Amazon, we put in the wrong sorter and the wrong conveyor and the wrong pick the light system, because we didn't know what we're doing. And so I would argue first, you have to understand your processes, and then you can use AI, and then you can use automation. You can use robots, but if you don't understand those first you're just wasting your time. And there are applications where AI is super necessary. Elon's driving cars and Amazon's Amazon Go stores completely, top tier AI folks. But let's not fool ourselves into thinking. Everybody can start up an EC2 instance and use Amazon AI make it work immediately.
Aatish Nayak (08:03): Yeah. Jason, do you agree with Dave's view on being an AI skeptic?
Jason Murray (08:12): Well, I'll just say I agree with the trend line. I think that it's clear that the pandemic acted as an accelerant of an already growing market segment with eCommerce. And from what I've seen, it changed a lot of people's habits and once habits change, they aren't going back. So I do believe this was a one-time event that it's going to cause an increased growth in eCommerce for years to come. I do agree with Dave also just on the notion of what's holding back growth. I think one of the more interesting things about eCommerce is there's this physics component to it.
Jason Murray (08:51): And unlike a Facebook feature or Google feature in order to improve operational experience, you need physical infrastructure, which adds complexity and trying to tie that ultimately to the user experience is even more difficult. But for me AI is a tool. So in the sense that Dave's saying AI will not solve everything, I agree with. For my particular space eCommerce is real time complex and dynamic and humans making those decisions just won't scale. You won't be able to build a scalable supply chain supported by humans doing manual processes. And so for me to get to a superior experience that is continually competitive, you're going to need AI and ways to scale those decisions in real time.
Aatish Nayak (09:44): Yeah. I love what you particularly said around there's this physics component for eCommerce. Part of the reason I really like working on eCommerce technology is because it's this unique combination to both the bits and atoms the digital and the physical. But it actually makes it a lot harder because the AI and technology you build needs to really respond to dynamic events in the world, whether it's a global pandemic or disruption in supply chain because a ship gets blocked. So I, I'm curious Akshat from your perspective and connecting home buyers and really enabling home tours in the physical space, how are you thinking of making your AI systems in businesses robust to handle this dynamic world?
Akshat Kaul (10:28): Yeah. Yeah. I think it's been a really interesting time. So I think just to give you some context we've seen just a really crazy amount of price growth in the real estate market over the last year. So we saw almost 26% year over year growth in May of this year. And things have softened up a little bit, but even in August of this year, we were seeing 16% growth year over year in home prices across the country. And another really interesting number is in 2020, 63% of home buyers bought homes without ever actually stepping inside them. So that's just mind blowing.
Akshat Kaul (11:12): That's a big change from how things were before the pandemic began. And even before the pandemic, Redfin was already showcasing 3D scans on the website, really prominently and Redfin already had automatically created 3D scans for every Redfin listing. But to help our customers understand the physical layout of homes even better, we started automatically tagging all floor plan images using computer vision. So there are lots of things that we have been trying to do. And AI has certainly been a big part of it of making the whole experience of being inside a home without actually being inside the home better.
Aatish Nayak (11:53): That's super interesting. It kind of relates to like with eCommerce you really have to mimic this brick and mortar experience on a store and get it delivered, right? Krish, I'm super interested in your perspective here. There's this AI skeptic side, and then there's this combining the physical, digital and what makes actually, eCommerce pretty hard. So yeah Krish love your perspective here.
Dr. Ganapathy Krishnan (12:19): I started in AI many, many years back when it was a lot of hype and I got my PhD in computer vision and AI and for a long time, I didn't do any AI. And the reason is there was no clear way to make it practical. And I got back into AI pretty much at Microsoft. But what you've seen here in coming back to being a skeptic, I was a skeptic, but I'm no longer a skeptic about the power of AI. So what I see in Flipkart like in India, India hasn't jumped on the eCommerce bandwagon as early as the US, for example, right. And India is a very complex country with 26+ languages and thousands of dialects and so on.
