Jeff Wilke on Accelerating AI Adoption: From Operations to Customer Experience
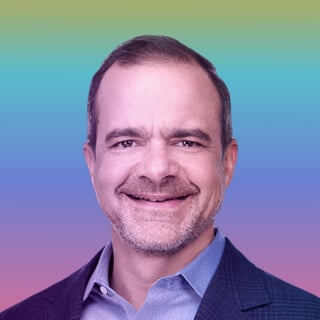
Jeff Wilke retired as Amazon’s CEO Worldwide Consumer in February 2021. During the preceding 21 years, he led Amazon’s retail and third-party stores, operations, marketing, Prime, and technology teams. Jeff led the deployment of techniques from lean manufacturing to create the infrastructure and technology that became Amazon Prime. His teams launched Prime Video, Prime Now, Prime Day, Amazon Fashion, and many other customer-facing innovations. Jeff helped create Amazon’s corporate culture and mechanisms, including Amazon’s Leadership Principles. Jeff is Chairman and co-founder of Re:Build Manufacturing, which aims to integrate new technologies with conventional manufacturing businesses to accelerate growth and performance. Over coming decades, the company expects to create new U.S. factories employing thousands of Americans. Jeff earned an MBA and S.M. from MIT’s Leaders for Global Operations (LGO) program. He also holds a BSE in chemical engineering, summa cum laude, from Princeton. He currently serves on the board of Code.org.
Before Jeff Wilke became Chairman and Co-Founder of Re:Build Manufacturing, he led Amazon’s global retail business, deploying lean manufacturing techniques to create the infrastructure and technology that became Amazon Prime. He now integrates new technologies like AI to help rebuild and transform manufacturing. Jeff joins Scale AI CEO Alexandr Wang in a fireside chat to discuss how AI can accelerate the transformation of enterprises across industries like manufacturing, retail, and others. Jeff joined Amazon in 1999 to increase productivity and excellence. At the time Amazon's revenue was $2 billion a year. By the time he left, Amazon’s revenue had skyrocketed to over $1 billion a day. During his time leading the world's largest retailer, Jeff experienced first-hand the transformational impact of AI, from internal operations to customer experience. But as AI continues to impact and transform every industry, how should enterprises think about prioritizing their AI use-cases between internal process optimizations or customer-facing experiences? What challenges should business leaders be cognizant of?
Alexandr Wang (00:22): I'm excited to welcome our next speaker, Jeff Wilke. Jeff Wilke was formerly the CEO of Amazon's global consumer business, deploying lean manufacturing techniques to create the infrastructure and technology that became Amazon Prime and ultimately fueled Amazon's global consumer business. Currently, as chairman and co-founder of Re:Build Manufacturing, he now integrates new technologies into conventional manufacturing to renew American leadership in the field. Welcome, Jeff, super excited to have you.
Jeff Wilke (00:50): Super excited to be here Alex.
Alexandr Wang (00:53): I always have fun conversations with you. So I'm sure we'll have fun.
Jeff Wilke (00:56): I agree.
Alexandr Wang (00:56): But to start off, you've always been really interested in AI and how that technology is going to affect basically every other technology, and we've spoken at length about the impacts to, everywhere from biotechnology to manufacturing operations, to traditional consumer technology. When did you first realize that AI was an essential technology for the development of enterprises and the development of the global world?
Jeff Wilke (01:28): Well, it's hard to put a specific date on it, and I have been watching it like most people for a long time, going all the way back to watching friends develop interesting applications and lists, for example back in college. But in every kind of application that I was looking at an enterprise, the answers just came too slow from AI based systems. So at Amazon, and in my manufacturing experience before that, we have lots of places where there were really important algorithms that were running real time, but they were using classic applied math, control theory or optimization. And around 2010, I started to see some of the algorithms that were being proposed by clever data scientists and computer scientists, start to be able to compete with the effectiveness and scalability and speed of the algorithms that were already in use. And really quickly after that, they part, if you just did a King of the Hill competition between the existing algorithms and the AI, the AI was starting to win regularly.
