Panel: Breakthroughs in NLP and Future Potential
speakers
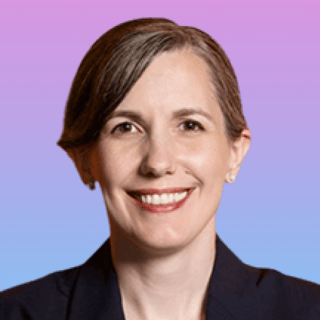
As the Global Head of iQ at Qualtrics, an experience management software platform, Dr. Catherine Williams leads the engineering and applied science teams that build advanced intelligent features into the Qualtrics experience management products and platform, leveraging cutting edge text analytics, predictive intelligence, and statistical analysis to help customers better understand and act on their data in real time. Catherine has extensive background and expertise in data science and beyond; prior to her work at Qualtrics, Catherine led data science, analytics, and product organizations at AppNexus and Xandr (part of AT&T) as Chief Data Scientist and Chief Data and Marketplace Officer. She received her BA from Grinnell College and her PhD in Mathematics at University of Washington and held postdoctoral fellowships at Stanford and Columbia Universities. Outside of work, Catherine enjoys exploring the outdoors, running, and spending quality time with her two boys.
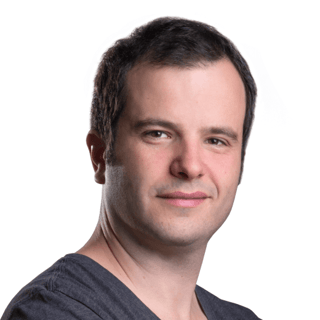
Julien Chaumond is Chief Technical Officer at Hugging Face, a Brooklyn and Paris-based startup working on Machine learning and Natural Language Processing.
Hugging Face has been described as the most influential platform in modern Machine learning. As a co-founder, Julien is passionate about democratizing state-of-the-art NLP and ML for everyone.
After graduating from Ecole Polytechnique and Stanford University, he has been a founder or team member on several Machine-learning based startups. He was also an advisor to the French Minister for Digital Affairs, where he managed to get the French laws published in Git!
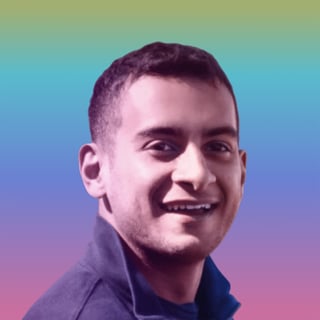
Aatish is the Head of Content & Language products including NLP, Speech, Cataloguing, Classification, and Search Relevance. The team focuses on empowering customers in social media, e-commerce, and broader enterprise to get diverse human insight on content quickly and fairly. Aatish previously was an early engineer at robotics startup Shield AI, focused on building AI systems to protect service members, and Skurt, an on-demand car rental marketplace acquired by Fair.com (http://fair.com/). In college, Aatish ran Autolab.com (http://autolab.com/), a learning management startup used at CMU, Cornell, Rutgers, NYU, PKU, and others. He graduated with a B.S. in Computer Engineering from Carnegie Mellon University.
SUMMARY
Industry leaders share their perspectives on the state of NLP research and applications, and discuss where NLP goes from here.
TRANSCRIPT
Aatish Nayak: Hey, everyone. And thank you for attending this panel at this conference. Hope you’ve enjoyed the talks and the panels so far. I’m really excited to kick this one off. I’m Aatish, I lead our content language engineering teams at Scale. Addressing annotation use cases in NLP research evaluation and product cataloging. And excited to be here with Catherine and Julien. And really, I think what’s special here in regards to NLP is Catherine, as the Head of Global IQ at Qualtrics, who leads engineering and ML teams applying NLP in Qualtrics products.
Aatish Nayak: Catherine, you really bring this platform or sorry, in this industry perspective on the use case and deployment of NLP solutions at an enterprise scale. And Julien as the CTO of Hugging Face, through the community driven approach that you guys have done, really has been described as the most influential platform in modern machine learning. You really bring this platform perspective on kind of making the horizontal tools, community medium to democratize access to NLP models and data sets out there.
Aatish Nayak: So really excited to have these two perspectives. And so just to kick off, to learn a little bit about your guys’ backgrounds and what you do, Catherine, like many others in AI, and machine learning. You really come from this deep math background, how does your background lead you to AI and NLP?
