Modernizing Global Trade with James Chen of Flexport
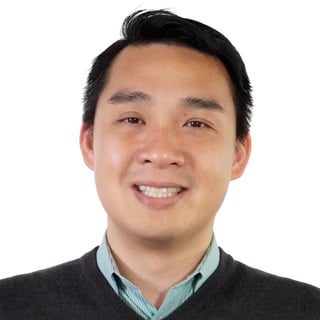
James Chen is responsible for driving Flexport's product vision and technology strategy. He was most recently a Director of Technology at Amazon, and prior was a CTO at both Jenzabar and Rakuten. James holds a BS degree in Computer Science and Electrical Engineering from MIT.
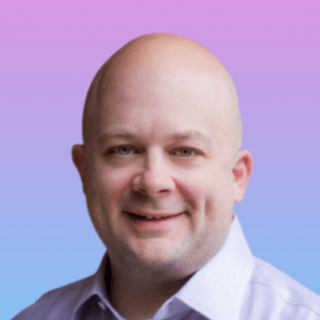
Brad is the CTO of Scale AI. Prior to Scale, Brad was the VP of Robotics and a Distinguished Engineer at Amazon where he was responsible for driving improvements in the safety and autonomy of Amazon’s global operations. His experiences at Amazon as well as Tellme Networks has given him a deep understanding of AI’s engineering challenges as well as opportunities.
James Chen, Chief Technology Officer of Flexport joins Scale AI CTO, Brad Porter, to discuss leveraging machine learning to modernize global trade.
Brad Porter: Well, hey, James, welcome. Thank you for joining us at Scale Transform today. For the audience's sake, I'll introduce myself briefly. I'm Brad Porter. I'm Chief Technology Officer at Scale AI. And I'm incredibly excited to be here with James Chen, who's the Chief Technology Officer for Flexport. James, maybe I'll just start off by asking you to introduce yourself and share with us more about what Flexport does.
James Chen: All right. Yeah, thanks, Brad, for inviting me here today. Again, my name is James Chen. I'm the Chief Technology Officer here at Flexport. I joined about a little over a year and a half ago, leading all the product and technology teams here. Here at Flexport, our mission is to make global trade easy for everyone. And we're tackling a very large scale global problem, where we believe by applying technology, we can really help the entire global trade ecosystem.
James Chen: And we sort of have two parts. One part is really building the technology platform for global trade, where we want to make the entire world's supply chain programmable, and that's through digitization and then applying machine learning on all the data inside of the movement to both understand what is happening as well as predict the future.
James Chen: And then on the business side, we operate a freight forwarding business, which is also helping businesses to move their freight around the world, and through that process, making it really easy for us to help them focus on all the handoff coordinations across usually 18 plus different business or entity exporting, importing goods around the world.
Brad Porter: I think it's fascinating. Scale Transform is obviously an AI conference and we have a very AI-centric, AI interested audience here, so I'd be interested to go a little deeper into how you see AI transforming global freight forwarding.
James Chen: I mean, the impact of AI is going to be very transformative and massive, because I hope people can feel and understand like nowadays our global trade topology is getting more complex and we're getting tons more data. And when I say complexity, maybe three plus years ago, right, typically a global movement is you move a bulk freight from one country to, let's say, the central part of a country like the US, and then you have two weeks distribution on getting the product out to the end customer or different large local warehouses. But nowadays, everybody expects to get the goods within hours or definitely on the same day. So the topology and stuff coming into, let's say, 10s of nodes, but now it's going to be hundreds or even thousands of nodes.
James Chen: How do you coordinate global movement where your endpoint is that much? The second part is with the awesome advancement of technology, we have IoT devices or just a lot more systems that're emitting more real-time information. It's at the point where both the complexity and data, I think it sort of exceeds what humanly can understand very well. I think this is where AI or machine learning can come in here and hopefully extract insights on what are the options we can look at. I'm pretty sure for a while humans will still be involved with making the final decisions, but hopefully, certain parts of the supply chain is predictable enough where it can be on autopilot and let AI make the simple changes.
