The Impact of AI in Financial Services & Beyond With R. Martin Chavez
speaker
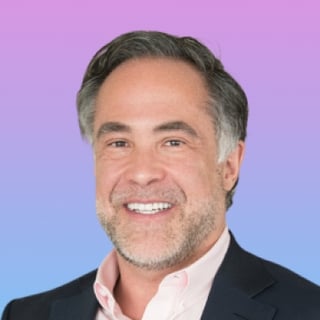
R. Martin (“Marty”) Chavez, Ph.D. is widely renowned as a trailblazer and leader who turned the Wall Street trading business into a software business, revolutionizing the way that capital moves and works. He is a partner and vice chairman of Sixth Street Partners, where he works on research and development; diversity, equity, and inclusion; the sourcing engine; and the More Than Capital business, driving deeper engagement with the portfolio companies. Before joining Sixth Street, Marty served in a variety of senior roles at Goldman Sachs, including Chief Information Officer, where he oversaw the firm’s 9,000 engineers; Chief Financial Officer; and global co-head of the firm’s Securities (now Global Markets) Division. Marty was also a partner and member of the Goldman Sachs management committee.
SUMMARY
R. Martin (“Marty”) Chavez, Ph.D., is Partner and Vice Chairman of Sixth Street Partners and former CEO and former CFO of Goldman Sachs. widely renowned as a trailblazer and leader who turned the Wall Street trading business into a software business, during his time at Goldman Sachs. Marty joins Scale AI CEO Alexandr Wang in a fireside chat to discuss how machine learning is impacting the world of finance and beyond. Together they discuss the 'digital revolution' of Wall Street and financial services which saw a transformation where traditional finance roles worked directly with programmers to build new tools that unlocked new opportunities. Join this session to hear how Financial Services transformed with AI, and how those lessons can be applied to other industries.
TRANSCRIPT
Alexandr Wang (00:23): I'm excited to welcome our next speaker, Marty Chavez. Marty is currently partner and vice chairman at Sixth Street Partners. Before joining Sixth Street, he served a number of senior roles at Goldman Sachs, including CIO, CFO, and global co-head of the firm's global markets division. Welcome Marty. Super excited to have you.
Martin Chavez (00:43): Hey, Alex, it's a pleasure to be here. I dressed up just for you.
Alexandr Wang (00:47): I appreciate that. You know, we're gonna- I think we're gonna talk a lot about, um, some things that are interesting to both of us today. Talk a lot about AI, machine learning, finance, computer science, um, and, and just try- try to see how far we can dabble outside of that. So, uh, originally, when, uh, you, you wanted to actually be a doctor, you know, we've, we've actually talked about this before. However, when you started at Harvard, uh, you were actually studying both biochemical sciences and computer science. Wha- what was it about computers that kind of drew you towards them and, and ultimately drew you towards algorithms and AI and all that stuff?
Martin Chavez (01:21): Actually, Alex, uh, the, uh, origin story is a little bit, a little bit different, in that, I was a total computer geek when I arrived at Harvard and my parents wanted me to be a doctor. (laughs). And So I, uh, I went to the Science Center and all of the professors from the various departments were, were recruiting. And I remember it like it was yesterday. Professor Stephen Harrison, still a professor at Harvard in biochemistry, uh, grabbed me and he said, "Who... What, what are you?" And I said, "I'm a computer scientist." (laughs). And, and he said, "The future of the life sciences is computational."
Martin Chavez (02:02): And that was a nonobvious in 1981. (laughs). And of course, I dated myself. And he said, "If you sign here, you can be a biochem major and I'll make sure that you only have to take one wet lab." I, I think he knew right upfront that I'd be a disaster at the wet lab. "And everything else can be CS and other sciences. Uh, a- a- and the future is gonna be building digital twins of a scientific reality, and let's get started." So that's, that's really it. I was a hardcore biochem, uh, concentrator, but really in the latter part of Harvard, um, went way into theoretical computer science, which has really evolved the things, the one that I most love.
Alexandr Wang (02:47): And so it must be so exciting right now to see kind of the confluence of, uh, of biotechnology and computer science with like the recent advancement in AI, like AlphaFold, with protein-folding from DeepMind. It just must be like super exciting to watch this all kind of come to fruit.
Martin Chavez (03:03): That's incredible. I mean, if you, if you live long enough, you get to be considered a, a, a pioneer, but the, the dark truth of it is that, uh, back in 1985, when I went to Stanford for the MD-PhD program and joined the artificial intelligence and medicine, the SUMEX-AIM project, uh, i- i- it was pretty early into it that we began to realize that the compute power was just way too low to do anything interesting. Uh, I was right in the middle of the first nuclear winter of AI. Lucky me.
Martin Chavez (03:39): Uh, I, I remember in those days, we would- I wouldn't even really say AI, I would sort of mumble it and look down because the term sounded so, so glamorous compared to what we were actually able to do in real life. But again, second half of the chess board, few generations of Moore's Law and look what's happening. It's incredible.
Alexandr Wang (04:02): A lot of people in AI actually still feel that way and they still don't like calling it AI. So, uh, you know, some things-
Martin Chavez (04:07): (laughs).
Alexandr Wang (04:07): ... some things never change.
Martin Chavez (04:08): I say machine learning myself.
