Panel: Lessons Learned Scaling ML Systems
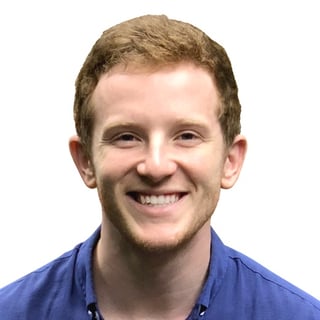
Russell Kaplan leads Scale Nucleus, Scale's Dataset IDE for machine learning engineers. He was previously founder and CEO of Helia AI, a computer vision startup for real-time video understanding, which Scale acquired in 2020. Before that, Russell was a senior machine learning scientist on Tesla's Autopilot team, and he received his M.S. and B.S. from Stanford University, where he was a researcher in the Stanford Vision Lab advised by Fei-Fei Li.
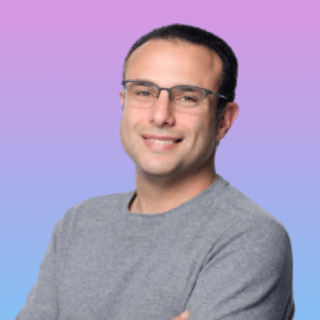
Hussein Mehanna is the Head of Artificial Intelligence at Cruise. He is an expert in AI with a passion for machine learning. He has over 15 years of experience and has successfully built and led AI teams at multiple Fortune 500 companies. Prior to Cruise, Hussein led the Cloud AI Platform organization at Google. Under his leadership, his team revamped the product line and rebuilt the organization. Cloud AI Platform became Cloud AI's fastest growing product segment. Before Google, Hussein worked at Facebook where he co-founded the Applied Machine Learning group that combined applied research in machine learning and advanced platforms. He helped democratize artificial intelligence with more than 2000 engineers using the technologies, and his team added billions of dollars of revenue. Hussein has a Masters in Computer Speech, Text and Internet Technology from the University of Cambridge and a Bachelors of Science in Computer Science from Ain Shams University.
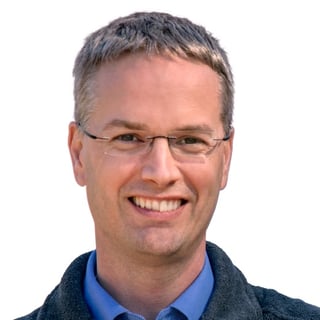
Bart Nabbe is the VP of the Strategic Partnerships and Corporate Development team at Aurora, where he handles all of the company’s major corporate partnerships. Prior to joining Aurora in 2017, he was the Director of Strategic Partnerships at Faraday Future in 2016 and was a founding member of Apple’s Autonomous Systems team from 2014 to 2016. He was a Research Scientist at Toyota, Tandent Vision Science, and Intel Research. He has a B.S. in Electrical Engineering from Venlo College of Engineering and a Masters in Computer Science from the University of Amsterdam. He earned his Ph.D. in Robotics from Carnegie Mellon in 2005 where he met and worked on mobile robotics with Chris Urmson and Drew Bagnell, who are co-founders at Aurora.
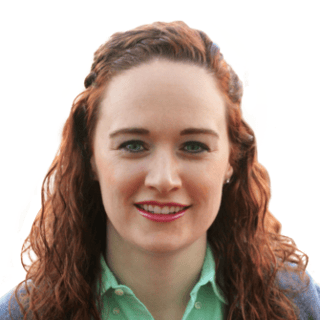
Danielle Dean, PhD is the Technical Director of Machine Learning at iRobot where she is helping lead the intelligence revolution for robots. She leads a team that leverages machine learning, reinforcement learning, and software engineering to build algorithms that will result in massive improvements in our robots. Before iRobot, Danielle was a Principal Data Scientist Lead at Microsoft within the Cloud AI Platform division. There, she led an international team of data scientists and engineers to build predictive analytics and machine learning solutions with external companies utilizing Microsoft's Cloud AI Platform. Before working at Microsoft, Danielle was a data scientist at Nokia, where she produced business value and insights from big data. Danielle completed her Ph.D. in quantitative psychology with a concentration in biostatistics at the University of North Carolina at Chapel Hill.
Danielle Dean, Technical Director of ML at iRobot, Hussein Mehanna, Head of AI at Cruise, and Bart Nabbe, VP of Corporate Development at Aurora discuss the lessons learned scaling ML systems.
Russell Kaplan: All right. Welcome, everyone. We’re super excited to have the three of you hear today. For the audience, this panel is on, Lessons Learned Scaling ML Systems. And we have three experts in the room who have seen a lot of this firsthand. I’m really excited to have them, and start talking about what they have gone through and what they’ve learned building incredible revised programs at different companies. So, maybe we could start off with folks introducing themselves. Danielle, if you’d like to start, and then Hussein and Bart, sharing a little bit about your background on what you’re working on.
Danielle Dean: Yeah. Absolutely. Thanks. So, I’m Danielle, the Technical Director of Machine Learning at iRobot, the makers of products like Roomba and Braava. We’re doing deep learning solutions, reinforcement learning solutions, for millions of robots in homes worldwide, and excited to be here.
