Advances in AI Robotics With Marc Segura of ABB
speaker
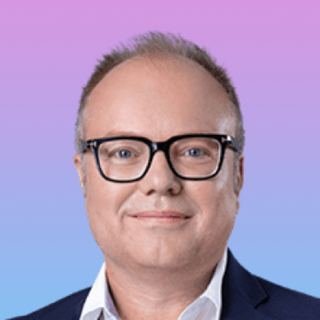
Marc Segura is The Managing Director of ABB's Consumer Segments & Service Robotics (CSSR) Business Line. He was appointed to this position in 2019.
The CSSR business line delivers value-added scalable and flexible solutions for the entire value chain of consumer goods and healthcare across the whole lifecycle, helping our customers to sustainably provide the goods and services people need.
Marc, a Spanish national, has been with ABB for 23 years serving in various management roles based out of Spain. Between April 2013 and December 2017, he was the Local Division Manager for Robotics and Motion in Spain and Portugal until he was appointed Global Manager of ABB’s new Service Robotics business.
He holds a degree in Industrial Engineering from the Catalonian Polytechnic University UPC and two executive management programs obtained in SDA Bocconi and IMD Lausanne.
SUMMARY
Marc Segura is the Managing Director of ABB's Consumer Segments & Service Robotics (CSSR) Business Line. He joins Scale AI CTO Brad Porter in a fireside chat to discuss the current state of AI in robotics and how AI can be used to advance the field to create robots that are more advanced, efficient, and safer.
TRANSCRIPT
Nika Carlson (00:22): For our next speaker. We're thrilled to welcome Mark Segura. Mark is the group senior vice president and managing director of consumer segments and service robotics at ABB. Mark has been with ABB for 23 years, serving in various management roles based out of Spain. He now manages the delivery of robotic solutions for the entire life cycles of consumer goods and healthcare services to sustainably provide the goods and services that people need. Mark joins Brad Porter Scale AI's CTO for a fireside chat. Brad, take it away.
Brad Porter (01:05): Welcome everyone. I'm Brad Porter. I'm Scale's CTO, and I'm incredibly excited to have the opportunity to host this conversation with Mark Segura from ABB. Mark, and I had the chance to cross paths a bit when I was working on robotics at Amazon. And it's great to be connected again. Mark, I think I'll let you do the honors of introducing yourself because we'd love to hear more about your background.
Mark Segura (01:37): Thanks Brad. And thanks Gail. It's an enormous pleasure for me to be here and to represent ABB as well. So I'm Mark Segura. I'm the global managing director of consumer segments and surrogate robotics in ABB. I've been 23 years in the company and I think I can say I've done most of the jobs you can do robotics. So I'm really passionate of technology of solving problems with the customers. So I hope I can contribute in this chat to share a little bit of division about robotics in general, especially how AI is really changing the game here.
Brad Porter (02:15): That's awesome. I actually want to start and learn a little bit more about your background. What was your path to robotics and to ABB?
Mark Segura (02:24): I think that was kind of destiny because I mean, I'm from Barcelona in Spain and I started engineering, electromechanical engineering, and then a master degree as well in robotics. But while, while I was growing up, I mean, I had a cousin of my mother was working at the Nissan factory. He was working in repair shop and while I was starting, he said, okay, you need to choose one topic, make a little work on it. I said, okay, maybe my cousin can bring me in the car factory. I've heard, it's amazing. There's full of robots. And I went in and the first thing I saw my face was some ABB in the repair shop okay. I was about 15 years old and that really shaped me. There was huge machines, big things, moving fast. And I could feel I mean the bits and bytes flowing through to make that animated.
Mark Segura (03:14): So that really triggered my interest. So that's why it started. And at the end of my degree, I just sent one resume that was to ABB and I was called, okay, that was June. And the 1st of July, I was starting in '98 in ABB and the induction process was pretty sophisticated. I was given a four days training on robots and I was given a flight ticket to a renewal factory. And at that time robots were orange robots are the orange ones. So that started then I was a couple, three years doing installation and projects in fourth in Australia, in France, in renewal doing PLC, PC, robot programming and the mechanical electrical installation the side management. And then I ended up at General Motors in Mexico. One year they're building the factory in Silao with a lot of American companies, Allen Bradley and others.