Catherine Williams: Great. Well, thanks Aatish, it’s a pleasure to be here. My background started off with academic pure math, doing geometric analysis in general relativity. Pen and paper math has nothing to do with NLP as a matter of fact. It’s a little bit of a securitas route, I initially aspired to stay in academic, but then partway through realized that I felt very out of touch with what was happening in the world, and the impact of my work just felt like it was non-existent, five people read your paper and then that’s it.
Catherine Williams: And so I jumped into industry and sort of rode the wave of data science and machine learning as I went. And so that’s what’s brought me here today, and I’m really excited. My goal is to bring these math and technology to life to have an impact in the world, and I’m in a good place to do that.
Aatish Nayak: And tell us a bit about what your teams do at Qualtrics for those unaware.
Catherine Williams: Sure. Well, first of all, Qualtrics is an experience management platform, and what experience management really means is, helping companies of all kinds use experienced data, that is to say the experiences of the customers, or their employees, or their prospective customers to be better versions of themselves, to have better customer experiences, have better employee experiences, et cetera.
Catherine Williams: And a huge amount of that experience data is in text form. Think about some of it’s in survey data, NPS surveys, C-SAT surveys, whatever, but an awful lot of it is in social media reviews, or open-ended verbatims, or just people writing out, or saying their thoughts. And so part of my role at Qualtrics is leading the AI team that extracts that signal, and really tries to understand those experiences at Qualtrics for the benefit of our customers.
Aatish Nayak: Awesome. And in Qualtrics and in your past, what’s really changed about working with ML and NLP over the years for you and your experiences?
Catherine Williams: Oh, my gosh, it’s a sea change. When I jumped to industry, it was 2012, which as everybody knows now is a huge year for machine learning. But at the time it was a blip on the radar. And in fact, because I was coming out of academia, I really just wanted to have an impact. So I sort of wanted to stay away from hype, and I really just focused on some of the simplest tools to do the job. Use logistic regression, don’t go to the big fancy stuff, logistic regression will work do that, scikit-learn was our friend.
Catherine Williams: Over the years, as deep learning has proven that it can actually be really effective and even cost effective at solving some of those problems. It’s just completely transformed the way we think about NLP from bag of words to transform our models today. It’s entirely different, it’s actually a hammer worth using now, whereas previously it was sort of more about the hype.
Aatish Nayak: Awesome. Turning over to Julien, tell us a little bit about your background, and why you and your team started Hugging Face?
Julien Chaumond: Good question. At Hugging Face the whole team has been really passionate about machine learning for a long time now, coming from diverse scientific backgrounds, echoing what Catherine said. My co-founder Thomas Wolf, was actually doing quantum physics. So kind of different from machine learning obviously, but also kind of similar in some sense. We’ve been really excited about machine learning, and in particular natural language, we started the company back in 2016, which was another big year for machine learning and NLP in particular.
Julien Chaumond: We started really seeing a lot of what we were doing in open source, and we’ve been completely floored and completely impressed by the contribution of the community on top of the code that we released. And we’ve been super lucky to be at the center of this community of contributors from scientists to machine learning engineers, and of building this community of people, super talented people, working together to build the future of artificial intelligence.
Aatish Nayak: Awesome. In this kind of short amount of time, Hugging Face has really become a commonplace term for anyone doing NLP. And so why do you think this community driven open source approach really took off for you guys?
Julien Chaumond: I mean, pretty simple reasons, actually, if you think about it. The big tech companies like Google, Facebook, Microsoft, they’ve been doing incredible research in machine learning. But they haven’t been that great at bridging research to production, most of the time the great projects that come out of their research labs, they kind of push it into the open and it’s great, but they don’t invest in them over time.
Julien Chaumond: And we’ve been lucky to be able to work pretty early on with those teams, and also a lot of other teams in smaller companies, in universities all over the world, and aggregating all those people doing great projects, and making them easy to use, easy to benchmark, easy to compare has been a pretty cool project.
Aatish Nayak: Really interesting. It’s kind of bridging this research and production environments and really making sure that developers on the production side are actually empowered to use a lot of cutting edge research that is coming out. And so I know probably a lot of people in the audience are wondering, in the audience and in the world, why is it called Hugging Face? You have this really cute emoji on your shirt. Why did you guys decide to name it that?
