Panel: Tools to Accelerate ML Development & Rate of Innovation
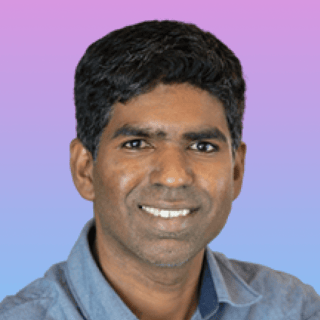
Siva leads engineering at KeepTruckin. KeepTruckin is a next-gen fleet management platform that aims to connect all the trucks online to disrupt the trillion dollar long-haul transportation industry. Prior to joining KeepTruckin, Siva led consumer relevance eng at Twitter which played a big part in turning around Twitter’s user growth. Before joining Twitter, he spent time at Yahoo! Labs and IBM Research pioneering work in machine learning, real-time search, and graph analysis. He holds several publications and patent in ML and was awarded an Edelman Laureate in 2014. Siva earned his Bachelor of Technology degree from the Indian Institute of Technology, Guwahati, and completed his Masters in Computer Engineering at the University of Massachusetts Amherst.
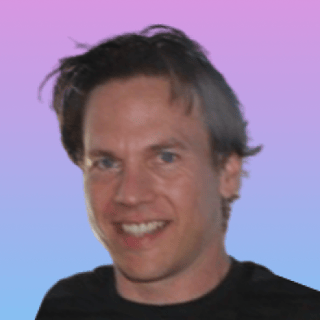
Currently I lead the Radar development for retail-autonomy programs at GM, spanning teams in Israel and the United States. At my current role, I’m responsible for all aspects of Radar technology development, Radar product development and Radar integration into GM vehicles – focusing on next-gen technologies for autonomous driving at the retail market (L2-L4). This is done with close collaboration with the Radar ecosystem – Tier1/2s, start-ups etc. Formerly at GM, I led in-house development of radars for L4/L5 autonomous driving (RoboTaxi), in collaboration with Cruise automation at SF (owned by GM). Prior to GM, I’ve led HW, SW and ASIC development at several start-up companies – mostly at the fields of communication, PLC, IoT, 3G/4G and Radar. I have a B.Sc. at Electrical Engineering from Ben-Gurion University, Israel.
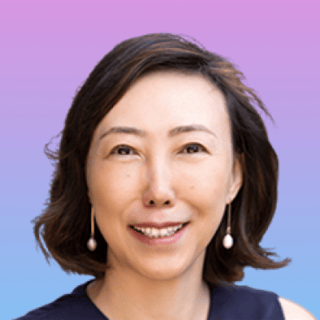
Dr. Yanbing Li is a global business and technology leader with extensive leadership experience building market leading products and hyper-growth businesses of $1B+ in the US and China. While deeply rooted in technology, she has led large scale P&L and global operations. She has expertise in cloud, large scale enterprise software, cloud commerce and marketplace, cloud operations, server and storage, business continuity and disaster recovery, and EDA etc.
Yanbing is currently the Senior Vice President of Software at Aurora. She joined Aurora from Google where she was a Vice President of Product and Engineering, leading the Enterprise Services Platform (ESP) organization in Google Cloud. Her areas of responsibilities include: Google Cloud Commerce - a monetization platform which transforms service monetization and accelerates revenue acquisition for Google Cloud; Cloud Operations - planet scale operations management for both Cloud and all of the Google services; and Service Infrastructure which enables consistent and reliable services for Google. She leads engineering, product management and user experience, and led the ESP portfolio to grow 100+% YoY in revenue in the past 12 months.
Prior to Google, Yanbing was the Senior Vice President and General Manager for the Storage and Availability Business Unit (SABU) at VMware, responsible for the P&L for a rich portfolio of products in software-defined storage, hyper-converged infrastructure (vSAN and VxRail), vSphere storage, data protection and storage and availability services for the cloud. Under her leadership, SABU became one of the fastest growing businesses for VMware and in the storage industry, with vSAN growing from $60M to $800M, and the BU from $200 to $1B, in 3 years.
During her tenure with VMware, she held multiple executive leadership roles including General Manager for vCloud Air Storage, VP of Engineering for Storage, VP of Central Engineering, VP of Global R&D Sites, and Managing Director of China R&D. She was based out of China for 5 years building VMware’s China R&D operations and was subsequently responsible for all of VMware’s global R&D sites.
Prior to VMware, she worked at Synopsys (a leading Electronic Design Automation software maker) for nine years in various research, development, and engineering leadership roles.
Yanbing holds a Ph.D. degree from Princeton University, a Master's degree from Cornell University, and a BS degree from Tsinghua University (Beijing), in Electrical Engineering and Computer Engineering. She completed the Stanford Executive Program from Stanford Graduate School of Business in 2014.
Yanbing is a member of the Board of Directors at Neophotonics (NYSE: NPTN), and serves on the Audit Committee. She serves on the Silicon Valley WiE Advisory Board at San Jose University. She was named one of the Most Powerful Women Engineers multiple times by Business Insider. She was inducted to the Women in Technology International (WITI) Hall of Fame in 2018.
