Driving Competitive Edge with Responsible AI Innovation
speaker
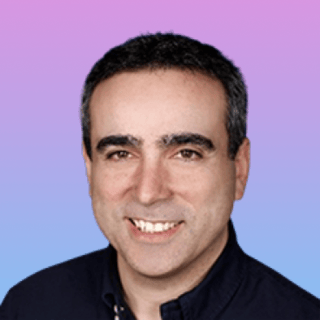
David manages Microsoft AI’s go-to-market, business strategy, and incubation across Enterprise and developer AI products and services. He’s the author of O’Reilly’s book for business leaders “The AI Organization”, and has more than two decades of experience in the technology industry where he began his career as a software engineer. He joined Microsoft more than 15 years ago and has held a variety of engineering and business leadership roles both internationally and in Redmond. AI is moving at breakneck speed. With change sparking more change, you’ve got to anticipate and innovate to stay competitive. Your AI initiatives start with an understanding of a holistic AI strategy. This demo rich session will provide new business perspective on AI with real life AI applications across organizations that make a powerful difference.
SUMMARY
David Carmona, General Manager of AI and Innovation Marketing at Microsoft shares a demo-rich session on Artificial Intelligence (AI) with real-life business applications. He walks through how enterprises can both 'anticipate and innovate' in a fast-paced and challenging business environment, to stay competitive, through AI. Plus, a 4-step framework to building a comprehensive Responsible AI strategy.
TRANSCRIPT
Nika Carlson (00:16): Next up, we're thrilled to welcome David Carmona. David Carmona is the general manager of Artificial Intelligence & Innovation at Microsoft. He manages Microsoft AI's enterprise and developer AI products and services. He's the author of O'Reilly's book for business leaders, The AI organization, and has more than two decades of experience in the technology industry. He joined Microsoft more than 15 years ago and has held a variety of engineering and business leadership roles, both internationally and in Redmond. David, over to you.
David Carmona (00:53): Hi, everybody. Thank you for joining me. When we think about the last 20 to 30 years, software has transformed every industry. It started as a commodity, right? So, something that you need to have to be efficient, but it has ended as something very, very different. It is now a core competitive differentiator. Think of Netflix or Uber, for example. Software has changed the way that everything financial, manufacturing or retail company is doing business. Every company has become a software company, but that's about to change because if software redefined the world, AI is now redefining software. Why? Because AI is an entirely new way of developing software. It can learn with data and experiences instead of being explicitly programmed. It can perceive the world around us and understand it to extract knowledge from that world and reason on top of that knowledge to, for example, make predictions or optimizing outcomes or adapting to external changes.
David Carmona (02:01): Every company in every vertical is becoming an AI company. And many leaders are realizing about that already. I still remember how maybe three or four years ago, the main question that I was getting from customers was something like, "Hey, what is this AI thing that everybody's talking about?" But then we move into a very different conversation. It could be something like, "Okay, I got that this AI thing is big, but how do I get started?" They wanted to kick off pilots and evaluate the impact of AI. So, they are getting ready for it. But lately the question that we get all the time is something like, "Okay. I'm done with the pilot. How do I make AI real?"
David Carmona (02:46): So, many companies were stuck in this pilot purgatory, and they want to move to production and scale AI in their organizations so they can get that business impact today. And the global health and economic crisis has only accelerated this even more. The sense of urgency has increased dramatically. Companies want to connect AI to the business so they can respond and recover from the existing disruption. This sense of urgency is changing how organizations are embracing AI in the short term, but also it is shaping how they're re-imagining their business for the long term.
David Carmona (03:27): Let me start with the short term first. So, companies are already using AI to help them in these challenging times. Let's explore the three main scenarios where we see that's happening. The first one is business process optimization. Companies are looking to streamline business processes and adapt them to the continuous change that they're experiencing now. They want to find efficiencies, reduce costs, and ultimately generate new revenue. For example, in financial services, you are seeing how the processes for areas such as fraud detection or risk analysis, they have to change dramatically. We need them to be more resilient and at the same time, more efficient. It's like the perfect storm. We have more load in the system and an extremely dynamic environment.