Hussein Mehanna: Hi, everyone. My name is Hussein. And I work at Cruise. We’re an autonomous vehicle company. I’m the VP of AI and Machine Learning there. So, my team builds the perception and prediction systems that run on the car. It also builds the machine learning infrastructure that we use to build these models, including the data science and machine learning deployment. So, super excited to be here.
Bart Nabbe: Hi. I’m Bart. I am VP Corporate Development and Strategic Partnerships at Aurora Innovation. A start up company. We are with now 1600 people that really focus on building the Aurora driver. We are doing this not on my own, clearly, but with my great buddies from my grad school at Carnegie Mellon. And what I do there is really look at opportunities to accelerate development. And I’m happy to be here. Thank you.
Russell Kaplan: And my name is Russell. I’ll be your host. I’m the Head of Nucleus here at Scale. Nucleus is one of Scale’s newest product offerings, a data set management platform for machine learning teams. Before that, I started a computer vision company called Helia, and was senior machine learning scientist at Tesla on the autopilot team, responsible for the vision neural network. I’m super excited to have you all here today. And I want to just get right into, what are the key differences between your ML life cycles and workflows, from when you were operating at one tenth of your current scale and now? What’s changed? And maybe, Hussein, you could start sharing some detail.
Hussein Mehanna: Yeah, sure. I think there are three main differences. The first and biggest one is reproducibility. Maybe two or three years ago, when we were much smaller scale, models were not reproducible, or shipped without reproducibility in mind. The second big difference is the scale of training. So, we now train on far more data than we have before. And then, the third biggest difference is automation. So, we are increasing the amount of automation we use, to train more and more models without having our engineers involved. Those are the three biggest differences, I would say, compared to two to three years ago.
Danielle Dean: Yeah. Great points, Hussein. I double click on all of those points, because those are really good ones. Some additional ones, at iRobot, that we found really helpful in increasing scale is increasing testing at every layer, because as you scale up, and you find issues, as you always find issues, especially as you start to automate systems, you need to then know, “Where did things go wrong?”
Danielle Dean: So, increasing testing, at the system level, and then at the individual pieces, let’s you debug and jump into those parts easier. And then, it just… I absolutely agree on the data piece. At iRobot, we’ve created a data collection, and data annotation team, focused around machine learning algorithms. So, having that dedicated team thinking about data has really helped us increase our scale a lot.
Bart Nabbe: Yeah. I think they’re all heading somewhere similar to the topic here. You’re not building, really, ladders to get to the moon. Right? We always said, “If you want to get to the moon, you need to build rockets.” Right? Collecting the right amount of data is key for this. When you begin doing this, every single data item counts. When you know, at this scale, it’s not really that thing anymore. It’s really what are the unique items. Right?
Bart Nabbe: And it’s really those important cases, long tails that we’re going to talk a little bit later about, that really counts. So, that’s really a big difference between, in the beginning, when everything counts, towards what now is, “What is really important?” And I also like to point out that not all cases are equal. You already mentioned this step. It is very important to understand how you validate. It really doesn’t matter, for me, that the statistical performance went up on the average, if what I now lost is detecting one pedestrian, but I’m very good at 10,000 traffic cones.
Bart Nabbe: And so, really being very explicit about what counts, what matters, what is the system influence of your performance… Yeah. I think that’s a big difference from in the beginning, when everything, every single thing, was awesome because I’ve made it better. Now, we have to be very precise about that.
Russell Kaplan: That makes a lot of sense, that move from aggregate metrics to kind of very specific measurements on all the different parts of the distribution you care about. So, I guess, to dive into that a bit more, a frequently encountered challenge that we’ve all seen, of scaling ML systems, is dealing with this long tail distribution of the real world. And it seems that, at each increased order of magnitude of scale, you encounter new ever-rarer edge cases. So, what I’m wondering for you all is, first of all, how do you deal with that today? And what’s the future here?
Russell Kaplan:: What’s the plan for the next order of magnitude, and the next order of magnitude? Are we going to keep this constantly uncovering new parts of the long tail, and always be debugging them? Or is there a more scalable approach here? Yeah. What do you think, Danielle?
Danielle Dean: Yeah. So, at iRobot, we take two big approaches. One is designing our data collection and model analysis procedures to purposefully find those edge cases, so that we can go after them and know what those are. The second big thing is thinking about, how do we design the system as a whole so that the ML model can work in conjunction with other parts of the system? We all know that ML systems, data driven, they’re going to have failure modes. How do we make sure those failure modes still provide a good experience at the end? So, thinking about those two, it’s always trying to improve, but also knowing that, these data driven systems, they are going to have some weaknesses. And how do we complement those weaknesses?
Hussein Mehanna: Yeah. I think I agree quite a bit with Danielle here. You have to have a robust fast continuous learning cycle, so when you find something that you haven’t seen before, you add it quickly to your data, and you learn from it, and you put it back into the model. But that’s still reactive. And therefore, designing your system…
Hussein Mehanna: And I’d like to expand a little bit more about that, particularly focusing on high recall methods. So, if you look at the autonomous vehicle problem, you can generalize the world into things that move and things that don’t move. They have volume and they have velocity. And so, you can still build high recall methods that operate at that highly generalized level. But then, the rest of the system needs to react in accordance to that. And this brings up uncertainty. So, you might not… You have high recall, but you may not be super certain what exactly this object is. And therefore, the rest of the stack may need to drive the car a bit cautiously for a second or two.