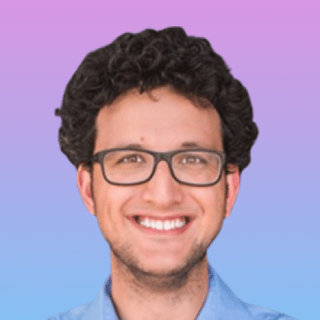
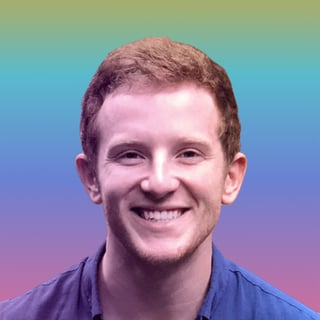
Russell Kaplan leads Scale Nucleus, the data management platform for machine learning teams. He was previously founder and CEO of Helia AI, a computer vision startup for real-time video understanding, which Scale acquired in 2020. Before that, Russell was a senior machine learning scientist on Tesla's Autopilot team, and he received his M.S. and B.S. from Stanford University, where he was a researcher in the Stanford Vision Lab advised by Fei-Fei Li.
Great machine learning teams iterate quickly. Learn from experts at Aurora, GM, KeepTruckin, and Motional about the tools and techniques they use to accelerate their ML development at every stage—from building datasets, to trying new experiments, validating models, deploying ML in production, and improving model performance.
Speaker 1 (00:41): [inaudible]
Russell Kaplan (00:42): Hello. Thank you all for joining welcome. We're excited to have you today on a panel about increasing your ML team's rate of innovation. I'm very fortunate to be joined here by several experts in this field with a diverse set of backgrounds, spanning machine learning, autonomy, great software engineering practices and hardware development with machine learning teams. So I'd like to start by having our panelists introduce themselves. Maybe you can vent, you can start and we can get to know each other.
Dr. Yangbing Li (01:09): Thank you, Russell. Thank you for having me on the panel. Hi everyone. I'm Yan Bingley. I'm a head of software engineering add aura. I have to say I'm new to the autonomous driving space and new to machine learning. So a lot to learn from, from this, from this panel and you know, from my experience at aura. So I have been at aura for, for two months. It's been a very energizing, invigorating journey, you know, learnings things in, in my experience here. So I came from a loss of background in cloud, in enterprise software development, you know, large scale cloud services, et cetera. I was most recently at Google as VP of product and engineering as part of Google cloud. So really excited to be here and looking forward to the discussion back to you.
Russell Kaplan (02:05): Great. Sami can you introduce yourself next?
Sammy Omari (02:08): Yes, I'm. My name is Sami Mori. I'm the head of autonomy at motional. I've joined motional around nine months ago and leading all of the machine learning machine learning infrastructure and core robotics programs here at motional. I've run 15 years of experience building robotics, machine learning and machine learning infrastructure teams. Most recently that lift of five before that at GoPro and also my own sort of,
Russell Kaplan (02:36): I guess, Emmy and going in.
Gonen Barkan (02:38): Okay, so hi, I'm Matt Barton based out of Israel. I'm leading the reader development for retail autonomy, general motors leading a global team, both in Israel and the U S been in GM for almost eight years. Now. We'll see develop Raiders for cruise automation and the past five years, and now we're taking the next step on what readers need to do on retail autonomy. So happy to be here.
Russell Kaplan (03:08): Thank you. Got it. Ship up.
Siva Gurumurthy (03:09): Hi, everyone. Nice to meet you. All. My name is Shiva. I joined KeepTruckin about three years back. Believe we build like feed management software for trucking companies from the last year or two, we have been entering into the field of like, yeah, I building automated coaching and automated even detections for the, for the vehicles before keep tracking. I've been at Twitter. I was there for seven years and then the consumer product machine learning teams, optimizing engagement and user growth. Yeah. So very excited to meet you all and looking forward to all the discussions.
Russell Kaplan (03:55): Thank you. Shipra and welcome. And I, my name is Russell. I'm the head of nucleus here at scale, which is our dataset management product that helps teams improve their ML models by improving their data sets almost a year ago and scale acquired my computer vision startup. And before that I was a machine learning scientist at quest law on autopilot team focused on the core vision neural network. Welcome to all our panelists today. Really excited to talk about this topic of increasing your NL teams rate of innovation. I think we've seen a lot of progress in the past few years with a lot still to go. And I wanted to start with some concrete examples in workflows that might help here specifically beginning with dataset development. So there's this question of when you need to add a new output from your perception stack, say for example, you want to start detecting traffic cones for the first time, folks are starting to measure what is the end to end cycle time for an ask like that and how do we increase it? And I want to just start with, with you Sandy, you know, what is your process for addressing something like that today and how have you, have you changed over time?
Sammy Omari (05:01): Absolutely. I think one of the key aspects in building a self-driving autonomous car is really to, to not just handle the average case or the normal case, but really start tackling the very long tail of events, the very rare cases. So, you know, to use one very specific example is, you know, we have, obviously, for example, if you do traffic light detection, you know, we have lots of green lights, lots of red lights, but Amber yellow lights, we don't have too many, right. And that's just a very benign case. Obviously there's, there's a lot more complex cases, but using that as an example, what, what you can do to really kind of boost the development workflow to development velocity is V we need to start building out a lot of automation. So once we build that emotional as what we call the continuous learning framework.
Sammy Omari (05:46): So what that means is this basically the framework that starts at data set, labeling, or detecting of rare scenarios, then labeling those scenarios that aggregating those labeled 10 hours into new datasets, and then having a very effective distributor training framework to then train these new models. And then the last stage is really about large scale simulation to then understand how this sub components. So in this case, for example, like improve traffic, light detection then impacts overall end to end performance. So really our goal is to keep improving every single part of that continuous learning framework, starting from detecting these rare scenarios, all the way to understanding how the new model impacts end to end performance and to give a little bit of a perspective, what that means in the context of traffic light detection is if we start having maybe a very naive, like a very early traffic light detector, that's not particularly good, right?