Dr. Ganapathy Krishnan (13:04): So it's a very complex ecosystem there. And the other interesting thing about India is that people have not really relied on the postal service to deliver things, right. People have used couriers and they've used... The last mile technology was built out much faster in India than EMZL right. What happened in Amazon because you had FedEx and UPS doing the delivery. So the problems that we had at India were that some of these things were already solved from a last man perspective.
Dr. Ganapathy Krishnan (13:40): The other thing is that during the pandemic, what happened is the lockdowns in India were very, very severe compared to the lockdowns here. For example, I could go to my grocery store and still buy stuff. India, it became more difficult. So a lot of essential stuff had to go through eCommerce. So we automatically saw an acceleration in lot of eCommerce activities in the US. And the interesting thing is what happened is that we started getting more activity in what I call the next 200 million. So the people that outside the metros, right? So there are these big metros where people already use eCommerce, both Flipkart and Amazon, but outside the metros, you have a lot of people who struggle to use eCommerce. And the reason is that they're not being exposed to things like Google search and apps on a phone and so on, right.
Dr. Ganapathy Krishnan (14:37): They all have smartphones, but we don't really want to interact with it the way that most people do. And that's where eCommerce comes in, AI comes in play. AI is really good at things like translating a catalog between different languages, right? So those are limited use case AI that actually works really well in that AI is really good at limited computer vision tasks. And it's really good at telling sellers what metadata they need to put into working. And Flipkart has developed ASR technology, which is really, really awesome, which beats both Google and Microsoft for the vernacular languages in India. And so we really invest around that and we find that a lot of people in this next 200 million place they actually like to come through voice other than through other needs. And the reason is very simple. It's pretty hard to type on a keyboard using vernacular languages, right? For a variety of reasons AI is super relevant. For us it's not a nice to have, it's not like Alexa turn off the lights kind of situation. I don't about that. It's really important to connect, people the next 200 million to the eCommerce platform.
Aatish Nayak (15:58): Yeah. Thanks so much. Yeah, that makes a lot of sense. It seems like Dave and Jason are seeing really this last mile problem. In India, this last mile problem is more or less some are solved and functional and really this first mile and connecting consumers to even starting off the first mile using AI and chatbots. Awesome. So shifting gears a little bit, you mentioned something about really wanting to hone in on, around the catalog quality. So I think one of the elements that I think is under appreciated in eCommerce is how much work actually goes into making a trusted marketplace, which often means maintaining a really high quality asset catalog. So I'm curious for panelists here, how do you think about marketplace trust and catalog quality in your space and how do you actually bring these massive catalogs to customers in an easily accessible and digestible way? Jim, do you want to kick us off again?
Jim Miller (16:59): Yeah, sure. We've got about 16 million items in the catalog and growing, and we look at catalog quality as pretty foundational to everything that we're doing. So first of all, we've historically depended on our suppliers to provide information about the various items and attributes, and that has its own series of challenges because the data quality tends to be suspect. So we use AI and models to basically continue to refine the accuracy of the catalog. And as anybody can attest here, it has all kinds of compounding issues to the customer experience, whether that's selection, recommendation, substitutability all the way down to dim weight accuracy and the mode chosen. So it's very, very foundational to everything that we do. And we use ML and AI as a way to improve the catalog quality.
Jim Miller (18:06): Now, that being said, it really is foundational for us because we build on top of that. Most people can't describe furniture or home decor in the way that an interior designer or decorator could. So we're using more and more auto tagging in a way to actually feed our recommendations and substitutability engines. So for example, we made a significant investment a number of years ago into going to a full digital catalog. And that has really enabled us to work up the stack. And it's enabled all kinds of applications and algorithms for many, many parts of the business. And we can to talk about that, but I'll leave it for the others to comment as well.
Aatish Nayak (18:57): Yeah. One particular thing you mentioned was especially around the dimensions, weight, different packaging. That really has to be correct to ensure the delivery of goods and successfully to custom customers, right? So I'm, I'm curious, Jason, especially you're in Dave's space, how does the catalog quality of your end customers play a role in enabling you to deliver goods to customers?
