Panel: Managing Compute With MLOps to Handle Growing Datasets & Model Sizes
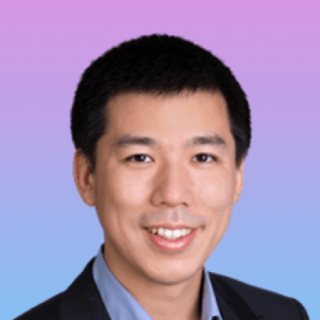
Jack Guo is the Head of Autonomy Platform at Nuro, a robotics company that aims to better everyday life through robotics with its first application in autonomous goods delivery. Autonomy Platform consists of simulation, evaluation, data platform, data science, ML infra, ground truth eng teams and data labeling operation team, and its mission is to build tools, infra and services that accelerate the development of autonomy. Before joining Nuro, Jack was managing the machine learning infrastructure team at Twitter, powering key ML applications like ads prediction and feeds ranking. Jack earned Bachelor degree from Tsinghua University and Masters in Electrical Engineering from Stanford University.
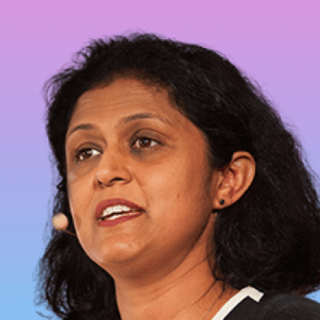
Anitha Vijayakumar is a Technical Project Manager on the TensorFlow team. She got her engineering degree from India and a Masters in Computer Engineering from UCLA. This is her 10th year at Google and is most recently leading program management for the TensorFlow Machine Learning Ecosystem.
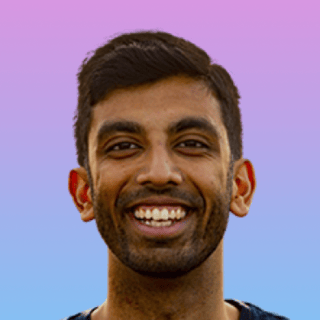
Vishnu Rachakonda is a machine learning engineer at OneHot Labs. At OneHot, he helps build and maintain production machine learning systems that reduce administrative waste in healthcare and provide billing capabilities to thousands of doctors nationwide. Vishnu is also the Head of Operations and Content for the MLOps Community, the world’s largest online hub for MLOps practitioners and enthusiasts, and co-hosts the community’s podcast “MLOps Coffee Sessions”, whose past guests include Jeremy Howard, D. Sculley, and other industry luminaries. Prior to this, he was the first machine learning hire at Tesseract Health, a 4Catalyzer company focused on ophthalmic imaging, and a teaching assistant for the spring 2021 edition of Full Stack Deep Learning. He obtained a BS and MS in bioengineering from the University of Pennsylvania.
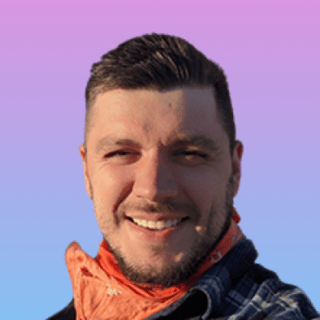
Oleg is a co-founder of Outerbounds, a company building the modern, human-centric ML infrastructure stack based on open-source tool Metaflow. A startup veteran, before Outerbounds he spent most of his career either getting ML from zero to one at companies like Headspace and Alpine.AI, or building tools for data scientists so they can do that themselves, most recently at Tecton.
Hosted by MLOps Community. Panelist to be announced soon. Demetrios Brinkmann, founder of MLOPs.community leads a panel managing the increasing compute requirements of AI models, whilst striking the right balance between flexibility for experimentation and stability in production. As enterprises collect more training data, and in many cases label it with Scale AI, they face the challenge of their models growing in both size and compute complexity. Join this session to learn how companies can develop robust and maintainable pipelines to ensure that ML experimentation remains possible, despite increasing model sizes and longer training times. This session will also cover compute for lifecycle phases from experimentation to scaling (with Metaflow, TFX, etc.) pipelines that are ready to deploy to production, including via microservices.