Alexandr Wang (04:11): Um, you know, one of the things that, uh, that has always, uh, really struck me about you and, and we've talked about this a bunch of times, is that, you're always really interested, uh, at the intersection of various disciplines. Like you just talked about biochemical engineering and computer science. Um, we're gonna talk later on about, uh, financial services and computer science. So what is it that, that kind of draws you to multiple fields? Uh, whereas a lot of people pick careers where they're very single-tracked and sort of very focused on individual fields.
Martin Chavez (04:39): Yeah, that's a great question. I, I, everything about me is, is, is intersectional. And like you can iner- interpret that in a bunch of ways. One of them is that, uh, that's where all the excitement is, but, but here's another one. I, I remember, uh, a little town hall I gave when I became chief information officer of Goldman Sachs, little town hall, 10,000 people. And I, uh, I said, um, "I'm not the best mathematician in the house and I'm not the best software engineer, nor the best software architect, but, um, I'm pretty good at all of those things, and I can write a solid English paragraph, therefore I am your leader."
Alexandr Wang (05:21): (laughs).
Martin Chavez (05:27): (laughs). It ha- it's, it's been great for me to be pretty good at a bunch of things and, and not nearly the best in any one thing.
Alexandr Wang (05:33): No, but I, I just remember like, uh, when I, when I visited your place in New York where, uh, um, you were excitedly telling me that the, the curve of your staircase was a paraboloid. And that was a- that's a very iconic, uh, Marty, as in to me. Um-
Martin Chavez (05:48): (laughs). Not just any paraboloid, uh, a hyperbolic one. (laughs).
Alexandr Wang (05:53): There you go. There you go. Um, so, you know, let's, uh, let's kind of talk about Wall Street for a little bit, obviously where you spent, uh, a good amount of your career. And, and, you know, one thing that I'm, that I'm interested to learn about is like, what- share a little bit about what you originally found on Wall Street, kind of when you arrived and, and how you realized over time that that kind of change was needed?
Martin Chavez (06:17): Well, so, a story I love to tell. I'll tell the short version, is, is how, how Wall Street found me. So I a- am just about the only Wall Street person from the, the class of 85, and that's because everybody went to Wall Street and then the 87 crash happened and most people went and did something else. Um, but in 87 I was, I was, uh, dissecting cadavers in, uh, in med school, and, uh, really had nothing to do with the stock market crash. And hadn't thought about Wall Street at all. But as I mentioned, I was in this AI in medicine program, a joint venture of CS and medicine at Stanford, and the compute power was low.
Martin Chavez (06:58): And we could do interesting things with what were essentially toy problems. And, and we were all pretty, pretty despondent. Um, one of my colleagues who- with whom I shared a cubicle for a period, uh, had the, had the excellent idea of using computers to help people figure out what movie to watch. Um, and that of course is, is, (laughs) [inaudible 00:07:21], and so, by far the more successful. And I, I did not figure out an interesting problem that was actually tractable. Uh, but if you get a letter from a head hunter saying, uh, "Goldman Sachs is looking for entrepreneurs in Silicon Valley with PhDs in computer science from Stanford."
Martin Chavez (07:39): The- and the days before LinkedIn, he'd just done the intersection. And so I went out there more as a joke, to be honest, more for a free trip, uh, to New York, see some Broadway shows, have some fun. Um, and then they were telling me about this project, which I thought was certifiably crazy. Um, the project was to build a piece of software that would contain all the trades, all the time series, all the models, all the reports, all the risk and put it all in one place. And so of course, to do that, we need to build our own transactionally-protected, object-oriented database. What 20 years later, you might call DynamoDB.
Martin Chavez (08:21): And of course, we need our own programming language that's interactive and scripted and dynamically-typed, which 20 years later, uh, it would have been Python. (laughs). "And we're gonna build it ourselves, in C. And your job is to write the database." And, um, I thought, "Well, I'm gonna do it for a year, see what it's like. Uh, and it sounds like fun. It sounds like a hard problem." And they clearly had the need, the pain and the ability to pay and the desire to pay. And that was a bad time in Silicon Valley. 93 was another dark time in Silicon Valley. So I took the bait thinking it'd be one or two years, pay off some student debt, and, uh, and then go back to Silicon Valley. And I ended up staying for a, for a real long time.
Alexandr Wang (09:06): There you go. There you go. And, and like, this thing that you just mentioned, even like, I think it's, it's a bit hard to even comprehend how, um, how visionary it was, especially at that time to say, "Hey, we're gonna have one software platform that's gonna have all the information, all the data in one place, uh, and we're gonna be able to use that tech to sort of...." I mean, I know of plenty of financial institutions that even today, don't have that, or didn't have that sort of clarity of vision early on.
Alexandr Wang (09:33): And so, what do you think is... You know, um, for- in, in terms of like, uh, what some of the, the things that, that were holding- that like this technology solve for the, for the financial institutions, like what, what was- how were they being held back by lack of technology? Was it resulting in slower trading decisions, missed opportunities? Like, what was the- what was that kind of impact?
Martin Chavez (09:54): So, great question. I, I wish I could say that I was the brilliant person who, who, who dreamed up this, this vision at Goldman Sachs. I, I was not, I was the, I was the fourth person to join the core team to actually make it real, to, to, to build it. Um, but, but I think this is very, very important to understand, and people forget this. I know with certainty that the secret at Goldman Sachs was organizational and cultural, more than anything else. It wasn't just having great people, other banks have great people too. Many of our great people went to other banks who, who CEOs came to them and said, "Hey, would you please do that Goldman Sachs thing, that SecDB thing?"
