The Week in AI: Open Pretrained Transformer, Medical Imaging Roadblocks, Ocean Wave Breaker, AI Helps University Students
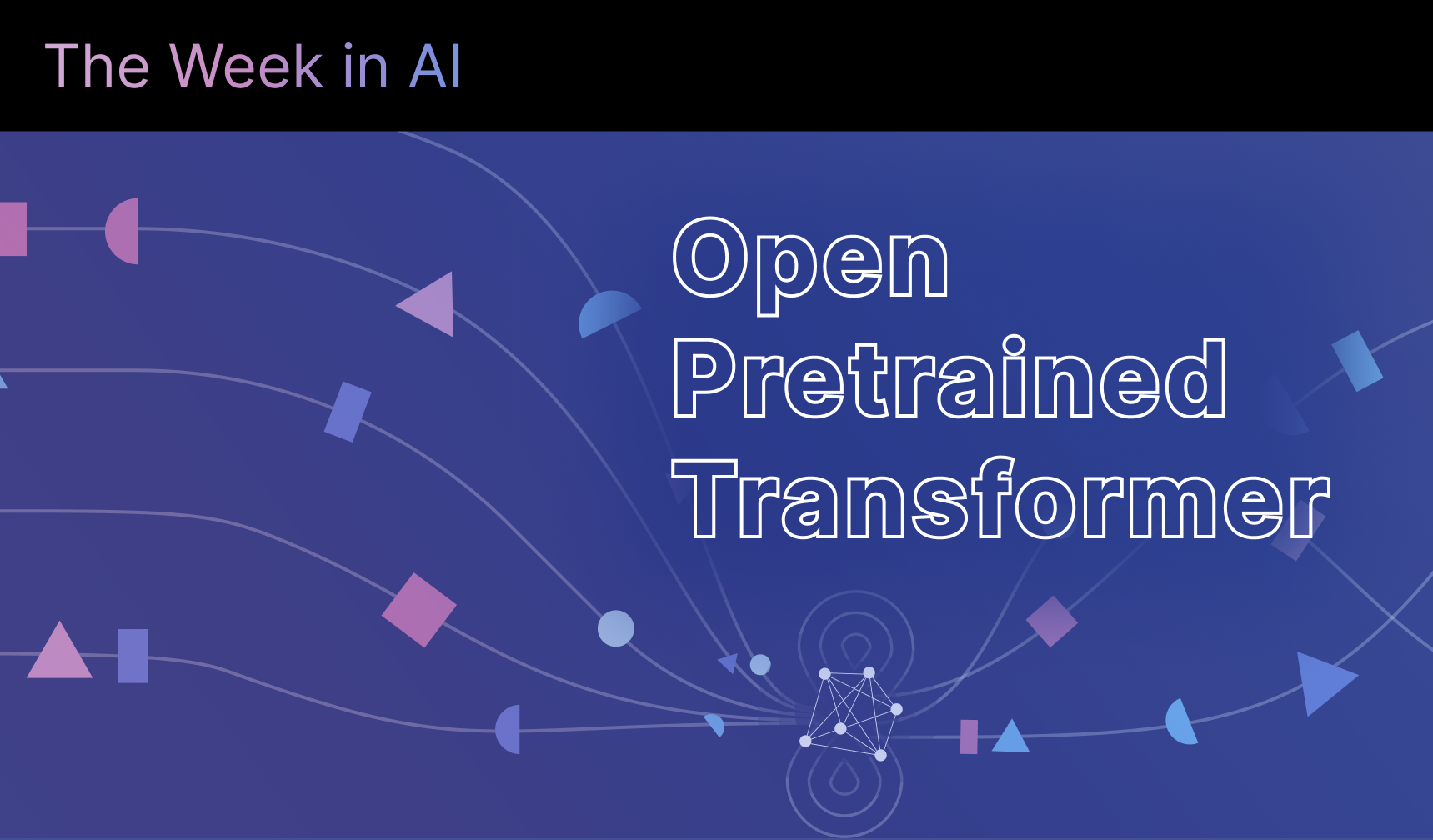
Meta open sources its large language model, data limitations hinder clinical machine learning, AI improves offshore structures, and at-risk university students get a second chance at success

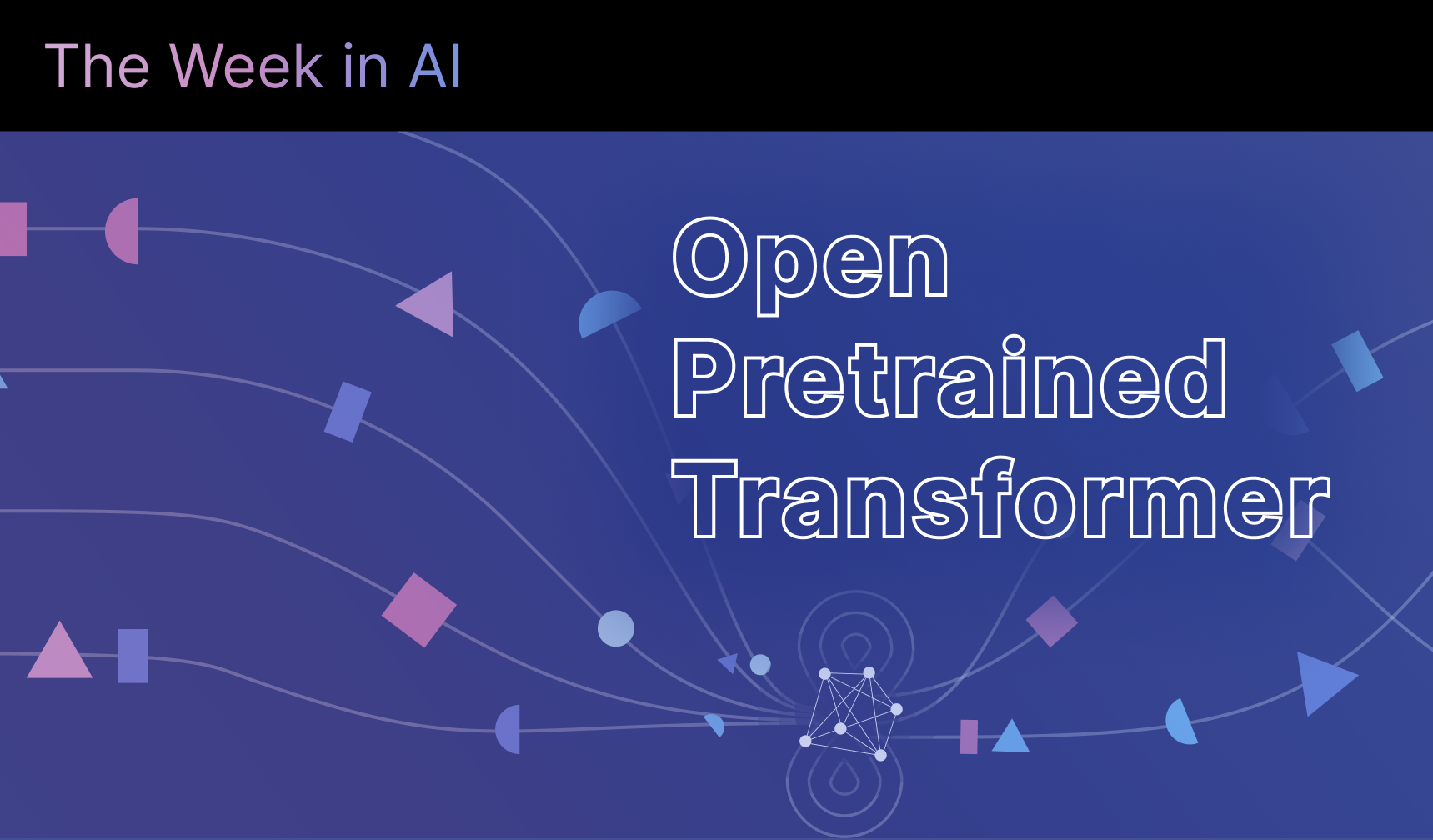
The Week in AI is a roundup of key AI/ML research and news to keep you informed in the age of high-tech velocity. From Meta’s large language model, now available to the public, to AI helping students succeed in university, here are this week’s highlights.
Meta’s OPT-175B Is Now Available More Broadly
Meta has given access to its Open Pretrained Transformer (OPT-175B), the first language model with 175B parameters, to the broader AI community. Large language models are natural language processing systems trained on massive volumes of text that can both understand and generate texts. Meta also announced the availability of the code and dataset used for training and using OPT-175B.
By giving researchers free access to the model and the data used to train it, Meta hopes to accelerate AI research, as well as reduce ethical risks that often come with these technologies. Furthermore, Meta developed OPT-175B with energy efficiency in mind; it has one-seventh the carbon footprint of GPT-3.
In the recent past, instances of biased AI models have led to terrible consequences regarding race and gender, not because of the model, but due to the dataset used for training. Meta believes that access restrictions have also caused improvements related to robustness and risk mitigation to slow down significantly.
This was the company’s motivation for releasing OPT-175B under a noncommercial license to academic researchers, government officials, industry research laboratories, and the civil society at large.