Jason Murray (19:25): Well, anything related to kind of the physical dimensions of the product or properties of the product, like does it have lithium ion in it and requires special handling or on and on and on? If you don't have that data captured in some way, in an accurate fashion, it's going to affect which ship method you might choose, how much you pay, how you route and manage orders through your network, how you place inventory and which building what you promise to the customer and what the timing's going to look like. So all of those are effect affected when the dimensions are incorrect. Even things like cartonization with other items and it all combines together to basically bad outcomes, an unhappy customer, more returns all that stuff kind of gets fed by that process, that upstream process.
Dave Glick (20:19): Yeah, I was going to put a little finer point on it. And Jason, Amazon, when I was there had billions of items, maybe tens of billions item in the catalog. And how do you figure out which ones have the right dimensions? And it's a very hard technical problem. Even FLEXE where we have tens of thousands or maybe hundreds of thousands of items in the catalog, we get it wrong, a bunch and the suppliers give us the wrong information. One of my favorite stories is like, hey, go run a query and see how many items I have that have one by one by one inch dimensions. And it's like 10% of the catalog. And I'm like, I bet that 10% of the catalog aren't that big.
Dave Glick (21:02): And so we've built basic scripts to identify the one by one by one dimensions and go after the customers or after our suppliers to get the right dimensions. The other one that I always talk about is a box of granola bars, right? There's 12 granola bars in a box, and you have to know, do I ship one of those granola bars, or do I ship the whole box of 12? And if you ship one, you get a nasty customer complaint, you get a nasty customer view, but there's 11 other time bombs sitting in that warehouse now because... And so how do you build the processes around going and finding that box of 11 granola bars and making sure you don't ship 11 more defects and we had built system upon system to make sure that didn't happen.
Akshat Kaul (21:48): But for Redfin, the catalog is basically all the homes in for sale all across north America. And I think in this is actually one area in which I'd say AI is extremely powerful. So I was listening to David talk about his skepticism. And I think a large part of my job is also saying no to problems. This is not the best fit for AI or for machine learning, but cataloging and recommendations is very much one of those places where AI really fits in and really makes a huge difference. So just to give you some context, when I initially joined Redfin, five years ago, recommendations were responsible for about 8% of all of our traffic. And now it's close to like a quarter of all of our traffic.
Akshat Kaul (22:43): So it's grown tremendously. And the really interesting thing about recommendation systems is that they keep getting better as people engage more with them. So you want to get started early. The earlier you start, the more value you get out of it. And it's really been a really powerful tool for connecting people with homes that they would be potentially be interested in buying. And in fact, we've seen more people clicking on homes that we recommend to them than they do on homes that are matching their own safe search. So along with this it's also become very clear that people have started expecting us to know what they are interested in, even before they tell us what they're interested in. So they no longer want to tell you explicitly that this is the area in which they're looking to buy a house, or this is the price range that they have in their mind. They basically expect that we know that already, and we should be giving them the right and relevant information without them having to tell us anything.
Aatish Nayak (23:47): Yeah. Customer is always right, and customer is all knowing, but we have to be even more all-knowing than the customer. Krish I'm curious how recommendations really plays into as you said these dozens of markets with different languages for each market, how is Flipkart thinking about AI powered recommendations in India?
Dr. Ganapathy Krishnan (24:09): Recommendations is definitely a very, very important thing for us. And a lot of times it depends on the catalog, what we can recommend and so on, right? So I'll give you really good example that we face all the time. So someone may ask, let's say that you really want to recommend a certain type of sari to someone. A sari is a dress that a lot of Indian women wear. So now, if you want to recommend the sari let's say the person is from a place called Gujarat. Gujarat is a state in India, and if you want to recommend sari to them right now, if you were to try to find Gujarat saris, you may not find as many Gujarat saris in the catalog. So what we are doing to solve problems like this is we're building a knowledge graph. And what we are doing with the knowledge graph is we are connecting this concept of Gujarat with something called Patola.
