A Responsible Approach to Creating Global Economic Opportunities With AI
speaker
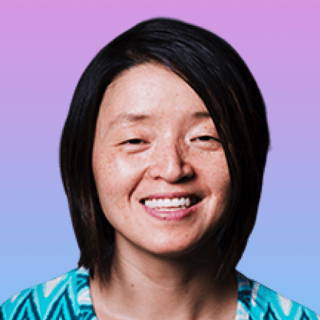
Ya joined LinkedIn in 2013 and has since truly helped make LinkedIn a Data First company. She leads an exceptional team of talented data scientists whose work covers metrics, insights, inference and algorithms and they tackle data science challenges across product, sales, marketing, economics, infrastructure, and operations. This centralized group has 300+ data scientists distributed across US (Sunnyvale, Mountain View, San Francisco, New York), India, China, Singapore and Dublin, Ireland. Ya is passionate about bridging science and engineering to create impactful results. She and her team try to keep LinkedIn on the cutting edge while ensuring that its A.I. systems avoid providing biased results while maintaining user privacy. They help the company take active responsibility over the data they collect to ensure fairness and protect privacy. In addition to her work at LinkedIn, Ya’s contributions outside of her day job, such as the book she co-authored on Experimentation and her Stanford commencement speech, are meaningful to the entire industry as well as future Data Scientists. Before LinkedIn, she worked at Microsoft and received a PhD in Statistics from Stanford University.
SUMMARY
Ya Xu, VP of Engineering and Head of Data Science at LinkedIn discusses how LinkedIn is applying responsible AI to create economic opportunities for every one of the nearly 800 million members of their platform, across 200 countries worldwide. She shares how AI can be most impactful in creating opportunities and focuses on best practices in fairness and privacy to build a better and more equitable user experience.
TRANSCRIPT
Nika Carlson (00:16): We're delighted to welcome Ya Xu. Ya is vice-president of engineering at LinkedIn. She leads a team of 300 plus data scientists who work on the cutting edge data science opportunities at LinkedIn. She is responsible for using machine learning to keep LinkedIn the popular platform, we all know and love, while ensuring that its models reduce bias and respect user privacy. Before LinkedIn, she worked at Microsoft and received a PhD in statistics from Stanford university. Ya, over to you.
Ya Xu (00:52): Hello everyone! My name is Ya. I lead data science at LinkedIn. Today, I am super excited to share with you some early progress that we have in the space of responsible AI. This is probably the most important slide. This work is not possible without the work and collaboration across several teams across LinkedIn, the responsible AI team, the applied research team, the big data engineering team etc. So really big kudos to a lot of individuals across LinkedIn. So, before we get started, how many of you have watched the Netflix movie, The Social Dilemma? Well, I don't necessarily agree with everything that was portrayed in the movie. One thing that was loud and clear and hopefully resonates with everyone working in our field is that AI and tech applying a increasingly critical role in the society, and there are immense challenges if we don't play the role responsibly.
Ya Xu (02:00): So what role does LinkedIn have to play in this as the world's biggest professional social network? LinkedIn has become the platform where hundreds of millions rely on, for economic opportunities, such as learning a new skill, applying for a job, getting a referral, building a network, and all these are powered by AI and technology. The [inaudible 00:02:23] impact we can bring to the world is tremendous. For us, is not enough to just build a good product, ship it and go home. As technologists, we ask ourselves on a daily basis, what does it mean to build AI and technology that puts our members first? If our vision is a guiding principle in our design process, then what are some of the considerations that we need to take into consideration as we build our technology and AI systems, and we call this responsible design.
Ya Xu (03:01): One of the core pillars of responsible design is responsible AI. We follow Microsoft's responsible AI principles and the six values that we strive to build into our products. So in addition to the six values, it is really important to emphasize that responsible AI is about both intent and impact. For example, it is important to make sure that the training data has the appropriate demographic representation and model performance passes specific fairness criteria. But that's not enough. Ultimately, it's about impact. It's about how people are interacting with the product, the value and outcome they are gaining. It's about putting our members first. In the rest of the talk today, we're zooming to the two areas we have made the most progress on fairness and privacy.
Ya Xu (04:09): Starting with fairness, let's actually do a little warmup. Can I just ask everyone to pause for a little bit and think about how you would define fairness.
Ya Xu (04:25): While the exact words you may use are differ, the sense of fairness is actually very deeply rooted. I would say that one of the most common used words between my six year old and my nine year old, when they get into argument, is that it's unfair. Even monkeys revolted against their boss when they are not paid equally for the same task. So while the definition of fairness is simple, can I then challenge you to think about how would you then go about quantifying it? Can you come up with a mathematical definition? And this is why that this fairness problem is a hard problem to solve.
Ya Xu (05:11): So before we any deeper, it's really also important to mention that even though that we're going to be focusing on talking about algorithm fairness today, fairness, obviously goes beyond just algorithm. If we take a member centric view that people care about, is the overall fairness, regardless whether it's introduced by algorithms or presentations on the platform, or even just copying the wordings that's used.