Martin Chavez (10:43): And so they called the project to hear at our bank. And, and none of it, none of it worked to the same degree at all, as it did at Goldman. And so, so really, here's another creation story. The traders in the Jay Aron currency and commodity business, which Goldman had bought a few years earlier were having a, a very unpleasant and painful interaction with IT, the technology division, where the traders would say, "Please build a risk management system." And then it would go off and spend a bunch of money in maybe a year, and then they unveil it, and the traders would be very unhappy and they would express themselves, (laughs), in a very loud and unhappy way.
Martin Chavez (11:26): And this unpleasant loop continued, endlessly, until one of the traders said, "I am going to go across the river where you find engineers, and I'm going to go to the, the heart of where the engineers are, Bell labs. And I'm going to get my own engineer, and I'm going to sit him next to me and I'm going to pay him well. And maybe he will learn the trading business by osmosis. So I won't have to explain it because I obviously haven't been able to explain it to any of these IT people. And maybe he'll figure out what to build."
Martin Chavez (12:03): So that's what Loyd Brightfine did. He hired Armand Evanescence from Bell Labs. And, Armand, was the one who had this brainwave. We're gonna build one piece of software and, and, and why did Armand get there? Efficiency, outcomes raiser. And the big problem I'm having right now is whenever I want to find something, there's 117 different databases and disconnected systems that I might be in, and I want to put everything into one place where everything is, so I will always know where to find it. (laughs). And, and it was really just that. And then the rest of us worked on making it real, over 25 years. It was a long, long haul.
Martin Chavez (12:45): And even in the early days, I remember we would say, "And one day, we're going to have the equities business modeled up in our system." And then we'd laugh hysterically, because we didn't know the equities people, they were in a different building. There was a different team. They wouldn't have liked us if they had known us and they wouldn't have had anything to do with us. But even in the early days, we had this strong conviction that there was something really powerful about building a high fidelity digital twin of our trading business.
Martin Chavez (13:20): And we would start with the course granularity, not high fidelity. And then we just keep working until all the corner risks and all the edge cases and all the flows were part of our system. And we had that conviction because we said "Hey, wouldn't it be great if you can lose billions of dollars every second of every day in software simulation, and then wake up and say, 'Thank God that didn't actually happen. It was only simulation. It was only a counterfactual.'" And in those counterfactuals, you would find the source of your action, the way to mitigate the risk. Later on, this worked out so well that we encountered a risk we'd never expected, which was that people would accuse us of having seen the future or having created the future.
Martin Chavez (14:13): Um, that happened around the time of the financial crisis. Um, I, you know, I wish I could see the future. Instead, as we like to say, we were excellent observers of- and predictors of the present. Unlike every other bank, we had our present modeled up in a way that we could then ask all these what-if questions and be ready for all those things, if and when they did happen, that's the essence of risk management. And this was a virtuous cycle that worked amazing. And I would say, I would describe myself as just the most junior person who, who rode the success of this platform and this vision, um, into the executive office.
Alexandr Wang (14:58): No, but it's so, it's so, um, it's so visionary and it's so im- important in that, like just the exact same things that we're talking about. With the b- biochemical industry, it's like when you get to a point where, uh, all of your data can be in one place and used to, to basically serve as a sort of platform or foundation to fuel all sorts of algorithmic efforts, AI efforts, simulation efforts, digital twin efforts, and, and basically power all these, uh, these sort of innovative things that everybody wants to talk about, um, but, uh, but you know very well that you kind of had to go through this like slog of like actually building this beautiful data platform to kind of get there. Um, and that was, uh, that was, I don't, it sounds like 99% of the work. Um-
Martin Chavez (15:39): Oh, absolutely, abs... I mean, we- I realized early on that the wiz-bang analytics, if they were not harnessed inside the workflow and used to make decisions, it was a complete waste of time. Why bother? And also, I observed over time that the people who could conceive of this end-to-end digital model and make it real with this heavy emphasis on the unglamorous part, which is the cleaning and the curating and the storage of data and the capturing of all workflows in software, uh, uh, those were the people who really got rewarded a- and actually the people with the hardcore specialist skills in those days, stochastic, uh, calculus, for instance, for derivatives modeling ended up being ec- eclipsed by the, the data engineers.
Alexandr Wang (16:30): Yeah. You- you know, you, you, you did this really wonderful Stanford class. I'm going to ask you, uh, in a bit later on to give your, to give your one-liner, 'cause I think it's so great. But, um- but, uh, one of the things, and it talks about the, the confluence of technology and financial services. And, um, one of the questions I have for you is like, how have you seen the financial services industry transform, uh, during your time on Wall Street?
Martin Chavez (16:55): Well, (laughs), let's see. I, I, uh, I remember the day my boss said, "The oil strategist has resigned. Uh, congratulations. You are the new oil strategist. Go out to the trading desk. (laughs). That, that corner on the oil trading desk, where they throw phones into screens and are really loud. Go there and, uh, introduce yourself, (laughs), and make yourself useful." And, uh, well, right. Uh, guys like me, "I don't know. Can you help me turn my computer on?" That was, (laughs), that was- that was the interruption.