Demetrios Brinkmann (00:38): (Music)
Demetrios Brinkmann (00:43): Hello, everybody. This is an exciting session. I've got some superstars with me today for this TransformX panel discussion on MLOps and AI Compute Infrastructure. Before we jump into some serious questions for the panel, let's get an idea of who we've got with us. Starting out with my man Vishnu, who helps me in the MLOps Community, he is also working at Onehot Labs as an ML engineer.
Demetrios Brinkmann (01:17): To his right, or probably my right, you have Oleg Avdeev and he is working at the startup that is known as Outerbounds, you may know them because of that Netflix open source tool called Metaflow, and you may also just know him because he's a great contributor in the open source community. To his right, we have Jack Guo who is working at [Nuro 00:01:52], which is doing some incredible stuff. Jack, happy to have you here. I'm really excited to hear some of your thoughts on everything.
Demetrios Brinkmann (02:00): And to finish it off, we've got Anitha who is coming at us from the Google Brain TensorFlow Team. I'm super excited to talk to you all, and I know you have some very, very interesting things to say, so let's get into it. I'm going to lob one over to you, Jack, because I just see you on the front of my screen looking great. And I want to ask you about MLOps and how you look at Nuro. How do you say when something is successful? What are the metrics that you are looking at as you are trying to figure out when something can be successful?
Jack Guo (02:50): Great question. So Nuro is building self-driving robots to delivery goods, so we have a lot of deep learning models and we have a lot of data to feed into those models. And the success criteria that we'll consider for MLOps is really to shorten the turnaround time of the entire cycle of data collection, mining for interesting scenes, doing the model development and deploying the model back to the robot and collect more data and mine more interesting data from them. So the shorter the entire end-to-end cycle is, the better the productivity is.
Demetrios Brinkmann (03:34): That is so awesome. I know we don't want to just end it right there, and I'd love to hear Vishnu's take on this, because Vishnu and I talk at length about these kind of things all the time in the MLOps Community. I realized that in my haste of wanting to introduce everyone else, I forgot to mention that my name is Demetrios Brinkmann, I lead up the MLOps Community. It's something that is pretty cool, and you might want to check out if you are into MLOps, but Vishnu, let's hear it from you, man. What do you think?
Vishnu (04:07): Hey, Demetrios, long time no see. First off, thank you so much to scale for running this conference and for the opportunity to be here and thank you to my fellow co-panelist, Jack, Oleg and Anitha. In terms of what you just shared, Jack, about how Nuro goes about thinking about the success of MLOps, I think that's a great definition. What we all want to do is reduce the time from thinking about creating a model to actually getting that model into user's hands and into our company's bottom line.
Vishnu (04:38): Well, I have a question for you actually, is how you guys track that numerically, if you do at all? Demetrios and I actually had a chance to talk to Stefan who is an engineering manager at Stitch Fix, and helps run their ML platform team there. And we had a great conversation on our podcast about how it can be tricky to actually track that time from thinking about the model, so to speak, and actually getting into production. And I'm wondering if that's something that you guys quantitatively assess.
Jack Guo (05:07): Do you mean track model performance or do you mean track end-to-end latency?
Vishnu (05:12): End-to-end latency.
Jack Guo (05:14): Okay. Yeah, that's a very interesting topic. So first of all, we're definitely trying to move towards that direction and definitely echo that this is something that's quite not very trivial to do. But if we look at each component, we can track them individually, at least. Like for example, when you identify some scenes, you want to get them to be labeled, this may involve some operations, some human in the loop labeling, and you can track the turnaround time, given certain amount data, like how long it's expected to get it labeled.
Jack Guo (05:47): And for model training, you can do something very similar, you can try to optimize with distributed training to make it faster. But yeah, you have a pretty good understanding of how long it takes to train a model on the amount of the data that you typically train a model on. And the remaining part like evaluation, that should be fairly, fairly quick. So if you add those all up together, I hope we can have some dashboard to show that, but we don't have it today. This is definitely direction that we're moving towards.