James Chen: And then a good example of what that means is, we know when there's disruption in the global supply chain, that the customers or the consignees or the retailers, right, they're very agile on moving their network. Hopefully through AI, we can see, oh, okay, if you want to make T-shirts or something, you can start popping and see, oh, maybe there's a trend on sourcing from a different country that you never thought before that can give you a competitive advantage. Obviously, the other one is really the impact of weather on supply chain, whether it's local or global. Those are things, I think, only through AI machine learning that we can a little bit understand the impact. Otherwise, I think as humans, we're just guessing.
Brad Porter: Yeah, I think that's what's fascinating to me about AI. There's this concept that AI you just build the model and then it runs, but these ML, AI systems are always interacting with the real world, and the real world is super messy. I mean, when I was at Amazon last year, obviously, a lot of Amazon's supply chain forecasting is ML-powered now, but all of that had to change when COVID hit.
Brad Porter: I saw a visualization today that showed that there's a significant amount of rerouting of global trade around the southern tip of South America right now, because 10% of global trade is at a standstill because there's a container ship blocking the Suez Canal. Does that kind of real-world disruption affect Flexport and how you think about the models you're building?
James Chen: Oh, yeah, definitely. The big ship is stuck there right now. Fortunately or unfortunately, because our business is so big now, we actually have containers on that ship right now, and we also have containers sitting on the ship waiting for it to be unblocked. And then we have ships that's trying to decide, “Should I go around the south part of Africa or do I go forward?” I think the wonderful thing about working in Flexport and global trade is that a lot of times we hear on the news it's actually very applicable to our business. It's pretty funny and I always have family members asking, “Hey, is that container falling off the ship, is that the one you were transporting?”
James Chen: Definitely, definitely very relevant. But the hard part, right, is really trying to figure out what does that mean. How do we use what you see visually, and then hopefully through technology understand what should we do? That's the most interesting question. We know it's being stuck, then can we create a model, which I think is really hard since this is the first time it happens, where when is this ship going to be unlodged? Right? When is global trading being unblocked?
James Chen: And the other thing that's a little more practical is, a lot of these goods, need to be in a warehouse where people can buy them online. Now you have a lot of companies who might be running on inventory for people to purchase, so that's a big problem. And then before that, we sort of have a trucks schedule or a barge schedule, a labor schedule, or terminals to take these goods from the ship, but now you have no idea when it's going to show up, right?
James Chen: For me, there are definitely a lot of very interesting real-life situations. But what's interesting for me is that actually I feel this problem is not that hard yet, because we actually know where it is. But I think a couple months ago, we've definitely seen containers falling off ships. To me, that's really, really hard because today, we have no idea if one of our customers' containers fell off that ship.
James Chen: The only way to verify is when that ship docks itself, and then when they start pulling containers off, then at that point you know that, “Hey, is it on there or not?” And remember, there's insurance, right? There's capital. There's restocking. There's so many business decisions that need to be made. Hopefully someday, we can have a good model to say, “Yeah, there's an 80% chance it's on there or 20% based on the position of the container.” Those are things I think that can really help out to improve that in this space.
Brad Porter: Yeah, fascinating. Hey, I want to dig a little bit deeper into your background. As we were preparing for this event, we were sharing some of our shared stories of MIT. And we were both fortunate to take our kind of intro to artificial intelligence class with Professor Patrick Winston. And so I'm curious what you remember for that course and really how you've seen AI change in the last, well, I guess in the last 25 years really?
James Chen: Yeah, definitely remember taking a class from Professor Winston. I mean, one thing I remember is that it's amazing how much knowledge he has in his head. And he can go through the whole entire lecture without looking at notes, so he really understands what he's talking about. But I think from that class, I think I really learned the fundamentals of AI, whether it's rule-based, expert systems, breadth, depth search, min/max, alpha-beta.
James Chen: I think a lot of these things that people take for granted that 25 years ago these are sort of leading ideas that can be done. But the thing that I learned, which is still very applicable today, right, is that you have all these different framework algorithms or tools in my mind, it's really important to understand what is the fundamental problem you're solving and then pick the right tool to apply to it to get the best result.
James Chen: And I think with the speakers earlier today, right, that's sort of what they talk about. Sometimes the algorithm could be good and you need to fix the data, right? Sometimes the data might be bad and you need to change the algorithm to take advantage of maybe the sparse dataset you have. I think learning what to pick is probably the most important thing I learned in that class.