Sammy Omari (06:43): But it's like, you know, at first MVP that we can then deploy and evaluate at very, very large scale, like understanding, you know, on, on maybe the last six or 12 months worth of driving data. Hey, give me all the potential traffic lights scenarios, where it looked like they could have been an orange light, an Amber light, right. We are not quite sure. Right. But then it can then take this very, very large set of data where our detector wasn't quite sure, but you know, there might've been something there. And then what we can do about this is basically twofold. One, maybe we already have like an offline perception system that can run on the cloud where we can deploy significant more resources and higher capacity models to then basically create branches data through auto labeling, and then use that to then train our online systems.
Sammy Omari (07:29): So that's one avenue that we can create large scale training data very fast. And secondly, we can, we can then send this to our manual annotators in particularly in areas where our offline detection system maybe says, Hey, you know what, I'm still not quite sure. Right? It's I'm still quite uncertain. And so then this, we want to send to human labeler to then basically give the, the final yes or no, whether or not there was a number of traffic Canton, if there was one where in the image it was right. So basically each of these pipelines at each of these stages of the pipeline, you basically want to start, we are in the process of optimizing posts, throughput, as well as the actual quality of the output of each of these, these components.
Gonen Barkan (08:17): Yeah. Great. Well, I like, thanks Sam. Yeah. I'd like to give, maybe give the, the, you know, the view from the other side. So I'm not, I'm an expert, I'm actually, we're working on what all of you need to actually do something eventually. So we had exactly the same challenge, you know, trying to when I San Francisco drive through construction zone. So the first thing was how do you detect traffic homes, which are plastic round very hard for radars? So the assumption of getting was okay, when you, you need Heights. So, you know, you know that it's, non-drivable et cetera, but, you know, doing you closely the relations with the perception teams that you figured out that what actually helps is the connection between the cone to the ground. And that drives completely different data that is coming in and actually was much more useful than what we thought to begin with. And so eventually we need to look at it, you know, eventually you're as good as the input you get.
Dr. Yangbing Li (09:20): Yeah. So love the points that are raised by both Pamela. This is coming back to the, certainly the data set a collection. You know, we also use a variety of approaches. You know, what are, is a fresh data's through, you know, collected using our fleet of vehicles or, you know, really tapping into existing data that we already have and extracting out certain relevant data, you know, automatically through those existing dataset. And I also like how Sammy touchdown, the power of simulation and assimilation for us is not just the Holly help us validate things, but really help us provide extensive set of synthetic data based on data that we've collected either from fresh logs where existing logs and use that to dramatically extend into different cases that you normally wouldn't see, you know, driving on the road. So for example, we were analyzing a case where a child was running onto the street where the truck is coming at it and started playing. That is one great data point, you know, through the power of simulation, we can put in different weather condition, different vegetation, the environment around the same segment of road, and just create a lot more different type of coverage that we can achieve. Even though, you know, we have this started was one particular data point. So a combinations of collecting data, leveraging existing data and using a simulation instance, scientific data to dramatically expand the coverage, as well as the quality of our data. As you know, we use all of these different approaches.
Russell Kaplan (11:04): Really interesting. Yeah. Lots of, lots of great threads to share, to kind of build off of here. I know ship, I think you had something to say as well on this. I would love your perspective on how to kind of compare to the approach to keep trucking.
Siva Gurumurthy (11:16): Yeah, this is awesome. Great points about like, you know, like deploying a Hy-Vee call model and trying to collect that data first and then going deep on the places where your monitor doesn't do well. So we do something like that. So for keep trucking one great advantage is that we have more than 500,000, you know, vehicles already, you know, like our, our hardware running and almost a hundred thousand cameras, which have been running for several years. So we have invested in, in, in human annotation and, and we have actually labored almost like more than 20 million videos over the last few years. And we have English the data set with almost every scenario that you could encounter from, from the talking perspective. And so if you, if they cover [inaudible] or any other instances stop saying, or if you have the speed sign, like very likely that our pool of videos already have an annotation out on that.
Siva Gurumurthy (12:16): And they likely that, you know, sometimes that our presentation isn't, isn't strong, I'm like, for example, it's on a snowy day and we want to detect the speed sign better. The amount of data in our sample set may not be enough to train good models. So how do you go and figure out those edge cases? I think [inaudible] being covered about like, you know, like deploying and getting more data for, you know, to improve on those cases. Like one specific example for this as recently, we launched a feature called like a driver distraction, almost using cell phones. So almost all the current state of the, you know, the, you know, the methods used like head post based detection. And like we could identify cell phones from the videos and be able to like apply a unified model to be able to detect the combination of factors, you know, cell phone and the body pose and the environmental condition to detect whether it's, it's a, this key cell phone detect like behavior versus, you know, non-discounted cell phone behavior.
Siva Gurumurthy (13:22): So, you know, like it helps us like, you know, like start from Heidi, call a system and then, you know, like, and then like get bad, you know, behave bad examples. I think that's where your model needs to go and do better. And we send it through the same pipeline and, and we kind of know similar to like, you know, there are multiple confidence to this and we have the optimist each piece of software separately. We'll talk more about it, but I think it's, it's kind of similar that everybody uses a similar set of approaches, but I'm pretty sure everybody has an automation in Walden in pieces of this.