Demetrios Brinkmann (06:18): So good. Anitha, I'm wondering about TensorFlow. I see you want to jump in there, go for it.
Anitha Vijayakumar (06:25): I just wanted to say that, what we do is, because of the scale at which the company does, each team does have their own way of tracking and figuring out what is the best metric that they want to track on, accuracy and performance are definitely stable stakes. The new model has to perform as good as the previous one when you're upgrading things into the production workflow.
Anitha Vijayakumar (06:50): But there's lot of other things that we don't have standardization and would love to standardize on is, "Hey, model version architectures, hyper parameters, how long did this train? Who trained it? What went into production?" And of course, efficiency and how resource-intensive it is when you are training a model. Sure, you can get a little bit of accuracy improvement, but if it doubles the training time, then is it really worth it to actually deploy this particular model to production?
Anitha Vijayakumar (07:19): There are many parameters, it's really hard to narrow down on one. I think Jack put it nicely and said, "Hey, I want to reduce the iteration time." That is, if you do go with that approach, also, there are many nuances, which iteration time are you're going to reduce? How are you going to reduce it? At what cost? And to on.
Oleg Avdeev (07:41): Yep. And just to add to it a little bit, and I've seen a good number as someone who doesn't necessarily, at least in my current role, work, build models in production, but I've talked to a lot of companies and at Outerbounds and previously I was at Tecton, which is a feature store company that talks to a lot of folks in this position.
Oleg Avdeev (08:00): I think one important they mentioned there is also, I think there are like two types of companies that use machine learning. Some of them have pretty much one magic, special, golden cow of use case. I was in that tech before, it's like 90% of your ML business is about predicting clicks. And this is the model that makes the most impact on the business. No matter what, there is maybe something else, but this is it.
Oleg Avdeev (08:28): In this case, it's actually relatively easy, relatively clear to use. Like a quarter over quarter, the same team creates new durations of the model that predicts clicks, so you can easily measure their velocity as a team. And then there is another aspect, there is a lot of companies that have much more diverse use cases for ML. I think actually with [Mike Hunter's 00:08:52] Netflix was one example, whereas there's long tail of all kinds of business cases, all kinds of new models getting built, new aspects of machine learning being introduced in small bits and pieces all over the product.
Oleg Avdeev (09:05): So it's not necessarily the team that, I don't know, works on some kind of predictive input field, somewhere deepened settings. They don't necessarily work for years and years to improve accuracy on this one thing, I think in this case, it's much to come to measure, it's getting more into kind of general engineering management metrics, where you try to figure out how long it takes to form a team around a problem for this team to ship something to production and build production infrastructure around it, and most importantly, how much time they spend to keep the lights on after this has been shipped, and to support this and how much this distracts them from working on new exciting things that also bring value to our business.
Demetrios Brinkmann (09:55): Super point, Oleg. That's awesome, it makes complete sense to me. I'm wondering now about automation, and this is something that we also talk about in the MLOps community quite a bit, especially when you look at papers that come out of Google and they talk about the different maturity levels of teams who are using ML. And a lot of the way that the maturity levels go is that you have more automation, the more mature you are.
Demetrios Brinkmann (10:27): And so I'll kick it over to Vishnu and ask, how do you feel about this whole automating something and becoming more mature, do you feel like there is that give and take, again, when it comes to automating for automation's sake or is it always a good idea?
Vishnu (10:54): It's a great question. Automation is sexy. Automation is appealing. So let's get that out of the way. The perspective that I bring to the table is one of an ML engineer at pretty small startups. My co-panelists work at incredible organizations that are doing ML at a different scale, so I'll let them talk about their level of maturity. But sitting at a small startup, thinking about how to get machine learning into production and how to have the windmill that allows you to go from idea to model and production quickly, I found that thinking about MLOps maturity and leveraging automation in your process early on to be very, very helpful.
Vishnu (11:37): Because it helps you avoid a lot of the pitfalls that come later on as your team grows, as your needs grow. If you have automation baked in from the beginning, it really helps you scale in the future a lot better. That's been my experience, but I'm also cognizant of the fact that it is very dependent on different contexts and that there is different automation that's required across the spectrum of, to quote Martin Fowler, data, model, and code.