James Chen: But what has changed in the last 25 years is really the practical applications of the AI field. There is also an earlier example, right, there's like medical screening, self-driving cars, infinite worlds in games nowadays, you have an AI engine that can sort of simulate life, it makes it very entertaining.
James Chen: And the part that I like the best is really more about creating these realistic arts and videos. I thought it was a pretty cool way of applying AI to say, “Hey, let's create art that looks like Monet or Van Gogh,” or something like that, and it's pretty good. It's great to see that it's becoming easier and easier to take advantage of all the AI advancements in this world.
Brad Porter: Yeah. I mean, what I remember from that class was reviewing another set of students in the class. They were presenting their project and they had built, solved the robotics control problem with a four node perceptron.
James Chen: Oh, wow.
Brad Porter: These days, the deep networks are quite a bit bigger. But it's incredible how much has advanced.
James Chen: Yeah, definitely.
Brad Porter: Beyond just the shared kind of MIT connection, there's a lot of similarity. You and I have both done, have been entrepreneurs working at startups, and then both had the opportunity to lead big teams at Amazon. I'm curious what you take from each to your role at Flexport now?
James Chen: I think, yeah, it's great that we share both working at a small company, super agile experience, right? And then working in a large company, try to remain agile as you scale, so definitely a lot of learning. In addition, to me, the part that's very interesting is that technology is always evolving, right? Going back to my first startup in 1999, I think we use things like PEARL, co-fusion. You had to install and set up your own servers.
James Chen: And then like the second startup, I sort of moved into the LAMP stack, PHP, MySQL, jQuery. And then even more recently, I think here at Flexport, we used Ruby and then Postgres and then maybe DocumentDB related. For me, technology is always changing and it's really important to make sure that you are always in tune on what is the latest and then make a really good judgment on, “Is this something we should use for the startup or is it too early?” Right?
James Chen: Because you don't want to spend time playing around with the tools and not really figuring out how to build a business. I think on the startup side you really need to be able to learn stuff quickly and see how it can apply to the problem you're solving for your startup. And then at Amazon, even Rakuten, I got a chance to meet a hundred and also a thousand people.
James Chen: It's trying to figure out how you coordinate that many groups and teams to go after the same mission or product line, right? As you get bigger, as your product and service offerings become more complex, you sort of have to figure out what things you want to standardize, what things you don't want to have duplication or nonproductive duplication of work, right?
James Chen: Sometimes it's always okay, but a lot of time you should not. Meanwhile, you want to remain agile in trying to make sure that you create an environment where each team can be autonomous as possible, where they can do full end-to-end ownership of the service. But as a whole, it still runs, right? I think that's sort of the, I think the thing that we probably learned at Amazon.
James Chen: And then here at Flexport today, the thing that I learned is like, yeah, we should always … Luckily we're pretty well-funded and we're pretty big right now where we always need to invest a little bit into the future R&D to make sure that we put enough time to evaluate proof of concept of the latest tech versus saying, “Hey, let's just keep on going. We know how it works, let's push on this.” This way we can stay relevant and not fade away.
James Chen: And then in terms of scaling, we're planning to grow from around 425 people in my org today to 650 plus. We're hiring 250 people in scale. And what does that mean? Well, it means our IT and product infrastructure, right, needs to be also uplevel so that each of these different teams we provide can remain productive.
James Chen: And of course, it's really hard when you're in the transition that you definitely need to slow down a little bit before it can go fast. That's sort of what's happening at Flexport. But how about you, Brad? What are the two or three things that you found to be pretty interesting learnings from your startup in Amazon?
Brad Porter: Yeah, it's really cool because I am finding that I'm tapping both the things I learned in kind of the first 10 years in Silicon Valley hypergrowth and things I learned at Amazon. I think one of the things you talked about at Amazon, just allowing for empowered teams to move very quickly, it's something Amazon's done really, really well.
Brad Porter: And I think it's a key thing that as I've come into Scale, I've wanted to reinforce and accelerate, like how do you make decisions that unblock teams quickly? And yet at the same time with a startup, you need to be able to swarm. You need to be able to scramble, right? You need to be able to jump on a hotspot very quickly, right?
Brad Porter: You don't have mature systems everywhere yet, right? And so problems can emerge and you need a team that can both … So trying to balance that, giving everyone autonomy and independence and empowerment to move quickly with the need to be able to swarm flexibly is a key thing that I'm building alongside my leads in my team.