Martin Chavez (17:30): And, uh, and I very early on, um, you know, I've al- I've always thought about the use case, the, the pain point, who pays what to whom? That was my early lesson in Silicon Valley. So, so I, I love dreaming up stuff. I get really anchored by, "How, how am I going to get paid for this?" And, uh, that turned out to be a great survival skill on Wall Street. Also, growing up as a gay kid in Albuquerque, New Mexico, I, uh, had another survival skill, which was, identify the beautiful and popular people and help them with their math homework.
Martin Chavez (18:08): So I was really made, made for Wall Street. (laughs). I identified the popular people and, and helped them with their math homework. And, uh, and I- I said to the trader, "Here's what I'm going to do for you. I- I'm gonna get you out of here at five o'clock every day, half an hour after the market close. And you will have perfect risk and P&L reports." And for him, he was wrangling Excel for, for several hours at night to maybe get the right risk for the next trading day. And I said, "No I'm going to make all that go away and it's gonna be, you, you push one button. You, you gotta tell me when all the trades are in, and I'm going to do the rest."
Martin Chavez (18:50): We thought that was an amazing deal. And so that's how I made myself indispensable. Now, um, even back then, I started thinking, "Okay, so I'm doing all these analytics and the analytics are saying that, 'If you've got one second to hedge the entire derivatives book, go buy this many futures contracts.'" And I remember thinking, "Why do we, why do we need the trailer to- the trader to call the broker and say, 'I want to buy 500 for a month WTI?'" I- I'll, I'll get to that part too actually. (laughs). And, uh, but I didn't tell the traders that, that took, that took another decade and half.
Alexandr Wang (19:32): Um, and then, you know, o- one thing that I'm always curious to get your views on since you're, uh, you're, as one of the few people who has a very deep understanding of the technology, as well as, um, the, the financial, uh, lens. You know, where do you think Wall Street is kind of today in terms of a, uh, progress with technology and, and fully in bringing technology into, um, into how it operates? And in particular, wh- where do you think there still remain gaps, you know, with, in terms of the use of data or the mindset and the culture, or the talent, or even just the, the use of technology? Like, where do you think, where do you think we are? Where do you think the opportunities are?
Martin Chavez (20:09): So, so first, I would... Goldman Sachs is a very special and wonderful place. It is hardly representatives, right? So Goldman made this bet 30 years ago to bring in people like me and, and compensate them at a level that had been unheard of before. And to, to think of them as something- to think of some of them beyond IT, right, not just back office. People can help us make money and avoid losing money. And so a lot of things happened at Goldman that are taking- that are happening now at other places, but it's really taken decades. I think, uh, I think Goldman was that far ahead.
Martin Chavez (21:05): And so I would say, the industry as a whole, financial services, is a huge industry and it's incredibly fragmented, right? So you c- so Wall Street, and like, what does it even mean? There's so much happening in Wall Street and it's like that, that cliche, right. That the future's already here, it's just not evenly distributed. So the future's here on Wall Street. There are places on Wall Street where the- you, you can find some of the best computer scientists and engineers in the world doing the most extremely innovative R- R&D, uh, that you can imagine, but that is far from uniform across Wall Street.
Martin Chavez (21:50): And it's going to take decades for it to, uh, to permeate the rest of the industry, um, i- in the sell side where you tend to have to provide... The whole point is to provide liquidity to clients. They, they have a risk they don't want, they want a risk they don't have, you make it happen for them. It's not a surprise that the sell side has, has evolved more rapidly. Many parts of the, the buy side, almost all of it are still in Excel and cutting and pasting and PDFs and email and voice. And a lot of expensively educated people participating in ever prone, manual workflows. That's, that's the state of it.
Martin Chavez (22:35): So there is so much opportunity, uh, to, to, to clean up the workflow. I think, uh, o- one thing that I've... So a couple of things that I've said in the course, the Stanford course you mentioned, um, that got a fair bit of play and were intended to be provocative. One of them is, the future of fintech is banks. And, you know, people say, "Well, what do you- what do you mean by that? You think that the banks are gonna win?" No, no, it's just, banks have been making financial software for many decades now. And so it's the term fintech which I don't love. That's, that's new. Right.
Martin Chavez (23:15): And banks that aren't really great at making software and automating their workflows and consuming APIs produced by others, they're going to be roadkill. And there are activities relating to money, the storing of money, that, (laughs), you know, I, I wouldn't recommend to any fintech that, "Store money and pretend not to, and hope the regulators avoid looking at it because they're calling it fintech, not bank." I think that is a very bad strategy. (laughs). Right.
Martin Chavez (23:51): And so the, the whole point of my Stanford class was, we're seeing a great, uh, bifurcation happening right now, where the distinctions that we used to use on Wall Street, buy side, sell side, trader, customer, quant, salesperson, market data provider and market data consumer, market infrastructure provider, market infrastructure consumer, all that's going away, and there's an API boundary. And if you wish to survive, you better get really good at packaging your products and services in an API, and you better be a world-class producer of some set of APIs that are connected to what is core and differentiating about your business.
Martin Chavez (24:38): And you better be a really astute consumer of APIs provided by others for everything else, because it's silly to build all your own stuff. There's no way that's going to work. And the regulatory boundary will equal the API boundary. And we're seeing it happen now. I can give you a long list of examples, but there are companies that don't ever wish to be regulated by the financial regulators. And I get that. And so they want to be able to say, "Oh no, I just called this API provided by that bank. You- you better go regulate that. It was just a black box for me."