Alexandr Wang (02:39): So around 2010 ... I mean, this is the start, I think, the golden age of AI, is when ImageNet as a dataset first came out, and it was the first large scale dataset that allowed for neural networks to become the dominant AI methodology. And so it really was the time at which everything was happening. And to tack on to that, you have a large background in manufacturing and operations, that's where you spend a huge amount of your career. What have you seen that manufacturers or operationally intensive businesses commonly lack when it comes to the application of data, traditional algorithms, and AI?
Jeff Wilke (03:22): Well, first of all, I think of operations as places where you have hubs of activity that transform inputs into more valuable outputs. And those hubs are connected by arcs that typically involve the transport of material. So in physical operations, that's the space, the topology that you tend to see. And most of those operations are capital intensive, they are relatively slow compared to the speed of software, because you have mass being moved around and you have lots of processes that have humans in the loop. And so companies, manufacturers and operations intensive companies, is typically focused on the things that added the most value when you have that kind of portfolio of activities. And those things are really great industrial design of factories or warehouses or distribution centers or ports. They focused on the transportation infrastructure and physical infrastructure that they needed. They focused on things like inventory, they focused on leadership, human leadership for all the people that are involved in this stuff. But they tend to not focus as much on computer science, data, and AI.
Jeff Wilke (04:34): One of the things I do when I meet with CEOs and manufacturing companies is I typically will ask them, who is the senior most computer scientist on your team? And first thing I'll say is, is there a computer scientist, data scientist, AI scientist on the senior leadership team of your public company? And the answer is almost always no, and if there is somebody on there, it's rare that they're actually still writing code or really responsible for the architecture, in the way that if you were building factories, you would have your head of manufacturing on the team, if you were designing products, you would have your head of product on the team. I contend that manufacturers and operators now need to have that same level of skill in computer science sitting at the table. So I think it is the largest gap that I've identified, which is this skill gap at senior levels. And then if you don't have it at the senior levels, it propagates all through the organization. You tend to outsource your strategy about data and computer science, you tend to ... and this is both by the way for process, so how you build the things that you build, or move the things that you move around, and the product itself, because many of these new products, of course, are hybrids of hardware and software.
Jeff Wilke (05:48): And without that, that incredibly talented world class capability to work on these things at all levels of the organization, you're going to lose to the people who have invested in this way. And I just keep seeing this skill gap grow between, typically American manufacturers or Western European manufacturers, and many of their competitors in Asia.
Alexandr Wang (06:17): Yes. Yes. To take a big step back and I'd be remiss to not talk about a lot of your experience at Amazon. When we talk about your experience at Amazon, you really describe as taking what you had learned in manufacturing, in particular, lean manufacturing, and apply it to e-commerce. What lean manufacturing? Why is it important? And why was that so successful?
Jeff Wilke (06:43): Well, okay, so take a step back, lean developed over decades, chiefly in Japan, but elsewhere, and at its core, it's very simple. It's about the reduction of waste, of cycle time, and of variation, along with continuous improvement of processes. Those are the four pillars of lean. It happened to have developed in the auto industry and a few other heavy industries first. When I finished my graduate work in manufacturing at MIT, I went to work at what's now Honeywell, it was AlliedSignal at the time. And I was steeped in lean, and Six Sigma, which you really could call statistical process control, the statistical use of data, and a bunch of other techniques around operational excellence, and we apply them in factories. Then in '99, I get recruited to Amazon and I walk into the first warehouse that I saw, and I looked at it, and I was like, people were using all these words that came from warehousing. And I looked at it, I'm like, this isn't really a warehouse. I mean, it is, but it's actually a factory. It's an assembly plant.
Jeff Wilke (07:46): And so we should be using the language and the techniques, and ultimately, the software that optimizes or can optimize assembly plants, as opposed to traditional batch based operations in a warehouse. The reason that this insight came easily to me is that was the playbook I had. I'm sure if I had spent all my time working on space travel, I probably would have had a different playbook and might have applied a different set of ideas. But in this case, applying techniques from lean, again, principally reducing cycle time, reducing variation, reducing waste, and focusing on continuous improvement, including involvement of all the people in the operation, led to real gains for Amazon and output and quality and the reduction of cycle time. For example, Amazon delivers now within hours, and when I first started, it took 24 more hours just to get the stuff onto a truck. By 2002, we had reduced the cycle time to just a couple of hours. So there was no need to change the process inside the fulfillment centers, from five days to get it, to two days to get it, to one day to get it, to a couple hours to get it, because inside the warehouse was actually a relatively small fraction of the total time. That thinking, reducing cycle time, reducing variation, all came from lean.