Dr. Ganapathy Krishnan (25:05): So in that area is called a Patola sari. And so we are able to recommend things in a very intelligent manner, because we are able to connect things, connect the dots, using the knowledge curve. So those are some of the ways that we are doing recommendations. The other way that I've seen recommendations done, not necessarily at Flipkart is really to... And I did this in a previous, another company is to use the concept of embeddings, right? So you take the whole concept of word embeddings, right? And you apply it to the space where you can find objects in the similar embedding space that are close to each other. So I actually did this at a company called Sweet Green, where I was heading the data science and data there. So we actually created salad and embeddings where salads that are close to each other so we could recommend that. So people are actually going to things like this, other than some of the old approaches, like collaborative filtering approaches and so on. So these embedding approaches are becoming more popular and we'll probably leverage more of it in Flipkart as we build out a recommendations engine.
Aatish Nayak (26:14): Yeah. Jim, particularly you want to hone it on your point around you said Wayfair, went from physical to complete digital catalog. How recent was that and is that really enabling you to do more fancy things like embedding based recommendations?
Jim Miller (26:32): Yeah, it's a journey that started about three and a half years ago. And we built tools that enable our suppliers to do a lot of digital capture in their facilities. But yeah, it's enabled, it has been foundational and an enabler for many of the things that we're doing now. One of the challenges that we've got is most of our customers are women. They don't know how to describe home décor. They want value. They want something unique for their home, but they don't know how to describe what they want, but when they see it, they know that that's something that matches their style. So again, once you have a catalog that's enabled with digital tagging, and you can build a knowledge graph around it, you can take, for example, a photograph and do image search against it.
Jim Miller (27:22): And you can actually tag something and say, if you buy that, it will clash with that style. Because again, these are significant purchases for people. And through a lot of focus groups, there's a high degree of fear and anticipation around am I buying the right thing for my house? But there's also a very emotional connection to it. So, using that foundational data and then being able to apply it to style guides, to the real customer intent and what they're trying, if you do it right, you create this incredibly emotive attachment and people feel, there's this joy and this, you know endorphin flow of how they're decorating their home. And it creates a tremendous amount of stickiness with the app and the application. So yeah, it has a nice flywheel effect around.
Aatish Nayak (28:20): Yeah. Yeah. I'm assuming one of the things that especially with this recommendation, you're trying to reduce a basis of returns that customers have to do if they don't really like a product they buy, right. And customers really want flexibility above all else. And that often means free returns and of course returns are quite costly to retailers and marketplaces. One crazy fact that I came across was that an average return represents around 30% of the purchase price. So and for reference the average margin to a marketplace for a retailer for an online order is only 10%. So for $100 shoe or $100 piece of décor, the retailer has to sell four units of that same shoe to make up for that loss for one return. So I'm curious, especially in Dave and Jason, your space in the context returns, how do your teams think about this problem? And you may disagree, but what role can actually AI play to reduce the return rate through the customer journey?
Jason Murray (29:29): I think generally speaking returns are kind of sometimes necessary and sometimes unnecessary. If you don't have good information and sometimes, people like buying multiple pairs of shoes and drawing on the one they like or there's entire business models centered around I want to buy all this stuff and see what it looks like on me. But there's an aspect of returns that has to do with delivery experience and basically item description.
Jason Murray (30:00): And I think, in the classic sense of trying to really improve those inputs as much as possible to try to reduce that very, very expensive transaction on the backend, which in addition to the cost of that you have a situation where you've also kind of lost the trust of the customer. So all kind of negative in that sense. So we think of it in terms of if you've got the correct information, you're going to be able to do a better job, making the correct delivery promise. You're potentially going to be able to get it to them faster and cheaper, and the item is going to be what they expect undamaged, et cetera, and all of that leads to lower return rates on the backend.
Dave Glick (30:44): Yeah. And I'd throw one other thing in there that, it costs you 30% of the total cogs or total top line to take the return. But then if the product is damaged, it costs you 100% of the cogs. And so it's just a nightmare. One of the funny thing I've seen is lots of people saying, oh returns is not well met. There's no good operation who is doing well at returns. And so people are starting their companies and asking me to look at their perspectives. And it's often marketing people because logistics, people don't want to have anything to do with it because it's like it's 100% defect rate. Everything that hits your dock is broken, either it's missing a barcode or the customer didn't want it, or it's damaged. And so it's just a nightmare. And so, I guess I would challenge people with, or without AI to try to figure out how to do returns well. There's a huge market there.