Martin Chavez (25:21): And then similarly, banks are understanding that their core value is to operate effectively and efficiently inside the regulatory boundary and largely leave activities outside the regulatory boundary to others who will be much better at it. The bank will never have the CAC of some of these tech companies and so let them do the customer acquisition. And then the banks will do the core, core things, like storing money, risk transformation, um, things that... You- you want your bank to be regulated, um, if you want- and you want to get the services from a regulated entity and you want to do it in the most efficient way, and you want to put those entities in competent- competition with one another.
Martin Chavez (26:07): And you want them to be API-compatible so that you can switch if one of them stops performing the service you want to the SLA you want. So that's, that's the way it's evolving, and it's evolving fast.
Alexandr Wang (26:18): Yeah. Well, well, you mentioned, it's very, um, it's very insightful. This, this thing that you mentioned around how it's, it's based in evolu- almost an unbundling of the bank. Because, you know, sort of the, uh, the past few decades have kind of been all about banks doing more and more and more and more, you know, becoming more and more full service, having more and more products and services and, and sort of being these like big lumbering companies. And then what you're saying is, the trend is almost the opposite, unbundling, where, hey, the, the, the core regulatory functions, which is the- or the, the core things that you get from being a bank, you know, those are your differentiators.
Alexandr Wang (26:53): Those are the things that like, banks jobs differentiate on. And then the, whether it be a tech company or a marketing company or whatever it is, like other companies will, uh, will sort of, uh, consume those APIs, and so kind of handle the rest of the, rest of the, the work. And it's a, it's hu- I mean, it'd be a huge shift from the current ecosystem. And it's sort of, as you mentioned, like happening in real time as we speak.
Martin Chavez (27:17): It's, it's very clear that it's happening. I could give you, uh, many examples. Um, my, my, my favorite, which my consultant only say a little bit about at the high level is of course one from, from Goldman Sachs. It's the, it's the Apple credit card. The Apple credit card, um, just look at it, it's got only three logos on it. Apple obviously. Do you happen to know the other two of those?
Alexandr Wang (27:42): Um, Goldman Sachs and, uh, I think it's a Visa Card, right?
Martin Chavez (27:47): Mastered.
Alexandr Wang (27:47): Mastercard? My bad.
Martin Chavez (27:50): B- but I, I th- I think you can understand the future of the financial system by looking at that card. (laughs). Right. And so Mastercard is the orchestration layer. Apple is the marketing customer acquisition distribution layer. And Goldman Sachs is the risk transformation, risk storage lab.
Alexandr Wang (28:15): Yeah. it's, it's-
Martin Chavez (28:17): [crosstalk 00:28:17].
Alexandr Wang (28:18): Yeah. It's- that's actually a really beautiful- one of the most beautiful illustrations, I think that, uh, that's out there. You know, one question, um, I have for you is like, so given this context, right, given how we think the future of, uh, uh, how this future will evolve, what do you think is the... Imagine a, a future best-in-class bank or a financial institution, and, uh, how are they using technology data and AI? Like, what are, what are... How do they do that and how do they use those to differentiate against their competitors?
Martin Chavez (28:51): Well, so there's, (laughs), there's so much that can be done, that, that isn't being done. And we touched on it before, but it really just have to make the point again. Without tight integration into the workflow and data of the firm, it's a waste of time to implement the latest AI tech. So I'll just, I'll just start there. And, and people get this wrong all the time, right? And it's a failure mode. Let me, let- let me acquire painfully, inexpensively, some machine learning engineers, and maybe they will sprinkle machine learning everywhere and we'll be good. No, no, no, (laughs). You can't retain those people. You can't find them.
Martin Chavez (29:38): And you, you- arguably you don't even actually want them. Right. You, you, you probably want an API provided by someone like you, Alex, who is able to, to concentrate these people, uh, a- a- and this talent. Um, and then, here's another thing I would just say, more as a throwaway remark. I think the idea that AI is going to predict the future market price, if anything, I think that was a silly idea. Personally, I spend no time on it. Um, to me, it's pretty easy to see this, right, the techniques we have, and please disagree, um really work if you've got a stationary distribution. My favorite example is, is, is cats. Right.
Martin Chavez (30:26): Cats evolve slowly in evolutionary time, but right now, you can get a billion pictures and they've been labeled cat or not cat. All right. That's, that's great. If, if you can do a problem that way, if you can dichotomize it, label it that way, then AI is gonna win every time. But market prices are not that. And so we'll have to come up with something else, or maybe we will never, more likely, I think, ever be able to predict market prices, which is the first thing everybody thinks about when they think about finance, but where all the action is, is everywhere else. I mean, I could go on all day about the things that you, that you can do with AI.
Martin Chavez (31:09): So, so commercial banking use cases are, um, customer development. What's the right conversation to have with each customer to foster loyalty, or like another classic, preventing churn, identifying early signs of customer dissatisfaction, anti-money laundering, uh, identifying false positive alerts- alerts to get more effective and efficient and, and allocate resources to the right place. Um, recoveries, um, in, in, in, in, in charge-offs, there's a lot that can be done, uh, with AI there. And this is very fruitful. And I would say that there's a huge, still unrealized opportunity that I'm sure AI can help with.