Mark Segura (04:07): There was a great learning. And that was really, I mean, great time to really learn in the field, what this is all about. And then from that, I took into project management, operations management and was running the whole business of ABB in Spain for automation for three or four years. And then when I was running a 300 million business with 400 people, I was asked by the global robotics boss, you want to jump into a startup? We're looking beyond robots in manufacturing. We're looking at logistics, healthcare, surrogate robotics.
Mark Segura (04:41): And I said, yes. I mean, what's the deal. He said, we have 10 people in UK. You want to take it? And I said, yes. So that was four years ago. And now our business line consumer segments is above the 300 millions. We have done some very nice acquisitions of logistics in Belgium, recently, the mobile robotics of our state. And it's not only our merit my team's merit it's really, we are in a change of paradigm in the market. I mean, Amazon is a new bread person they are pretty much guilty about this change in the world, but very much the supply chain and the value chain from manufacturing to distribution to commerce is changing, has fused. And this is now where we are on a mission here today.
Brad Porter (05:24): The yeah, incredible progress. I mean, I think we often think about the combination of robotics and AI as being a big unlock for a robotics revolution. But in many ways, robotics has been transforming industries for a long time, right? And so what have been some of the kind of game changers you've seen in your 23-year career in the way robotics are used and deployed
Mark Segura (05:56): It's really changing a lot. So that's why I always say that robotics it's for all the problems with we've it's in its infancy, because robotic was born in automotive and it grew up in automotive in the '80s to basically take on repetitive and old tasks, like spot welding and paintings, tasks that were unsafe, that required a higher degree of quality and repetitiveness. So that's why robots were massively growing in the body shops and in the paint shops and in repair shops. That shaped the robotics of the '80s and the '90s clearly because of automotives have certain needs and they are extremely good at certain things. So the focus on the technology development was driving robustness, repeatability, reliability of the product, as well as cost down. There was tremendous innovation to bring the costs down. So automotive was driving that. And that evolved then in the 2000s, because also one thing from robotics is, I mean, it can take any technology development.
Mark Segura (06:57): That's also the beauty of robotics. We can derive any exponential. So when the 2000s have started, there was an expansion from a segment perspective, we started to do more in food and beverage in pick pack palletize later on came the boom of electronics from 2008, 2009, we started to install lots of robots in electronics. So this was this manufacturing expansion within different segments. And this is just a data point, which is very referenced. If you took the IFR international Federation of Robotics numbers, the decade of the first decade of 2000 was stagnant. The number of robots sold per year was steady around 100,000. So you would wonder we were doing a bad job altogether in robotics, but after the 2009 crisis, and until in one decade, it has multiplied by four. So we are above the 400, really close to the half a million industrial robots sold per year.
Mark Segura (07:48): That's only industrial. It does not account for service robots. So robots were still in this manufacturing, working in a controlled environment, it's manufacturing. It's a jig based. So robots had, I mean, I would say not a lot of intelligence. Okay. If you look at it from a modern perspective, they were doing repetitive tasks. So they needed the control environment. They needed the jig, they needed a predictability. Everything is designed for the robot automation thinking, and that worked very well and still working very well expanding in industries, but there was a natural constraint.
Mark Segura (08:19): How do you get into unstructured environment? How do you get into where things are unpredictable and robots need to be adaptive? And that's the latest development where Cloud computing, AI, the development of batteries for mobile robotics, you name all these developments, the sensors developed by iPhones and so on. All this is flowing into the robot as technology breaks. So we are now ready and we'll talk about what AI is bringing to solve more problems to expand robotics, really, really mainstream in any kind of segment. We're seeing the growth of robotics in healthcare, in logistics, in restaurants. I mean, and this will be a total game going forward.