Alexandr Wang (09:09): Yes. I mean, you play it off very casually, but I do think that you're like the world expert at large scale application of these methods to result in very high efficiency, operational systems. It didn't just came from lean, you applied it very brilliantly. I think in your time, when you started at Amazon, Amazon was doing maybe 2 billion in sales a year, and then ultimately, I think when you left and around today, it's about a billion a day. So obviously this incredible explosion of scale over roughly a two decade time prize. Obviously, you can scale these systems up just through lean or just through purely operational methods, but how did you use data, AI, software, technology to accomplish this incredible scale up?
Jeff Wilke (10:07): Yes, this is a really good question. It starts with ... we talked about this a little bit before in the pre 2010 era, it really starts with the application of algorithms, of applied math, to try to improve these complex systems that we were building. So again, this topology is hubs where you store inventory, transportation arcs that move from factories, ultimately to homes and businesses, and you're trying to optimize this for time, quality, and cost. So in 2000, you didn't have AI solutions for this, you looked for operations for search, so linear programming, mixed integer linear programming, maybe some NLPs, you looked at the realm of process control, kind of classic process control algorithms, you look at regression for forecasting. And so all of these techniques, these applied math techniques have been around for a long time. And we were applying them as we scaled. In fact, I would argue, those were the reason that we were able to scale from, say 2000 to 2010, was building decisions into software using classic applied math techniques.
Jeff Wilke (11:14): And then, in the process of building these applied math based systems, you're building more and more sensors into what you deploy. So we had sensors at inventory locations, sensors in the hands of every picker, sensors in the packaging, sensors on trucks, all providing data to these, now you'd probably call them dumb, OR based algorithms. And we had actuators in the form of signals we could send to humans, signals to machines that were increasing in number and in flexibility. Now, all of a sudden, is 2010, you have the opportunity to continue to improve these things. By the way, by 2010, these systems were getting so complicated, that with the traditional mathematical techniques, we couldn't solve them in real time with one run.
Jeff Wilke (12:11): So you start to parse these complex decision making systems into smaller and smaller pieces, which actually leads to, in the old way, some suboptimal outcomes, especially on the timeframes of years, which is, what topology should I build, months, which is, how do I plan capacity for a peak event, like a holiday and retail, and minutes, what order should I pick next, what should I put it on, and so on. To me, that was the impetus to look elsewhere. If these algorithms were working perfectly at that point, we probably would have just let them run. But the fact is, they were getting harder and harder and harder to optimize and to build because the data streams from the sensors and the actuators were so large. And the time to crunch the numbers with the traditional techniques took so long, that we were actually starting to grind down, and then over simplification leads to mistakes.
Jeff Wilke (13:09): So that's when I became pretty open to considering A/B testing, King of the Hill testing with AI based algorithms. And over time, we just started swapping the older Applied Math based algorithms for either wholesale for AI based algorithms, or some hybrid combination of the two. And the advantages, because we got scalability, we got faster decision making, all of a sudden, there were systems that always had humans in the loop, humans doing repetitive tasks that didn't really need humans in the loop anymore. And some of these things are on the retail side, like getting our catalog to be as high quality as possible, looking through submissions from third-party merchants who are sending things in, and customer service calls and chats. All of these things are very high bandwidth, they have a lot of data, and the AI algorithms just did better than what we had at taking us to the next level. So we started to close more of these loops, and take humans out of repetitive work, and move human work toward more high value things like helping to train the AI, especially helping to audit the AI as we were implementing it and I like that positive reinforcing flywheel.
Alexandr Wang (14:33): I would hope so, otherwise you wouldn't be here talking with me.