Martin Chavez (31:51): If, if someone could provide an API that will just tell me, is this transaction fraudulent, or does it comply with all my anti- anti-laundering- money laundering, and then your client rules? That's a Goldmine, and it doesn't exist. It's a super hard problem. And I know AI techniques are gonna be super, super important there. And then on the sell side where I come from, um, I've, I've seen so many exciting applications. He- here- here's, here's one, back to my earlier point about not predicting prices, and I don't think that's really gonna happen. One of my colleagues loves talking about how he used some early mach- machine learning techniques, uh, to come up with, um, some ways to trade, uh, the dollar Mark Cross.
Martin Chavez (32:40): So this, the date said it's a little bit early, and you know, calculated all night, and it came up with medium term trend followers, which we already had, right? So, so sometimes the AI just recapitulates things we already had, and maybe doesn't discover something brand new, but here's- here... There are a couple of examples that blew me away. Um, uh, here's one of my favorites. Um, this, this is for derivatives geeks. So bear with me, but, um, pricing options, pricing puts in calls. So there's a legendary formula called the Black-Scholes, uh, formula, which is a closed-form analytics solution, to option prices under some really strict abstract theoretical conditions that never actually in real life, like constant volatility, log normal, underlying distribution, and on and on.
Martin Chavez (33:34): But it's a beautiful formula. So what have people done on Wall Street for decades? Tweaking Black-Scholes, hacking it in all kinds of beautiful and ugly ways, torturing it to get back to the option prices that actually make sense so that you can hedge and manufacturer, uh, the option. Well, one enterprising strat said, "You know what, this stuff is painful. Why don't I just train up a model on all the options prices that we've ever made? I just skip all this hackery." And this, this model, this model was unbelievable. (laughs). It recapitulated at all prices, it wasn't overfit- fitted. Um, and it gave better, this, I think, was shocking.
Martin Chavez (34:22): It gave the gradients for free. The legendary Greeks, or, or hedging parameters on Wall Street. And they were much smoother than any of the Greeks that we'd ever had before with all of our hacking techniques. They were just better. Right. So, so that's a real commercial financial example of something that machine learning, uh, can actually just nail. And then another example that machine learning, AI, has nailed is a very hard problem. If you are a market maker in stocks, you end up owning a lot of stocks that your customers gave you. And then the question is, well, what do I do? Like, like, I'm not just gonna sit on these blocks of stock, right.
Martin Chavez (35:08): That, that would be a very bad thing, and that's incredibly risky. And who knows what will happen? But neither can I just sell them all, because that will dislocate the market and then I'll really get stuck. And so what do I do? How long do I hold these? What do I hedge them with? How do I predict the arrival of new customers who will actually want to buy that from me so I don't have to sell it into the dealer market? This is incredibly hard problem, and another one that, that, that admits to a beautiful solution, uh, with machine learning techniques. So there's a, there's a lot going on. I think a lot of wasted energy on predicting the future of some, some asset.
Martin Chavez (35:54): And I would rather see all the effort go into cleaning the data, harnessing the workflow and these kinds of applications, uh, that I described rather than the, the pipe dream of predicting the future price of some stock.
Alexandr Wang (36:08): Totally. A- and you m- you mentioned something that, I mean, we just see all the time with, with our customers, which is that, you know, without data and without the proper data foundations, there really is no AI. That was kind of your first, um, your first statement. And then, uh, and then obviously, I mean, I think, uh, uh, we've had this conversation, but I agree with you, like predicting the future asset price, you know, is, is hard because there's, you know, the distribution's changing, the world's changing and it's a competitive process. Whereas, all this other stuff of just like having your business operate more efficiently and effectively, um, using AI, that's where there's a lot of very real, very real opportunity.
Martin Chavez (36:45): And I didn't- Alex, I didn't even mention some of the things that we're seeing in NLP these days, that are mind-blowing, and again, would be revolutionary for finance, right. Here's, here's one way of thinking of every financial services company. It's got a million legal docs that are generally in some filing cabinet somewhere, that no one's ever seen, and maybe there's an electronic copy. And those docs are meant to govern every aspect of its business. I've agreed to do all of these things with all of these clients. And, and many times, in regular- regular way business, when the sun is shining, you don't even look at the docs.
Martin Chavez (37:30): But I can tell you when there's a financial crisis, everybody's pulling those docs to see what's gonna happen because nobody knows what's in them. And, and just querying a million docs to know what credit terms did I grant. Right. That's, that's an incredibly hard problem. And we're starting to see NLP solutions, um, that actually let you ask these questions about a vast copies of documents. And I think that one's gonna be huge.
Alexandr Wang (38:01): Yeah, those are massive. You know, I, I think I already know your answer of this next question, but, um, you know, the next q- the question I think is on, on many people's minds is, how do you get to this future where, um, you know, data and AI are being used across the business? And, and I think that, you know... I'll, I'll go first and then you tell me how- uh, you add onto it, but it's like, first, you gotta be very focused on the data. So, so acknowledge that, hey, you're gonna be spending years and years working on the data foundation to be able to like actually set you up to succeed in the future.
Alexandr Wang (38:32): And then, you have to, you have to take a cultural approach, which is really, uh, embrace and, and, um, empower the technical people within the company who are gonna actually be able to design these solutions for you. But, but what else, uh- w- what am I missing? What else would you add?