Brad Porter (09:02): Yeah, you alluded to the differences when you move beyond manufacturing, but I wonder if you can color those in a little bit more for our audience, as you move into logistics and others, where did the problems become stickier?
Mark Segura (09:18): Yeah, I mean, in a manufacturing environment where robots are taking tasks, they are taking repetitive tasks that are also designed for aesthetic conditions. For example, if you need to weld to metal sheet parts, okay, this metal sheet parts will be located, will always be the same because you are doing a limited number of cars. Even if it's lots of different cars in more lines, it's still a limited a known number you know what, from the parts that going to come. You're going to have toolings and fixtures to locate them in the exact same position. So then the robot with the field inputs can blindly go and repeat the task. I'm going to apply welding point here, here, there, and there. Okay. So the conditions, the environment is fixed is engineered upfront, and then it stays forever at the same. So robot interaction and robot adaptability is not dated.
Mark Segura (10:11): The robot is kind of blind. Okay. But that's solving a lot of problems as long as your environment is like that. Because robots, for example, have been doing machine tending for ages, that was one off, but to load or unload on a machine is a simple operation because you are picking an object and putting it in a concrete place, and then you're taking it from that place and you're dropping it out. But that same problem becomes extremely difficult when you don't know which parts you have to pick when you have never seen, which is the challenge in, for example, eCommerce.
Mark Segura (10:47): So robots have been doing machine tending for ages, but the environment was that I know that I have two, three, four, 10 different parts. Anyhow, I know them. I have the cat throwing and I can make some jigs to locate them. And I know where to put them. So the robot can do that repetitive task. Take the same abstract thing. It's the same operation because you're going to pick and place. But instead of having 10 known parts, you have, I don't know, one million different SKUs that are changing every day. You cannot program that. You cannot have the drawings for that. You just need to deal with that a new thing every time over and over. And that's something that traditional engineering could not solve until AI came up.
Brad Porter (11:28): Yeah. Pivoting more into AI. Give me a little bit, your thoughts on how you build up robotic skills. Do you build them up one at a time and compose them or learn fully integrated skills at once? Or is it a combination?
Mark Segura (11:45): First I think a little bit too grim what Scale is where, when we think about Scale, not even in a robotic environment, we see that as a sequence of actions and decisions now, I mean that are solving some tasks. The first thing in robot skills, which I believe by the way is a very important concept going forward and I'll elaborate on that, thinking about Cloud-based robotics and so on. We really need to break down these skills into certain smaller skills. For example, if you want, as I alluded, you want to pick something from a box and put it into another box. That could be an order fulfillment process, simple as that in eCommerce. You need to break it down. First thing is you need to localize the box. That could be one skill. Then you need to localize the object inside the box, segmented out from the rest, and then you need to decide how to pick it, actually pick it and then comes a dropping process.
Mark Segura (12:39): So those skills are now becoming... First there are some hard skills that are more related to the motion control of the robot, but then there are other skills that are more related to the application. And what AI is bringing is a great possibility to develop robots skills that are learning over time. And if you really reinforce them by having them located in some kind of a central learning place could be the Cloud would be the Edge, it doesn't matter.
Mark Segura (13:09): Over time, you're going to do a specific skill better and better and better. And then of course you can start to relate them and sequence them again at the Cloud level or an Edge level. So robot skills and eventually a skills net. Okay. It's something that is very likely in the future. As robots are using more AI to become better and better at a certain skill, the skill could be grasping. The skill could be quality inspecting a welding seam. Okay, I'm doing the welding seam, it could be setting the parameters for painting a car with all the parameters of viscosity, humidity that only AI can do that is a specific skill. And that skill will be growing over time if we have the right architecture, which now is possible.
Brad Porter (13:55): How do you think about, pushing the boundaries of the types of robotics skills that can be built? Is it the framework and architecture, or is it the new techniques or new sensors? Where do you see the greatest innovation?