James Chen: Right. And it's also really hard to maintain a right schedule, right? You're also trying to build a service and product out there, and then meanwhile, short-term comes in it's … As a startup, you don't have all the resources like Amazon, that tens of thousands of people can come in. And if it's that important, you immediately can do it, but as a startup, right, it's always hard. You have to make that trade off on, “Is it important not to swarm on this now? And what's the impact?” And it's hard. There's no right answer, just have to make the best judgment as it happens.
Brad Porter: No, exactly. The other ingredient I'm bringing, obviously, and I think it's not just that I'm bringing it to Scale, one of the things I selected for in deciding to make a move from Amazon was to find another company with a similar commitment to customer experience, right, to customer obsession. And I know Flexport talks about that a lot as well, and I'm sure that's deeply ingrained in you. How are you thinking about customer obsession as you shape the technology at Flexport?
James Chen: Yeah. People know, right? This is one of the few remaining large industries where not too much tech adoption. As an industry, there's definitely companies who're really, really good at it. And then when Flexport came into the space, we put the customer as front and center on what they want to see, versus what's happening behind the scenes to get the work done? Because I think a lot of the historical user facing applications come from that view, right?
James Chen: “Hey, we have these data, let's show it. We're doing this process, let's show it. Oh, let's treat these, the ocean, the air, the trucking, very differently, right? These three different business units doing all that stuff.” Well, but from the user's perspective, with that sole focus, we actually have … We measure NPS scores and C-SAT scores to make sure that we try …
James Chen: Although it was very fuzzy, right, but it gives you a really good indicator that are you doing a good job or not? And whenever there's a pain point that pops up, I think if you constantly solve it, then the score should remain high. That's sort of one of our temperature checks on how well we're doing, a measurement of how we're doing for the customer.
James Chen: But through this process, right, we understand that at least for the global trade customers, they really care about intent, visibility, right? When an exception happens, what do you do? They need real-time collaboration, messaging and reporting on the things they care about. So you feel these types of things are fairly generic, but historically, those are all very fragmented systems where if you're a shipment have an issue, right, like, “Oh, let me go call the trucking team at Origin, right?” versus saying, “Hey, you have an application that can really show you, no matter what mode you're doing, a really nice unified user experience.”
James Chen: And with the COVID hitting where people are working from home more, luckily with this approach, we saw our usage increase by 22% as a general daily active user, and Q3 of last year I think increased by 50%. So essentially everybody, right, has to figure out how to use the tool to get information they want, because you just can't go to the building. You cannot talk to another person, getting information in-person anymore. So I think that's really, really important. And I think we do solve the problem a little bit differently compared to others in industry. Yeah.
Brad Porter: No, I mean, it's fascinating because Flexport really has thousands of customers who operate in their own way, right? And the space has a lot of unstructured data, paper data. How do you get all that into your systems and use that to drive the efficiency and logistics?
James Chen: Well, it's really every way possible. We have to take in manual entry, text files, Excel files, API. I mean, luckily there are standards like EDI where I think the larger comp carriers out there or freight forwarders, we can send data across electronically, right? But we are picking and delivering goods in some of these countries where our partners might not have the best systems, right?
James Chen: As a design, we really need to figure how to turn these unstructured even physical documents into a digitized format in order for us to do something. We've been trying to solve this problem by creating our own standard and creating our own Flexport language of global trade. Hopefully, as we communicate to different parts of the world, we're using a common way to describe things.
James Chen: And meanwhile, one of the biggest pain points in this space is all the documents, like all the legal documents you need to do to, to make things float very efficiently and applying sort of AI, machine learning to digitize these physical document, maybe you can scan PDFs, right, into structured data, is really, really important.
James Chen: And then the other thing we're doing in terms of product portfolio is that this last month we launched order management. Order management is like a step before all the shipping happens, right? For us, that's really important for our customer, because what they want against the end-to-end view, from their purchasing all the way through shipment … So we start with shipment, right, but now we're expanding our reach to make sure that holistically now we can tie all of our shipment data to the skew level of their inventory, and calculate all their costs.