Martin Chavez (38:48): Okay. I agree with you 100%, but I would add to it something that is so important, and it's much more than an implementation detail. Okay. Which is, you mentioned bringing in people with these different skill sets, these data science skills, let's just call it that. Y- yes, y- you have to do that. But how do you then set these people up to succeed? And this is a place where I see all kinds of companies in this financial sector and having nothing to do with the financial sector make a, make a big mistake. Okay. So here's, here's a, here's a failure mode. Data science center of excellence. Whenever I hear that, I just want to bury my head in my ass. (laughs). Right.
Martin Chavez (39:43): Like, that's, that's what they talk about. That's like, get a few people and then sprinkle their magic over the company, and then some amazing thing will happen, no. That is a complete, complete waste of time. So the... I, I see two approaches and, and every company will need both. And just what matters is the degree. Okay. The first, the first is an evolution that we went through at Goldman Sachs over 25 years. When I joined, we said to ourselves, "The only thing crazier than making all your own software is not making all your own software." And then we laughed and we patted ourselves on the shoulder and we thought that was very clever. And we went and made our own software.
Martin Chavez (40:26): That was great, in 1993. That gave us an edge. The firm was willing to invest heavily in the kind of people who could do that to, to a world-class standard. We probably held onto that way too long. And so somewhere around the time, I became chief information, officer, 2013. We changed it and we said, "There's gonna be new, new waterfall. And it is download, build, buy." And what I meant by that is, get it in open source, download it or participate in the open source community. "But if you're sure that's not gonna work, then we'll build it. We'll still be better at building it, and only buy software as a last resort."
Martin Chavez (41:15): Couple of years in, we flipped the last two obviously, and we said, "So we're gonna download first and then after that, we're going to buy it, but we're going to want, and we're going to demand, and we'll use every inch of whatever cloud we've got to insist on standard APIs. And we would many times actually insist in our contracts, "You can't sell us your proprietary add-ons." Because we wanted the ability to switch. That was the only power we had. And then as a last resort, if none of those are gonna work, we're going to go build it. And so I recommend that waterfall to nearly everybody. And, and I think the idea that you're going to build all of the capabilities inside your company, I just don't think it's gonna work.
Martin Chavez (42:06): I don't think anybody's gonna be able to afford it or keep up with the latest and greatest. They're gonna want to consume the latest and greatest through APIs. And the last point I would make, and this was, this was a key, there's a special sauce at Goldman. You've got to take people with the data science skill set and put them next to whoever your professionals are. In one business, it might be doctors or medical scientists and another business, it might be salespeople, and yet another it could be bankers, or it could be traders, or it could be portfolio managers. And they need to work side by side as equal partners in the business.
Martin Chavez (42:48): But if you set up the dynamic that, "Oh, this, this person is gonna turn on my computer and, and make some cool software for me, and I'm the boss and I rock. And this person is my, uh, uh, subservient entity." That is a terrible failure mode. So something that Goldman did that was brilliant was to actually, for the first time, take people like me and welcome us onto the trading desk, as partners in the business, not as service providers to the business. And so whenever I find data scientists say things like, "The business wants X. The business needs Y." I'll look at them and I'll say, "What are you? If, if you're not the business, then why am I even talking to you?" Right. Data scientists as partners in the b- business, hugely important.
Alexandr Wang (43:45): You know, one, one thing that I'm, I'm curious, uh, to, to get your thoughts on as well, you know, one thing that, a- and this plays off of this idea that we're talking about where the API boundaries are where, th- that's, where the, the regulatory boundaries, or the API boundaries are gonna be over time. You know, one of the things I think is interesting is, is, um, where does, where does machine learning, AI, sit with respect to that- to- with respect to that boundary? And I think the answer is on both sides, but I think that, you know, one question is, uh, obviously there's been a lot of growth in the fintech sector recently.
Alexandr Wang (44:20): There's been a lot of fintech powerhouses created recently. And, uh, I think a lot of traditional banks are trying to figure out, "Okay, how do we, how do we cope with this? How do we compete with them? And then what is, what is the role of... Like, how do we build our AI strategies when many of the fintechs are also quite capable at AI and they have the ability to hire talent that's great at AI and, and do things that are... And they have a different architecture than us because they don't have all this baggage from the past, et cetera, et cetera. One thing that I think is super interesting about where we are right now is, uh, you know, obviously there's a lot of fintech powerhouses.
Alexandr Wang (44:51): And we kind of talked a little bit about how, you know, uh, over time, the regulatory boundaries, or the API boundaries are gonna live. And there's a question of, where does AI and machine learning stand? Is it on both sides of that API boundary? And in particular, you know, um, for most traditional financial institutions, as they're thinking about this, you know, there are these fintechs who have potentially the ability to- they also have the ability to AI, they have potentially better data architectures, they have the ability to move faster, ability to hire different talent. And so how do you think that a traditional financial institution should be thinking about this and, and what, what they need to be doing to sort of remain competitive?
Martin Chavez (45:31): So the answer's, clearly, there's gonna be AI happening on both sides of that boundary. And it also goes back to what I mentioned before, which is, what are the core banking services in some, in some jurisdiction? And one of them will be, well, holding deposits that are insured. That's a core activity. Right. (laughs). And another one is, originating a loan and holding it on my balance sheet for 30 days, so that now that we're on a season, they can later be repackaged in other ways. That has to be done by a bank. There are number of things that aren't subject to the baking regulation.
