How to Use Privacy to Prevent Adverse Customer Outcomes
speaker
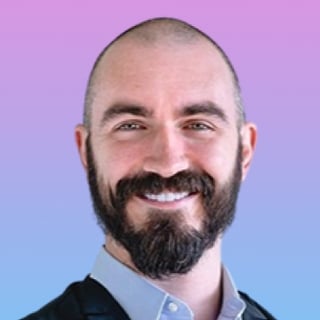
Dr. Chris Hazard is co-founder and CTO of Diveplane. Diveplane’s understandable and privacy enhancing AI spun out of Hazardous Software, a company Chris founded in 2007 that focuses on decision support, visualization, and simulation for hard strategy problems in large organizations, DoD, and government. Chris holds a PhD in computer science from NC State, with focus on artificial intelligence for trust and reputation. He was a software architect of CDMA infrastructure at Motorola, worked on robot coordination and logistics at Kiva Systems (now Amazon Robotics), and advised NATO on cyber security policies. He has led simulation, serious gaming, and software projects related to cyber security, social engineering, logistics, economics, and psychology, and is a certified hypnotist. Dr. Hazard is also known for his 2011 game Achron, which won GameSpot’s Best Original Game Mechanic award, and for his research on AI, privacy, game design, and human-computer interaction, for which he has given keynote speeches at major conferences and been featured in mainstream media.
SUMMARY
Chris Hazard, CTO and co-founder at Diveplane Cooperation, discusses some of the different techniques that can be used to protect privacy including encryption, differential privacy and privacy budgets. He explores how enterprises should adopt a thoughtful privacy strategy that balances the risks and rewards of these privacy-enhancing technologies. Join this session to learn how you can develop a privacy strategy that considers the business value, liability and user privacy to unlock key use-cases.
TRANSCRIPT
Nika Carlson (00:00): (music).
Nika Carlson (00:15): Next up, we're excited to welcome Chris Hazard. Dr. Chris Hazard is co-founder and CTO of Diveplane. Diveplane's understandable and privacy enhancing AI. He has worked on robot coordination and logistics at Kiva Systems, now Amazon Robotics, and advised NATO on cybersecurity policies. He has led simulation, gaming, and software projects in cybersecurity, social engineering, logistics, economics, and psychology. Chris holds a PhD in computer science from NC State, with focus on AI for trust and reputation. Chris, over to you.
Chris Hazard (00:57): Welcome to TransformX. And today I'd like to talk to you about an issue that affects virtually everyone here, which is privacy. And in particular, I'm going to talk about how it can affect your customers and their, outcomes and the longevity of their business and the successfulness of their business.
Chris Hazard (01:12): To begin with, we're all probably familiar with the complex privacy landscape. It seems like every month or two there's a new privacy standard being rolled out in a country, or a new governance method, or a new standard or technique. And across all the different countries in the world, there's a hodgepodge mishmash of different types of laws that people need to make sure that they abide by when they're dealing with and handling customer data. Now, we're all familiar with this, regardless of whether we're using the data in an actionable way for our business, or whether we're labeling data for others, or using services to label data. However we're transferring or handling or sharing our data with our partners, all of these things matter.
Chris Hazard (01:51): But a lot of folks think of this as just a cost, a regulatory requirement, and once they check the box, that's fine. So let's look at a few places where it's not necessarily fine. Back when COVID first started to come around and affect university campuses last fall, universities were offering free COVID tests for students. They could come in and get the test. But the students knew that if they tested positive the university might perform some action and limit their ability to participate in some activities. They might them to quarantine or be off campus for a while. And so students would pay money to get these tests done off campus on their own dime, because they thought that they knew how to handle it, and they would want to not share that information with the universities, because there was a break in trust. There was a break in the incentives between the university and the student, even though fundamentally the incentives were aligned. They both wanted a good outcome.
Chris Hazard (02:48): We can also look at how different types of collection of data and its effects in AI and machine learning, and even just the feedback loop between the consumer, the customer, the business, and how that affects their behavior over time. Progressive insurance has been doing very well the past couple of years, past several years, partly due to a program called Snapshot. Now, for those of you who are less familiar with it, Snapshot is a USB device you put in your vehicle, and it can track how you drive. Well, what it allows Progressive to do is monitor who's driving well, and help the customers that drive well save better in insurance. This sounds like a win-win universally. However, because there's not necessarily a gate between the privacy, there's a little bit of a confluence of incentives here.
Chris Hazard (03:37): You get folks who are saying, "I've been driving around in suburbs, and all of a sudden a child ran into the street, and I didn't want to hard break because that might raise my insurance premium." So they'll swerve. Or they'll figure out what are the things that I'm being measured on, and work around that. People talking about gunning the throttle or the gas pedal when they reach yellow lights, because they don't want to register that hard break. Or driving further and further, incurring extra emissions, extra gas, wear and tear on their vehicles, just to work around these. So privacy is not just about making sure we meet the laws, it's about making sure that when we're sharing the data, when we're using it for AI and machine learning labeling, that we're using it in ways that really lead us to good business paths, and don't bring in customers that we don't want. We don't want the adverse customers. We want to actually provide a good product and service to our customers throughout.