James Chen: Even though everybody does it differently, I think there is an interface or language that people use to communicate with one another. And our goal is make sure that we deliver the application for the end user to easily, manually digitize everything, right? We build the backend services to process the ones out. If we can leverage technology, that'd be great.
James Chen: But ultimately, we want to create that tech platform where everything is programmable and we made it so easy for any company to share data across the globe. That's our goal, right? And on top of that, of course we can have collaboration messaging so that humans can always step in there to resolve stuff that we can automate. Across the board, no silver bullet on solving this problem. So yeah, a lot of interesting challenges.
Brad Porter: I think at Scale we're excited to be able to help Flexport with some of your most ambitious projects in AI. How do you think about where to partner and what to try to keep in-house?
James Chen: Yeah, that's a really good question, right? Because working together like, oh, when maybe Flexport will take it ourselves. But just like any business, especially a startup, you have to think about what is the true intellectual property that we're building, right? What things are we creating that provide enterprise value for us, for our users and our customers?
James Chen: And for the field of AI, I think Scale is set up to help Flexport scale. This is all you guys do, hopefully you guys are learning the latest and best on all the different algorithms for us to easily experiment, and as well as the operations to do the first step of the process, like labeling. Working together we need to identify what are the meaningful attributes that we need to label and digitize.
James Chen: I think our focus is around how can we be that domain expert in global trade? Just like I think Andrew Ng talked about how as you see things moving forward there is this need for sort of vertically integrated applications who are very domain specific. And I think Flexport, our goal is to be that domain expert for global trade and global supply chain, right?
James Chen: And leveraging AI is definitely a tool we really, really want to do. And hopefully, we're partnering together to really see how collectively we can tackle this and get to the end faster, right? Because the other way is like, “Oh, you know what, Flexport we can build our army of 200 machine AI data scientists and maybe do almost the exact same work.” Maybe five years from now, who knows?
James Chen: I mean, we definitely want to do our own experimentation, drive our own innovation, but our problem to solve is in the space versus generically for everything. I think that's one of the big differences. And carries us over to even like a financial system, right? Like shall I build an ERP ARAP or should I leverage one? Each one of these decisions, I think if there's a great partner that can do it really, really well, and the way it's structured is mutually beneficial.
James Chen: I think a partner is always a good way to go. Especially if it's not in your primary problem you're solving. So then this way you can focus your limited resources on what makes the biggest impact for your company. But so far so good. I really love working with Scale so far.
Brad Porter: No, we've really enjoyed the partnership with Flexport and look forward to continuing for a long time. We have some questions coming in through the livestream chat. And so folks, please go ahead and ask questions for James or myself, and we'll field some of those questions in kind of the next 20 minutes.
Brad Porter: One of them comes from Teddy, which I think ties into what we were just talking about. But obviously, I'm going to paraphrase these questions a bit, so apologies to the question askers if I reframe your question and miss the intent. But just to try to keep it all in context, I'll kind of reframe things a little bit.
Brad Porter: But obviously, this kind of AI transformation is not just happening in startups and not just happening in big tech companies like Amazon. But bootstrapping is hard, right? It's a different kind of discipline. And so as you talk to industry peers who are trying to figure out how to bring AI into their businesses and into their practice, how do you encourage them to think about adoption and where even to start on the software and the people and the hiring?
James Chen: Yeah. On how to start, right, I think … I mean, luckily, there are many, many companies out there that package it pretty well like a meaningful application. And I think chatbot, like the one I mentioned earlier, you have your support team, and again, there's like … Historically, you sort of do level one, level two, level three, and then maybe you can apply AI machine learning on your knowledge base and see if you can try that. And if it's really successful for your domain again.
James Chen: The beauty of this whole entire thing is you don't know. Even though it works, it might not work for your case or your scenario. So the only way to find out is really to try, and hopefully the cost of trying is becoming lower and lower. That's number one. If you don't have a large budget to build your own technology team, a data sciences team and stuff like that, I think trying these applications would be a great way to start.
James Chen: But if you, which I hope everybody will, treat AI and machine learning as a competitive advantage, then you really just have to hire one or two people who really love this area. And who's in tune with all that's happening, and get to a point where you feel there's somebody within your organization that can provide very filtered advice on what area to pluck in and try out within any of your applications.