Vishnu (12:07): So at my current employer, it's a lot harder for us to apply automation to the data that we obtain, because we get it from external service providers in the healthcare industry who don't necessarily have maturity on their side in terms of how they think about data feeds. So we have to do a lot of manual auditing at Onehot Labs to ensure that our data that's going into these machine learning models is actually the kind of data that we want to be using.
Vishnu (12:34): So I think automation, particularly for early stage companies, is a good thing to aspire towards. It's maybe not exactly what you want to only build towards.
Demetrios Brinkmann (12:44): So I'm looking at you, Jack, and I'm wondering how the process was where you're at and how much automation you would say you've been able to leverage and how that has been.
Jack Guo (12:59): Cool. Yeah, just to echo Vishnu's point. I think automation is very sexy that saves a lot of the friction that may hamper the ML engineer productivity, but also sometimes it's very important actually for ML engineer to have certain touchpoint to check, to make sure, "After I dump the data, I want to visualize it. I want to make sure the logic is correct before I go to training. And the moment I get trained, I want to look at all the metrics."
Jack Guo (13:32): Because sometimes if you fully automate to say, "Oh, if the metrics are better than this, I will deploy the model." It's hard to have that logic to be super comprehensive. You may be looking at 20 different metrics across different dimensions and make a judgment call, "Is this model better than the other?" So what I see in the practice is probably like a hybrid approach. You try to automate for the areas that should be automated. Like for example, ML engineer, shouldn't worry about a lot of the nitty-gritty details or babysitting the entire training process. If there's something happens like certain errors they should be pushed to you as opposed to you probing into it.
Jack Guo (14:11): So those unnecessary touchpoint should be reduced while there's certain touchpoint we still want a human to be in the loop. But we try to make that process as easy as possible. So maybe it's just like triggered by a button click, and then the following steps can continue.
Demetrios Brinkmann (14:27): Ooh. So you make me want to ask another question and I see Anitha was taking some notes. I want to ask about where you feel those touch points need to be. Is it something that should be standardized or is each use case specific?
Anitha Vijayakumar (14:47): I do like the way both Vishnu and Jack just added. I think automation is cool and it makes a lot of things easier, but we have not reached a stage where we can 100% automate everything, the end-to-end workflow. Somebody writes a model, you click a button, boom, it's there, and everybody's using it. We are nowhere close to that world, but there are some huge benefits, if you take data for example, feature extractions, you want to label this data, or you want to get analysis tools. Are there biases in your data?
Anitha Vijayakumar (15:21): All these are much better if you're automated, or if you have standardized tools for this. I think that we are a little far from standardization yet, even in our company we do have different teams having their own version of managing this and not actually being standardized in this. So I think a combination of a human in the loop with automation for where ML is today is probably the best solution at this point in time. But I'm pretty sure that more, and more, and more tasks will get automated over time. And maybe we will get to a date where we press the button and everything will just work automatically.
Demetrios Brinkmann (16:00): So Oleg, Metaflow?
Oleg Avdeev (16:07): 100%, nothing to say against automation as usual, just maybe coming from experience in smaller companies, sometimes you've got to be mindful about where you're spending the efforts. And if this ML model, or this process, or this ML-based product is going to be really the thing you're going to invest a lot more in the coming years, or maybe it's something, a hypothesis that you try today and you give up on this in two months and you have to build another pipeline, make sure ... I don't know, maybe there is some rule with someone and you're like, "Do something three times, then maybe it's time to automate it" but first make sure you've gone through the process manually a few times.
Oleg Avdeev (16:50): Because every automation also adds some kind of factor of tech depth or keeping the lights off if this automation breaks, someone has to fix it occasionally, that means you need more people, you need to spend more time on this. The anecdote maybe from software engineering, I've actually seen one team even onboarding new, yes, it was fully automated on their team and they would ... I think they're using Terraform to add users to their GitHub account. And when they joined, some automation broke.