Aatish Nayak (31:46): Yeah. One way to avoid the word returns is really to understand the customer well, recommend them the right thing. And that often begins with customer support, customer service. And so, Krish, I know you've thought a lot about applying conversational AI in the context of customer support and helping with returns and throughout the buying journey. So I'm curious, where do you see really, it's like return rate coming into play in the context of Flipkart?
Dr. Ganapathy Krishnan (32:18): So the thing about returns is, when I was at Amazon, I visited one of the centers there and they said, the best customers call is no call, right? And that's what Amazon does all the time. And the reality is that what do you need to have is a process by which you can quickly identify which products are having problems and for you to be able to deal with it quickly, because you can't cut all these things out completely, even with all the processes in place and so on, you can't cut it out. So the key thing is, when you notice it as a problem, you've got to essentially pull the cord and say, there's a problem here. Let's go fix all these issues as to why this product is getting returned, right?
Dr. Ganapathy Krishnan (33:07): And it may sound reactive, but in some cases, we need that safety net so we don't bleed more than necessary. From a customer service perspective, our goal is really to make the process as simple and is really not focused around saving money at that point in time, right. If the customer is already pissed off with us and we'd rather, address them as quickly as possible and save them for another day. So that's really what any kind of conversational AI order is focused on. And we have agents in the backend. So we'll try to answer them as far as possible to bot. And if he can't, then we will transfer them to an agent who can take care of them. And really the role of AI is how can I answer 80% of inquiries with a bot and 20% of the more difficult queries with an agent? That's what it is, it's about optimization, right? So that's what we're focusing on from a CX perspective, it's to reduce the cost of actually dealing with the customer. And the savings are quite high. I looked at that and if you can save these costs, it would mean a big addition to the bottom line.
Aatish Nayak (34:23): Yeah. Akshat, I'm super curious customer support is super important in the context of a realtor because this is one of the biggest purchases that the customer is going to make online. So I'm curious how you guys are thinking about this problem.
Akshat Kaul (34:37): Yeah, yeah, absolutely. Redfin's business, we are a real estate brokerage, so our entire business is customer service. So just like David is a bit of an AI skeptic. I'm a little bit of a chatbot skeptic, at least in the context of real estate. So, instead of building chatbots, what we are doing is we're building tools that help our agents and our customer service professionals become more effective. So whenever one of our users has a question about maybe the Redfin estimate. So the Redfin estimate is a tool that effectively values each home, every home that's on sale, or even that's not on sale in all of the US and people of course, are really invested in the value of their home. So as you can imagine, a lot of people have questions about why the Redfin estimate is a certain value.
Akshat Kaul (35:26): So we have built tools that help our customer service agents explain why the Redfin estimate is a certain value. So they might be able to tell customers what are comparable homes that are being used to generate the estimate. They might be able to tell them this is all the information that Redfin has about your home. And if any of this information is incorrect, you can tell us and we can correct it and then that will hopefully, you know, change the estimate or improve the estimate or make it more accurate.
Akshat Kaul (35:54): So accuracy and explainability are both equally important for us. And what we're doing is we're trying to build these tools that help our customer service agents help our customers better as opposed to building a chatbot. Another thing that we have is, there's this thing called a competitive market analysis. The CMA, which is something that a listing agent will create for somebody who's trying to sell their home. So we have used machine learning to automatically generate CMAs. And that shows information about the home compatible homes, information about the neighborhood and this saves our agents thousands of hours of work every year. So these are really the ways in which we think about using AI to improve customer service right now.
Aatish Nayak (36:47): Yeah, super interesting. Thanks so much for walking us through it. Taking a step back. We've talked about a lot of applications of AI in some applications that don't make sense. One of the common things though, as you scale technology teams, utilizing data science and utilizing AI in production is how to ensure all the data management model deployment productionization are standardized and taking advantage of economies of scale. A typical path we see companies take is moving from decentralized ML and data science teams to centralizing the common building blocks into a cohesive platform. So I'm curious, Jim, how do your own experiences match or differ from those?