Russell Kaplan (14:02): That's really interesting because a lot of these, I think a lot of the common threads here are around focusing dataset building in, in sort of the long tail and knowing where the models are struggling to be able to do that effectively. So I'm really curious to kind of follow dive in a little bit more on this and how it relates to automation, because what I've seen is there can be multiple workflows where in one type of workflow, there's a team that is looking at the performance of the system and you're kind of qualitatively or quantitatively assessing, Hey, where are we? Where are we? Where in the long tail, are we struggling? You know, where, where do we need to do better? And there's that kind of person in the loop component of sort of targeted improvements in scenarios. And then there's an attire, you know, kind of active learning literature on potentially doing that more automatically. And so I'm curious, maybe semi and others, how do you, how do you think about the balance there between kind of automation and making sure you have people looking at things going on and doing targeted improvements,
Sammy Omari (15:03): Russell, I think you actually summarized it really nicely, right? There are these kind of two approaches, and I think we, we basically need to invest in books and I think it really depends on the use case and on the exact scenario to kind of, kind of see in what direction you want to go, let me use the example of prediction, right. Or predicting where other agents are going to go next. Right? So in the context of long-tail scenarios, for example, detecting pedestrians that are stepping out between cars and then predicting whether or not they will actually step in front of our car or they will stay, you know, between, between the cars that are parked on the side, it's actually something that's inherently challenging. So basically what that means is you need to be able to infer whether or not, you know, this person will step out or not just potentially with a few pixels of a human that you detect in a, in a camera maybe like 50 or 60 or 80 meters, or similarly, if you use a LIDAR system, you know, there's a number of points that you will have on this person is going to be very, very limited, right?
Sammy Omari (16:02): So in this case, what's most important is how can we mine for, for this data, right? Either fully, automatically on supervised or maybe supervised, right? Either way. The most important part is to build this mining system and framework in a way that allows the developers to iterate incredibly fast. So what we did at motional is basically what we call scenario, search and scenario mining framework. So what this allows us to do is basically after every mission that we drive of each of our cars, we compute the very large set of attributes that we can then use to query at scale throughout developers. And for example, what that means is one of the attributes, for example, prediction, error. So, you know, we can offer the fact that, Hey, for example, we can have a developer write a very simple credit of saying, Hey, give me all the pedestrians that you always don't have to detecting relatively close to eco.
Sammy Omari (16:58): And that had a large prediction error, right? And if we can start mining for those and that, this is basically a very targeted, very top that mining example, right? And then again, we can then take that and then either send that to alter labeling or maybe to human annotation, right? To me, the most important part is really that these attributes are very, we have a very large breadth of attributes. So these queries can be done in seconds. Basically it's just an Elvis equal credit, right? But these attributes that can be both model error, model errors, for example, prediction, error, or perception, error say perception, flickering, right? You can think of a large variety of model or attributes, but in addition, you also want to have scenario attributes. For example, if we are not doing particularly well at unprotected turns or when the, when other agents are cutting in, et cetera, right?
Sammy Omari (17:45): So you also want to have basically computers that have attributes that can then be used by the developers to query and, you know, to create new data sets in a matter of seconds or minutes instead of, you know, hours of days beyond that. I think the other big aspect is we also need to have the ability to after the fact compute a larger number of attributes that we may have not thought of ahead of time. I'd say, if you do hit a new problem that you haven't thought of before, you need to have the ability to basically retroactively go back onto a very, very large scale set of data that we've collected in the last year or two or three years, and then retro activity computers attributes, and doing that again with relatively high and low latency and high throughput. But at the same time, of course, these kinds of things are actually coming at a relatively high cost.
Sammy Omari (18:34): So doing that in a way that is actually cost-effective is absolutely critical for us. So for us developing a self-driving car, it's not only about making sure that the technology is going in the right direction, that we are really tackling this kind of long tail of, of issues, but at the same time, we need to do this in a commercially viable context, right? So whenever we design our systems, it's always about accelerating developer velocity, accelerating the velocity at which we can burn down the long tail, but at the same time, doing that with cost in mind as well.
Dr. Yangbing Li (19:05): Yeah. I love to add on that developer of velocity, notion of me mentioned, you know, having come from the non autonomous driving world as I quickly, you know, getting, get up to speed here at aura, I distinctly see is there is one aspect of video that's core ML development, you know, beating the model training and, and et cetera. But there is also a loss of element is fundamentally about accelerating ML. This is about taking the friction of, you know, Buting those core ML technology taking the friction out of it. This is actually where there's plenty of opportunity to apply automation for, you know, for example, how we make launching a experiment, a truly push button experience so that, you know, your Elmo developers, they focus on making small changes around their, you know, ML code PI torch ML code, but they get this automated experience of, you know, running experimentation and getting the results.
Dr. Yangbing Li (20:08): There is also a loss of work we do around making the validation process. Push-button seamless. There is the aspect of making your infrastructure kind of invisible and completely elastic and behind the scene. Again, you know, we abstract away all the complexity around the underlying infrastructure. So we allow our ML developers to really focus on that experience of quickly developing validating, VML model. But we take all these other frictions that often can be very cumbersome out of the way. So I call these the true ML accelerators and they play a phenomenal role in making our ML team very productive.
Gonen Barkan (20:57): Yeah, thank you. That that's actually, I agree that that the automation is, is critical. And I think one additional aspect, which makes it even more critical because lots of time we, we treat the input. Data is fixed. Like changing sensor is, is a big event, but you know, when we look forward and how sensors will behave in the near future, it's not going to be fixed. So for example, readers today, you can control the way they operate on the fly per scenario. So in practical, practically, even though the hardware is the same, you might control it on the fly in a kind of a cognitive way. And actually you have multiple sensors changing on the fly because eventually you want to reuse the hardware to what you need. When you go into a parking lot, for example, you have like the most expensive area in your car looking 300 meters and you don't need it to look 300 meters.