Hussein Mehanna: And us, as human beings, we do that. So, I think that’s a solvable… Or you can sort of make the long tail tractable that way. The third point that I’d like to add is that there are a lot of product tricks. And so, there was a video where the Cruise car, with our CTO Kyle, and Sam, the CEO of Open AI. And essentially, our Cruise car witnessed a sort of funky construction vehicle at an intersection. And it seemed as if the Cruise car just handled it naturally. But what really happened, in the background, is as we approached that intersection, before we got there, the car notified remote assistance, and someone took a look, and just quickly charted a path around it, and with minimal destruction to the user experience.
Hussein Mehanna: So, there are product tricks that you can deploy, to Danielle’s point, that help you, sort of again, make the long tail tractable.
Bart Nabbe: I don’t think you should call that tricks, because I think that’s really the solution. As we pointed out already here, and all of you are saying the same thing, it’s very careful system architecture. We have to build safe systems. And that’s just one single component that needs to be strategy. And from day one, we knew that these edge cases were going to make or break a product. So, focusing on being good at dealing with edge cases is extremely important. And you can’t do that by relying on just one single component or network.
Bart Nabbe: When you look at, for example, unprotected left turns, there’s always one of those examples where, in self driving, people look at performance. Right? It’s really going to be like, how many of those cases have you seen, and how do you perform in those, and making sure that you’re really getting towards the end of that.I think also, very importantly, you also mentioned that, what if this was a generic case? We have this philosophy that no single measurement can be left unexplained. If you don’t know what it is, that doesn’t mean you should stop reasoning about it, because there was something there. And maybe your model hasn’t figured this out yet, but it is important. So, realism of that system approach, I think, is key. And I think we all agree to that.
Hussein Mehanna: Yeah. I think we do. Maybe I labeled it as a trick. It doesn’t make it any less important, to be honest, Bart. But the thing is, that I see, is people often obsess about solving the long tail for one model. You have one model, devoid of an ecosystem, devoid of anything around it. And solving that problem is extremely huge. Luckily, there are domain tricks, systems, thoughts, whatever you want to call it. There are domain aspects that you can benefit and leverage that help you make the long tail tractable. So, I think that is doable.
Hussein Mehanna: And I often see a lot of people in the AI industry tell me, “How are you going to ship a car? How are you going to handle the long tail? This is impossible. No one has sold that. You need artificial general intelligence for that.” But that’s when I explain that there’s a lot of things that you can leverage, that it’s actually solvable.
Russell Kaplan: Yeah. I think that’s a super interesting point. And it’s one trend that just we’ve been seeing more generally at Scale, and especially internally, where, for our machine learning systems, we are very explicitly kind of trading off between, “Okay. How much of a distribution can we capture with machine learning?” And then, “Where can we augment that solution with human intuition to kind of completely solve the problem?” And depending on the domain… In self driving, there are latency requirements. There’s a lot of tricks there, and… whether you call them tricks or it’s system architecture designs, to make sure that you can safely transition, if you’re going to make that transition. But I think what’s…
Russell Kaplan: One general thing we’re seeing is, as long as you can architect that system in such a way that you can make the trade off and go from model to person, you just get better over time. The people fill in the gaps. And then, your system gets more intelligent. You have fewer gaps. And I think that segues into this next question which I wanted to get at. And maybe, Bart, you can tell us, from your perspective, both as a PUC roboticist and a leader at Aurora on the technical level, and at the kind of strategic partnership level, how does this approach, as we’re describing right now, scale to the next order of magnitude, the next order of magnitude? And is that something that…
Russell Kaplan: Obviously, there’s going to be more architecture system design, but are there new things you plan to kind of introduce, new processes, to help keep making this better?
Bart Nabbe: Yeah. That’s a very interesting question, because we talked about, how do we go from where we were to where we are now? And it’s, where do you put your next best efforts to make that count for you? We’ve always been setting very outrageous goals for ourselves. We acquired a simulation company to really get a boost in getting started on making that simulation as accurately as we can, to represent the world. And so, allowing that, allows us to really sort of put that focus on that, so that is just a continuation of what we’re going to do. It’s a very large problem that we’re going to solve, and we can’t just do this all ourselves.
Bart Nabbe: And so, you continue making these investments on better infrastructure, better technology, new technology. We don’t have everything ourselves. And so, I see improvement on ML platforms as being one of those key measures to do this. But also, keep making internal investments as well. We spend a lot of time collecting data over various platforms. So, the that out driver could actually be independent of what architectural or vehicle class we are going to put the driver in. So, it’s a little… because 12 weeks to bring up a truck, after we’ve only been driving beforehand on mini vans. But that’s really because we’re looking at the problems that we’re going to solve ahead from us, and try to make investments currently today that will actually grow with us.
Bart Nabbe: So, really looking in the future helps you do that. And there’s certainly a question, also, from what are the technology we’ll be needing in the future. And I think one of those things, specifically, that are useful… better tools for identifying, how do you get reliable networks? How do you make sure that, when you learn something new, you can incorporate it quickly in your stack. Those are all important aspects to that. Just continuing making those types of investments is what, I think, is going to happen.