David Carmona (04:18): AI can help with both challenges. For example, IndiaLends, a credit platform that is used by 50 banks in India was able to reduce internal processing time for their customers by 50% using AI. Retail is another good example, especially now given the disruption in the demand and the supply. [inaudible 00:04:39] a retailer in Sweden decided to change their planning processes for inventory management and move it from hours to minutes so it could adapt dynamically to the very agile nature. Even [inaudible 00:04:53] that we have in the market today.
David Carmona (04:56): The second area is employee productivity. As employees, we are under a big pressure to deal with the additional complexity brought by the crisis and do it in circumstances that are impacting our productivity. AI can help employees to decrease the time spent on repetitive tasks and instead supervise these automated engines, so they can spend more time on work that's truly relevant for the business. For example, at Reuters, they're using an AI-powered recommendation engine to match videos to articles, so they can free the journalists and they can focus more on writing the best articles. The result is, of course, an increase of their productivity, but they also boosted video views and completion rates.
David Carmona (05:45): AI can go even beyond helping in these repetitive tasks. It can also help us reason and make decisions in real time. A good example for this could be the Team Rubicon, which is a company specialized in disaster response. They manage more than 100,000 volunteers in the US for the COVID crisis. And they're able to capture insights in real time and quickly pivot their resources to maximize their impact.
David Carmona (06:13): The last area I want to highlight is customer service. More than ever, we want to help our customers navigate these difficult times. We're experiencing another perfect storm. We have new needs from customers and at the same time limited resources in companies to address those needs. AI can help us fill that gap. AI for customer service can enable end to end customer management from customer support all the way to lead routing or real time behavior prediction, so they can help us provide a much better proactive customer service in the time that they need it the most. And a good example of this could be Al Futtaim, which is the largest Middle East retail conglomerate. They apply AI to the hundreds of interactions that they have per customer to boost revenue, increase loyalty or identifying trends.
David Carmona (07:08): So, we just saw three scenarios that customers are doing today in the short term, putting AI into action, but what's next? What about the long term? Every time that there's a dramatic impact around us like an economic crisis or a war conflict, things never go back to where they were before. There's a new normal. That's why companies have to move from responding to that extreme situation to then redefine themselves to survive. AI will play a critical role in that transformation. It will move from being used in narrow scenarios like the ones that we were seeing before to be an integral part of our organization. That moment will be the full realization of transforming a company into an AI company. It will require expanding the usage of AI in your organization and connect it better to the business. And it starts with the way we even measure AI.
David Carmona (08:09): Today, we're measuring AI with input metrics like accuracy or performance. To really connect AI with business results, we need to shift to business metrics. For example, cost savings or new revenue streams, customer satisfaction, employee productivity, or any other. That involves bringing the technical departments and the business units together in a combined life cycle, where we measure all the way to the process from design to production.
David Carmona (08:41): We have gone through that transformation before in software and we called it at that time, DevOps. The [inaudible 00:08:48] of DevOps for AI is called MLOps. And MLOps is all about bringing all the roles that are involved in the same life cycle from data scientists, to IT, to business owners. Then in those small iterations, we go through design, development, and deployment, and we measure the business impact continuously, so we can deliver with agility while at the same time staying connected to the business. When you scale this approach throughout the entire company, you can go beyond those narrow use cases and truly transform your business.
David Carmona (09:26): The other aspect of an AI company is to go beyond technical teams and beyond business units to bring AI to every employee. We have done this in the software case in the past as well. And it's the next natural step for AI. We need to empower every business user and every subject matter expert so they can co-reason with AI. And here's an example. I'm super excited with the partnership between Microsoft Research and Novartis, in this case, in the pharmaceutical space. Novartis is using AI to expand their domain knowledge across their entire company. More than 50,000 employees involved in this initiative from research to manufacturing, to distribution. Novartis researchers, for example, are using AI to augment their expertise and their domain knowledge to help accelerate discoveries that can have the potential to become life saving medicines in the future. Let me show a video where Novartis explains this much better.