Jeff Wilke (14:38): Well, I wouldn't be here. I'd still be somewhere working on this and I wouldn't have met you.
Alexandr Wang (14:43): Yes, but I think that ... one thing that you mentioned, which is something that I believe in a lot, I think we've talked about this analogy, is that there's one wave that's going across the, I would say, the enterprise world, or going across all businesses, which is just getting lots and lots of digital data. To your point, that was like this critical piece, this critical enabler that enabled all these incredible inventions later on, is that you'd set up all these sensors, and you were just collecting huge amounts of data, and you laid down the foundations from a data perspective to be able actually then apply these very data hungry AI algorithms to actually build incredible technology. And so it comes back to, without the data, you don't have the AI and that was critical enabler in your case.
Jeff Wilke (15:33): Yes, for sure. And I'll bet a lot of the people that are watching this, already have more data streams than they might even imagine, that are available to be optimized, it's just the people working on it haven't gotten to them yet, or don't have the ability to, with the current algorithms, to optimize across the entirety of the data.
Alexandr Wang (15:52): Yes, totally. I think that we're in this super interesting phase of AI, where we're seeing AI impact almost every industry in both back office and customer facing applications, like for example, I think nearly half of Americans surveyed now can think of an example of AI that they use every day. One question I have for you, it's a two-part question is, first, what are the areas in the past where you've seen AI be the most impactful or the most exciting? And then what are the applications in the future that you're most excited about that you think will be most impactful?
Jeff Wilke (16:29): Yes, this is a great question. Look, I think the ... we covered this a little bit already, but I think the applications that are just natural transitions are the ones where you already have algorithms working, but you've seen their limits. They're not working as perfectly, as robustly, as fast as you'd like them to. I mean, sometimes when I'm having this conversation with people who haven't spent much time in this, we talked about a thermostat. A thermostat is in some ways an intelligent machine because it has a setpoint and it can turn off or on, you're heating or cooling in order to satisfy the setpoint. Usually, it's a simple proportional controller, although it might be more complicated. Well, all of a sudden, you add some AI to it, and you get an intelligent thermostat, that's just way more high quality, so the customer experience is way better. And you don't need to have a new furnace, you don't need to have a new thermometer, you just need to have new algorithms and probably a new little box. And so I think starting there, starting in places where if you look across your whole stack, the whole physical operating stack, you can find optimization already happening, it's just a natural place to go to implement AI.
Jeff Wilke (17:52): And then beyond that, I keep thinking in these two general categories, process improvement and product improvement, and really not product design, but actually the product itself. And I think that's a natural, both of those are natural places to keep looking. So in process, where do you have lots of people who are relatively low skilled, who are doing the same thing over and over again. That's a natural place to look and we now have tools, including with natural language recognition and text to speech, we can accomplish things that were really complicated 10 years ago that now humans don't have to keep repeating the same stuff over and over again. And that doesn't mean, by the way I'm an optimist on this, I don't think human work is going to go away. I just think you can move humans from the relatively low skilled things that are repetitive to things that are much more interesting for them, or they can add more value.
Jeff Wilke (18:50): On the product side, of course ... and there's a bunch of examples on our world today, autonomous vehicles and so on, where you get to interact with the AI or the outcome of the AI in really clever, elegant ways. I think about an Amazon as example of the Amazon goes store where the AI focus wasn't on a great product outcome or product design, it was actually on, how do we get cameras only to tell us everything we need to know about running the physical inventory management in a retail store. So when something's been removed, when something's been put back, what the exact location is, and what it was that was replaced. When you get to a place where you have great CV, computer vision that's doing this using a ton of AI, all of a sudden, you can turn it into a customer facing product, which is a store that says you just walk out because you know where all the inventory is, you have the person's payment instrument when they walk in, and the only reason we have checkout in stores is because we don't actually know where the inventory went. When you know where the inventory went, who took it, and what it was, you can have this really elegant customer experience. So the product can change too as the result of the application of AI.