You can access the open-source code and small-scale pretrained models here, request access to OPT-175B here, and read the paper here.
The release also includes more than 100 pages of notes, where researchers log every bug, crash, and reboot in a three-month training process that ran nonstop from October 2021 to January 2022. Meta’s move to demonstrate a commitment to reducing algorithmic biases–-after facing criticism in the past for the lack of transparency–-should be widely welcomed by the general scientific community.
Machine Learning Faces Challenges with Medical Imaging
A recent study published in NPJ Digital Medicine reveals that data limitations, evaluation issues, and publishing incentives are slowing the clinical progress of ML in medical imaging. ML, which relies heavily on data, has been considered a promising yet controversial tool for healthcare providers. Appropriate regulations must be implemented to ensure that ML is effectively implemented.
Studies have shown that health disparities tend to arise when artificial intelligence systems are plagued with fairness issues and biases, limiting the clinical use of ML in medical images. Issues can surface from the beginning, depending on how the data was collected, how the dataset was created and distributed, and what biases exist in the data.
At the evaluation stage, researchers discovered further challenges in selecting evaluation targets, choosing suitable metrics, and adopting statistical best practices. During publishing, some incentives may negatively affect the usability of the presented information, due to a lack of clarity from authors or omission of important details, reducing the reproducibility of a study.
To tackle these challenges, researchers recommend raising awareness about data limitations in healthcare imaging, promoting the use of established best practices for ML evaluations, and improving publication norms through transparency.
Despite obstacles, there have been notable successes for ML recently. A study revealed that ML can help detect abdominal hernia surgery complications with high accuracy. Moreover, according to the study, reducing the U.S. hernia recurrence rate by just 1% by leveraging ML could save the healthcare system $30 million.
A Neural Network Algorithm Predicts How Ocean Waves Break
MIT engineers have found a new way to model how waves break using machine learning along with data from wave-tank experiments and tweaking the equations traditionally used to predict wave behavior. Such equations were typically leveraged by engineers to help them design resilient offshore platforms and structures.
Today, a neural network algorithm, supported by the Swiss National Science Foundation and the U.S. Office of Naval Research, helps them capture the complexity of breaking waves. To achieve more accurate predictions than conventional models of how and when a break occurs, the model estimates the wave’s steepness just before breaking, as well as its energy and frequency after breaking.
The researchers’ results, published in the journal Nature Communications, will help scientists understand how a breaking wave affects the water around it. Knowing precisely how waves interact with one another can help improve the design of offshore structures.
Scientists can also improve predictions for how the ocean interacts with the atmosphere. For instance, they can predict how much carbon dioxide and other atmospheric gases the ocean can absorb. The team’s updated wave model is open sourced so that other scientists can study additional climate simulation use cases.
AI Prevents Students from Failing University
The Learning Intelligent System (LIS) project, managed by researchers at the Open University of Catalonia (UOC) in Barcelona, Spain, recently demonstrated how AI can offer new opportunities to improve university education. The researchers leveraged AI to help students who are at risk of failing or dropping out to improve their academic performance.
Backed by the eLearning Innovation Center, the automated system was created by a transdisciplinary research team at UOC and has already produced encouraging results over the past year. A study, originally published in 2021 in the International Journal of Educational Technology in Higher Education, revealed LIS’s ability to successfully identify students at risk of failing a course.
In addition, LIS provides relevant information to improve the learning process and student performance by providing guidelines on the importance of planning and setting goals, then evaluating if they are met. To measure the effectiveness of the system, three groups of students received a different number of messages based on the system’s identifications: One group received full support, the second group only one message, and the third group received no message.
The first group experienced higher academic performance, followed by the second group. The third group had the worst performance and highest dropout rate. However, the researchers warn, while a correlation may exist in the data, they cannot confirm whether there is a causal relationship. Also, self-selection bias is embedded in the data, since students volunteered to participate in the study and therefore were motivated to perform better at school.
While the model achieved promising results, the researchers hope for widespread adoption, which will require extensive study and experiments before gaining public trust.
Why These Stories Matter
Though research on ML use in medical imaging has grown significantly in recent years, improvements in the clinical use of such data remain limited. Medical practitioners, ML scientists, and lawmakers must come together to define guiding principles that will protect private user information for the long term.
Meanwhile, Meta’s commitment to transparent AI sends a clear message to the broader community that improvement in AI ethics and biases cannot be achieved without the contributions of extended multidisciplinary groups.
Lastly, news of AI helping to improve the design of wave-breaking offshore platforms to protect lives, as well as assisting students to succeed in academia, are further signs that this technology is critical to people’s daily lives.
Until next time, stay informed and get involved!