Ya Xu (05:40): And now LinkedIn, the way that we put fairness into practice really involves three steps. Number one, audit and assess existing product and systems. Number two, mitigate unfairness on the platform and build and number three, build into our development process as a default and continuous detection and monitoring.
Ya Xu (06:08): So let's walk through with one concrete example. So how do we improve fairness in the connection recommendation, also known as PYMK - people you may know. So I know that you are already users of LinkedIn and if not, drop me a note so I can come and see you. So you are familiar with this product where we suggest other LinkedIn members for you to connect with, with a simple click of a button, you can send a connection request, and if the recipient accepts the invitation, then voila, congratulations! You are now connected and you can start talking and interacting with each other on the platform. Just to show you how critical this process is to our members' success. Our research have shown that applicants are four times more likely to get a job at a company where they actually have connections. And through analyzing hundreds of [inaudible 00:07:15] of experiments. We also realize that when LinkedIn is able to increase members' network, openness or diversity, they're also up to 15% more mobile in the labor market.
Ya Xu (07:25): On top of that, PYMK the connection recommendation that we just showed, actually accounts for 40% of all total connections that's made on LinkedIn.
Ya Xu (07:39): So, I hope it goes without saying more that it's really important that we evaluate and improve the fairness in the PPYMK algorithm. So, this is how we're going to do it. We start by analyzing representation at every step of the AI decision funnel. Do we have gender parity when we generate candidate pool for the recommendation? Are male and female equally likely to receive an impression? How about getting an invitation and accepting an invitation?
Ya Xu (08:17): So one really challenging aspect about measuring whether everybody's representation is fair or not, is really to know what is the reference distribution. And here we are address it using what we call the funnel survival ratio, which we essentially use the previous step in the funnel as a reference to see if the representation has changed from step to step. As you can see from the data here, what we discovered is that even though our algorithms don't seem to introduce any disparities in PYMK, as males and females are actually equally likely to be seen on the recommended list. However, females are more likely to be invited and are less likely to accept invitations.
Ya Xu (09:15): So representation analysis is a good step one, but it's still just looking at the numbers in aggregate. Let's go back to our commitment of equal opportunity for equally qualified members, and let's break it down a little bit. So in this PYMK recommendation, we say that we give someone an opportunity to be connected when we show their name on the recommended list. So here, the opportunity is really determined by the algorithm scores, right? The higher the score, the more likely somebody would be on the list. On the other hand, the best evidence that we have that would indicate that this candidate is qualified is when my invitation is sent and a connection is made. In other words, through outcome.
Ya Xu (10:14): So putting in this all together, in the algorithm ranking setting, where essentially asking, do we see equal outcomes for equal scores? So now with this translation, we can go about quantifying it, right? And here are some results.
Ya Xu (10:37): I also just wanted to mention that this concept of all contexts is actually not new. It was first introduced by economist Gary Becker in his 1957 book. So applying this concept and be able to quantify that for PYMK, we can see that for a given score bucket, we actually solve very similar outcomes between the gender groups. Again, the outcome measured by the invitation acceptance rates.
Ya Xu (11:09): So in the intent versus impact framework that we introduced earlier, the two assessment approaches that I've shared so far really has a very strong focus on the impact aspect of it. And the third approach here is certainly a lot more focusing on measuring the intent, evaluating how the models perform differently between gender groups. Do we have sufficient gender representation, training data, other differences in precision recall and AUC etc. Again, for the most recent PYMK model that we found the differences to be very small.
Ya Xu (11:48): Now on to mitigation. So the approaches that we take for mitigation can be broadly put into three categories, adjusting the training data and the training model itself, and also adjusting for the model scores after the training is finished. So I'm not going to go into depth on each one of this. However, I do like to share a couple of learnings, first of all, re-weighting the training data, so that we can make sure the training data is representative may seem the most obvious approach.
Ya Xu (12:27): It's actually also just very often mentioned in the literature. It turns out that it's actually not as effective at improving the model towards passing out contest. Re-rankers which is falls into the third bucket of mitigation approach, it tends to be the easiest to implement across different models, across all the different approaches, and can be very effective actually to be achieving our contest. Even though that it may not be the most optimal when it comes to balancing both the model performance, which is really the precision, recall, and AUC for example, and also the fairness outcomes. While it's important to assess the existing products and intentionally improve any fairness gaps we have identified, it's even more important that as we are evolving our product with every change that we introduced, we are also doing it responsibly, right? Because we are constantly evolving and changing our product all the time.
Ya Xu (13:37): And so we really need to build fairness, monitoring and detection as a default into our product development cycle. That's the third step out into this process. That's why we actually turn to the most powerful tool we have, experimentation. So a little bit context taking a step back experimentation in particular. So at LinkedIn, all our features actually go through every testing, regardless whether it's a UI feature, whether it's an algorithm changes. Because of this, experimentation really provides us the platform to leverage, to be able to introduce this sort of fairness awareness in every changes that we make. So our goal is to ensure changes that we make can really benefit every member.