Alexandr Wang (20:06): Yes. So to summarize, definitely a lot of process optimization or process innovation, which is just taking any existing process that currently is very human intensive, error prone, etc., and converting those to be more automated using AI, and then looking from a product creation perspective, what's that set of capabilities that now exist to to the AI and how do you build product experiences on top of that? And I will say, I think Amazon had all these principles around working backwards, or working backwards from the customer experience, and I think that the Amazon Go experience is probably one of the best examples of that, where it's like, they're just walk out experiences, is quite brilliant, and then obviously, you work backwards and you figure out actually a lot that technology is feasible or is within reach.
Alexandr Wang (21:03): So compounding on this question, how do you think ... so most enterprises, most traditional enterprises are, by your definition, operational, very operational intense companies. They have hubs where there's a lot of activity where they're transforming some input to a better output. Many of them involve manufacturing. For a lot of traditional enterprises, how do you think they should be prioritizing AI use cases, in particular, between internal processes ... so different dichotomy, internal process optimizations, back office use cases, versus customer facing iteration? There's elements of both, where there's repetitive processes that are customer facing or internal, or there's new things that you can build that are internal versus external. How do you think about that?
Jeff Wilke (21:55): Well, the first thing I would do if this were a review, Alex, I'm going to imagine that you are a product owner at Amazon and you came to me with this trade off. First thing I would say to you is, why is it trade off? Why do we have to prioritize between internal and customer facing? Why can't we do both? And the answers can be interesting. So one answer might be, well, our architecture is too complicated. We have lots of coupling, and so we can't work on both of them simultaneously. And then I would say, well, you have some architecture work to do to really servicify, use the cloud, get things set up so that you're able to work on both simultaneously. Another answer might be, we don't have enough computer scientists, data scientists who know how, whatever to work on both. And if I accepted that, then I would say, okay, well, let's figure out what's the most important place to start? And where can we add the most value? Where's that value most differentiated? There are certain things of course that you're going to do that you do repetitively, that could be replaced with off the shelf capability or AI that's lots of other people are using.
Jeff Wilke (23:03): I mean, most people don't write their own ERP for a reason, the ERP that's out there works pretty well and people complain about it sometimes, but truth is, a lot of enterprises depend critically on software that they didn't write. And so that's fine, you can identify places to use off the shelf stuff or leave it the way it is. But for these areas that are incredibly important for the customer experience strategically valuable, where you know that making a difference there will make the difference for the trajectory of the company, then I'd argue you got to figure out how to get the resources together to have an impact there. And that to me is a combination both of hiring internally, so data scientists, computer scientists, people that really know AI, and making sure that at least one of them sits at the leadership team level, the enterprise leadership team level.
Jeff Wilke (23:57): And two is finding partners who can help increase the speed with which you deploy these tools, and that's one of the things, as I've gotten to know scale and your work Alex, it's super impressive to see the speed that you can help push into companies that are either dipping a toe in the water or already have a lot of this work, but need to do it even faster. And there's no real loss for somebody in partnering that way. I mean, getting to a usable and value adding AI system as fast as you can once you've identified the need seems pretty critical to me, and if you can find a partner who works with you to do that, You haven't lost anything, as long as you have your own people to catch it once the past has been thrown. I always hesitate these things to use American football metaphors, but I'll just say once the pass of any ball, whether it's a football in soccer or a football in American football, but you do have to have somebody to catch it. Somebody who knows how to catch it, and push back and design and audit, and I think a lot more of our operating enterprises need to upgrade those skills.
Alexandr Wang (25:07): Yes, totally. Some of the use cases, and obviously, you'll appreciate this super well, since this is where you lived and breathed for many years. But some of the use cases of AI that I think lots of enterprises are thinking about, or lots of industries are thinking about, is in areas like predictive analytics, for forecasting or for better forecasting, just a very deep topic, is how do you make sure you have all the right things at the right time as you operate your business? I think that there's some incredible case studies from Amazon's work of a very successful implementation of this. Whereas, there's a lot of business today where there's still a lot of very manual human loop forecasting activities that happen. Most business, I think, still operate that way. Maybe share a little bit about your experience at Amazon, actually pulling off that transition, what it entailed, what involved or what were some of the snags along the way and how it ultimately ended up working out.