Mark Segura (14:10): I think with unlike any innovation, but I think the bottlenecks for scaling robotics and our robotics are still pretty old I would say. Here, we're matching hardware with software, and that's the big difference from AI in pure software. For more of that, we can scale the software part. There has to be some way to standardize or harmonize the hardware part anyhow, you're always hitting the same problem which is this, you need some hardware ad-hoc engineering to scale up or to define any project. And I think here the definition of the robot skills will help a lot. Okay. I think if we start to have some ecosystem of skills based on kind of app concept that people can start to reuse that will, and it's already creating an ecosystem of the matching and the factors for that, for example.
Mark Segura (15:05): So you can really relate the mechanical part with the software part and then this real, real, that's the boring part of it. But Brad, that's really the bottleneck as we're seeing right now. Okay. Then the innovation, we will not be lacking. Why? Because you take machine vision that is really industrial grade. We can really peak and place almost any object. Okay. Now the bottleneck is how we run the last mechatronic part, the end effector piece. If you take advanced navigation, I mean, and the autonomous car guys, no small, but that we know, and you know that in slow speed, autonomous robot, we can already navigate quite smart and save in 3D and do smart things about, for example, understanding the world semantically. So I'm an AMR. I know that if I move towards the person is not the same than moving towards a box because I have the semantic understanding.
Mark Segura (15:57): So I think the innovations that will enable robotics is everything related to be able to operate on a dynamic environment. Okay. That stands for the machine vision and the ability to autonomously grasp objects. And the other big, big element is the autonomous mobility to have the ability to robots having different flexible workflows, okay in a facility enabled by 3D navigation. And the third one is the quality inspection. The advances of quality inspection through thanks to robotics are also very important in industry because the ultimate vision is that you have embedded quality optimization, meaning I am performing a process.
Mark Segura (16:41): Take a very analog process, arc welding, or painting. You have lots of tuning there, the intensity, the temperature, I mean the humidity. I mean, and you have really gurus experts. A guy that's done that for 25 years is the best, but the only one in the world who can do it? That data we'll talk about data later. That data now can be used to be understood and to be in real time, correcting the process. So autonomous grasping autonomous navigation and in the loop quality improvement and quality assurance, these are the three big things that they are will change robotics.
Brad Porter (17:21): Yeah. Let's pivot a little bit and talk about data. I mean, as you have more of these systems deployed with more and more richer sensors, there's massive amounts of data, right? But you also alluded to other sources of data, the humidity in the room. Other things that you might not traditionally think the robot has access to. So there's a lot of untapped data. There's massive amounts of data. How do you think about using that data as an asset and how do you think about what's possible now is we can process more data?
Mark Segura (17:55): We are extremely excited about it because I mean, for good or bad, our robots or the robots are at the heart of the manufacturing processes and going forward in the distribution process. And so on, they are there, they are the witnesses and they are the starrings. So if we give them the sensing of the environment and we use the data on their process and their decision-making process, that can untap a lot of value. Going back to the data. So I was already alluding to it quality. Okay. I mean, quality is one of the most important things for our customers, of course, when you're a manufacturer. But the reality is that the data is unused, totally unused. I mean, when you cannot gather real time data and use it where you're based on statistical process and that's the state of the art, but we are using samples and statistics, but we are not using the real-time complete data.
Mark Segura (18:52): If we can use that on untaped data, the potential is huge. And again, I'll put you two examples that we are already having a real projects in the world. Take paint. As I said, painting any painting process. That's very, very complex from a process standpoint, as I said, you need to have a variety of viscosities of the paint. You have different temperatures, humidities. So that is very analog process. Now, how do we really correlate? How do we find out out of that data, the patterns, okay? And that's where AI is very strong. How do I look at the patterns? How can I find a heated model and how can I use that heated model to regulate or self-regulate or self optimize the process. That is one important aspect where data will be used in process. So processes can be paint, can be arc welding, can be many other things.