Jim Miller (37:30): Yeah, it's actually a great question. We have a federated model, so it's no secret that we use GCP, Google Compute Platform to run much of Wayfair. Our underlying data structures and ML platform is centralized. And our data engineering standards are centralized as well, but when it comes to, and I run a centralized data science team, but that being said, the actual data scientists are federated in a way that they're accountable to the business owner. So for example, there's an embedded data science team in search and recommendations and notifications. There's an embedded data science team in fulfillment operations.
Jim Miller (38:19): But again, I think it's the healthy balance of trying to figure out for the purposes of standardization, data standards and quality and ML platforms, again, around scale and leverage that that's a centralized, but would drive any results. And ultimately the actual tool or applications being engineered and the solution, it's a very, very tight alignment to the business owner who then can dictate the priorities and the things like service level agreements and the just the six critical success factors around that infrastructure. And it's an ongoing debate all the time around what is that right model, federated versus central.
Aatish Nayak (39:06): Yeah. Krish, how does Flipkart think about organizational models for ML and AI productivity?
Dr. Ganapathy Krishnan (39:14): I think pretty much the same way that Jim described it. It makes a lot of sense that a lot of the, what they call the [inaudible 00:39:22] part is centralized, but best practices and things like that. We do it in a central way, but all the data centers are embedded inside the business units. And it's actually more fundamental for data centers because you don't want to be a service provider. The worst model is for you to be providing service to somebody else. That's not the idea. The idea is you need to really understand the domain and the business. But at the same time, you also need to understand what are some of the best practices in the eye and how you do that. So in this model that Jim described is the one that we use, it works really well for us. And I wish other teams did that as well in the sense that sometimes there's a tendency to centralize certain things. And it actually removes the accountability from those people who are in the centralized team providing "a service."
Aatish Nayak (40:14): Akshat, super curious how Redfin does this.
Akshat Kaul (40:16): Yeah, I think everything that Krish, Jim just said really resonates with me. So what we are doing at Redfin, we actually started with a completely centralized model, like instead of having little pieces of different teams doing AI and machine learning across the business, we had just one team doing machine learning for the entire business, but all the businesses that Redfin in. And that obviously is not a scalable model. So we are now moving to a place where infrastructure is centralized and machine learning model building is decentralized. So people within each business that Redfin engages in whether it's smart guage, whether it's, iBuying, instant buying whether it's a brokerage, they all have people who have a lot of domain knowledge and they all have folks who are capable of building machine learning models. So we have sort of democratized machine learning across the business while centralizing the infrastructure and the best practices.
Jim Miller (41:14): Yeah. I'll have one comment to that. It's interesting because when we look at the amount of time that our data scientists are actually doing data science problems, we were, this is primarily in the past, pretty embarrassed by that because we found that the data scientists were actually doing machine learning engineering and data engineering, and again, part of the evolution. And it sounds like we've all gone through a similar evolution is to get the data scientists, to be doing real world value, add data science problems and not all of the backend scale and ML platform work. And again, I think that's another key ingredient of scale.
Aatish Nayak (41:57): Yeah. Speaking of organizational development, I'd love to start closing out this panel and learning really all about yourselves, you're so incredibly talented, experienced and impactful technology leaders on this panel. So I'm curious as you've built technology teams, as you've experienced multiple high impact growth, what's one cultural value you really emphasize with your teams. And then how do you put this into practice? Dave let's get started with you.
Dave Glick (42:33): Yeah. Great. I go back to the two-pizza team model at Amazon, which is you should own all the resources that you need to get your problem solved. And everybody hates shared resources. Anytime you say, I have to share my resources with anybody else I completely check out is that means I have to go fight with Jason, or I have to go fight with somebody about getting these engineers allocated. No, if it's important, we should have engineers who are single threaded, who are focused on the problem at hand.
Dave Glick (43:04): And anytime you have a shared resource... And sometimes you have to do it and we have to do it at FLEXE because we don't have the scale to give everybody their own data scientists, or we don't have the scale to have a whole team that's focused on this. But the goal is to get to a scale so that you can get rid of shared resources, one thing at a time, and whether it's data scientists or DBA's, or recruiters or whatever, I will guarantee you, if you go talk to your VPs of engineering, that they will tell you that their most frustration is, comes from where they have to share resources with others.