Chris Hazard (04:32): Now, you might think, "Okay, we're just collecting data for good purposes." Let me take a couple interesting cases of how data can be used very nefariously, even if you are doing the right thing at the moment that you're using and collecting it. Let's say that you're a small game company or some game company that's collecting data on your users. Maybe you're just collecting how well they performed on a particular level, the time that they played, maybe the location that they played in, maybe just their IP address or some basic information to make your game better. Or your app, for that matter. Well, it turns out that there's a lot of behaviors, both with how you move, the decisions you make, that can give information about whether or not you are dieting, about whether or not you're depressed, because of how you value events within a game or within the application.
Chris Hazard (05:22): And sure, it's not perfectly accurate, but now this seemingly benign data from applications or from games could lead to being health data, or yielding information about the psychographics of the person behind it, or even identifying that person. And even if you're collecting it for good purposes, suppose that at some point you share it with a third party, or your company gets acquired, or you sell that piece of IP. That providence can come back to haunt you in many different ways. And there're many ways that, sure, we don't handle ... Maybe we're protecting the user's names or their IP address or the obvious personally identifiable information, the different types of data that are recognized in countries as being problematic. But, even within the data, there are ways of re-identifying it. All you need are four spatio-temporal points, the lat long location and the time of the event, in order to uniquely identify the vast majority of people in the world.
Chris Hazard (06:24): Or pick several other features, and these can uniquely identify folks. Who went into the emergency room on this date, this emergency room, at 2:00 AM? There might only have been one person in some small hospital, and that might uniquely identify them. So one of the things we need to think about when sharing our data or when we want to prepare it for a more widespread use, is how we protect against these sort of events and how we future proof it to protect our customers for the future.
Chris Hazard (06:53): So looking across the privacy and the incentive landscape, there's several different ways of approaching this. And there's a value in doing all of these in different ways. First, I'll talk about encryption briefly. I'll talk about the idea of differential privacy and how powerful that is, and expand in to the privacy budget. And those two are tightly interleaved and are one piece together, but I'll break them apart into two separate pieces. Then I'll talk about some different anonymity attacks and attacks on privacy, and synthetic data and how synthetic data can help alleviate some of those problems as well.
Chris Hazard (07:27): First up, encryption. We're all used to securing our data, putting it in nice and secure places, but what can we do with it if it's encrypted? Well, there's a really neat and powerful technology called homomorphic encryption. And what this allows us to do is perform compute when we don't necessarily trust the compute. Now this is very powerful and useful. It solves the problem of not trusting compute, but it doesn't solve the problem of being able to share insights with a wider audience without sharing the data itself. So it solves a piece of that problem, but a little different problem with regard to general privacy and information sharing.
Chris Hazard (08:04): There's also something known as order preserving symmetric encryption, which allows you to transform the data set into a slightly different data set, where the individual features are transformed in a way that also can preserve privacy via the encrypted values. But one of the concerns here is that it's only generally built for a specific purpose, and can limit some of your machine learning and applications that you might want with the data. So I bring up encryption because it is a very important piece of the ecosystem, but it's not the only piece, and solves mostly the problem of untrusted compute.
Chris Hazard (08:39): So differential privacy is a term that many of you have probably heard of. So what is it? It's the idea that we can say, if I give someone an access to a statistical database, which means that I don't know for sure whether or not this person is in there. I can just get statistics out of it, not a regular relational database lookup. If someone is in the database, there's a certain probability that I have about whether they're actually in the database or not. And typically we express this in a relation of multiplying it by E, Euler's number, to an exponent. Which just gives us kind of a nice, easy number to work with instead of numbers with a lot of zeros. And it has some other neat properties we'll talk about it a minute.
Chris Hazard (09:23): One of the most celebrated and effective techniques is to use something known as the Laplace mechanism. Now, this is a technique of adding noise to your data, to the different features, in that sort of a steep long tail distribution, a double exponential distribution. And when you do that, you can prove in many settings that you can achieve this type of differential privacy with your data.
Chris Hazard (09:49): Now, the concern around differential privacy is with auxiliary information. So we've added this noise, and now we know we can't uniquely identify someone. But what if someone has some additional information from outside of the data? Dwork, Cynthia Dwork, one of the founding people who built the core ideas of differential privacy, gives an example of Terry Gross. So Terry Gross is two inches shorter than the average Lithuanian woman. If I know nothing about Lithuanian women's heights, then I know nothing about Terry Gross's heights, other than this relationship. But if I happen to have done a little bit of research, maybe I read something, maybe I just sampled myself. I gathered 20 or 30 Lithuanian women, measured their heights, and now I have that information. I can use that additional information to infer what Terry Gross's height is. So differential privacy is meant to make sure that, even if I had that information, I still wouldn't have enough information to deduce Terry Gross's height in this case.