Danielle Dean: Bart, you summarized it so well, hard to add on. I guess the only other point I’d make is just continuing more automation, so every aspect of the puzzle pieces that you need to put together to build the solution. So, for example, Bart mentioned simulation, synthetic data, all the data annotation. Where are the pieces that you’re doing to get to that scale? And how can you automate more and more of those pieces so that you can scale up exponentially?
Hussein Mehanna: Yeah. Totally agree. I just want to maybe dive in a little bit, in terms of simulation. I think simulation is extremely important, so that we can, as our head of simulation says, abuse the car virtually in all sorts of ways. And this is not just for testing. It actually helps you accelerate figuring out the long tail, accelerate understanding the limits of your system. And you can leverage, actually, machine learning to train models that try to get your system to crash.
Hussein Mehanna: And that is extremely valuable… And when I say “crash” here, I don’t mean crash as a system failure, or unhandled exception. I’m talking about where your car would be unsafe. And so, I see that as an extremely valuable tool. The point that Danielle mentioned about synthetic data, I actually see a significant amount of value there. I was personally sort of very skeptical about this. But recently, at Cruise, we’ve seen data that demonstrates you can even leverage synthetic data for training perception systems. And I think that is going to accelerate how we deliver safe systems, and how we can span into multiple cities. So, these are very exciting things.
Hussein Mehanna: The other thing that I just want to mention also, a little bit more concretely, is there needs to be more investment in understanding the performance of robotics within the environment they’re in. This is non trivial. So, when you compare machine learning and a company like Google or Facebook, these operate in the data center, so they’re fully instrumented. You can easily generate data about where your models are doing well, and where they’re not doing well. Now, take that to robotics, where you have a vehicle, or a Roomba, or whatever it is. It becomes, on several orders of magnitude, more difficult. And there’s a lot of innovation there. And I do believe that, if we can accelerate that process of capturing data from the environment, diagnosing it so that we can understand which parts of the system are responsible for these areas, this will be a massive step function in our capabilities to deliver such robots in production.
Hussein Mehanna: So, it is going to take some time. But it is definitely one of the biggest investments. And I think companies like Scale can actually deliver a lot of value there too.
Bart Nabbe: Yeah. Can I add something to that? Just to give you a share of the magnitude difference, how that is important. So, when we are going to make the first unprotected left turn… Again, that example. We actually had 20,000 hours of practice of really doing unprotected left turns in simulation, before we had done a single physical one. That’s 227 million or so left turns in simulation. Now, if you look at what somebody does for their driving license, maybe 50 hours of supervision before they… and they get let loose on the road.
Bart Nabbe: So, I think you’re really pointing out what is important, being able to do this safely virtually, but making sure that it is realistic, and so that what you actually prove out in simulation actually holds for the real world. And as you pointed out, that’s going to be key to be successful here. Yeah.
Russell Kaplan: Yeah. I wanted to dive in. Hussein, what you were talking about is super interesting on this kind of synthetic data component as well, and simulation more broadly. So, when we’re talking about this, just to be concrete. There’s many different sensors on a robot. And so, what are we simulating here? And do you think that the kind of learnings from this, from your experience… Do they translate to other machine learning tasks if we’re not doing robotics?
Hussein Mehanna: I don’t know really about non robotic applications, with regards to simulation. I think simulation is far more relevant in perception applications. And so, just as you said and as you referred to, whenever there are sensors and you understand their physical capabilities, you can actually leverage simulation and sort of expose these sensors to circumstances that you may not be able to, or would be far more expensive to actually collect such data.
Hussein Mehanna: Now, I just want to clarify that synthetic data will never be a full replacement to real data, but it actually enriches the data set. It may mean that you don’t have to collect as much, or you actually can collect data you may never be able to collect, for various reasons. So, I think, as I mentioned, it’s complementary. And I think it’s going to be extremely useful for robotics scenarios. Because the interesting thing about simulation is this concept of fuzzing. What you can do is, if you understand your environment and you have a couple of pivots, you can then vary these pivots far more than what you could see in the real world.
Hussein Mehanna: As an example, if we want to collect data related to emergency vehicles, there’s not a lot of them actually in San Francisco. In fact, even motor bikes, there’s not a lot of them. But if these are… particularly motor bikes, these are users that you would see, and in emergency vehicles you would also see, even though rarely… And so, simulation can play a massive role there to enrich your data, and put your emergency vehicle in various different circumstances that you may not be ever able to collect, because it’s such a rare case.
Russell Kaplan: Makes a lot of sense. Thanks for clarifying. And I want, then, to go back to an earlier thread that’s maybe somewhat related to this, which is the question of, how do we then measure the performance of these systems? If we’re doing well in this scenario, and this scenario, and this scenario, maybe even more broadly than just the system level metrics, what are the most important metrics? They could be anything: some way of measuring your team’s productivity, some way of measuring your model performance, some way of measuring your system.
Russell Kaplan: What are the most important metrics that the three of you care about when you’re kind of scaling up these ML systems?
