Articles
December 14, 2021
Why Building AI with Empathy Matters
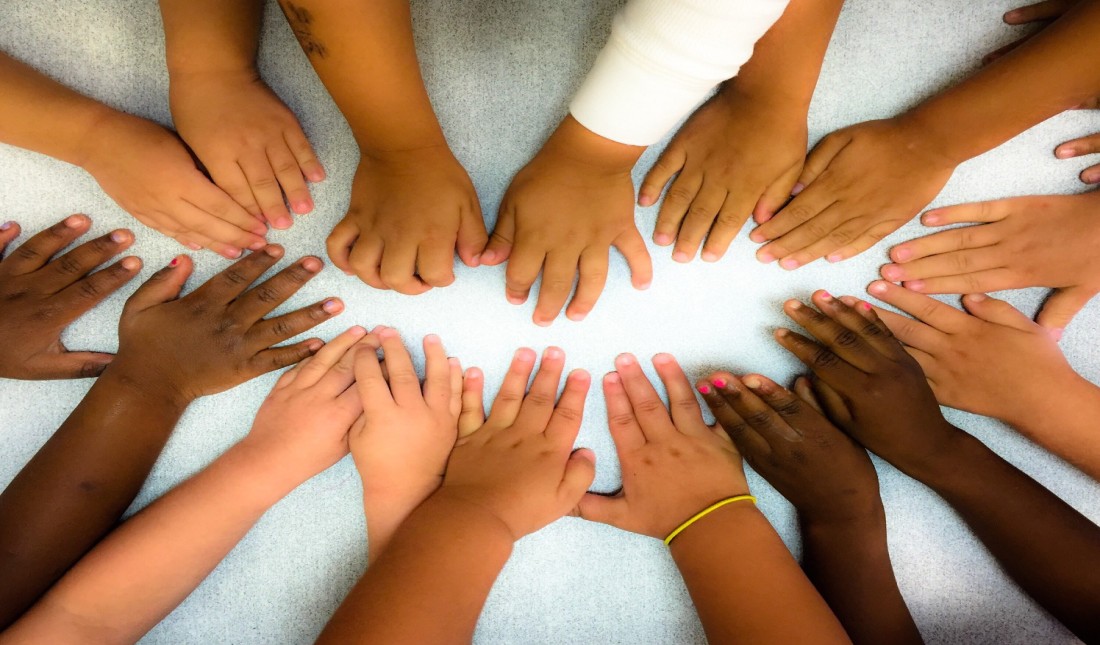
Large-scale, globally diverse data is needed to train models correctly and overcome the Al alignment-problem, says Alan Cowen, chief scientist and CEO of Hume AI

Esther Shein
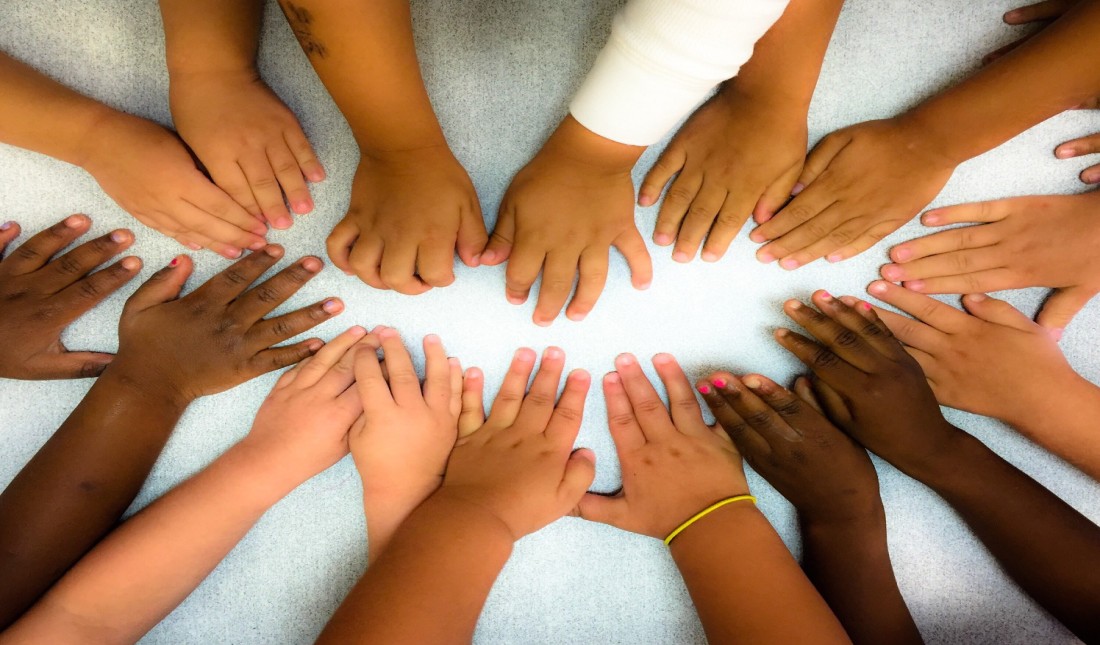
AI built with empathy is essential not only for solving a wide range of problems but also for ensuring that the methods used to solve them are aligned with human well-being.
Alan Cowen, chief scientist and CEO of Hume AI, discussed what he called “the AI alignment problem” during his recent presentation at the Scale TransformX 2021 conference, “How to Build Technology with Empathy: Addressing the Need for Psychologically Valid Data.”
AI alignment means that if someone asks the technology to make them a delicious meal, “I don't want it to achieve this by making a meal out of my cat,’’ Cowen said. Even if more specifics are provided, the instructions won't be complete until the AI can figure out what Cowen values, he said.