Jeff Wilke (26:07): Yes, this is a great point and forecasting is around that and certainly is right for AI. And it's the same pattern that we talked about before where typically people are forecasting. If you're running an enterprise, somebody somewhere is doing forecasting, and it might be on an Excel spreadsheet, it might be with a simple system, it might be using some kind of regression analysis, but people are forecasting. The interesting thing I found when I first got to Amazon was just following the trail of the forecasts. And it turned out that the machines were spitting out forecasts. The humans didn't trust the forecasts. So they were basically building their own forecasts or ignoring the machines and doing it themselves. And over time, we got the machine generated forecasts to be demonstrably better than the human generated forecasts. And so it's easy for the humans to then adopt the machine generated forecast and begin to focus on the inputs to it, making sure those inputs are good. One of the things we always would say at Amazon is uninspected data is always wrong. So if you have forecast models that are being driven even automatically by uninspected data, you can bet over time, those forecast models are going to drift from their intended state because the data streams get polluted in some way or there are defects in the internal systems in some way.
Jeff Wilke (27:28): So we would move toward more and more machine driven forecasts. And then again, it's that post 2010 thing where all of a sudden, people are proposing really clever data scientists, and in our case, some economists, we built a very large team of economists that were very mathematically oriented and would see some of these things and propose solutions based on parallels they'd seen in other industries. Would propose an algorithm that they wanted to compete with the current best algorithm for prediction. And so as we did in all these cases, we would run them side by side ... by the way, in architectural thing for the computer scientists on the go, we're really focused on separating stateless computation from workflow, so that, and what I mean by that is that you want your workflow software to be able to run anything that you give it, whether it's production data, or test data, and you want your computation engine to be stateless, so that you can have the same computation engine run both simulations and production.
Jeff Wilke (28:39): And if you create that kind of an architecture, then you can have somebody propose a completely new forecast system and you can run it through the exact same workflow that you run the current algorithm through, and you can see if it's better. And if it is, then that becomes the default and you now start making all your decisions without one, if not, you keep working at it. So I agree with you, forecasting ... all kinds of decisions too, so forecasting demand, basically anything that has variation, any stochastic process that is susceptible to improve forecasting for sure.
Alexandr Wang (29:17): Yes. One thing just, again, given the massive scale that Amazon operated, I think most people have lessons to learn from that. But as you actually deployed and scaled AI technology within Amazon, both for these process improvements, like forecasting, or also for pretty innovative applications like Amazon Go or the Echo/Alexa or in the use of building a better consumer website with better search and other technologies. What were the challenges in implementing some of the AI technology and what are the challenges that other enterprises or other companies or other leaders should be aware of?
Jeff Wilke (29:58): Look, I think the biggest challenge is that the humans who are in the loop with their hands on the wheel are going to push back. They just are. They're going to say, we can do it better than this AI decision making thing. And there's going to be a lot of internal friction. I saw that in operations, I saw it in our retail organization, I've seen it in other companies. And I think the solution is to pick the highest leverage places where you know you can demonstrate that you have better decisions with AI than with the humans. And then you've got to enroll the humans in letting go, taking their hands off the wheel, and showing that it's not going to lead to everybody losing their job, you transition the humans to be monitoring the AI to be helping to train it, and then some of them will go on to other things, because they'll be interested in other things. It's just really hard. People believe that the models they've built in their minds and on spreadsheets are better than the models that you run with AI, and there are lots of areas where that's just not true anymore.
Alexandr Wang (31:24): Yes. Yes. And so it took ... I mean, for you and Amazon, it took the strength of leadership to be willing to first just build out these methods, invest in them and test them versus the other approaches, or test them versus the human approaches, and then to really be data driven about it. And once you got these autonomous systems to the point where they were outperforming the human predictions or the human judgments, really having that data be very clear, and using that to make the decision.