Aatish Nayak (43:40): Yeah. Krish gives a thumbs up. Jason how do you emphasize cultural values and then how do you put them in practice?
Jason Murray (43:49): I mean, definitely to echo what Dave said, maybe another way to kind of lean into it is from the standpoint of the Amazon leadership principles. I would say ownership was up there as one of the top, most important things that made the Amazon engine go. For my own organizations, I just think it's extremely important that everyone's pointed towards the same north star and that they believe that they're contributing to the success of the organization. And I think pizza teams is a great way to do that. But just in general, this notion of I'm continually adding value to something that someone needs. I think the second piece though, which I personally just found to be the most striking thing in way Amazon leadership principles is that customer obsession component. And I think you have to build that in from day one.
Jason Murray (44:41): And I know that in general, everyone says that, we're customer focused. There is lip service to that for almost every organization unilaterally, but I just think that the way that Amazon made that such an important part of the culture and basically just demonstrated on daily basis, whether that was from press releases or all of these other tools, that relentless focus on the customer is to me something that was striking once you leave Amazon and you see how it's less of a thing in other organizations. So for me, that's a really, really important one when you're trying to decide how to kind of focus your organization.
Aatish Nayak (45:29): Yeah. Thanks so much, Jason. Krish, were you going to say something?
Dr. Ganapathy Krishnan (45:36): Yeah. So and firstly these two gentlemen beat me to it, but I'm going to say something else. I agree with all of the great things he said, but I think the important thing I think is that you need to experiment frequently. And because you know what happens is sometimes you want to try something out and then someone comes back and say, that's going to take six months to build it. And that's only the problem because you don't know whether that that's going to work or not. So one of the things that I tried to tell my teams is I want you to experiment frequently, with POCs and show some proof of results before we put a lot more resources into it.
Dr. Ganapathy Krishnan (46:14): So be smart about how you fill these things out, right? And that also goes into the customer part, right? Because it's easy to say not everyone says I'm customer obsessed. And the way that you should be customer obsessed is by experimenting and seeing what the customer is doing. Are they reacting to this or not? If they're not reacting to this, try something else, right. Then you need to do this thing very quickly because you don't have a time to build full products and so on before you determine what is it that the customer likes. So finding market fit, customer fit require experimentation and speed.
Aatish Nayak (46:53): Everyone says, they're customer obsessed because no one wants to say they're not customer obsessed.
Akshat Kaul (47:00): Yeah. I think we have a slightly different term for experimentation. We call it failing quickly. So just daily go out and try things out. And the sooner you fail, because many times you will fail, the quicker you can get to the win. So yeah, I think a lot of these things that folks have been saying, customer obsession, ownership, experimentation, these all resonate. I think we also have quite an emphasis on collaboration and teaching. So people stand on the shoulders of giants and we want everyone to be a giant. So that is a big focus, at least at Redfin, that senior engineers mentor junior folks, they share their knowledge. There are no silos being created inside the organization where only one person understands the product. So that's also a part of Redfin values.
Aatish Nayak (47:49): Yeah. My last question, before we close out this panel is what are your top predictions for AI's impact in eCommerce or otherwise over the next five years? Jim, you want to give your take?
Jim Miller (48:04): Yeah, I think relative to what maybe some of the other said, I still stand by my conviction that I think you're going to see ML and AI used pretty much everywhere. Now there are areas where I think it's going to be deeply penetrated, like search discovery, recommendations, notifications, et cetera, et cetera. And there are other areas of the business, like logistics, where I think it's going to be a surgical application where things like operations research and traveling salesman problem isn't likely going to be solved in some novel way by ML. But I do think we're going to look back in probably 10, 20 years and we're going to feel like, wow, we can't believe that we were doing things in such an antiquated fashion back then, but the computational power or the algorithms, the data is going to continue to improve. And I think it's going to have profound implications and it's going to be an accelerating force between the leaders and the laggards.