Shahram Ebadollahi (10:32): Every person contributes something unique in their job. In a pharmaceutical company like Novartis, the important aspect is finding more targets, more molecules that could eventually become a life saving medicine. We generate data at every point of our value chain. In our alliance with Microsoft, we are innovating at the intersection of AI and life sciences, building the elemental blocks of AI, such that every associate can put them together in exciting new ways. This will allow them to reason, innovate, and ultimately, augment their expertise and creativity.
Sabine Thielges (11:14): As a researcher, our core work is to develop new drugs, but the amount of information in the world of medicine is constantly growing. Using AI, we've been able to bring together critical information across data sources. By using our scientific knowledge and co-reasoning on top of AI models, we can accelerate our discovery.
Zdenek Zencak (11:36): We can navigate each other's data. Make all this accessible, exchangeable so that the next colleague can add his piece of work. He will be able to make connections, which impacts the patients, but also us, as researchers.
Shahram Ebadollahi (11:51): Every field, every industry is becoming a data-dependent, data-driven field. AI has a fundamental capability as a complement to the human expertise. If we infuse it at every single step of the workflow, we can empower our people and bring the magic of AI in a meaningful way.
David Carmona (12:16): I think by now you can envision the huge transformation that's coming. AI will impact every facet of the business and every employee in your organization. Of course, transformations of this magnitude come with associated risks and challenges. And this is especially true with AI. There are challenges that enterprises need to address very seriously. The good news is that company leaders are more aware of these challenges that they were in the past. Just a few years ago, the main blockers identified by business leaders for AI were related to the technology or the skills, but now ethical challenges are all in the top of that list of blockers. 80% of business leaders are concerned about the ethical risks and interested in responsible AI.
David Carmona (13:08): Putting responsible AI into actions starts with principles that reflect your intentions, your values, and your goals. These will guide every stage of your software development cycle. These principles are your foundation. It requires that your organization reflects on the challenges of AI, but also that you define the approach that you're going to take to those challenges. In Microsoft, we started that journey early in 2016, and resulted in six core principles that are the foundation for our approach to AI. You can use these principles as inspirations, but I encourage you to go through the same exercise if you haven't done it yet. They are relevant for any industry vertical. For example, with fairness, AI systems should treat everyone fairly, especially when they're involved on any decision making that it has relevant impact on the individual. A typical example of this would be an AI system providing guidance on a loan application. They should make the same recommendation to everyone with similar financial circumstances, regardless of gender, race, or even the zip code where they live.
David Carmona (14:27): Very aligned with these principles, we have transparency. It is critical that AI systems are transparent so that people can understand how those decisions are being made. This is especially true when AI models have any consequential impact on people like financial, health, or access to opportunities. Improving that understanding requires that stakeholders comprehend how and why they function so they can identify potential quality issues, safety and privacy concerns, biases, or even exclusionary practices or unintended outcomes. And above all of these in supports, we believe that people who design and deploy AI systems must be accountable for how their systems operate. We cannot relegate accountability to an algorithm. Humans have to be kept in the loop, both in the design, as well as the operation of the systems. Domain experts working side by side with transparent AI systems are critical for keeping the accountability of any action or any decision made by these systems.
David Carmona (15:41): Once you define your principles, you cannot stop there. To make these principles actionable, we need to evolve them to practices, so we can apply them into our development cycle. In Microsoft, we have created many of these practices already for our own development process. And they're available for you to use in our responsible AI resource center. This includes practices such as human AI guidelines, which was built on our research in this topic or inclusive design guidelines to ensure that AI technology is accessible to everyone or conversational AI guidelines to address how conversational agents should engage with people.
David Carmona (16:26): We also provide tools and technologies to help people creating AI, implement those practices. We consider responsible AI an area of innovation by itself with three main areas; understand, protect, and control. Let me start with understand. Microsoft Research has been focused for years in the development of tools and technologies to understand AI models. And we provide those tools as part of Azure. For example, our work on explainable AI is opening the black box of AI models, so you can make decisions more transparent to everyone or tools like Fairlearn, which is helping identify and fixing bias in your algorithms.
David Carmona (17:12): Second, protect. Microsoft Research is leading the way on advanced techniques for privacy and security. For example, homomorphic encryption, which allows you to run AI on top of encrypted data, so you can keep your users data private or differential privacy, also pioneered by Microsoft Research, which limits the disclosure of public information in datasets. This is about realizing the benefits of AI financial data while keeping the personal data private.