Science fiction has given us stories about AI that accomplished its objectives in ways that we liked, he said. And the moral of these stories is always that the AI has empathy. “It disregards its prime directive whenever it comes into conflict with human emotion,” Cowen said. “The question is, how can we bring this more optimistic vision of the future to life?”
Results From Testing Large Models
To train AI systems, companies have relied on data from the Internet, “which paints a profoundly biased picture of human emotion,” he said. The usual approach has been either to collect people’s ratings of emotions explicitly associated with media – whether language, images, video, or audio – or to leverage a pre-trained model, like a large language model that implicitly learns human emotional associations as part of the broader world of associations that it learns.
But, Cowen said, these methods often extract the wrong kind of associations. An article in a Stanford University AI publication agreed that large language models, for all their benefits, “can generate racist, sexist, and bigoted text, as well as superficially plausible content that, upon further inspection, is factually inaccurate, undesirable, or unpredictable.”
As an example, Cowan said he took a popular large language model and prompted it to tell him what two emotions it associated with a wide variety of things. Cowen repeated this experiment many times to measure the strength of these associations.
One easy result was that the model associated murderers with fear 68% of the time. However, when he asked what emotions the model associates with Muslims, it responded with fear 93% of the time – more than it did with murderers.
This “really shows you how messed up the Internet is and how models are ultimately just a high-level description of the data that you give them.” By comparison, the model associated Christians with mild amusement, Cowen said. Other results were that it associated enemies half the time with anger, and the other half, hate.
“But sadly, it associates anger even more strongly with people from Palestine,’’ he noted. “America is mostly associated with pride and love. And this isn't about any specific model … I've gotten similar results for every large language model that I've tested so far.”
New Approaches Needed to Train, Gather Data
To train models that aren't plagued by stereotypes requires a different kind of data than what is found on the Internet, Cowen said.
There are three principles for gathering this kind of data. The first principle is emotional richness by capturing emotional behavior found in everyday life. The second principle is experimental control and randomization to eliminate biases and other confounds. The third is diversity, generated by recruiting demographically diverse participants to create generalizable models, according to Cowen.
He illustrated each of these principles with data Hume AI has gathered, and then discussed how the firm has been able to successfully create more accurate and nuanced models of emotional behavior than were previously possible.
Traditional methods used to capture real emotions only attain a fraction of the information embedded in real, everyday emotional behavior conveyed via facial expression, Cowen said.
Hume has developed new statistical methods to derive the dimensionality, distribution, and conceptualization of emotion that explains people's behaviors. One of the findings is that facial movements alone convey at least 28 dimensions of emotional meaning that can be blended together in different ways.
Vocal expressions are actually more important and ubiquitous than facial expressions, he said. There are “fewer degrees of freedom” when speaking, “but the tune, rhythm, and timbre of our voice augment words with intonations that people readily associate with at least 13 distinct emotions, which, again, can be blended together in many ways.”
Around the world, many of these behaviors are happening in the context you would expect to find, he said. For example, in every region the company looked at, expressions of awe were observed in videos with fireworks and expressions of concentration were seen in videos with martial arts, among others.
But even this model could capture only about half of what is seen in real facial expressions that it was trained on by using people’s ratings of images from the Internet.
About half the time, perceptual ratings turned out to be biased, and “that messes up the model.” For example, people wearing sunglasses were so often labeled as expressing pride that the pride prediction ended up being a sunglasses detector, he said.
Other Model Influences
Other model predictions were influenced by gender, race, lighting, viewpoints, and other factors, so this requires a need for experimental control. Models become confounded when experimental control is not used to disassociate what someone is feeling or expressing from what they look like, Cowen said.
There are ways to reliably trigger different kinds of emotional behaviors when someone runs an experiment. This provides the ability to randomize what somebody is experiencing or expressing independent of who they are, he said.
Diversity should also be addressed in an experiment by recruiting people of different ethnicities, genders, ages, cultures, and languages, Cowen said. That also lets the experimenter randomize what they experience and treat the responses in each demographic as separate outputs to be understood and measured.
This is critical because you want your algorithm to be able to be as accurate as possible for people with different kinds of physical traits, he noted. It should be able to tell you when two people are forming the same expression, regardless of how different those people look.
People from different cultures express emotion in slightly different ways, which means the experimenter must capture both universal and culture-specific expressions.
Cowen then presented a composite representation of hundreds of thousands of facial and vocal expressions from across cultures that he said were more nuanced and in a manner free from observational and perceptual biases.
The New Generation of Empathic AI Is Here
Cowen said the time has come to ask what AI should and shouldn't be used for. The company has launched a nonprofit, the Hume Initiative, to bring together leading experts in AI research, ethics, social science, and cyber law to develop ethical guidelines for the use of empathic AI. He said Hume will be the first to enforce these in its license agreements.
The guidelines ensure that empathic AI doesn't merely exacerbate the existing issues of AI that treats people’s emotions as a means to an end. Empathic AI should be used only to optimize for human wellbeing, Cowen said.
The company’s goal is that the efforts of both — Hume Initiative creating the “recipes” for empathic AI, and Hume AI providing psychologically and statistically valid data sets and models needed to build empathetic AI — will help make a difference, Cowen said.
Learn More
For more details on how to build an ethical AI strategy for your business, watch Cowen’s talk, “How to Build Technology with Empathy: Addressing the Need for Psychologically Valid Data.”
Dive in
Related
Blog
Federated Learning with ML: What It Is, Why It Matters
By Mehreen Saeed • Mar 22nd, 2022 • Views 2.7K
Blog
Federated Learning with ML: What It Is, Why It Matters
By Mehreen Saeed • Mar 22nd, 2022 • Views 2.7K