James Chen: I think that will be how I imagine a good way to start that. I do hope almost every company, even if you're a startup, right, I think if you have a team of five, hopefully just one of them is really into the space, and start reading and pulling in ideas. And you can always say it's too early for us to try, but at least there's somebody that is bringing it to the table when you have discussions. I don't know. Yeah. What do you think, Brad?
Brad Porter: Yeah. I also thought Andrew Ng made an incredibly important point this morning, that if you have small amounts of data, it's really important that those labels be super precise. But even if you have large amounts of data, but you have long tail effects, it's really important that those labels be incredibly precise.
Brad Porter: And so obviously, that was a lesson I learned at Tellme Networks when we were training voice recognition systems and grammars. And I learned at Amazon, repeatedly, the quality of the data inputs matters so much. Obviously, I believe that thesis fundamentally that I came to Scale to help a lot of the most ambitious AI projects to achieve that. But I do think, I think Andrew made a compelling point.
Brad Porter: Like really you're programming these models with data, right? As he's pointed out, people want to keep tuning the hyper parameters, but at the end of the day, once you've got those reasonably tuned so they hear that your model is learning, then the question is, what is it learning? Right? Recently I looked at a data set where it had birds labeled, and so we typed in woodpecker and a whole bunch of hummingbirds showed up.
Brad Porter: And I don't know if that labeling was wrong or because hummingbirds were never really presented as an example, but there's no way that that system is going to learn to differentiate hummingbirds from woodpeckers if they're not precise labels that teach it that, right? And so I do, I feel like AI/ML is a fundamentally … There's a huge amount of software engineering that goes into setting up the end-to-end pipelines and the data flows, but at the end of the day, you are trying to learn a function from data.
Brad Porter: And so the emphasis really has to go into how do you have incredibly high-quality data that you're inputting into this model? And that can cause really the difference between whether a system can go live in production or not, right, is the quality of those inputs.
James Chen: Yeah, I have a question for you, because I think you brought a good point about the hummingbirds, woodpecker, right? I think a lot of people believe like, “Oh, once it gets working, right, it's going to … It's great. You can leave it off, maybe everything is automated,” but that's not the case, right? Based on what the next data stream comes in, the whole entire thesis could be wrong.
James Chen: Are there good practices on like how do you monitor it, when you monitor it? Because one of the benefits like, “Hey, I can be a little bit hands off the wheel, but then if you're too hands off the wheel, don't look at it.” The whole thing where the AI can really take a huge wrong turn. I'd love to hear like what are some of the best practices on a monitoring this. There's a point getting it up and running, right, put in production, but then how do you maintain and drive the thing?
Brad Porter: Yeah. No, I mean, it's a continuous … This is what I mean by AI systems that don't interact with the real world and don't interact with something that's changing, they don't have to change, right? But that's not most of the interesting AI applications in the world. Most of the interesting AI applications in the world are dealing with a changing world, right?
Brad Porter: And as a result, you have to be constantly training and adapting your models to different environments or to the changes in the environment. And so, yeah, it ends up being that it's not just like getting the model built. As Andrew said in his thoughts, it's like that's maybe 50% of the way there, right?
Brad Porter: It's bringing that in, continuous learning, active learning, or just a continuous refinement of the process so that as new events occur, either the system learns or you learn that the system has gotten stuck. It doesn't understand why everyone's buying toilet paper right now, right?
Brad Porter: And so, yeah, it's absolutely critical that the … I mean, I saw this a lot in robotics. There are amazing demonstration videos of robotics. I'm a big fan of Boston Dynamics, I'm super impressed by their capabilities. But it's always interesting, like there's a wired article that talks about when Atlas did that parkour course. And it mentioned that it can do it correctly once out of 20 times, right?
James Chen: Oh, I did not know that. Oh, interesting. Yeah.
Brad Porter: Yeah, everyone sees the video, now what was really impressive in their latest video this year was that they, and I don't think people noticed this either, that they had about, I don't know, six or eight different robots interacting and it was a single cut. They did not keep cutting to different dancing robots, it was all one scene.
Brad Porter: Now, if you watch to the very end and then they cut to a few little sequences at the very end. But the point is, you end up having to be somewhat skeptical of that first model result, right, of that first interaction. Because again, if it's not continuous and interacting with the real world and dealing with some of the changes and dynamism, then what you've done is solved … You've fit the function once, right? But again, the world is evolving and changes.