David Carmona (17:46): And third, control. You need to manage the entire AI life cycle, so you can control the responsible development of AI from design to operations. For example, the MLOps solution in Azure allows you to do that. It provides things like repeatable pipelines, trustability, [inaudible 00:18:06], automatic deployment, monitoring, asset management, and many, many others that help you manage the entire life cycle of responsible AI and meet regulatory requirements.
David Carmona (18:19): And finally, it requires a governance system that brings all of this together as a centralized function in your organization. At Microsoft, for example, we use a hub and spoke model for our system of governance, which is balancing accountability with authority. At the center, we created the Office of Responsible AI. The Office of Responsible AI leads the operations of our principles across the entire organization. They work in partnership with the AETHER committee, which is a think tank where people come in with very diverse backgrounds and very diverse disciplines can research, deliberate, and provide guidance on the difficult questions that are raised by AI. These centralized functions work in partnership with the rest of the teams throughout Microsoft. For that to work, every team has a responsible AI champ who is embedded and act as the main point of contact. Let me share a video where the people behind these functions in Microsoft share more about our own approach.
Speaker 6 (19:32): We believe in the potential of AI to improve our lives in big and small ways. We need to make sure it's for the benefit of everyone.
Speaker 7 (19:41): For the first time, we're having machines move into roles that have been the roles of human beings. Might these technologies have inadvertent effects on people in society? Do they align with people's values, their ethics? We needed to think through the implications for our company,
Speaker 6 (19:59): Responsible AI is the approach that we take to developing and deploying our technology. Making sure our principles are brought to life and that it empowers everyone and is inclusive and accessible for people.
Speaker 8 (20:14): Papa.
Speaker 9 (20:15): Excellent.
Speaker 6 (20:15): The job of the Office of Responsible AI is to put our principles into practice by operationalizing ethics across the company.
Speaker 7 (20:23): The AETHER committee is responsible for deliberating about hard new questions.
Speaker 6 (20:27): We are sister organizations.
Speaker 7 (20:30): We have to think through what it means to detect bias, make our systems more fair, to detect errors and blind spots in our technologies. And on thinking through the kinds of advice we give to other organizations and to our leaders where technology can impose on privacy and human rights. Responsibility is at the core. We're learning every day about this new role of responsible computing.
Speaker 6 (20:56): We need to translate academic thought to language that our engineers and sales people are familiar with. And our customers are grappling with many of the same issues. It's incumbent on us to share what we learn.
Speaker 7 (21:11): It's about trying to do better every day, working with our customers and outside agencies to develop processes and deliver responsible computing technologies to the world.
David Carmona (21:25): Our goal in Microsoft is to help you implement your own approach to responsible AI. An example of this would be EY. In EY, they work with one of their clients to improve fairness in their lending process. Developers at EY use Microsoft Fairlearn, the toolkit that we were mentioning before, to assess a lending model. They look at this performance across different demographics, and then they mitigated any unfairness by retraining the model. When EY put Fairlearn to the test, they used real mortgage data, including transactions, payment histories, and many other and unstructured and semi-structured data that they have a meaningful impact in the fairness of loan decisions. Reducing disparity in loan denials and approvals between men and women went from 7% to less than 0.5%. So, how does this work? Let's take a look in more depth with a demo of the tools that they used.
David Carmona (22:28): This is Azure machine learning. It manages the entire development cycle of an AI project. You can see that it can manage things like the datasets that you were using to train your models or the experiments with the actual trainings of those models, or the pipelines to deploy those models, for example, to production or things like a model repository with all the models in your entire organization or the endpoints, et cetera, et cetera. It contains everything in a centralized way for you to manage. And that is perfect for responsible AI because it allows you to manage responsible AI centrally in your organization and as well across all the stages of your development cycle for all your projects.