Ya Xu (14:38): So both of these two aspects, number one, we not only wanted to evaluate whether certain interventions that we have introduced work well at closing the gaps we have identified, but it's also equally important to be able to use this fairness aware experiments to evaluate whether we are introducing any unintended consequences as well. We are doing by not only looking at treatment effects, but categories and also other important attributes such as network size, but also whether we are shifting the distribution of opportunities. And I will go into a little bit more detail in the next few slides.
Ya Xu (15:23): So when we are looking at experiment results, we don't just look at the average treatment effects. So let me illustrate with a toy example. So now you imagine that LinkedIn has 10 members and every one of them actually receives one invitation a day. So now I have two different versions of a product change. So these are core two treatments. So they both were able to increase invite by one invite on average, right? However, one treatment benefited all 10 members equally, but the other benefited some members but hurt others. So we want to be able to capture, we want to be able to capture that and catch those unintended changes as one that is certainly much more unequal than other.
Ya Xu (16:20): And we do that using what we call, Atkinson's index. This again is another classical concept of economics that measures income inequality, and it can be applied to any metric, not just the invite send metric. So now we have this metric and every experiment is automatically checked for this inequality matrix so that we can detect any unintended consequences as we continuously evolve our product.
Ya Xu (16:58): I hope by now that you have a better understanding of the work we are doing on fairness, and even though we've only covered PYMK. There are certainly many more products and alogrithms that we work on. It's a challenging space for sure, and I wanted to say that we are certainly still very early in the space.
Ya Xu (17:23): Now I'd like to actually switch gear a little bit and talk about the other important pillar in responsible AI. Data privacy. As we were talking about fairness, you are probably already wondering, there's a lot of sensitive data that we need to leverage in order to measure and improve fairness on the platform. So how can we respect and protect the privacy of our members when leveraging all the data that they entrusted with us? And that is the challenge of data privacy. So you are not alone for asking those questions. Airbnb actually published a white paper last year showcasing their solution of addressing exactly that question. So even though that we are taking a different technical approach at LinkedIn, however, the overarching mission that we all try to achieve in data privacy is the same, and it's simply figuring out how we can utilize data while protecting the privacy of members. The balance of data utility and privacy is really a very complex one but it's really critically important to get right.
Ya Xu (18:48): So everyone in the audience should have already been trained, not to give out sensitive information such as your social security number, but did you know that for 87% of people in the United States, a hacker can still reconstruct your identity with just a combination of your birthday, your gender and your zip code. That is why traditional techniques such as obfuscation and K-anonymity are not sufficient to defend our members data privacy. Especially, not against attacks such as differencing or reconstruction attacks. This is why we are investing heavily in differential privacy, which really has become the new standard when it comes to data privacy protection.
Ya Xu (19:47): The idea is actually quite simple. What we can learn from the data should be the same with or without any single member's information. So if you imagine that this is the distribution curve we've learned from all these individuals, and now if I remove a member from the data set, the new curve that we can learn should be sufficiently close to the old one. So for those of you who are mathematically inclined, this formal definition is essentially saying there for all [inaudible 00:20:28] X and X prime, the privacy laws will be bonded by Epsilon with high probability.
Ya Xu (20:37): So next I'm going to go through very quickly, just a set of applications that we have leveraged differential privacy or DP. So first of all, at LinkedIn, we have certainly a ton of data, labor markets. We make an effort to share these insights with our members or external communities, such as trending jobs skills, and also how can one transition from one skill to another skill. And we do it in a way that protects privacy. So differential privacy, this is just one application. And another application is for in the space of advertisers. So when an advertiser puts ads on LinkedIn, we want to be transparent with them with regarding how much engagement they're getting on it. But it's also really critical for us to use differential privacy to privatize the data before we share this with our advertisers.
Ya Xu (21:43): We also apply DP on our audience engagement API product that provides insights on LinkedIn's content engagement and audience as well, questions such as what are the top 10 articles that our members like or commented the most and the application can go on and on, hopefully that gives you a sense of how we are using differential privacy at LinkedIn.
Ya Xu (22:08): But I really do want it to mention from technology standpoint, there are more and more research communities that have started to be actively sharing and open sourcing their work in the DP space. So we are [inaudible 00:22:23] at LinkedIn to be able to leverage some of the existing work. However, I do want to mention that one thing we have realized is that this is, relatively in the application space, relatively early. And in order to serve all the applications that I just mentioned, we actually also need to create new algorithms studying and leveraging in addition to the leverage that I mentioned earlier, and also we need to build the new systems that is able to integrate with regarding how we are serving those data externally as well. For example, how can we return a Top-K item, and how do we privatize the streaming data efficiently, and how do we manage privacy budget? For example, all of these are important aspects that we needed to invest in, in order to make differential privacy a reality at LinkedIn.
Ya Xu (23:22): So with that, I'm actually going to conclude my talk today by saying that it's a great pleasure to be able to share some of the early progress we've made with you all in the fairness and privacy space. And we have also just started our effort on transparency. So please reach out to me if you work in this space and we'd love to learn from you, and thank you.