Mark Segura (19:44): If you go more to other types of data, of course, you go into order fulfillment and you have this goods to person AMR saying, you need to fulfill an order, of course, using the data from the consumers. I mean, which SKUs are bought with which frequency when and using AI to better allocate. I mean, this is what I mean, Amazon pioneered and many people are following right now. You have a more efficient storage and retrieval system. Okay. That's also AI and robotics using data. And I could talk about many more. I mean, what we see also coming, as you said, is putting the human in the loop. Okay.
Mark Segura (20:23): We have the rise of collaborative robots and we're going to have more and more mixed work in between human and the operators and robots. So if the robots get to know and can measure, for example, the average stack time of a person, or if a person is behaving in a certain way, and they can adapt to the person and optimize the process, this is really something we're also looking at with sensor fusion. We're using graders, we're using 3D cameras and we are passing that deep learning to understand certain behavioral aspects. And that can in a simulation environment be also utilized under the design phase where you're designing a corporate station and you're putting the human in the loop there.
Brad Porter (21:02): Yeah. You just touched on collaborative robots and I've been excited to ask you more about robotic safety, but I think unless you're deeply in the robotics world, I don't think people have really fully picked up the importance of this transformation to collaborative robotics, or even what collaborative robots in an industrial or logistics or services space are. So maybe start by helping define what collaborative robots are and illuminate more that the transformation that's enabled.
Mark Segura (21:37): I think corporate has been kind of a fluid definition, but I think right now it's pretty static. I mean, the industrial robots are characterized by being very robust and fast. Okay. Because ultimately they were there to maximize productivity and output. And because of that, they had to be in cages. Okay. At the same time, they were probably because they were grown in certain industries. They were really complex engineering machines. Okay. I mean really the use was complex. The design of the process was complex. So what cobots try to address was somehow the two things, they are bringing robots that are inherently safe, meaning that you don't need any more the fences, of course, that comes sometimes at the expense of performance. But in many, many occasions, there's still a benefit. So you can have now robots closer to the people.
Mark Segura (22:32): And that is really triggering a totally different thinking of the workflow. That's the most important consequence. You don't need to separate robots and people anymore. And that together is boosting the productivity of the solutions we can have because it's a different thinking that where we can really optimize, because we don't have the physical separation, but then comes the second part, which is the ease of use the interfaces, the intuitive wizards. So we can make those robots also available for wider industries, SMEs and still I mean industry. But at the same time, the next step is to put them in retail or the robots we have in China allowed in restaurants. Okay. I mean, they have to be easy. You're not going to have a tool maintenance engineers of robotics in a restaurant in case they... It has to be easy to operate, it has to be easy to troubleshoot, it has to be friendly. So cobots are this new type of robots that are safe, light and easy to use. And they enable different workflow thinking on the industry and they are expanding robotics into more human environments in services.
Brad Porter (23:31): Yeah. I saw firsthand the transformation when you can design a robotic system where the human can help, right. Can get involved and correct something. I want to talk a bit about sensors. I mean, I alluded to my barcode work and I did get the chance to work with the first kind of digital CMOS sensors, which were 256 pixels by 256 pixels it's gray scale, super noisy. And now we have these phones with 20 megapixel or better incredible cameras. And obviously that kind of sensor improvement helps to make all these systems. I'm curious, what sensor advances you're most excited about these days?
Mark Segura (24:25): Of course, first and foremost, any vision related capability? Because as I said, seeing the world enables understanding the world. And this is coming from a human perspective, how we are mostly designed. And that's why probably we can better design things based on vision than anything else because of our own nature. And so as I said, if robots can see, okay in the end I mean robots sees an obstruction because robots will see bytes will feel bytes, but then they can't process that. They can't really start to have a semantic understanding of the world. This will enable even with those technologies to take super smart decisions that were not possible. I put the example of the AMR deciding which path to choose, depending on if a palliate is a static [inaudible 00:25:16] has a driver and can run or not.