Danielle Dean: So, I’ll give a couple funny ones to kick us off. And then, I’ll let Hussein and Bart put in some more real ones. But I always talk with my team on a couple of KPIs. One is, how many solutions are in production providing real customer value? I think, in ML and data science in general, inside robotics and other domains, there are so many cool techniques we can use. There are so many cool approaches we can use. There are so many potential solutions, but just focusing around what are solutions that make an impact to the customer and are running live in production, so just keeping a focus on that.
Danielle Dean: And then, the second one… This is what I call a tell-a-friend KPI, which is terms of not… Obviously, measuring model performance, measuring system performance, those things are very important. But how many iterations do you go, where you make such a jump step in your solution that you would tell a friend about it? Like… We weren’t using synthetic data before, but now we’re using synthetic data and we are way better than before. And you’re so excited about it that you’d want to tell a friend. So, we call that the tell-a-friend type of KPI. Those are two ones that we think about.
Bart Nabbe: I actually really like the tell-a-friend KPI. Because we have a dashboard in the company, where we actually basically use that as the tell-a-friend, because we’re doing this with our friends. And so, why is this important? Because it’s, again, that systems approach. If you just made your traffic light detector better, how does this really impact our overall system? You, as an individual, working on that with your data set… That’s very difficult to judge if you’re actually helping. And so, setting up your KPIs so they all sort of string together into overall system performance, so that you know that, if you improve this, it’s going to help the overall system, I think that is very key.
Bart Nabbe: So, these compound KPIs that are still individually meaningful… That is, I think, one of the techniques to do this. You also mentioned another one that I think is really important. How quickly can you actually get something from newly never seen it before, to this is one of those long tail events… How quickly can your system now become better? That’s just measured in time. It’s a very concrete one. I think we all can easily agree on that. That’s a really important measure. And why is this important? Because there’s always going to be new things to do. This is the whole game of level for autonomy, the operational domain. Right?
Bart Nabbe: If I want to increase my operational domain, and it’s safely, quickly, broadly… so yes, broadly. That means we have to address new data, new items, new scenarios. And how to bring this in, and then quickly make a better system, is also one of those key metrics. So, I think you pointed out, even though I think you have much lovelier ways to call them, I think the key one is that sort of measure. What is the thing that you actually can count and get the system to be better, but also that you can actually do the whole operation of making the system better quickly?
Hussein Mehanna: I think all of the metrics mentioned are very useful. I do believe there are one or two very powerful additional metrics. When you build these simulated environments, or even your offline replay testing environment, you want to measure how it correlates to sort of the actual environment in the world. Because if it doesn’t correlate, then the metrics you’re going to be uncovering from it are not… may not direct you in the right… may not give you the right direction, essentially.
Hussein Mehanna: And so, at Cruise, we’ve spent a significant amount of time and effort making sure that our offline tests and our simulation environment is as correlated as possible to the real environment. And it is not an easy job, because the real environment changes, traffic patterns, the proportion of driving during the day versus the night, the proportion of driving in different neighborhoods. They all have different characteristics. There might be some unplanned events which add additional pedestrians. And as we know, this makes the driving situation more complex. So, it’s very important that any robotics company understands, or tries to understand, the statistical composition of the environments where the robots operate, and then bring that to their offline world, and make sure their offline world is balanced towards those statistical compositions.
Russell Kaplan: So, you’re measuring overall system performance, and you’re measuring your traffic light detector performance. And you’ve improved your traffic light detector. But there’s some hidden coupling in the tracker system. And the common filters are now… Because the model is better, the overall system has worked. So, do you have every engineer who’s working on an individual experiment testing everything? Or how do you make forward progress?
Bart Nabbe: So, if you look at what our virtual test suite is like, it’s all about scenarios that are important and happen in the real world. What is the performance of the overall system on that? Now, you can still track your individual KPIs, and how well you do on your traffic lights. But while you do those experiments, it also gets rolled up in these virtual experiments that are simulating a whole drive, sometimes with real data, because we can just replay that to some extent.
Bart Nabbe: And sometimes, it’s completely newly created environments. The tools that we build off early On, when we need to get a very accurate simulation of our sensor suite is actually very important, because we’re making our own sensors. It’s not that I’m going to first hand over all my trade secrets for my FNCW LiDAR to somebody who’s going to make me a simulator. So, we do want to simulate that also quite accurately. And so, it is indeed very important that the correlation is very strong. Because otherwise, the results that you get in your virtual test suite don’t really mean very much. But the void needs to be done in simulation, because we can’t drive enough ever to convince anybody that the system is safe if you have to intervene in the vehicle.
Danielle Dean: Yeah. And I think there’s scenarios where an individual model metric might improve, but the overall system might decrease. Those are awesome scenarios to figure out what… Why does that happen? And is your validation set in that individual scenario actually representative, or is the system level test not representative? What is going wrong that is causing that?
Danielle Dean: Because in general, if you improve part of the system, the system should improve. Obviously, there are exceptions. But those are really good cases to dive into. Why is that happening? Is there something in the data collection procedure? Something in the simulation procedure? And focusing on that will help the overall system improve.
Hussein Mehanna: Great point, Danielle. Generally, this problem of changing a component in the system that may, in fact, upstream or downstream components, is a very complex problem. And it’s one of, actually, the most complex challenges that robotics engineers face. And the more you invest in simulation, the easier it becomes, because they can discover these problems ahead of time. And they need to solve them before they deliver. That said, I think the most important aspect is you solve problems from an end-to-end perspective.