Julien Chaumond: Good question. It’s kind of a unique emoji in a way because I mean, it’s an emoji, but at the same time, it’s one of the few emoji’s that have human features, the hands. And it’s also giving basically the most human of gestures, which is a hug. It’s kind of in the middle between a robot and a human, it’s kind of a good metaphor for what machine learning is.
Aatish Nayak: Really unique. It really allows you guys to stand out names and become a commonplace term. Honestly, I thought it was something to do with the unicode encryption of that emoji, but I guess not.
Catherine Williams: The real reason is so much better.
Aatish Nayak: Yeah, it’s symbolic. Love it. And so moving on a little bit, and this came up a lot in the rest of the conversations in the conference today, really over the past year advancements in NLP. I’ve really showcased the acceleration happening in research and industry access for both developers and ultimately end users. And so things like, obviously GPT-3, adoption of transformers, with Hugging Face’s open source library, self supervision, and Dolly clip, et cetera. It really feels like we’ve entered a new era.
Aatish Nayak: I think Catherine, you mentioned this, it does parallel what happened in computer vision in 2012, with the ImageNet challenge, and the winning from AlexNet architecture which really kicked off this deep learning revolution. And specifically that this imaging task really ensured that the pre-training of CV models, can learn general purpose features, and ultimately achieve state-of-the-art results in new tasks in that similar domain.
Aatish Nayak: And so we’re transformers in general, language models, many are saying that we’ve hit this seminal ImageNet moment for NLP. And so for both of you guys here, one, do you guys agree, have we hit it? And the second thing is really, why now? What are some of the things that have come together to make this possible?
Catherine Williams: Well, I certainly agree that we’re there. I won’t speak to the research side where obviously ImageNet opened up whole new avenues of research and problems and domains. But at least for us now with transformer models and NLP, it makes accessible a technology that just wouldn’t really have been fully accessible to us for business applications otherwise.
Catherine Williams: We can now quickly stand up classification algorithms that serve core business needs, and we can do it efficiently with our team, and with hardware in ways that we just really could not have. That contribution just opens the door to being able to use this technology where it just wasn’t previously. So it’s a step function for us.
Julien Chaumond: And the same feeling on my side. I mean, we’ve kind of like entered this paradigm shift where in NLP you used to kind of train a new model from scratch for every new use case that you wanted to achieve. And now you take your pre-trained model, take care of a super large model that we trained on a very large amount of data in a self supervised way. And then you find tune it on a specific use case. And it works really well, right?
Julien Chaumond: This shift to models that are super large, pre-trained, and then you fine tune on specific use case has been taking off in NLP for the past two years, and leads to a lot of companies being able to deploy models that work way better than before for a fraction of the cost, because you basically just need to fine tune the model to your specific needs to also way more data efficient. The amount of annotated data that you need for your use case is a way smaller.
Julien Chaumond: I mean we’ve seen a ton of companies where NLP was more on the research side a few years ago, and now it’s really getting into production, and they are building a ton of end customer value around NLP right now.
Catherine Williams: And a huge thing for Qualtrics too, is that cross language models are just huge for us because only needing to fine tune with a small amount of data across all the different languages that our business touches, Qualtrics is global, right? That’s incredibly important for us to be able to support our customers worldwide. Whereas previously building out individual models was just prohibitively time-consuming and expensive.
Aatish Nayak: There’s these trends coming into play where these large general language models actually require very large computation power as well. And so I’m curious about both Catherine and you Julien, how do you guys see this computation bottleneck actually coming into play when you’re deploying models into production?
Julien Chaumond: Obviously, most of those models are pretty large. There’s a lot of stuff happening on the optimization side, both like training and inference, which is how you use not only in production for a specific use case. We’ve seen a ton of breakthroughs over the past few months in efficiency. So yes, you need a lot of compute, but the whole community is in the process of making it easier and easier to do that, and in a way that’s more and more efficient on the compute side.
Julien Chaumond: What’s really cool, it’s only that, before companies used to train those models for themselves. And now we see this trend where a ton of companies are using pre-trained models, but then also sharing the fine tune models. It’s kind of materializing compute which is great, I think.