Mark Segura (25:18): But I can put you all the examples where this decision making of the robot, based on the fact that I know if something is here and it is this, and it has this properties, or is that it has different properties just with all logic can trigger a new level of performance of the robot. So for me, everything's related to machine vision and machine vision applied to mobility, as well, as I said. These are two fundamental then they are of course, when you go in the area more of the assembly, for example, or robot processes, all the interfaces for force sensor are becoming very important. And especially now that we have reinforced learning, if you can have the force sensing in the look, for example, for pegging hole problems for a fine assembly for polishing, that is giving you a new dimension also to solve this more problems that required a lot of accuracy in the process.
Brad Porter (26:12): For the last few questions here, I want to pivot a little bit more to giving our audience a perspective of where you might see things going. I was recently asked for an article to say where I thought maybe there was excessive hype, and I kind of pointed to humanoid cobots. And then a week later, Elon Musk announces he's building humanoid cobots, but I'm curious, how do you think about the evolution of this kind of very general purpose humanoid robotic capability?
Mark Segura (26:52): Well, I mean, of course that's the moonshot, but we have our vision where we want to take robotics. And somehow that's part of it. Two years ago, when we opened in Houston, at the Texas medical center, our incubator for robotics in healthcare, we unveiled our concept robot of mobile human, which was the collaborative dual alarm robot on a mobile platform. Okay. As a preview of the work we're doing to bring to the market mobile manipulators, okay. So robots, one arm or two arms in a corpse built on a mobile platform. Now in Houston, couple of ways, we just received the second version of that concept product. And we can say that we are working to develop that we have the acquisition of ASTI mobile robotics. So we have even more competence on the mobile platform part, but how that will scale, we are very, very down to earth in the sense that we are looking at real use cases where the need for a mobile manipulator makes the return on automation better.
Mark Segura (27:54): And I'll put you a very concrete example, low batch automation, and you can take a laboratory in a hospital. They never stopped. They run 24 7, but the different type of tasks they do during the year, they change a lot. They have a batch of work that a robot could do in one hour, they could pick and place, load the machine, but then the robot is finished. What are you going to do with that robot 23 hours? Because the next thing they have to do is a different process in the lab. So are you going to put another lab in that other workstation and have the robot working another one hour there? That will never pay back? You better have a couple of robotic peers in the lab on wheels. And the lab manager has a scheduler and say, hey guys, from 8:00 to 10:00 you work in that workstation.
Mark Segura (28:38): When you're done, there's a queue that's going to dynamically change it. You're sent to workstation number three. There, you need to load the centrifuge and so on. So it could be a lab hospital could be any other, an SME where it has low batches that would not pay off for a robot standing still 80% of the time. But if that robot can do always something different because it can move among [inaudible 00:28:59], that is a very important use case. Then what are the skills going back to the beginning? What robot skills you need for that? At the beginning, it's mostly going to be about machine tending and material handling. So we're really focused on developing mobile manipulators that have, yeah, it's quite a broad capability, but very much focused on in certain processes, in certain applications,
Brad Porter (29:22): We've talked about a lot of a diverse array of where robotics can be deployed from manufacturing, eCommerce, logistics, healthcare. Where do you see the most promise from an emerging sector standpoint for robotics?
Mark Segura (29:41): I think everything is telling us that logistics and eCommerce is still the by far, by very far, the largest market in the next five, six, seven years. Because I mean, there are companies pioneering the adoption of robots in certain applications, but the waterfall is really yet to come. Okay. We were talking about all these order picking applications yet it's getting to a mature state, but the mainstream deployment hasn't happened yet. And that is huge. Okay. Only 5% of the warehouses in the world are automated only 5%. So all the reports, all the discussions in the community are pointing at that direction. Then the second biggest growth arena, but that's more of a mid longterm development is healthcare. And that's going to be driven by a perfect storm being built there, which is the talent shortage, the increase of costs and degrees of complexity as we have more treatments and personalized treatment.
Mark Segura (30:37): But that sector has big inertia it's going to take a lot of time. But the need that is so astonishing that it will happen eventually. Okay. And then of course, we see that adoption in retail driven by the eCommerce is happening. And because that barrier now is very blurry. I mean, what is retail? What is eCommerce? I mean, so we see robots in supermarket just because they are offering online drive-through pickup, okay.