Hussein Mehanna: I often see, when people are more focused on solving the performance of a model, they are probably not thinking about the problem end-to-end. And often, what happens that… They may actually improve… The worst scenario is they improve the performance of the model, but nothing happens downstream. Because, let’s say, the car starts detecting car doors that are being opened, but the planning system does not react to that, then you’ve built a feature that no one else is using. So, the way we do it at Cruise is we encourage folks to work more from an end-to-end perspective.
Hussein Mehanna: And that way, they’re already thinking about the impact of these changes downstream and upstream. And they deliver sort of an end-to-end experience versus just improving a model in a silo, without understanding how the rest of the system needs to react, or leverage the outcome of this model.
Russell Kaplan: We have one question from the audience, to just add a little more color there, which is around… So, when you are using this simulated data, how do you make sure you’re not over using, you’re not over fitting to the simulated environment? How do you manage that domain depth?
Bart Nabbe: I think that’s with all data. You cannot train on your test set. And so, you have to be just very careful how you use data. So, there’s no difference there for synthetic data versus real data. You need to be honest with yourself there.
Hussein Mehanna: At the end of the day, we have to have live tests, so that we detect whether we are over fitting or not. And so, that is sort of a safety net there. But then, as we discussed, the correlation aspect is the antidote to reduce sort of your over fitting on a simulated virtual environment that doesn’t represent anything. So, it’s a great question. But that’s why we’ve also invested in correlation. And it’s not just for simulation. It’s even for your tests where you actually replay actual data.
Hussein Mehanna: So, if your test population is more during the day than you actually drive in the night, then you have a gap there. So again, as I said, correlation and making sure you have enough coverage is critical here.
Russell Kaplan: Makes a lot of sense. And I think one theme from a lot of these answers is there’s a lot of infrastructure here. There’s a lot of testing infrastructure. There’s a lot of work flows, so that when you do run these experiments, you can validate them. So, I want to talk a little bit more about that. And there’s been, I think, in the industry, a broader conversation of this concept of software 2.0, and machine learning being, in many ways, a fundamentally different mode of development and traditional software development, where we’re maybe lacking some of the tools that we really have well established at this point for traditional software development like CICD, version control, code review.
Russell Kaplan: What does code review look like for 100 million neural network weights? So, I’m curious if any of you have kind of taken some inspiration from those existing tools for traditional software development. And what have you learned trying to maybe translate them to a machine learning first mode of operation?
Danielle Dean: Yeah, absolutely. And we think a lot about, how can we… Hussein actually mentioned this towards the beginning, the reproducibility aspect. How do we think about the entire system being able to reproduce to understand where and why things happened. Obviously, sometimes, it’s very hard to get perfect reproducibility. But thinking about what are the aspects that matter, thinking about version control of models, thinking about a model registry, thinking about data reproducibility. And where did that come from?
Danielle Dean: We have an interesting aspect at iRobot, where we need to respect the right for data to be deleted from our testers. And so, how do we deal with model reproducibility and that whole thing around when data is deleted from within models? We also invest a lot in CICD, around things like Python packages, docker images. So, how do we make those workflows easier, and share knowledge, and share tooling around the team? So, all of those things are a really big investment, for sure.
Bart Nabbe: So, if you look at how we look at that at Aurora. If you look at the software team, they have a tenant that’s called Carry Our Water. What that really means is that we’re all responsible, not only for the work product of maybe the model that you just trained, but also getting the right tools together. There’s no point for everybody to write their own little Python script to do something.
Bart Nabbe: Yes, if you look at software engineering as a whole, there is a fantastic suite of tools that you can just buy off the shelf. That’s kind of my job to find some of those minute, unique tools that can help with that. I wish there were those unique tools that actually could help me enter into all my machine learning worlds. Now, there have been actually some startups in this space that give you some interesting pieces over there. But there’s no actual complete tool chain that I can just put to work. So yeah, that is something that we just somewhat have to build ourselves.
Bart Nabbe: And we’re doing this because it’s not quite out there. And that’s a team effort. But those tools really need to make sure that they can deliver on what is actually expected from us. We’re building here a safety critical system. And it’s only as safe as we can make it, based on the tools that we have. So, if you can indeed not guarantee integrity of data, how do you ever update a model when it is needed? And so we need a lot of tools around there to help us. And now, there’s just a lot of tool building going on. Again, back to this you have to build rockets to get to the moon to get there. Because it’s just a much harder problem. And the tools aren’t as mature. So, we do a lot of that ourselves. But it’s a very big investment. And fortunately, I think everybody is doing similar things there.
Hussein Mehanna: I want to maybe shift the question a little bit. What is the difference between building ML in robotics versus machine learning that has been done in many big companies for more than a decade? I believe that potentially Google was one of the first companies that developed a very large scale machine learning model, maybe by 2004/2005. So, we’re talking about 15 years of machine learning development. I think what is unique about robotics, and it turns out is a big gap in traditional ML, and traditional ML applications, like in commerce, or search, or ads, and so on, is that you really need to understand your input distribution.