Gonen Barkan (21:48): So you can on the fly change the way you behave to get to the optimal sensing for 20 meters in a parking lot. But this means you actually need to digest new data on the flight. It's like a new sensor for the emo pipeline. And today this is a major roadblock, like a lot of MLT just say, no, no, no, don't change anything. You, you, you cannot change the data and you lose a lot of, a lot of capability. You have very expensive hardware, very flexible software, but you can actually not use it. So having a very flexible ML pipelines to digest, train, adapt the noise modeling, adapt the way you treat the data is extremely critical to be able to utilize the sensor effectively.
Siva Gurumurthy (22:36): Yeah. Good points everyone. So I, I want to just touch back on that my data store that does have you want to talk about, you know, spoke about, it's actually a key piece of, of the ability to, you know, dig deeper into the scenarios and, and, and actually the problematic scenarios like, Hey, when is my model of fighting at this, this threshold for this type of scenario and running it as a, you know, relational query and be able to retrieve the data, visualize it, plot it, and see like, Hey, this paddle meter is what is misbehaving. It's kind of fundamental, inability to like dig deeper and actually dissolve it. So like, I, I believe for everyone it's a similar, you know, like in general, like, you know, it's, it's a lot of sub pieces, right? From the, you know, like how your sensors are being collected and how much it's central to the cloud and how your perception stack is running home with pre-processing and post-processing, and finally like a bunch of classifiers.
Siva Gurumurthy (23:40): So they end to fire the actual event, whether it's happening or not. And there is energy that happens at each of that, that gets propagated. And unless you have a tool that can isolate by competent and say, Hey, give me all the examples where it failed in this first component, what was successful on the later component, it's very hard to fall asleep. And we struggled a lot for a bit until we built this like muscle to be able to quickly get like an order of seconds, like this examples, you know, like do it. So one key element of this is like when we build models on the cloud and when we deploy that to the edge, obviously it supports certain position system and the close, how can we be faster that model? And when you do the sporting, you end up in a lot of losses.
Siva Gurumurthy (24:30): It's one thing that we try to get to is to take this model that we have deploying the edge and try to simulate or emulate or whatever you can use. We have a test bed system where you can still run experiments as if this model is running on the edge. And that's the key towards this end to end validation of your experiment. Without that, like, we ended up like spending a lot of cycles where your models will be fantastic and you're deployed to the edge. And obviously when you're deployed at the edge, you have to drive around and collect more data to validate, and it takes a longer cycle. So the whole spirit of experimentation gets killed. So, so in order to really speed that up, we had to virtualize that edge environment into the cloud. And then, you know, obviously the other techniques of experimentation of configs and like, you know, push of a button to, you know, like do the same too, and simulation comes to play, but that was one of our key, you know, like understanding as we, as we [inaudible] about breaking.
Russell Kaplan (25:42): It's a really interesting point of yeah. Being able to model what's happening on the edge accurately in the cloud and trying to kind of reduce the cycle time for validation there. And I think that's one of the common themes here is, you know, what is the end to end cycle time? And then where do you cut it? And so it sounds like folks have pretty sophisticated set of techniques for kind of building these datasets and kind of turning the loop to be able to boost these sort of long tail representations. Then the next thing I wanted to dive into as a point that Yan big made around, you know, once, once you've changed the dataset there, there's still all this friction and, you know, getting your kind of experimental results from that end to end. And so I'm curious, kind of from an ML training process standpoint, what are some of the things that your teams have have done to make it faster from, okay, we've adjusted our dataset in some way to, alright, now, now we actually have new models and we can validate how good they are. Are our ML engineers basically kind of doing all of the experimentation from scratch each time. Is there some sort of shared infrastructure at this point? How have you, have you all seen that speed up?
Sammy Omari (26:50): Yes. If I may, that's, that's a really good question, Russell. Right. I think that there's, there's friction basically throughout this process. Right. And I, I think too, for us, one of the, the biggest, the biggest challenge is actually to infer from, you know, we train our machine learning models, right? You have a prediction model of perception, maybe it's one big model with different heads, right. But that's more of an implementation detail, but at the end of the day, we have certain loss functions, right. That we want to optimize for in the machine learning more than, you know, during training, you know, people mentioned PI torch, right. Do you see how these kinds of losses go down and things were great, but that doesn't necessarily equate to overall end to end improvement of system performance, right. In particular, as you kind of go down the long tail of issues, losses very often just to reflect the average, right?
Sammy Omari (27:37): Because they are accumulated over a larger number of, of, of, of a large set of a large dataset. So really it's about figuring out, Hey, did I not regress on, in the average, in the nominal, but at the same time data actually boost my performance on the long tail of scenarios. Right? And so in addition to of course, having very kind of dedicated data sets that are tailored towards long tail D the other aspect is also to evaluate this at scale in simulation on very kind of highly specialized data sets. So the same way that we use kind of this scenario, mining technique to mind for particular scenarios that we need to train on, you also can use the same technique to actually mind for simulation scenarios, very contained on understand the end to end performance of our system, right. I mean, you know, since you asked about friction roster, right?
Sammy Omari (28:29): I think one key aspect in running large scale simulation is not necessarily actually running in that scale. Right? If you look in our industry, all the players they're boasting about, you know, having millions of miles of simulation, but the actual challenge, the underlying challenge is how do we optimize the signal to noise ratio when we run millions of miles? Right. Let me just give one example, right? Once our, the behavior of the autonomous vehicle starts changing, obviously this has an impact on other agents around us. So we need to start building what's called reactive agents that are then need to react to us in a meaningful way, right? So if, if you're not doing a particularly good job at that, basically two things can happen. One other agent starts colliding with us in scenarios where in reality, they wouldn't have collided with Australia. If you do run this at scale like thousands or millions of miles, suddenly even like a false positive rate of collisions of babies with sub sub percent starts ending up with very large number of collisions that then we need to investigate very closely.