Oleg Avdeev (17:25): And they couldn't add me to the repo for like two days or something and you look at it and I think about, "Okay," how much you probably kind of blew through your ... Like, all the time that has been saved for you by this automation, you probably will make up for it in like years, 2030, by just losing two times of two days of productivity for several engineers to fix this. So just to be a little bit contrarian there, you've got to be mindful of those things as well.
Vishnu (17:54): That is a really good story, Oleg. I can't think of a better one that summarizes, I guess, the pitfalls of automation. And to kind of summarize what I learned from a senior engineer I used to work with is automation as a mindset, not as a strategy, because as Oleg pointed out, it can really increase the brittleness of your system in unexpected ways.
Demetrios Brinkmann (18:13): Ooh, Vishnu, giving us some food for thought there. I like it. And Oleg, it sounds like you've been reading the MLOps Community blog, because we are strong advocators for that start manual first and then automate. I think that is something that is huge. You talked about a war story and I cannot let this opportunity go by without asking for more war stories.
Demetrios Brinkmann (18:41): I would love, Jack, if you could give me a war story. It doesn't have to be about automation, but it can be, but just tell me when something blew up in your face, MLOps-related.
Jack Guo (18:54): Something goes wrong with MLOps. So let me think. Maybe sometimes it's not like a completely broken in some sense, but sometimes we do find it takes a very long time for like an engineer to build a new pipeline to actually build it. Ideally, you extract a new feature, add a new feature to your model. It should be relatively simple, you just identify the source, you extract the feature, you should be done, like everything else be automated.
Jack Guo (19:33): But what we found now in practice, there's a lot of frictions in between. You may train a model, you get [non-laws 00:19:41], you need to debug the non-laws. It takes a lot of time to do that, then we build better tooling to identify, to help the ML engineers to debug those type of things. And there might be some frictions about when you scale up the batch sizes, maybe your model doesn't converge anymore, things like that. So there're a lot of frictions throughout the process, so you just need to solve as you encounter them.
Demetrios Brinkmann (20:09): I was hoping to hear a story about a model gone rogue, that lost millions of dollars, but you gave a very diplomatic answer and I like it, your boss probably loves that too. So, Anitha, what do you got for us? You got anything?
Anitha Vijayakumar (20:25): Yeah, not a model blew up, not as exciting as a model went rogue or anything. But the MLOps problems, what we have seen regularly is people build a model, it's running, everything looking fine and they leave or they change teams, or they go somewhere else. And then what data was this model trained on? What framework versions was this working on? What hyper parameters was used to train this?
Anitha Vijayakumar (20:53): All the problems that we have faced in software engineering, those kind of blow up for us at ML. Not having proper version management, not being able to see the whole trace about the whole model, what is being trained? What are the graphs, training duration? Who trained and what went into production? Everything's working fine, nothing's ... But the person had all of this knowledge in his head and he just moved out.
Anitha Vijayakumar (21:21): So those are some problems that we have tried to build two link for, but we've not yet standardized and scaled it on a big level. And we've not had this problem yet, but I can also see, you've trained your model on some dataset and licensing for that dataset is over, you no longer have access to that data. Now, can you use that model as it is? Do you know which all models were trained using this dataset? And can you take them out because you are no longer licensed to use that?
Anitha Vijayakumar (21:51): So these are problems, I think where MLOps is going to become very, very critical and having ways to understand that will be important.
Demetrios Brinkmann (22:01): A follow-up question on that, and then I'll go to Oleg because I'm just going to give him an easy layup for that one. But a follow-up for you, Anitha is are these problems solved by getting the right kind of tools or is it harder than that?
Anitha Vijayakumar (22:24): It is harder because we don't have standardized tooling to do any of these things, but we've solved it for software engineering. We have millions of lines of code, hundreds of thousands of engineers writing this code and we still have an [iCI 00:22:43], configuration management system, we have versioning of code. We have all of those and we've solved those problems.
Anitha Vijayakumar (22:48): It is harder for ML because we don't have those things set up in place. And each team when they face this problem are trying to invent something that works for their use case, which will solve maybe for 30 models. And I was just listening today that every time the problem scales by a factor of 10, you pretty much have to reinvent the tooling and everything. So I think once ML models, we have hundreds of thousands of ML models trained on hundreds of thousands of different data sets or even more, then the problem scale itself is different. And we don't have a standardized way to solve that ad scale today.