Catherine Williams: For us, I think the compute changes have gone hand in hand with the improved accuracy that we get from language model based transfer learning. Qualtrics has for years run our hardware on-prem, we have our own data centers that we take care of and so forth, CPU based. And so it had just never quite been worth it to buy GPU’s or to extend it to GPU based deployments in the cloud using hyperscalers until we got to some of the cutting edge models that actually give us the accuracy gains to make it worthwhile for business reasons.
Catherine Williams: But as that technology arrived and we’ve discovered that we can actually get really cutting edge accuracy when we use these techniques, it makes it worth it. Now we’ve switched to hyperscaler based deployments in the cloud for both training and inference, and that works out great. We have elastic scaling and everybody’s pretty happy. It works out nicely.
Aatish Nayak: Is it that these hyperscalers are like you’re trading off compute for accuracy? Or how are you thinking about the accuracy versus compute trade-off or curve here after you have all these GPU’s running in the cloud?
Catherine Williams: For us, I think it’s a cost versus accuracy trade-off. And so the increased cost of running in the cloud on GPU’s is offset to some degree by the elastic scaling that’s provided, which is nice. And it’s completely made up for by the business value of being able to run high accuracy say, sentiment and other types of classification models for the business, because that’s a key insight for our customers, so it winds up being worthwhile. Once the accuracy gets good enough, the cost is worthwhile, is what I’m trying to say.
Aatish Nayak: Julien, anything to add there?
Julien Chaumond: I mean, we’ve seen a ton of companies have different production constraints, and it’s super interesting because there’s a lot of diversity there, depending on the type of model that you’re running, depending on the latency or regulatory constraints that you have, there’s no one size fits all solution. But yeah, that’s pretty exciting because basically we are not over-fitting to like one specific model, one specific way of deploying this explosion of different ways to do it. And I think that’s great, because we’ll explore more of the space of potential things in the future. That’s cool.
Julien Chaumond: I’m pretty excited about custom inference hardware as well. It’s like as it goes like a GPU is still a very, very general purpose device. Basically you can do a ton of things, it’s not optimized for one specific type of machine learning operations, for instance. And like the number of tech companies are working on custom inference chips can already kind of play with them in the cloud and cloud providers.
Julien Chaumond: I think it’s going to be interesting and we would love to help facilitate the move to those kinds of production devices if it makes sense, because they are designed from the ground up for this trade of compute and accuracy that you mentioned.
Aatish Nayak: Just to dig a little deeper, how does Hugging Face provide the software framework and layer, thinking about redesigning it or changing it with these new hardware that’s actually coming out? How does that affect your software right here?
Julien Chaumond: We have a small research team that’s working specifically on the technical subject of sparsity which is basically a lot of the parts of the large neural nets, you can basically say it’s just zero, right? Basically kind of like saying that this huge matrix of numbers, you can pretty much get the same accuracy if you just say turn like 95% of the numbers into zeros, because they are not the important ones.
Julien Chaumond: If we find a way on specific hardware on the widest possible range of hardware to make it 95% more efficient, and faster with the same accuracy, I mean, it’s going to be a game changer in terms of ML deployment. We have a small team working on that subject. The technical subject is called a Block Sparsity, and kind of how you train models that are going to expose the right amount of sparsity at the right places and how then you make it efficient to run.
Catherine Williams: That’s really cool, it’s like compression, the compression algorithms applied to the models.
Julien Chaumond: It’s a kind of compression. There are tons of different ways of compressing a model that’s really cool with the Block Sparsity, is that it’s completely orthogonal to the other ones. The gains that you have on this side, you can pretty much multiply them by the gains that you have on other more classical types of compression like quantization and knowledge distillation stuff like that.
Aatish Nayak: How does that relate to you yourself, the work you did in 2019 around this distill parts method of really compressing some of these models?
Julien Chaumond: It’s completely orthogonal, so that’s nice because you can kind of combine the benefits of all of those approaches. So that’s nice. Obviously distillation is great, but in any trends on all hardware, it’s not hardware specific, but if you want to go like an order of magnitude, or two orders of magnitude, deeper into efficiency, you kind of need to do stuff around deeper architectural changes to the models.
Aatish Nayak: Catherine, you’re in production and enterprise scale settings. Are you thinking about these distillation methods, and shrinking down models, or is that not necessarily a factor you think about right now?