Sammy Omari (29:28): Or the immerse happens very actually have agents reacting too aggressively to our new actions. So when in reality, we would have had actual collisions when, you know, in simulation, we didn't, weren't able to catch them. So building a simulation system with the right reactive agents and the right end to end metrics where we can really automate evaluation at scale and basically get the same signal, or at least highly correlated signal to what you would have received from the fleet. If you would have deployed that on the road with actual humans in the loop with actual safety operators and [inaudible] team in the loop. So getting the same thing from simulation, that's one of the largest challenges. I think not just for us here at emotional, I think that's actually one of the biggest challenges for us in the industry as a whole to get to that point.
Dr. Yangbing Li (30:14): So I love to share some of my experience, you know, due to the space, you know, within the first few weeks of me arriving at aura, we were doing a sprint planning for next few weeks of work and outcome. And there was a proposals of adding some substantial new capability in a very short period of time. And also at the meantime, in the meantime on the new vehicle platform was new sensor, sweetie, et cetera. So it seems like, you know, where adding a loss of new software capability in the meantime, you know, was the platform changing at the same time and everything is meeting. I was thinking, gosh, my team is crazy because there is no way I'm going to just sign up for it. And I was very surprised to see that nobody thinks that he's crazy. And I was the only one who was very surprised that we, as an engineering team sign up to do this.
Dr. Yangbing Li (31:09): And so now several weeks into this journey, and we're looking at daily outcomes of, you know, the, the, the, the output from our trucks on the actual production route that we're running in Texas, it's just been amazing to see the progress that trucks have been made. And every day I, I see the trucks learn new tricks, you know, making certain turns that we couldn't make handling certain scenario, what are is around, you know, pedestrians or constructions that we have not been able to manage before. And it's just, it's like, you know, the truck is growing as if you're watching a small child learning new tricks every day. So, but then as an engineering leader, as we look into, how can we move faster? You know, one way to look into how we keep everyday productive. It seems to truly come down to a balance of how we keep the, the ML side of things smooth, but then we get all these other frictions out of the way.
Dr. Yangbing Li (32:15): And we constantly see, okay, are we managing our CICB pipeline correctly? So that because we're generating multiple magnet, PRS was new capabilities to send to the trucks every day. And are we managing those process tightly so that we have a very successful and smooth running pipeline and also doing very purposeful testing and, you know, not just in simulation and offline validation, but also actually in all the trucks in our way, deploying those codes to the vehicle in the most automated, efficient, smooth fashion. So all these other factors become highly crucial. So everyday we're in trying to really increase the amount of time we spend for the trucks to be in autonomous stage, because that gave us the maximum feedback of what are, we're trying to learn new behavior. We're trying to validate some of the new improvement were changing. So, but it's been exciting to see, you know, the collectively, how we quickly iterate on the ML, but keep everything else running really, really smoothly has made this rapid iteration possible. It's been very impressive for me coming from the cloud side to see the amount of faster iteration we we're achieving in that amount development.
Russell Kaplan (33:42): What if the, what are the points there around? Yeah. Kind of quickly iterating on the ML is I think especially interesting in the context of the folks on this panel who really span, you know, from leading ML teams to leading the infrastructure teams around that, to also kind of developing the hardware that ML teams use and depend, depend on. And I think going in, I would love to hear your perspective on this, this need for ML automation. What is the impact of that on teams that partner with the machine learning engineers like yours? You know, you, you're building, you're building highly advanced radars. And as Sami mentioned, what we ended up caring about is end to end system performance. And so how do you make sure that the experiments you're doing, you know, ended up translating into, you know, antenna improvements?
Gonen Barkan (34:31): Okay, Russell, thank you. And I agree. This is a really a key point. I think most of the discussion is, you know, very computer vision centric, and then also, you know, on high level autonomy and fleets. And, you know, we in GM definitely see radar as being a primary sensor. When you go to retail and, and the, the lead time to develop those radars who does not exist by the way, the readers need to make at least one or two leaps to get to the level that you can rely on them as a primary sensor on a retail vehicle. And you cannot put like tens of lighters on the roof, like you can do on other options. So, and the lead time is long. And to get this in cost in time, and to accelerate the development, you don't want to drive a ten-year-old reader on your car.
Gonen Barkan (35:21): So you need, always need to, to develop to a cost function. You cannot develop only two very simple KPIs, because as I think Sammy said, you don't know how it will affect your overall performance. So you need a cost function and, and you need to evaluate the trade-off. You do the software, you develop the hardware decision you make based on how does it support the mission. And for all of those return autonomy features, that's an ML system. So we need, I think we need to have a mindset of closing the loop end to end. Also with the hardware development, I'm not talking about something you already put in your vehicle, but if you want to make sure that your next generation or the next century you're going to use is going to do exactly what you needed to do and not waste efforts on something else.
Gonen Barkan (36:08): You need to iterate with it from day one. And that's not an easy concept, but I think it's extremely, extremely useful. And I see ML teams and data teams struggle with sensors. They get, and they don't do what they want, and they do a lot of things they don't meet. And I, I think for me, as, as develop the next generation of radars, we want to use, it's critical for me to close the loop with the actual customer system. And this is something that we're working to build and, and we need to fit it in the, in the right framework.