Oleg Avdeev (23:24): Yeah. And I don't think also there is a very standard set of tools that just solve this problem. I just wanted to add that even like on personal level, this is why I really like this space, like MLOps and building tools for ML practitioners. Because I worked a little bit as a person who creates models and I worked in the companies next to them, specifically for them. And the thing that gets me the most is that when there's a team of data scientists improving the model by percentage points over and over, week after week, month after month.
Oleg Avdeev (23:58): And then as a info person, you come in there like, "Oh, wait a second. We're not logging this feature somewhere, let me fix it real quick." And then it just blows away all the improvements from the model, it's like 5% improvement and accuracy or something. And that makes you feel good as a software engineer, but makes you feel bad because I guess the data science team or machine learning team didn't have enough tools to monitor those things. And it may be a little at disappointing.
Oleg Avdeev (24:25): I give you not like two ... I will not name names, but two people from completely different big tech companies from the sharing economy told me almost word for word, exact same story. When their pricing model for several months had their labels switched completely like zeros, or ones, or something and no own noticed, and of course, they noticed and freaked out. Like it's same story to big tech companies, it was like thousands of very smart engineers. So that tells you something that, I mean, probably tooling is still not quite there. There's still a lot of things we can do as ML for people.
Demetrios Brinkmann (25:04): Oleg, giving me those war stories I was looking for, thank you for that.
Oleg Avdeev (25:10): Well, it's easy if it's not my war story.
Demetrios Brinkmann (25:16): Vishnu, I saw you had a comment. You want to give it to us?
Vishnu (25:19): Yeah. Yeah. I think what Oleg brought up there, in particular, that war story reminded me that one of favorite areas of emerging infrastructure is around monitoring. I think that there is a lot that can be ... From the research side, I've been seeing a lot of really interesting papers that talk about some of the challenges of machine learning models in production.
Vishnu (25:42): For example, there was a really exciting paper last year from a number of authors like Google Brain, including [D. Sculley 00:25:48], that talked about some of the generalization problems that are faced by models in different trained test settings. Another paper from Microsoft talked about backwards compatibility as you evolve your train and test set, being able to ensure that a model that you thought was doing well on a certain set of examples is continuing to do well.
Vishnu (26:12): These are questions that I think researchers are finding subtleties in how models, and these complex models, and data sets are responding and I think that there's an opportunity. Not every company is going to have the bandwidth or the time to read these papers and encode the best practices into their monitoring. And that's where tooling and infrastructure can really help. And so I think I'm really looking forward to in 2021, and 2022, and beyond to seeing where that particular portion of infrastructure evolves.
Demetrios Brinkmann (26:45): So this is an interesting one, and I want to throw it over to you, Jack, because there's something that you said earlier about where the human touches the system, and where you're getting alerts on things, and then where you're automatically retriggering. And so I've heard a lot in software engineering, and it's starting to crop up in MLOps too, about going into alert hell and just getting too many alerts, and so then you're desensitized. How do you deal with that?
Jack Guo (27:16): Sure. I guess it's probably we want to tune the alert to be high precision. Basically, whenever alert is triggered, you want to make sure it's useful, there's some action items that's expected from this alert. Sometime it would be great if you can also provide a link that the engineer that can click link into the area that they need to look into and do some actions.
Jack Guo (27:39): So I think that's the key. So if you just blindly send a whole bunch of alerts, then people will just stop looking at them and it becomes useless.
Demetrios Brinkmann (27:49): Yeah. That makes complete sense. And now, the next thing that I'm wondering, and Anitha, this one is for you, which always comes up whenever we talk about monitoring is how do you choose the metrics to monitor? How do you know which ones you want to monitor out of, sometimes you're dealing with thousands or tens of thousands of metrics, so it can be huge. And you're trying to figure out what is the most important?