Catherine Williams: I would say for where we are as a business right now, not yet. Right now, we’re focused on getting product market fit at a reasonable price, and building up the models that are really going to help drive the business and satisfy our customers. And then I think there’s a certain point, we’ll kind of plateau, and start looking at the cost, and the margins and where can we do things more efficiently? Where can we fine tune? And at some point there’ll be some diminishing returns, so we’re not there yet, but we will at some point I’m sure.
Aatish Nayak: You actually mentioned that it’s really great how far NLP has gone, where you only have to think about NLP, and these layers of abstraction now. And so just like you mentioned in one of the other talks with Francois, around how TensorFlow is attracting the top of the hardware, and the Keras is kind of attracting the top. Catherine, could you break down some of the trackings from a technical exec level that you have to think about in terms of designing NLP systems?
Catherine Williams: Well, as an exec, I mean, I think about the top most layer of abstraction to me, which is, can we build out a thing called an AI algorithm that does the following? And I think what’s helpful here is that when you think about what really brings an algorithm to life, you have to have somebody like a researcher or a scientist. You have to have the engineering the way to implement it to connect it with the business, and then you have to have the business reason.
Catherine Williams: And they all have to be working closely in tandem, I call it a trifecta, right? In order to be able to actually make an impact. And in order to do that, there has to be a little bit of a closed loop. And so there have to be abstraction levels that the product manager or the person who’s reflecting the business needs can understand, and that you can quickly iterate. Can we get product market fit? Can we build an algorithm? Can we try it out? Can we do something quickly?
Catherine Williams: And what tools like Hugging Face provide is layers of abstraction that enable us to sort of quickly pull together some building blocks, some legos where we can say, “Hey, is this thing going to meet our business need, right?” And so I as an exec, only need to know sort of that you can put some shapes together that do what we need as a business. And then the team can go off and figure out how to sort of build it for real and fine tune it if that’s what’s necessary.
Catherine Williams: Being able to abstract away from hiring the people who know how to go deep into the technical deep neural network implementation, and fine, and tweak hyper parameters, and build out just so on, put it on this architecture before we even know whether it’s going to really solve the business problem. That’s incredibly useful to me. So the layers of abstraction that Hugging Face and others provide is critical.
Aatish Nayak: I mean, it seems like the main thing that can be a huge benefit here is iteration speed, and really evaluating, and confirming that this business problem is something that you need to do. And going back to what we were talking about earlier around, now we have the general language models that are pre-trained now, and then we have GPUs in the cloud, and computation is like is okay to have, because we have now more accuracy.
Aatish Nayak: It seems like the limiting factor, and this was discussed in a lot of the previous talks to the limiting factor to neural net performance is really this domain specific data, or data annotation that really is crucial to pre-training and fine tuning these models. Both Catherine and Julien, I’m curious, are you also seeing this data annotation bottleneck now emerging, since all these other problems are somewhat solved?
Julien Chaumond: Yeah, that’s super interesting, because kind of counter to the intuition, you could think that those large models they’ve been pre-trained on self supervised sources of data. Basically you need less data upfront, and then they are more data efficient to fine tune, so you need less data to fine tune them to a specific use case. I mean, you need less annotated data than you use to fine tune them to a specific use case.
Julien Chaumond: What’s kind of cool is that we’ve seen that the need for annotating data has actually grown up, because yes, for one specific domain you need probably less annotated data. However, as it’s been easier and easier to fine tune more models and also it’s been easier and easier to actually as Catherine said, deploy them on business centric use cases. People are starting fine tuning more of those models. Kind of moving away from annotating super massive data sets that are kind of low value to this more targeted approach of annotated, or ton of different pretty small data sets that you use to fine tune pretty specific models is what I’ve seen.
Catherine Williams: That tracks with exactly, I think my perspective, which is maybe there’s some sort of fundamental conservation of annotated data principle at play, or maybe it’s once you have cheap and easy to get electricity, you come up with all kinds of electronic gadgets or something like that. Now that it’s easy to do, let’s fine tune all kinds of different models for all the different nuanced business cases where we can drive value, yeah let’s do it. I think we’re continually going to be hungry there. I wouldn’t say it’s a bottleneck so much as taking advantage of an opportunity though.
Julien Chaumond: I would say it’s less of a bottleneck now, because you can start iterating with way smaller data sets.