Mark Segura (31:05): We see robots in a fashion store because you can buy online and pick up on store makeups. You can have in Korea, we have some robots that the cosmetic company has built a machine where you go and the AI will scan your face. It was recommend you, some colors of makeup you would choose, and our human robot would prepare a customized makeup for you. So customization is also pushing robotics into the consumer segments, quite a lot, and still manufacturing. Of course, there's a big expansion in manufacturing. And the big thing here is how we reach a smaller, medium enterprises to benefit from robotics. That's everyone's challenge in the industry as well.
Brad Porter (31:43): And how do you think about the, when do we have robots in our homes? When, when are the robots helping us day-to-day?
Mark Segura (31:51): Wow. That's big. I think there has to be innovation. That has to be still a lot of innovation. And the maturity of the technology of I mean, ultimate autonomy, but I foresee that there will have to be also some innovation in the business model, because no matter how cost go down, I think an individual, I mean, average family probably will not be able to spend some tens of thousands of dollars into a robot. But if you look now, for example, in the market, there's a robotic dogs. There are two very different approach. There is an American company who's selling that at $70,000 and there is a Chinese company, which is selling it at $1,700, but without software. So they are expecting an open source community to build applications. I don't know what's the right model. I really don't have a... But I do believe that, I mean, the hardware costs will be still such a cost that there has to be a business model innovation for us to have robots at home.
Brad Porter (32:58): What's the coolest thing you've seen lately that you can share?
Mark Segura (33:02): Sorry, what's the cost?
Brad Porter (33:03): What's just the coolest advance you've seen lately that you can share?
Mark Segura (33:09): Wow. I think I brought a lot of examples already. I don't know if I'm missing some, I mean, this Korean example of the makeup was very cool, because again, it was AI for something you would never think about. But I think we're really betting double down, I mean, for maybe based in the hybridization of stationary robots and mobile robots, I think we can do fantastic things and thinking about total different level of flexibility when you're going to have robots doing processes statically in a place, but then your whole design is connected by a flexible material handling flow, the AMRs.
Mark Segura (33:51): Okay, because this is not being explored. And I think we can have redeployable factories. We can have flexible factories can produce totally different things and the level of investment. I mean, the innovation that would be really on the business side, because there are many, many industries where the CapEx needed it takes so long to pay that you take a total different path, but if robot's movement is so flexible that you can evolve and evolve and scale and downscale and so on, that will be a total different picture. And the combination of mobile robotics and stationary robotics for us is the answer. And it's not only about the technology innovation, but it's really about the architecture innovation and the business model innovation. And this is what really we're really be investing more.
Brad Porter (34:39): You've given an amazing kind of perspective on robotics and the evolution and where you think it's going. I think in my kind of final wrap up question, I'll just offer you the opportunity to share anything maybe that you additionally wanted to share and maybe something to kind of inspire and excite our audience about the future of robotics.
Mark Segura (35:00): I think, as I said, robotics, fortunately it has the ability to take any technology development and apply it. And we are in an exciting moment. Acceleration in technology is all over and faster as ever. So robots will have the best batteries to move better and longer. Will have the best cameras and sensing and AI to understand the world, will have the best CPUs to be able to connect to the Cloud. And this connectivity will boost the collective intelligence of robots. So the vision of this robot skills being hosted in a big Cloud where all the robots are learning and improving and can be deployed remotely so that we lower the cost of ownership. We lower the investment. This is really the vision we see for robotics. More intelligent and flexible robot that has a collective intelligence through robot skills that can really scale and bring robotics to benefit many, many more industries and people that it has been in at least my last 23 years.
Brad Porter (36:07): Amazing. Thank you so much for taking the time. Really enjoyed the opportunity to work together at Amazon and hope to continue working together to help your AI needs. So, yeah. Thank you again.
Mark Segura (36:21): Yeah. Thanks a lot, Brad. It's been a pleasure. Thanks a lot for inviting me