Anitha Vijayakumar (28:17): Metrics, you will have to see what your use case is, but at a high level, you can see, for example, how many pipelines are run in a time window, how many models are trained. What is the time to run these things? What is the accuracy numbers that you're looking at for these metrics? Are they hitting those particular accuracy the more accuracy numbers, AUC numbers that you're looking at for a particular model? And how long is the whole training itself taking?
Anitha Vijayakumar (28:56): Those are, I think, performance metrics, time to run these metrics, efficiency metrics. There's a whole set of things that you can outline. For us, for some cases, depending on the usage, enduring inference, the tail latency becomes very important. So how is the latency like? You run a search query or something, you cannot wait for five minutes for it to respond with the result. So a lot of those things are use case-dependent, but performance, and efficiency, and latency at a high level, these are things that we care about a lot.
Oleg Avdeev (29:39): Yep. Just to add to it. I agree, and also I want to add, this is exciting topic, but some responsibility is on us people working on MLOps startups, there's crazy amount of monitoring startups out there. And I think one thing there is metrics they demo really well, so if you're building MLOps product, it's less exciting to talk about pipelines and SQL queries, and you can show metrics and fancy distributions and things drifting in different ways, but it's much harder to build a product that has a coherent story about what metrics you should actually be looking at and how that connects to business matters.
Oleg Avdeev (30:18): Does it actually matter if this model goes a little bit off, like how much money do you lose or make, if this model was more accurate? I think this is pretty much still an unsolved problem in many ways, because again, you can plot fancy distributions and shifts and have some kind of very interesting algorithm to detect anomalies, but maybe in a lot of those cases, the answer is just to retrain your model more frequently. In that case, you don't need a fancy product for this. So I just wanted to ... I have to remind myself as someone who works on tools for ML practitioners to build things that not only demo well, but also really somehow translate into end of the line business benefits.
Demetrios Brinkmann (31:06): And Oleg just got blacklisted by half of the new MLOps companies that are coming out. That's such a good point, though. And so I'm wondering, Jack and Vishnu, I'll throw it to Vishnu first because the automatic re-triggering, when you're getting some kind of drift that's happening, how essential is that to set up in your mind, Vishnu?
Vishnu (31:35): I think that's really context-dependent, problem and use case-specific. I mean, in the realm that Onehot Labs works in, which is healthcare and healthcare encounters, the minute that we sense that something is off, we really need to take a break and potentially pause our predictions, our inference engine and say, "Why are things behaving in a way that we don't expect?"
Vishnu (32:00): Whereas in another setting where there's perhaps a little bit less of a risk in terms of inference, you can't afford to simply retrain and deploy the model again. So I think that's really context-dependent. And I think to Oleg's point previously about the attractiveness of monitoring and charts, as opposed to fundamental sort of ML engineering work, I think that's a point that I hear loud and clear. And I guess it'll be interesting to see how tooling companies like Outerbounds can actually help us really do our jobs the way we should, almost.
Vishnu (32:38): I think sometimes that's the underlying question there, around writing the good SQL queries, thinking about pipelines or AOM. I mean, that's what I'm supposed to be doing, but maybe there's a tool or they can develop to help me with that.
Demetrios Brinkmann (32:54): That's nice. Yes. So Jack, any thoughts on this one?
Jack Guo (33:00): So on the topic of drift, yeah, I agree with Vishnu, it's very context-dependent. In a self-driving robots, self-driving car space in general, usually if we want to operate in an area, we collect a lot of data and get them labeled. And we also create our evaluation framework data to be coming from that area as well so like whatever metrics that we get from this evaluation system, that's predictive of our on-road performance if we deploy the robots in that area.
Jack Guo (33:36): So this is maybe a little bit different from a traditional. You have a service, you get a lot of live traffic and live traffic distribution can change. So in our case, it's a little bit of slightly less of a concern, but we also refresh our evaluation data once a while to make sure we're still evaluating on the right thing and minimize the drip as much as possible.
Demetrios Brinkmann (34:02): So we're running low on time. I do have a few questions that are going to piggyback off of what Vishnu was talking about and bringing the best practices to ML. I know not everyone's a fan of the phrase best practices, but I'm using it, sorry. That's what we're going to talk about real fast, because a lot of tooling companies that I've seen come out are saying that they bake in best practices into their tools.