Russell Kaplan (36:47): Yeah, I think it's, I think it's really interesting how there's this kind of second order effect of an ML team, improving their automation for retraining, automatic evaluation, et cetera, which is that it kind of accelerates all the neighboring teams as well, because, you know, then you have people who are developing hardware who are developing dependencies of the ML stack, or top of the ML stack can, can start to test those things in parallel without necessarily requiring those experiments to be run manually. But by each person,
Gonen Barkan (37:18): I just can give a very simple example. I mean, we have to meet the cost and size. Eventually I need to decide, do I put, you know, better resolution, but you know, low-risk in R or vice versa. Can any of the people here tell me what's best what will improve his system better? You cannot have them both. You want it, but you will never fit in cost and time. So you need to make a decision early in the development stage. What's more important resolution or SNR. And, and that's, that's a very hard, tough decision to do it without any data behind it. And to do this, you need to drive the data into an ML system because that's eventually the cost function for what we do
Russell Kaplan (38:02): Building off that point on cost functions. I want to, I want to transition a bit into a theme that many of you have touched on already, which is kind of really focusing on the experimentation and model validation side of things. And I think semi brought up a point around, you know, having sort of multiple hard test sets and measuring your, your kind of long tail performance independently. And I'd like to draw an analogy in terms of traditional software development. We have pretty extensive suite of tests, CICB infrastructure and the kind of ability to when you make a code change, ship it with confidence when all tests are passing. And I think one of the challenges we've seen as an industry working on ML is that these dependencies are much more coupled. And so you can maybe improve one scenario and silently regressed another one. And so how, how have you all thought about building this kind of model validation infrastructure, you know, with, with the long tail in mind, what are some of the lessons you've learned along the way?
Dr. Yangbing Li (39:00): I think Russell, that is a excellent point. I, I do think ultimately we need to have sophisticated multiple modalities of testing that give us both that precision results when you want to understand a particular change and the corresponding alpha, but also a system level end to end performance and results. So, so it's really not a one particular mode of test is this comprehensive modality and you know, how to best apply those. So for the particular problem you raise, I do think it is extremely important that we have a framework that allow us to test one thing at a time and make sure the test is high quality. And give us that clear signal. We, we talk about it. So for example, you know, we do for our perception system, there is a custom designed test approach so that, you know, we can have scenarios that focus on very task-based tests and give us that clear signal of, you know, the change of where we're making. So those are very, very focus. And certainly we also have a set of comprehensive test approach from offline online, you know, bench base to give us that system view, but definitely, you know, you need to have that full spectrum of testing available. Okay,
Sammy Omari (40:31): Thank you. I'm being, and to add on top of that, I think as an, as an industry, if we look at machine learning systems, I think there's a key trend to start doing more and more early fusion. That means basically starting to combine different sensing modalities very early on in a single model, and then basically have a common set of representation and then different heads as regressed over different attributes. For example, detections prediction, some agent attributes, you name it. So in this context, like as, as we start basically reducing the number of machine learning nets in, and basically it didn't visual nets actually become larger because they're doing more tasks as an industry, or actually more as a community, as machine learning community. We do, we need to find new ways. Have we even structured the teams around creating those large networks and in particular, how to do these trade-offs, you know, for example, in terms of error box, right?
Sammy Omari (41:29): Because if you do have like one end to end system that say detect agents, but also some prediction, photos, agents, you know, how do you make sure that, you know, for example, it can improve predictions, but at the same time don't necessarily regress into, into detections, right? And so there's a few very practical aspect here. And so one is for example, that you try not always to optimize the whole network, but maybe only two fine tuning. And, you know, once individual teams fine tuned the different heads, then you, you do a full sweep over the full network, right. And then really try to understand overall performance. But I think as an industry and figuring that out, we are still at the very, very early stages how to do that, right? Because the reality is, you know, you kind of maybe optimize your performance of your method in this particular aspect.
Sammy Omari (42:14): And then, you know, it starts progressing into a, in a, in a, in a, in an area where you actually didn't expect it to regress because then at the end of the day in the machine learning system, it's been an old Tecogen. So, you know, through backdrop, you might as well like impact the system in ways that you didn't expect you to ahead of time. So I think in order to really patch those regressions, the way that we think about this emotional is basically twofold. One. We need to understand this at scale. Like even if you talk about, you know, having dedicated test data sets for the long tail, it's still, it's still going to be at the end of the day, we really need to look at the distribution, right? The distribution, for example, if break taps or at, you know, distribution of different metrics, right?
Sammy Omari (42:54): Because individual pass fail criteria at scale, if you start to making at millions of miles or hundreds of thousands of miles start making less sense, right? So the way that we think about it is, Hey, you know, did you in aggregate in challenging scenario, maintain our safety and our ride comfort. For example, these are just two very simple metrics. There's obviously need to have a lot more complex metrics, but at the same time, we do have certain situations where we know we definitely do not want to re regress, right? So for example, we've traversed hundreds of thousands or millions of traffic light intersections, right. And we know because they are safe to drive, it didn't disengage, but if you did the right thing. So for example, we did detect a green traffic lights. And in fact it was actually green and we traversed or inversely, it was red.
Sammy Omari (43:41): So we decided to stop when we were collecting the data, right? So we can actually use this as very specific unit test pass, fail, binary pass, fail test to say, Hey, in the past, we've traversed these intersections 10,000 or a hundred thousand times, this was the I put, so we better not regress overdosed and dishonored really binary decisions. So at the end of the day, we kind of do both, right. You have for each head need to have a set of basically almost unit tests, but in the context of a machine learning system. But at the same time, we also need to have like aggregate metrics very, instead of looking at individual cases in a binary way, we look at, in aggregate, how does the distribution of a particular metric look like?