Martin Chavez (46:09): And so I would identify those things and get really, really, really good at them and offer them in, in eight lovely API wrappers to absolutely all comers, so that it would make no sense for anybody to attempt to do this on their own. They would be taking inappropriate regulatory risk and they won't be good at it. And that's the strategy for banks. Now, banks will also have particular expertise in some regions or some geographies or particular sets of customers. And so that's an interesting portfolio of, uh, of assets. Right. I've got my customer relationships and I got this core capability, um, to, to offer banking services, uh, that's under a regulatory umbrella.
Martin Chavez (46:56): And then everything else I would want to consume from other people in the form of APIs and distribute my core capabilities through APIs. I just don't think banks are gonna be competitive in anything else. And I also think that fintechs, um, will be prohibited from playing on that side of the boundary, unless they wish to have a banking charter, um, which you can just go buy. You have to then get all of the activities you want to do with your charter approved. And, and I would say, Well, just don't do that. Just get them an API form from somebody else. And, and I think the way I'd close on that is to say, look at Apple and, and Goldman Sachs. Um-
Alexandr Wang (47:39): Yeah, that was part of, it's-
Martin Chavez (47:42): (laughs). I would pay attention to that model 'cause that's where it's going.
Alexandr Wang (47:44): Well, it's a wonderful illustration. You know, just, to kind of, um, to kind of close at the end here, I want to switch gears a little bit. And, um, you know, uh, you've described, uh, even earlier in this interview, how, you know, diversity of your own background, uh, and your own identity was, was in many ways a boost to your own career. And, and I think you've spoken in the past about how, um, speaking Spanish was, uh, itself an edge. And you know, I also grew up in, in New Mexico, but I, I unfortunately never spoke Spanish. And so, (laughs), maybe I, maybe I missed out on that edge. But, but so w- why do you think it's, it's really vital to foster diversity, uh, within, uh, within the world?
Martin Chavez (48:22): Well, here's an analogy. So something that everybody in finance understands is the benefit of portfolio diversification. For the same amount of risk, you can have higher revenues, or you could have the same revenues with lower risk just by diversifying your portfolio. The absence... Same thing happens by diversifying your people. You get uncorrelated views, it's, it's another free lunch. And it's the only other free lunch that I know about on Wall Street, is these two kinds of diversity or diversification, if you will. This is something I give Goldman Sachs a huge amount of credit for.
Martin Chavez (49:07): Um, not only welcoming diverse people, like, but, but proactively finding them. Right. So someone had the idea, "Let's go to Stanford and get some computer geek who's been working in medicine and, and bring him here. And he knows nothing about finance and that's just fine. We will teach him the finance and then he'll teach us some other things. 'What will he teach us? We don't know.' Right. But if we knew, we wouldn't have to be running this experiment, right, by definition. We don't know what we don't know, and the only way we're gonna surf this, what we don't know is by bringing in, bringing in these people." Then once Goldman brings in a very diverse group of people, does something else interesting. And I was the beneficiary of this.
Martin Chavez (49:56): So I remember when I returned to Goldman after an entrepreneurial stint, I was asked, "Well, we really missed you in the commodities business." And I remember saying, "I'm, I'm a commodities burnout. I'll work on any business except commodities." In my second tour at Goldman, I got my wish. I worked on absolutely every business, just about, except, except commodities. And I was always the guy, when, when someone said, "Hey, Marty, would you like to go into business X?" I never said, "Oh, I have no idea what I'll do in that business. I don't understand that business. Is this good for my career or bad for my career?"
Martin Chavez (50:35): I was the guy who didn't torture anybody with those questions. I just said, "Yes." And, and I figured, "Hey, there's a chance I'll go over there, and there's absolutely nothing for me to do. But there's also a chance that I'll go over there, and just because I came from New Mexico and I went to Stanford and I studied AI and theoretical CS and I speak Spanish and, and I do all these other weird things, ma- maybe just because I worked in commodities and I've never worked in equities before, I'll think of something that nobody has thought of, no particular brilliance."
Martin Chavez (51:13): For me, it's obvious, it just- it came from another part of my reality, but nobody had ever welcomed that reality into this reality. So that worked out unbelievably well. The fact that I came into the equities business and hadn't gotten the memo and all the things that couldn't be done, turned out to be a very rich source of happy customers and growing businesses. And, and I learned a lot. So, and closing, I would recommend that, uh, diversity play to absolutely everybody. It is not just altruism, it's also good for business.
Alexandr Wang (51:50): Yeah. And it's so important, uh, especially in the AI community for us to be thinking about this because, uh, it's very critical that as we build the systems of the future and the AI system of the future, that we build so with, uh, with a diverse group of people and diverse community. So very relevant, uh, when you were starting your career, very relevant today and will be relevant in the future, for sure.
Martin Chavez (52:11): Always a pleasure, Alex, great talking with you.
Alexandr Wang (52:14): Thank you so much, Marty. This was a lot of fun, uh, a lot of gems of wisdom, uh, that we, we covered on today. And, um, uh, always, uh, always such a, such a fun time chatting with you.
Martin Chavez (52:25): Thank you for inviting me. Be well.