Demetrios Brinkmann (34:31): So Oleg, I'm going to throw this one at you first because you're working in a tooling company. What exactly does that mean?
Oleg Avdeev (34:44): Well, if I had a perfect answer, I mean, that would be great for my company. It's really, I mean, you draw on experience building those tools. You definitely draw on software engineering, best practices, even though I should say, I feel like there is a bit of a, I don't know, inferiority complex in MLOps world. It's like, "Oh my God, software engineers, they have this figured out and we poor data scientists we have to follow in their steps and adopt those practices."
Oleg Avdeev (35:16): But I've been doing software engineering for a while. All those practices in software engineering, they change year over year a lot. When I started my career and I'm not that old, it was completely different field. There was different tools for testing, different best practices for deployment. People even were doing source control differently. We were not using GIT, we're using much worse.
Oleg Avdeev (35:41): It's not a static target, and I think this will evolve. You learn from everyone you can and other companies, and try to come up, see what works, what doesn't, see what sticks. And I don't think that is I have a formula. And I also keep in mind the size of the company and size of your use case and the team, can they actually ... Like to the previous point on automation, is it wise to build something super magical, super great, cathedral where everything is automated, but you require, I don't know, 50 people to support it in production?
Demetrios Brinkmann (36:21): Speaking of requiring 50 people to support something, Anitha, how can we as engineers escape the common pitfall of over-engineering something like Oleg was talking about?
Anitha Vijayakumar (36:38): Yeah, first of all, I want to say, I think we are relying on a company like Outerbounds and others to actually provide these best practices for us, provide these end-to-end infrastructure for, let's say, version control of machine learning, or trace it back to the data it was trained, or models got pushed on what data did they get trained? Or different between two different runs. So many things. Data provenance, what are they training? Where are they coming from? Where are they going? These are all new things.
Anitha Vijayakumar (37:10): And we clearly can do with some standardized tooling that we can just use like it, or use that we've done. And I'm sure we'll get there. It's not over-engineering, I think most of the times in terms of model development, I've seen over-engineering happen. You are getting a small improvement in accuracy, but then you're spending double the training time to get that accuracy, cost versus more risk. Cost versus benefit analysis is not done all the time, you just want to have the best model and you just want to have the best, but you're paying a price to get to that best model.
Anitha Vijayakumar (37:51): And I've seen a lot of over-engineering happen in that space. In the MLOps space, I would like to see more over-engineering happen over here in the sense, more engineering happen over here. And I think this is like a greenfield right now, it's a gold mine. Any tooling you build will improve the lives of these people, of all of us, actually.
Demetrios Brinkmann (38:19): Brilliant. Jack, I'm going to throw it to you and ask the same question. How do you keep from over-engineering your systems?
Jack Guo (38:28): So my approach would be, take one use case and try to solve that use case very well, but also at the same time, you also understand what other use cases requirements are. And when you're building the tools or solutions you build in a way that's as general as possible, but the key is really to solve one problem really well.
Jack Guo (38:51): So I think one way you do that, you probably can build something that's very useful. And on avoiding over-engineering, just echo what Anitha said. You need to just do the cost benefit analysis, basically how much effort it takes to build this infrastructure, and then how much effort it takes to maintain that. So usually, the maintenance cause is sometimes higher than building the tool itself. So yeah, sometimes you just need to take a look at the case by case basis and make a decision from there.
Demetrios Brinkmann (39:28): Incredible, thank you all for this super informative session, I've learned a ton. And as I expected, it was exciting, it was interesting. You all threw out so many great points that I was taking notes on, and also I will probably recycle and plagiarize your words. So forgive me in advanced for doing that. Oleg, Vishnu, Jack, Anitha, this was great. We will see you all later. If you like this kind of stuff, I'm going to just give a shameless plug right now, because we made it this far. Jump into the MLOps Community. We do it every week. All right. See you all later.
Anitha Vijayakumar (40:07): Thank you for having us.
Jack Guo (40:07): Thank you.
Oleg Avdeev (40:07): Thank you.