Brad Porter: Yeah, that's why I was super interested in kind of the Suez Canal and how that's affecting your work. Well, here's a couple others that have come in. Fast forwarding five years when Flexport's able to process all of these paper and digital documents, what are some of the most interesting use cases you think that enables?
James Chen: Yeah. Five years out, it gets almost fairly practical. One interesting one is, today, a lot of our system is built to be template-based or rule-based systems, where you sort of predetermine what a workflow should be. Again, even though everybody, every operations team can do everything differently all the time, right, but then in terms of building a system, we sort of have to structure it, right?
James Chen: We have to create a rule-based system that triggers based on business logic or events and stuff like that. Reality is that I think once we can digitize all the information and capture the steps of actions that's happening inside a building or as a freight forwarder, coordinating freight, it would be awesome to have this AI sitting behind the scenes observing what people are doing and they can ask why.
James Chen: I don't need to have somebody to go back and change the rules behind the scenes to change the workflow, right? The AI will say, “Hey, for some reason, everybody keep on reassigning this freight to this port, from trucking company A to trucking company B, right? Why is that?” I don't know, but it seems like it's happening, right? And it needs to be smart to say, “Oh, maybe the cost is lower. Maybe the other trucking company ran out of capacity.” I don't know.
James Chen: But today, those are human judgments and then maybe when we need to go back to our system to create that new rule for routing, or a lot of time if it's short-term and not sustainable, you do a manual call and direction, right? To me, I think five years now the exciting part is that I think if AI is in every single touch point, it's going to self-suggest and learn and tell you what should be done without you telling what to do.
James Chen: And that's going to be massive savings on what's going on. And if Flexport is that platform for global trade where we see across multiple different carrier, freight forward or customers, right, if in aggregate we're helping each other understand what's happening, it would be interesting to see if this AI can provide options that otherwise if you're just by yourself or you're limited data view, you don't know there's a better option, and I think AI with all this data should be able to show you.
James Chen: Oh, and then another one just came to my head. With all these data, another thing that's really interesting for global trade is that we are objects. We're making sure we are compliant and we follow the latest government rules. So whether it's tariff, like you have to pay more money or whether they're blocking a certain type of goods, again, through AI and machine learning, right, if you suddenly see a certain type of shipment being blocked or screened more and more and more, right, the AI should go in there and see like, “Oh, across all these different shipments, this is happening, why?” Is it because there's a government policy change too?
James Chen: In addition to operational issues, I think there's a lot of government rules that institutes it and now you need to adopt really fast. And today what happens? Well, one, you don't know about it until it's way too late, right? So it could be days before you find out why it's sitting there. And two, you don't know what the solutions are, and through AI, maybe you can see, “Oh, how does a couple of these companies solve that problem?” and you can bring that automatically into our own workflow and help our customer to move stuff around. So yeah, I think in five years it should be fairly possible to do that, the suggestion part.
Brad Porter: And how do you think about the ROI, the return on your investment, and which investments to make an AI? I mean, there's a bit of an imprecision in exactly what kind of performance you can achieve. I'm curious how you thought about that in global logistics and document processing. Yeah, how do you think about which of those investments to make?
James Chen: Yeah, there's different areas we can apply. But document digitization is a really easy one, right? Because the economics is you can do it on a per document level to say it takes a human X number of minutes to get 100% accuracy, or if they go a little bit faster, right, maybe human accuracy is probably not 100% either, right, it goes to like 90 something.
James Chen: And then based on the labor, you have a dollar amount to say it costs X dollars to process the document. And then applying machine learning with what we believe is a target, a percent accuracy, we can just do simple math, right? Like, be able to digitize to 95% it's going to save us 90 minutes per document out of 10, right? So then you can do pretty simple math. I think at least in logistics, there's a lot of these very tactical tasks where it's very clear there's a simple ROI that you can do.
James Chen: I think the harder one would be around the automatic planning and routing, because I think there's way too many other factors involved. A good example would be like SLA, right? We believe if we package this together into something, it's going to go faster, maybe at a lower cost, but reality, maybe not. You can put in mathematics this thing … But for example, there are many companies out there today, I'm sure, who just consolidated a container at Origin, somewhere in Southeast Asia, right?