Julien Chaumond: When before you were limited by the need to construct the super big data sets of, I don’t know, 100,000 samples before being able to even see if it was going to work or not. I think it’s less of a bottleneck now, but it’s more of an enabler of new cool use cases. And I feel like also the iteration cycle of labeling training. I mean, labeling training using in production has gone way, way faster and more seamlessly which is great.
Aatish Nayak: That kind of parallels what we’re seeing in the market, where a lot of customers are asking for this like really fast experimentation platform for getting label data and really, really quickly as well. And that is just a shift from what was done before, where it’s like you compile these huge massive data sets, then train someone offline, and then come back and then iterate, where now it’s like really small packets of data they’re usually done really quickly.
Aatish Nayak: Catherine, related to what you said around electricity is very much like a Moore’s Law where there was authorization, computing, and transistors on a specific chip, and how many you can fit on there. But that didn’t really reduce the computation and chip market, it really honestly just increased it, because the big thing is that there are just a lot more problems to solve now.
Catherine Williams: Exactly, exactly.
Aatish Nayak: Shifting gears a little bit, going back to your Catherine, what you said earlier around language coverage. It seems like user applications are global, coverage in English like many other languages is not that much of a concern where through transfer learning we can be confident that we can take a German model, and retrain it for French let’s say. But I mean, how confident are we in non European languages, low resource languages like Malay, Bengali, and others. Julien, I’m actually curious from your perspective, how are you seeing companies solve low resource languages in NLP today?
Julien Chaumond: Well, the thing is that probably the business use cases are kind of lagging, because a lot of companies they use to focus for first maybe 10 most popular languages which is bad, right? Because if you do that, you kind of perpetuate this circle where less resources are going to go into already kind of low resources languages. We try to foster the community into kind of taking the role of building this community where there is a focus on increasing the diversity, and the coverage of those models.
Julien Chaumond: We’ve been super lucky to work with tech companies that have been providing compute for instance, we are in the process of doing very deep community events, where community members fine tune speech recognition models. Speech to text models in, I think, 80 or 85 different languages. And what’s super cool is that some of those languages, we couldn’t find any public machine learning model to do speech to text on them up until now.
Julien Chaumond: I think that’s great, just like speech to text outside of maybe 20, 25 languages. Just there were no models until now. If we can help the community to experiment with those models, we have kind of like breaking the loop of more resources going into already resource rich languages.
Aatish Nayak: Catherine, I know you mentioned this earlier. How are you guys thinking about Qualtrics as a global company, addressing a lot of these ML and NLP use cases in so many languages?
Catherine Williams: Well, two things. One is, this is absolutely a place where industry follows research. I think we’re leaning on the research community to sort of help figure this out and we will try to fast follow with whatever they find. And number two, that’s because we’re driven by business needs. Our global footprint is expanding into regions where I think customers have some expectations for these tools.
Catherine Williams: In a lot of places, we’re not necessarily being asked to fill gasps for low resource languages, which is great for now, but I think we will quickly get to a point where we need to figure out solutions. I’m hoping that Julien and the community will come up with all the answers and then we’ll use them.
Aatish Nayak: I mean, particularly Julien, you mentioned the models, but what about the data sets in these different languages, how do you think about the community driven approach for that?
Julien Chaumond: We’re building a lot on the data sets that have been built by the community. To continue with the example of speech to text. The community events that we are doing right now is based on a data set called a Common Voice, that has been built by a ton of different volunteers and the leadership of Mozilla. It’s like a Mozilla project, great contributors have been basically reading texts, and they released what they’ve been reading. And so we kind of have this great data sets of, I don’t remember the number of hours of recorded speech, but it’s pretty unique, right?
Aatish Nayak: And I presume you like the data set marketplace that Hugging Face has opened up in addition to your models marketplace, because even the language I speak Kudrati, which is a somewhat archaic Indian language, there are so many different data sets that I didn’t know existed. That I found through Hugging Faces marketplace, the community driven approach is working within a surface that is kind of working.
Aatish Nayak: We’re heading towards time here, but I want to give a chance to ask one of the questions from the audience. The question was asked, what are some of the biggest weaknesses in your mind of transformer models in NLP today, and how are we trying to as a community research industry overcome them?
Julien Chaumond: I can maybe go first. In my mind, those models are still too hard to use at scale in production. They’re also kind of hard to train, hard to fine tune. That’s a lot of things that we can still do to make it easier and easier to train, to fine tune, and then to run. A lot of the things that we are going to build in the next couple of years are going to revolve around that.