Aatish Nayak (49:08): Yeah. Dave, I'm curious your predictions for the next five years for eCommerce or otherwise.
Dave Glick (49:17): I think it's gone now. I think it will miss our expectation in the short term, in the next few years and exceed our expectations in the decade in the 10 years’ timeframe that like all of a sudden, oh, that doesn't quite do what we need. We can deal with this script. And then all of a sudden what just happened you know, we just got left behind if you're not continuously experimenting, making sure you're keeping up with the latest in AI and ML and all the latest technologies.
Aatish Nayak (49:45): Yeah. Krish top predictions?
Dr. Ganapathy Krishnan (49:54): Yeah. I'm going to start with a not so fun prediction. Not so fun prediction is that governmental will intensity regulations about the use of data and hence companies that are very transparent with users about how their data is being used will prospect. Companies that hide this information will face increasing government scrutiny and punitive lawsuits. So this is what's going to happen, whether you like it or not. Next is that, on a more positive note, I think conversational AI will become very big in much of the developing world. Voice will be the primary medium in many parts of the developing world in contrast with text or interaction with an application. I think generative models will be able to combine information from different sources and create cogent answers. And lastly, I've been a fan of visual search for a while. Visual search will finally have its day. It's not as big as we want it to be, it will have its day. People are really visual, and this will be huge for areas like lifestyle and fashion, where a picture is worth a thousand words and really the other thing put up by saying, I really enjoy using both Wayfair and Redfin. I use it on a regular basis. So thank you. So all the rest of your doing, over.
Aatish Nayak (51:21): Jason, top predictions?
Jason Murray (51:24): Well, I mentioned at the beginning, just the inherent tie of physics to eCommerce specifically, and I actually think that we are about to watch a massive growth in physical infrastructure to support eCommerce, which is going to do a couple of things. One it's going to increase the complexity. And two, it's going to give us access to a lot more visibility into where and how things are moving. And those two things together I think we're just at the beginning of being able to use those data sets and access to that such that companies that don't adopt this method are probably going to fail in the next 10 years. And secondly the use of ML and AI to basically give customers transparency to their goods, create business models around delivery speed and expectations, and just bring cost efficiency to the whole segment of fulfillment and logistics is going to change the result of AI.
Aatish Nayak (52:25): Last but not least Akshat, top predictions.
Akshat Kaul (52:28): Sure. Yeah. I can maybe talk a little bit about the real estate market since other folks have covered all of aspects of eCommerce. So one area that I think, AI will really have a big impact is instant buying. So for those who aren't familiar with, what that is iBuying is when an institutional buyer like Redfin or Zillow or Opendoor makes an instant cash offer on your home and then make some improvements and then puts it back on the market for resale. So the Redfin estimate is a really critical piece of technology that is powering iBuying business. And it really helps us decide what price to buy a home and what price to sell it after making improvements. So just looking at [inaudible 00:53:05] 21 in the second quarter of this year, about 1% of all homes sold nationwide were bought by iBuyers.
Akshat Kaul (53:13): And this was up from 0.5% in Q1. So it's doubled in one quarter and in more established by iBuying markets there like markets like Phoenix and Charlotte and Raleigh, iBuyer's account were almost 4% of sales in the second quarter. So we expect that this trend will definitely continue and AI will become more and more important in real estate pricing. Another area within the real estate space is this 3D tours, virtual tours. So I expect that we'll be able to use computer vision to do even better at giving people a sense of things like the condition of a home. Is it good? Is it bad? What types of appliances countertops, floorings does it have? Things like that. I think those are going to keep becoming more and more interesting and important.
Aatish Nayak (54:08): Great. Well, that's all we have time for today on this panel. I want to thank our five panelists. Jim, Krish, Dave, Jason, Akshat for really spending their time and then sharing their insights with us today. I really hope everyone's excited as I am for future of online commerce and AI. And yeah, I see my packages I've actually arrived, so I'm going to go get them right after this. Thank you.
Jim Miller (54:34): Thank you.
Akshat Kaul (54:35): Thank you.
Jim Miller (54:35): Take care.