James Chen: And they're putting it on the ship that's going to the Suez Canal right now, you know it's being stuck. For me, yeah, we can build all this technology, all the AI, right, it's going to automate it for you, right, but then is it the right thing? To me, there's stuff like that where it's hard to tell is it the right solution to that problem.
James Chen: And what is ROI? I don't know. Their ROI is to reduce labor, but then I think human intelligence, making a judgment call might be better in that case. I think there are a gamut of very clear ones and then very fuzzy ones. But occasionally, as long as we can ultimately use it to better our decision-making, I think it's worth it to a certain amount.
James Chen: But from a CTO view or a company view that's sort of using technology as a main strategic advantage of that, you just have to allocate experts on your budget. You know it's just experimentation and you go for the one that you think has the biggest impact. If you don't get anything, it's fine, right? It's just the way it is. There's no guarantee in this area, but you have to invest. That's the most important part.
Brad Porter: Yeah, I think about that a lot in AIML projects I have managed or been part of is, how do you speed experimentation velocity? There are a lot of different techniques. But increasingly, for me, it's coming to how do you speed data curation, data cleanliness? Once you have a set of experiments that look like they're within range, then so much of it becomes about the data that you have underneath.
Brad Porter: I want to go a little broader in our last few minutes here. The world of transportation is changing. Not just through global freight optimization, but autonomous vehicles, drones, integrated supply chains, just-in-time manufacturing, just-in-time delivery. How do you think of all that evolving kind of over the next 25 years?
James Chen: Yeah, I think it's going to be super, super exciting because of both the AI technology as well as physical hardware that exists in the compute power that you can do on a tiny, tiny little chip. Yeah, I think 25 years from now we should be able to model the entire global trade like a living organism. Like literally every single spot you know what's happening. And there's feedback on what's happening. And then not to a level of SkyNet or anything like that.
James Chen: I think there will be a system out there. There will be a technology platform that can act as the global real-time traffic cop on giving every single location on what you should be doing, right, like, “Go this way. Do that. Drop this off,” because you have this entire near real-time sensing or communication of data from one end to the other. And that's going to really help you hopefully position resources a day, a week, a month ahead of time before it needs it.
James Chen: And meanwhile, when things change in real life, right, everybody in an entire ecosystem can really sort of adjust already in real time. I think that's really, really cool. But then on a practical level, right, speaking of the Suez Canal, I believe 25 years from now, when that ship is going to a channel, the captain of that ship on their dashboard say, “Warning. Warning.” 90% chance you will get stuck, right? You're like, “No, I won't,” push the button and go through, or it's like, “Oh, really? I didn't know about this,” right?
James Chen: And then maybe some other groups can say, “Oh man, I don't know why this guy is taking this risk. He's going to go down,” and then some other team's like, “All right, let's prepare 30 tugboats, 20 diggers. In case it gets stuck, I'll be able to sort of take it out.” But what's happening today, right? You have like one little digger, I love that picture, where it's trying to solve this big problem.
James Chen: And then you have the swarm of everybody trying to help out. I think 25 years, hopefully because of all the data, we know percent of risk, and then potentially have a way to create contingencies in case it happens so you already have a game plan in place that you can activate, right? It doesn't mean you need to do anything, it just means you have the plan in case it happens. I think that's going to be a really exciting role.
James Chen: And the thing that I feel is not going to be able to make the reality happen, right, it's still the same thing because of the silo of data. Or in global trade, the hard part is really the, for good reasons, right, there's different country-specific policy. So there's stuff that you want to share, you don't want to share for privacy security, many reasons, right?
James Chen: Those are the major blockers for us to achieve that vision. But for a private enterprise that have their own assets and they control some of that, I definitely think it's really possible, and that's a really exciting role in logistics and freight forwarding.
Brad Porter: Yeah, I like your idea. It's not just engineering teams that need to be able to swarm. There are global bottlenecks that you need to be able to swarm, and these systems can help make that faster and more efficient. Well, hey, thank you so much for taking the time today, James. And really enjoyed the conversation, really enjoying the opportunity to work with your teams at Flexport, and so thank you so much.
James Chen: Yeah, and thank you, Brian. It's great working with you again in a different context and, yeah, let's continue to do exciting stuff. And thank you for inviting me.
Brad Porter: Awesome. Thanks everyone.