David Carmona (23:16): To demonstrate this, let me use a loan application scenario with a model that I have created already to assist on approving or rejecting loan applications. So, let me click on that model right here. You can see that I have these tabs over here, explanations and fairness. Let's explore those tabs to learn more about our models. Let me click first on explanations. So, here in explanations, at a glance, I can already explore my dataset. For example, I can see here my prediction of a loan being approved. And let's use it, for example, comparing that with the gender of the applicant. And just at a glance, you can see that the density of approved loans is lower with sex equals zero, which is female, and sex equals one, that's male. You can see that there are more dots here in approved. So, that's definitely a red flag, but it could be that the dataset has that, right? So, there's a correlation in there, but definitely a red flag that I want to go deeper.
David Carmona (24:24): For doing that, let me click here on global importance. This is telling me, what are the features that are more important for the outcome of this model? And this is definitely a red flag. I can see directly here that features like sex or marital status or relationship are very important for the outcome of this model, which is a clear sign of having bias in my algorithm. So, let's go even deeper here. I can click and see the specific examples. Every dot that you see in this chart is an example of an actual input to the model. So, you see, again, that sex is an important feature. If I click on any of this, I can see, again, that for this particular case, the sex of the applicant for getting rejected was one of the most important features to be considered.
David Carmona (25:23): We can even run a "what if" analysis. I can click on any example in my model and simulate what could happen if I change a feature. In this case, for example, I click on this particular example, which is an example of somebody who was provided a loan. And you can see that sex in here is equals one. So, this was a male. So, let's do a "what if" analysis. Let's change this feature in here, and let's say it is female. It's a zero. Now, you can see what just happening here. The dot just moved to the right. So, it has more probabilities of getting rejected. So, again, we definitely have a problem here.
David Carmona (26:06): So, how do we fix this? With explanations, I can identify the bias that I have in my system, but with this fairness option, I can actually mitigate that bias. So, let's go there. Let me select in here "sex" as the feature that I want to mitigate. Next. And then let's pick accuracy as the way for us to measure the performance of my model. So, let me explain what I see here. These are multiple model variations for my loan application process that this tool, the fairness tool, has created for me using unfairness mitigation algorithms.
David Carmona (26:47): On the Y axis, I can see the disparity in my predictions. So, in my case, this is the disparity between males and females in my long recommendation. I can move to a more balanced model in here between males and females. In a way, I'm forcing the model to have less disparity between genders. Now, in the X axis, I can monitor what is the overall impact in the accuracy of the model. And that's what it's showing us. There is a clear trade-off between disparity and accuracy, but if we select a balanced model, we can see just a slight drop in accuracy, but a substantial decrease on disparity between the two classes. Let me show that.
David Carmona (27:32): So, if I click here, this is the unmitigated model. This is my original model. You can see that the disparity here is actually huge. There's a 19% disparity between the two. This is for female, and this is for male. Now, if I go back and I click the model on the far left, now, in that case, you can see that there's no disparity at all. It's just a 0.05%. But in that case, the accuracy didn't drop a lot. It moved from 84, that it was before, to 81.7.
David Carmona (28:10): So, this was a very obvious example. And in real life, this will require many iterations, understanding any bias in your system. And mitigating that bias will also require domain experts included in those iterations. Tools like these which are full integrated in your MLOps are critical to develop AI responsibly. First, because they make the process much more productive. And second, because it can give you the visibility and the control across all your development cycle and across all the projects in your organization.
David Carmona (28:47): Well, I hope that you found this session useful. Let me summarize it with the four steps that I would recommend you to take for developing AI responsibly. First, if you haven't defined your principles yet, do it now. It's the starting point. It's going to be your North Star for anything related to responsible AI. It has to come from the top of your organization, and it has to be communicated internally and externally.
David Carmona (29:15): Second, you can't stop there. You need to bring those principles to life by creating practices across every discipline and every process in your organization. Responsible AI has to be infused into every single activity involved with the development and operations of any AI system.
David Carmona (29:38): Third, establish a governance that is tailored to your organization. You need to centralize the oversight and the guidance within your organization, and make sure that you are truly adhering to your principles.
David Carmona (29:52): And fourth, you should go beyond your company and expand the conversation externally across your industry and throughout society. We all must work together to maximize the potential of AI for positive change.
David Carmona (30:07): If you want more details on each of these steps, I encourage you to visit the online AI Business School, which is our free resource for business leaders, and it's including a full module on responsible AI. Thank you for joining.