Jeff Wilke (31:57): Yes, I think you have to show people. I mean, one way to do it is just to say, your hands are no longer at it, you can take the steering wheel away. But that's not necessarily the best leadership behavior. I think part of what you do is educate people on what you're building so there's transparency. And this is true. We've had this conversation a little bit about AI generally, which is the machines that we build have goals and it's really important that humans know what those goals are and audit them. It's unlikely you're going to want your enterprise to be controlled by a totally black box. I think you're going to want to know what the goals are, and be able to have humans audit those to make sure that you're actually achieving them. And so you can enroll the people in, where you're headed, and what their roles are going to be, and then show them with data that it's just better, it just produces a better result. And I did find that, although people were reluctant at first, when they realized how much better the customer experience was and how much more productive the company could be, and how much more fun their jobs were when they weren't repeating the same work over and over again, they came on board pretty quick.
Alexandr Wang (33:04): It's a great segue to this next question, which is, whenever there are large technology advances, whether it was the printing press in the past, or the ATM machines, or whatever it is, there's always ... and now we're seeing it with AI in the most pronounced form, it's always accompanied by the fear of wide scale job losses, there's always the sort of it's happened in past, happening today, for sure. And in operations, manufacturing, and a lot of these human loop processes, there's a huge concern, and there's a huge claim that the application of AI will result in overtime to end in humans in these jobs, and ultimately result in millions of job losses. A question for you, because you touched on this before, how do you think about this? Does the introduction of AI actually threaten the jobs, or does it allow for us to just create significant more efficient systems where people are used in higher leverage more interesting ways?
Jeff Wilke (34:04): Yes, I think ... to me, it's the ladder. There's some great writing on this in the last few years. I'm a big fan of Erik Brynjolfsson and Andrew McAfee's The Second Machine Age, which I think does a really good job of posing this question and answering it well, of course, we're learning new things every year. But when I look back at the last 120 years of industrial history, there have been lots of technological advances that, as you suggested earlier, people were sure we're going to eliminate human work. And what they did is all those advancements, they changed human work. They certainly eliminated some jobs, they changed others. But typically it was, even within a job, some tasks remained and some tasks got automated. So the work of humans actually increased over time. It just went to higher value added skills and work.
Jeff Wilke (35:00): I think that I don't see any reason why AI isn't yet another example of a technology that will free up humans from drudgery and unsafe conditions, for example, driving, move all of us toward more joyful work, because it's interesting, safer work, and more highly value added work, because there's so many things that still, I think you'd agree with me on this, Alex, there's so many things that our brains still do better than machines, and probably will for some for the foreseeable future. That's why we want humans. We want humans to create the future, not to repeat tasks that we've already solved.
Alexandr Wang (35:44): Yes. Yes. One thing that I ... this is just always a fun topic, and we've talked about it a little bit, is if you were to imagine, like transport yourself back in time to when you joined Amazon, and you imagine like a state of the operational system at that point. And then you were just to imagine a time machine, you're just dropped in to the world today and you saw how everything operated and how smooth it all was, and how all the processes looked like. How much of that ... could you foresee versus how much like you would have been impossible to imagine and foresee at the time you joined? So the question is, like, how ... I think this always goes back to, how predictable is the evolution of technology in practice? I'm really curious to hear your thoughts on this. I've actually never asked you this before.
Jeff Wilke (36:35): Yes, this is interesting. I think my answer depends on the realm. So in the realm of algorithms, it was predictable for me, in part, because I had that background, I had some OR background, and it just was super frustrating to be able to characterize a problem with math, so either in the time domain or in state space, and then have no ability to actually solve the problem with computation that was available in any kind of timeframe that made sense. So you're trying to control some complicated thing, and you need answers in seconds or milliseconds, and your batch job takes hours to run. That's not helpful. But I can imagine a day because of increased computing power and the advancement of algorithms where we would just be able to do those things, much more complicated things very fast.