Julien Chaumond: And obviously, I mean, the models, there’s a ton of research on how to improve those models. I think that we are still at the beginning of this scale, it’s going to be super exciting to see what’s going to happen, but I feel like we still have a lot of progress to make on those models.
Aatish Nayak: Very much day one essentially, right? Catherine, anything to add there?
Catherine Williams: I don’t know that I have any complaints about existing transformer models, I think we’re pretty excited about being able to use them in the ways that we are. Sure, if they could be cheaper and easier to use, I would certainly take that, so let’s do that. I think I’m particularly interested in the next wave of research on embedding more structure, so that rather than being… Some of the discussion on GPT-3 has talked about how its understanding is shallow and where we can embed a little bit more reasoning into some of these. I think that will be a very, very interesting next frontier. And when it gets there, I’ll be happy to try to make use of it for business applications.
Aatish Nayak: One trend we’re seeing is that moving some of the or using some of the context from texts definitely, but also using a lot of the context from vision, and audio inputs for multimodal data, and multimodal models. And so I’m curious, is multimodal learning and models being used in practice, or is that still something that’s being explored?
Catherine Williams: For us in our applications not yet. I can imagine use cases where it would be, but I think we got to nail the individual pieces, the modes one at a time before we go-
Aatish Nayak: One mode at a time.
Catherine Williams: Yeah, yeah. But I think that there are some pretty interesting possibilities there. So Julien, when will we have all the multimodal goodness?
Julien Chaumond: Hopefully soon. I mean, I agree that it’s still more on the research side. I haven’t seen a ton of applications or companies using multi-modal models right now. But it’s definitely something to follow. To kind of follow up on the topic of structured data, putting more structured data into NLP, into machine learning. One of the models that I’ve been super impressed with in the past a few months is this model from Google called TAPAS.
Julien Chaumond: And what it actually lets you do is basically not only query tables in natural language, also actually do computation, you can ask for the sum of the number of words in a document, or some of the average value of specific piece of data that you find from Wikipedia, or stuff like that. And it actually recomputes them, so that’s really awesome. I mean, it’s still like a statistical model, obviously we are very far from having actual intelligence inside the model, but I felt like it was one of the demos where I was super impressed and I feel like the business opportunities of using those kinds of models are basically endless.
Aatish Nayak: Can’t wait for that to come into Google Sheets, or Excel, or something like that, just to reduce formula making.
Catherine Williams: There are a lot of [crosstalk 00:42:02] out there on the frontiers of research that I think could come together over the next era, like automatic theorem proving, and this kind of technology reasoning could come into play. I don’t know, that’ll be interesting.
Aatish Nayak: I mean, two particular examples of that are with these large self supervised models trained on very large internet sources, we’re seeing them learn for example, the periodic table of elements from millions of scientific journals, or really writing code through clever prompt engineering. And I find this pretty fascinating just because no one really intended to make that happen, but it is now happening. Curious as we’re closing, do you guys foresee models being used successfully in these very open-ended ways, or still fine tuned and trained for specific tasks?
Catherine Williams: I’ve been surprised by what is possible a few different times over the course of my career now as an AI observer and practitioner. I don’t know, but it seems like it’s at least plausible it will.
Julien Chaumond: I think that in real world systems, people are already kind of mixing and matching deep learning models with other types of systems. Kind of this vision of the next generation of machine learning, and software engineering, as this kind of meshed subject where some parts are machine learned, and some parts are more kind of like traditional computer science. It’s something that 's very exciting, but it’s also kind of already the case in some form, as soon as you can reflect to those machine learning models inside all the complex systems. I think we’re going to see more and more of those types of complex systems, and are going to achieve pretty cool stuff.
Aatish Nayak: It’d be amazing if every system just takes natural language input, whether or not it’s generating images, audio, or videos, or anything. A great tool just for example, generate stock images just from texts as we’ve seen just three or four months.
Julien Chaumond: I mean, natural language is basically the API of humans, right?
Catherine Williams: Exactly.
Aatish Nayak: Yes, yes. Well, a great way to end this presentation and in session, natural language is the API for humans. Thanks so much, Catherine and Julien, for taking the time to chat with us today. And hopefully you guys learned a lot too.
Catherine Williams: It’s been a pleasure, thank you.
Julien Chaumond: Thank you.