Hussein Mehanna: You really need to understand where you are deploying the system. You can not just make trade offs there. So, as an example, in the ads world, as long as you’re one percent, or two percent, or three percent better, you can deliver them all. But that might mean that you’ve traded off one cohort above the other. And that’s why some social networks have now… have problems where their ad systems are biasing against colored users. Now, in robotics, we can’t do that, especially in AD. We can’t go and say, “Well, our system works really well with silver cars, but it doesn’t really work so well with yellow cars. But since there’s far more silver cars, it’s okay. We’re one percent safer. Let’s ship them off.” We can’t do that.
Hussein Mehanna: We have to understand the input distribution. And that’s the reason why I think machine learning for robotics may not suffer the same problems with bias and unfairness that other machine learning systems have suffered. And this is a completely new concept, in development in general, that you don’t even see in normal software development.
Russell Kaplan: Yeah. I would chime in that the observation of making these kind of aggregate trade offs for edge cases it’s definitely a recurring theme. And I don’t think you can escape it, whether you’re an ML system for robotics, an ML system… a computer version system for retail, or any number of these different domains. There’s constantly going to be… As machine learning pervades in more and more of these societal systems, it becomes really important to not just work well enough, but also have the audit ability, have the measure ability, to know that we’re serving all different groups, and in this case vulnerable road users, that we’re doing a good job on all of them. And I think that makes a lot of sense.
Russell Kaplan: Given that it sounds like you’ve all implemented many of these systems yourselves, to kind of do this analysis, have there been any surprising, or unusual, or really tricky bugs, that you’ve discovered, where it was maybe at the intersection of multiple systems, or something that the process of solving that really illustrated why some of these investments paid off? Danielle, I don’t know. Got anything to share?
Danielle Dean: So, at iRobot, we’re constantly developing new products. So, those new products have new sensors, which obviously means the data for those new sensors will be different. So, at the beginning of the life cycle of new products, we need to collect data to make models for those new products. And one thing that we do… But when the hardware and the software is not yet ready, it’s not as easy to collect the same distribution of data as you’re going to get when that product is ready.
Danielle Dean: So, one simple example you might be able to imagine is we might use a camera sensor to collect images of kitchen tables, so that we can build experiences that do things, “Alexa, clean under my kitchen table.” But if we collect still images of all the kitchen tables, but then, in the real world, the Roomba’s going to be driving around and getting motion blur… So, that distribution of the data that we collect is often really tricky to figure out. Is the collection data going to be similar enough to the production data that we’re going to be able to have that same level of performance?
Danielle Dean: As we scaled up our systems, and built in more automation, built in more testing, it allowed us to figure out where those types of errors were, and to figure out what to prioritize on the data collection front in order to improve the systems.
Russell Kaplan: Right, Danielle. So, you have a whole data collection curation team you mentioned. And I guess that I would be really curious to understand, both for you and for other folks as well, how do you prioritize what unlabeled data to label? Because one thing we’ve seen with ourselves, and among our customer base, and the kind of machine learning, to me, more broadly, is that there was this kind of hierarchy… There is this evolution that machine learning teams go through where, “All right. We’ve collected our data. Let’s randomly sample some subset, send for labeling. Cool. We’ve got a model.”
Russell Kaplan: And then, as people start realizing, “Oh no. We need it to work better and better and better”, people get more sophisticated about that curation component. So yeah, I would love to hear how you prioritize what unlabeled data to label at this point.
Danielle Dean: Yeah. Absolutely. So, a few different factors. The first is diversity, so diversity of places around the world. With Roomba, for instance, it has to operate in all the places in the world, so diversity of environments, diversity of locations. Then, the second main one is model in the loop sampling, so figuring out where is the model not working well, with the highest loss, issues with the model, and sampling based on that.
Danielle Dean: And then, the other one is just simply spatial sampling, because we care, for instance, being able to understand what the different furniture items are, different things within the home, so leveraging other sensors or other metadata that tells us about the uniqueness of the data point. So, diversity, uniqueness, and model the loop.
Bart Nabbe: I think there’s two questions in there. Let me start with the first one. Our mission at Aurora is really to deliver the benefit safely, quickly, and broadly. Safety is really critical to everything we do. And so, when you say an insidious bug, that’s something that I have to have caught in my virtual tests already. That’s why I need to catch them. Not only then… If I catch it then, that’s an opportunity to learn for the long tail. Because hey, I hadn’t seen it before. But it’s important. So, it goes directly in our list of new scenarios, new events.
Bart Nabbe: And this is also how you get curated data. That’s sort of your second question, right? So, how do you manage a stream of data? How do you understand what is important? Yeah, we have a triage team for that. We’re really going to look very carefully on these events that… Behavior is different than expected. And then, we’re going to understand what parts of the system work, what didn’t work, and then collect examples of that in those long tails. So, it’s really a very meticulous process to look at what has… these events that you don’t know how you perform yet, and collect a new bank of interesting scenarios, in addition to all the scenarios that you already had.
Bart Nabbe: So it’s, again, this whole scaling out of an operational domain, so that eventually we’ll, at some point, have a level five system growing out of these increasingly larger operational domains.