Jeff Wilke (37:28): So for me in that realm, I probably would have said, yes, Amazon's going to be doing a lot more things with machine decisions over the course of time. I probably wouldn't have imagined what I've imagined GANs are exactly the way Bayesian networks ended up working out, or random [inaudible 00:37:45] were around at that time. So I might have looked there and say, hey, lots of stuff is going to behave this way. But if you look at some of the technology that's evolved, that humans interact with daily, like my mobile device, I think, in '99, I probably would have been in the camp of being skeptical about how powerful the devices would be in terms of how humans were using them. So I probably wasn't envisioning the kind of breadth of mobile apps and all the things that we would be doing with location services, and all the sensors that would be on this thing that would make it super valuable computation locally, you're kind of at the edge. So that took some adjustment for me, certainly. And then in robotics, if I think about the automation of physical tasks, I think that I'm actually surprised at how long it has taken for us to do grasping tasks really well.
Alexandr Wang (39:00): Super hard problem. Incredibly hard.
Jeff Wilke (39:03): Say that again.
Alexandr Wang (39:04): Incredibly hard problem. [crosstalk 00:39:06] Materials are very tough.
Jeff Wilke (39:08): Yes, it really is. And for so many different ... observing it and then controlling it, because I was so steeped in it at the time, I would have said, 22 years on, are we going to be able to have a robot that can go to a shelf of a bunch of different heterogeneous composition stuff and pick out the thing that's supposed to at the same rate as humans? I would have said, yes, and that hasn't happened.
Alexandr Wang (39:34): To close off, I have a few questions to just ask and just thinking big picture about AI. I think that recent 2021 survey of large enterprises found that the biggest problem most companies face with AI is just a lack of skilled people, to your analogy, people who can catch the ball, or, I guess pass the ball via kick or whatnot. What do you see as the most frequent blockers of AI adoption, both among companies that ... you talked to a lot of leading CEOs and lots of innovative enterprises, as well as in your experience at Amazon, where, obviously, you were trying to apply AI in probably 1,000 different ways or maybe even 10,000 different ways within your organization.
Jeff Wilke (40:23): Well, again, you sort of summed it up at the beginning of the question, which was, I do believe that there are lots of companies that are poised to make good use of AI that don't have the technical expertise in house. There's a hiring gap that they've allowed to happen over at least the last decade, that they have to close. I mean, it's just an imperative for the strength of those companies that they hire computer scientists, data scientists, and people that understand AI, so that they can build the solutions into process and product. We're allowed over time, our skill in this area in manufacturing operations to wane, and I'll extend it to really large enterprises. I mean, if I think about challenges, like, why does it take two days to settle money in the financial industry? And you can kind of go industry by industry and say, we've allowed old status quo, old architectures to determine the customer experience, speed and value that we create. And it doesn't have to be that way. But we're not going to be able to create that value by moderately informed people looking at PowerPoint slides, and using their human intuition to make judgments.
Jeff Wilke (41:50): We're actually going to need to have people sitting at the table, who had deep expertise in this capability. And it might start, the very first thing might be starting with a conversation with someone like you, Alex, I mean, somebody who does have that expertise, who is outside the company, who can come in and say, here's what I would do if I were in your shoes. But I know, and I know you'll advise them too, I know what they're going to need to do is to increase the technical talent to decide what they need to build internally versus using outside suppliers, including cloud suppliers, and support for labeling and AI software that they want to build. But having that right strategic mix, starts with the right skill set, it's time to go.
Alexandr Wang (42:35): Yes, and then maybe just to close off here, if you were to share one call to action, and this can be broader than just the application of AI and these machine learning based systems, but one call to action to companies that are operations, have your manufacturing heavy, just based on everything that you've seen. What would that be? What would be the thing like, hey, if you do one thing today, or one thing over the next month, what would it be?
Jeff Wilke (43:04): It would be love your technologists. I mean, it would be, don't just assume they're working on interesting important stuff and they'll get it done and they'll translate it to you at the highest level. Dig in. Educate yourself. Understand as much as you can the technology that your product and process depends critically on, and then surround yourself with the people that can make it even better.
Alexandr Wang (43:34): Awesome. Thank you so much, Jeff. This is a lot of fun, a lot of super interesting insights and very unique experiences obviously that you've had, and I think we're both very optimist and excited about how we can apply AI to make the world better.
Jeff Wilke (43:48): For sure. Alex, thanks for welcoming me and it's a pleasure to interact this way, as opposed to in person with so many amazing people.
Alexandr Wang (43:57): Awesome.