Russell Kaplan (44:22): It's, it's really interesting because that trend towards early fusion, it tends to make it harder to have clean abstraction boundaries, you know, between teams work on things. And so in many ways as the machine learning community, you know, we're kind of taking more best practices from traditional software engineering. And there's, I think been this historical desire to maintain thin interfaces, have clear boundaries and, you know, have people be able to kind of run in parallel optimizing things, the challenge being that, you know, if from a machine learning standpoint, what we're seeing is that, no, you actually want to fuse this stuff earlier. It, it almost places a higher premium on the infrastructure, so that you kind of have that unit testing. You can have that validation because if the systems half of the couple for optimal performance in, in some sort of fusion type setting, then yeah.
Russell Kaplan (45:09): That validation just becomes so, so critical. And so I would love to basically tie that validation piece into how, how you think about it, both sort of pre deployment and then especially kind of like post deployment in the loop. And I know for example, it, Shivah gonna keep trucking is operating at tremendous scale here. And so how are you thinking about, you know, what you validate before you send out and then, you know, using that massive fleet of, of deployments that you have to continue to, to kind of refine and improve and then kind of get the next iteration quickly.
Siva Gurumurthy (45:42): Yeah, it was great points made by everyone here about validating. The painting said test said and before deployment. So one key piece for us is that the regression is actually real. So when you know, the overtime as you're training that I was, we kind of keep adding training data enrich with more examples where the model fails to perform from the previous scenarios. But as if there was, it also starts under presenting more common scenarios in, in places. So, so if here is where the, the versioning of models and versioning of training data becomes, and actually the test data also becomes important. As we have observed examples where, you know, we would start doing really well in low light situations when, when, when people are wearing like, you know, vests that have reflective gears. And then, you know, the models would actually work detecting cell phone.
Siva Gurumurthy (46:41): Although their cell phone is visible on the, on the phone, outside on the, on the screen, it would still detect that cell phone is working, but then that model would actually skip something. Yes. Right. So how do we make sure that the regression doesn't happen and, and it has to be happening at scale, you know, one more time over, you know, over a year you would have like 24 different versions of model and 33 different versions of test sets. And one thing is indexed towards other, you know, like certain scenarios other than one is indexed to a certain scenarios. So it's important to get all of this tooling into an automated engine that kind of like spits out, Hey, like, you know, vendors, where does this model fail? Where does this model perform better? And not the guarantee that it's physically improving over the last, you know, and number of last and models, it's actually a pretty trick, you know, it's tricky for us.
Siva Gurumurthy (47:39): In fact, when we, you know, it seems to work well in the cloud where it's says predictably, that this is going to be a strictly, but my model, but when you are deployed to the edge in the large fleets, you see that it's not, you know, it may not necessarily obey the key here is that sometimes the false positive kind of occurred at a faster rate than the two positives are. So your model starts. So if you're looking at the experiments, say for the first, say 10, you know, like two weeks or so, you would have started getting enough false positives, and you would assume that your model isn't doing well. But the reality is that the group has to hasn't even occurred by then. So you have to figure out how to not Malays the models that are being in, you know, in the field for, for the same amount of time and be able to like, you know, transpose that over time and figure out, okay, this is an AB test that this model, you know, planning for the same amount of duration produces the similar.
Siva Gurumurthy (48:39): So it kind of like, this is where, like the traditional software engineering practices of like versioning, like, you know, of automating and making sure there are no bugs and it all comes to fruition to help us get better. But, you know, it's the it's little wild, wild west in the sense like the, I think everybody is trying to do what works best to form that in my mind. And, and there isn't like a traditional set of practices. Like, so, you know, you do, you know, your, you know, your modern development years old, you, your, you know, like code reviews, model reviews, and yourself, you should add your test reviews. Like everybody's throwing a lot of ideas and see what sticks to this ML engineers. And that, that also off drives that 3d model. So overall, you know, the point I want to make is like, I think as the misfit was like, we will Elaine on the best practices already from this conversation, this my data store, this, like, you know, the, the fact of like, you know, changing the hardware confident and young beings, point on like bringing good software practices, all of that will converge to a certain goal standards that I believe is essential and it's going to help all of us.
Dr. Yangbing Li (49:52): Yeah. I think this is a, you know, the, where I felt, wow, this is quite a familiar territory coming from cloud dealing with large amount of data and analytics. So I do think lots of the, the good practice we are using here at aura, you know, first, you know, was this giant fire hose of data. You know, how we make sense of it, how we can ingest a most efficiently, how we can search and query to most efficiently. Then there is the other aspect of, you know, how we can handle that, you know, Hondo the reverse scale and complexity, you know, applying a loss of the well, use the data lake technology to really homey and better analytics. Then there is the, you know, great work we've been doing on data and labeling, you know, applying a mallet to make that process allow more efficient and, and the intelligence. So, so to me, this is really about looking at the entire data life cycle and apply a lots of the well-established technology into this space. And that is another key ML acceleration point.
Russell Kaplan (50:59): Absolutely. I think there's, you know, really interesting common threads across these points for sort of improving the rate of innovation of ML teams. And it seems to, it seems to really start at the kind of infrastructure level for each stage of the life cycle and making sure that teams can iterate quickly, keeps going iterate in parallel. So that ML teams, hardware teams, validation teams, can all be kind of doing things kind of in parallel while continuing to make the system better. And that keeping the sort of long tail challenges of machine learning top of mind throughout this process from dataset to deployment op is totally necessary for reaching where we want to go. So thank you all so much for your time and insights. I think this was a really interesting conversation and I really enjoyed it. Thank you.
Speaker 1 (51:56): [inaudible].