Hussein Mehanna: These are all great points mentioned by Danielle and Bart. I just want to add an additional category. It’s really useful to collect data on any use case where the system is making mistakes, maybe not the entire system, but maybe a sub component of it. Right? Now, the question becomes, as our cars are driving, or the Roombas are going around, how do you determine that? So, we’ve realized that a combination of rules to leverage auto labeling, or even machine learning models that try to estimate, “Well, it looks like something is funky here.”
Hussein Mehanna: The car hasn’t necessarily gone into an unsafe situation. But maybe there’s a pedestrian far away that we should have seen and we’re not seeing. Or maybe there’s a vehicle that we’re not estimating its dimensions correctly. And so, we actually found out potentially if you could use some machine learning models to capture these samples, and some of these learning techniques to enrich the samples you capture, it could be very very useful here. And I think there is going to be some more innovation. Because what you really want to do is you want to turn every mile, where you have a car on the streets… Whether it has a driver behind it or not, you want to turn every mile into an opportunity to learn something. But in order to do that, you need to automate the ability to say, “This piece of data is useful versus not.” And just doing random sampling will very unlikely help you.
Russell Kaplan: Totally. I think that’s such a good point on… In some ways, the kind of model debugging process is very deeply tied to the data prioritization process. And this is on my mind a lot, as the three of you know, just from leading Nucleus, where the kind of primary goal is to help make it easier to help people debug their models by debugging their data, and then improve their models by being really automatically thoughtful about what to prioritize next.
Russell Kaplan: One question we got from the audience on this topic is, how then do you measure loss, say, on unlabeled data? If you don’t have the labels, how do you know that it’s problematic?
Hussein Mehanna: That’s a very good point. It’s very hard to measure recall. It’s really really hard. And I don’t think there’s a good way to do that. That said, we have some proxies, where we’ve already detected problems on the road, and we have that category. And it has some data points. And we use our automated techniques to see, how many of these examples are we labeling as actual issues?
Hussein Mehanna: So again, it’s not a true metric for recall. But at least it gives us an indication that, here’s how much it’s capturing out of what we know.
Danielle Dean: And I would just say leveraging as much as possible other sensors, or other information that give insight, or even other models. At iRobot, we have a very small chip on our robot. And we have to deal with the whole quantization of models aspect. So, a lot of times, we can run much bigger, much fancier models in the cloud. And we can use those models to also help find issues with the smaller models. So, just whatever other information that you can use to help inform that model, those predictions, is often really helpful.
Bart Nabbe: We see that also very much the case what you said, all of these other modalities. So, we have quite a few modalities. And using that also when you cross label things is a lot easier. If I already have some LiDAR points over there, and I have a camera image there, now, all of a sudden, it becomes much more tractable to do something with that greater data. And so, that does help. But yeah, it’s not necessarily an easy problem, as you all point out.
Russell Kaplan: I’ll chime in. The other things I’ve seen, both in my personal experience as a machine learning engineer, and then also helping other folks now with Nucleus, is that even if you don’t have labels for your unlabeled data, you can still have model predictions. And so, people can run one set of model predictions. You can potentially run multiple sets of model predictions, and there’s a lot of active learning research around taking the differences between that ensemble of model predictions, and using that as a proxy for maybe loss or how tricky it is, as well as, for that subset of data you do have labeled. Well, you can measure errors on that. And then, you can find similar examples to the erroneous ones.
Russell Kaplan: And whether that’s through the structured metadata you’re talking about, whether it’s through more kind of semantic similarity, there’s a lot of diversity in how that gets done. But those are some other things that we see. I guess, we’re kind of getting close to time here. So, I just wanted to ask you all, maybe upon reflection so far in your careers as machine learning leaders, what is one ML process change or infrastructure investment that you wish you had made earlier, now kind of seeing things from where you are? And Hussein, we’d love to start with you.
Hussein Mehanna: This is a really hard question to say because I wish I’d made all of them earlier. We have a very long road map and a lot of things to do. I don’t think ML for robotics is there yet. It’s in its infancy. So, it’s just really hard to choose. There’s just so many things that I wish we had done already.
Danielle Dean: There’s so much infrastructure investment, and so many things that need to be built. One thing that I would call out is, I wish we had focused a little bit on creating a machine learning data collection and data team that we talked about earlier. I wish we had done that earlier. We obviously have a lot of systems tests. We have a lot of testing of Roombas. There’s a lot of data that’s being collected through other means. But having a dedicated machine learning data team, who can work with machine learning engineers and can focus on what is the meaningful data collection that will impact the models… Doing that earlier in the cycle definitely would have accelerated us as well.
Bart Nabbe: I think we’re pretty aligned here altogether. So, we have actually built fantastic visualization tools now. And oh boy, we wish we would have done that earlier, because now we much better understand what we’re actually spending our efforts on, what our KPIs are, and all of that. And so, we really didn’t know that beforehand. And now we do. And so, we wish we would have known earlier.
Russell Kaplan: Visualization, data curation teams, makes a lot of sense. Sounds like some hard won lessons from scaling ML systems in the real world. Thank you Hussein, Danielle, and Bart for spending time with us today. Really enjoy the conversation. And thanks to the audience for tuning in. Take care everyone.
Danielle Dean: Thank you so much.