Chris Hazard (10:49): So what does this look like for real data? So here's a nice little sample dataset. It's easy to understand. Alice, Bob, Carol, the typical folks from cryptography. And we'll say that each of them has a secret. And just for understandability, we'll say that the secret is the numeration of the first letter of their first name. They're in a house number, so that Alice and Bob are in house one. Carol and Dave in house two, et cetera. And they each have a vehicle color which alternates. So we can see it very easily. Now, if we add Laplacian noise to this data, we get the chart on the right. And as you can see, the numbers are perturbed by quite a bit. Now this allows us to do a certain type of query on the data, as I mentioned before.
Chris Hazard (11:36): Now, if we look at what the data looks like with the Laplacian noise added. So the original data is nice and even. I can see the secrets, and I can pick out a given secret. The signal's very clear. And on the right, when we apply the noise, we can see it's perturbed. I don't really know if it matches or not. I have some uncertainty around that, which is good because we want to protect privacy, we want to align our incentives. But if I'm able to query the data too many times, or pull too much from it, or introspect it too much, I can smoothen that data and basically identify the underlying pieces of information behind it.
Chris Hazard (12:13): So now what we've done is we've built ourselves a great security gate that you could drive through or just drive right around. So how do we solve that? Well, the idea of differential privacy also comes with the privacy budget. That means that when you're performing these queries on the data, you're limited with how many queries you can perform. And traditionally, as I mentioned before, we'll deal with this epsilon, taking the logarithm of the ratio of probabilities, and making sure that our total budget, the number of queries we do, the epsilon adds up to no more than a certain budget. We can also relate this to the mathematics of information theory in a number of very powerful and unique ways, which can help us characterize the uncertainty around the data.
Chris Hazard (12:54): Now, if we do this, these types of queries, with an epsilon of eight here, we could perform any one of these sets of queries on the right, but not all of them. Only one of them. So Alice's secret. We could say what is Alice's secret? 0.59. That's the answer we happen to have gotten. But now we cannot query the data anymore. It has provided enough uncertainty, enough plausible deniability, that Alice's number might be different than that. But we can't use it anymore.
Chris Hazard (13:22): We could perform four queries. We could find Alice's secret, Bob's secrets, the number of secrets greater than 10, and the average secret for house 1,005. But by doing so we have to use an epsilon which is smaller, such that if we use an epsilon of two, we spend two on each of these queries. And as you can see, we've added noise to each end. And also notice that Alice's secret is negative. That's because, in order to apply Laplacian noise properly, we have to allow it to go past the bounds, unless we perform some other more complicated mathematics to add privacy that would be eroded by adding the clamps at the end.
Chris Hazard (14:00): Another technique is to apply local differential privacy, and this is putting the privacy and the noise making, or the noise adding, on the device itself, or on the user's local compute environment, whether it's in the browser, on the device, or on the IoT device, to make sure that it randomly gives us a response back to the server. So that the server, the consumer of the data, really doesn't ever have the data. Well, the way you apply that, a real simple way of thinking about it is, imagine flipping a coin or rolling a die. And if it turns out with some number, you give the real answer. If the die roll or the coin flip is a different value, then you give a made up answer, a random one. And so you can see that if an attacker is looking at this data for an individual, there's too much noise there to really be able to pull out what's going on, but an aggregate. Someone could determine and ascertain the trends that are going on.
Chris Hazard (14:57): And some local differential privacy techniques can be applied even if the data is a database. So just because you don't apply it to the user's device, you can still apply it within one part of your organization to make sure that the trust is maintained as you move the data from one part to another.
Chris Hazard (15:13): Now, let's consider a case that we have an outlier. So let's say Judy in our database has a value of 1000. Everyone else has a secret, a number between one and 10. And hers is very high. Well, first up, if we use an epsilon of four, if we find what is the lowest value, that's our single query that we apply here, we get negative 321. Now, that's not very usable from any analytics perspective. It's private. We made sure that we maintained privacy. But it falls so far out of bound it doesn't really tell us much. And if we say, "What is the value at the house 1,002?" That's pretty far off too. We've added too much noise.
Chris Hazard (15:55): So if we use a random response for this, we we'll use the local differential privacy, and we're going to pull a different number from each of those, randomize it up. But if we happen to know that Judy has the highest value, maybe that's our auxiliary information, we just happen to know that, well now we can make a single query or maybe a subset of queries and determine what Judy's value is by applying that information.
Chris Hazard (16:19): So now we've taken a very secure mathematically sound system, but we've given the attacker a way to climb right over it, and leap frog into extracting the information that they want. So there's many challenges to differential privacy in practice. You can compose it with other data. You can apply post processing. You can correlate data. It's very strong to all of these, but it's not immune. And some of these correlations can leak privacy, especially with regard to time series or graph data. If you have many transactions from one particular individual, and only a few from another, if you just apply differential privacy you may be leaking more data about the first user, and you may be overprotecting the other one and misapplying your privacy budget.
Chris Hazard (17:05): Some data sets, for example if you have a surname, in some parts of Europe and some other parts of the world, there are surnames that are fairly unique. And if you leak the fact that this surname does business with your company, you've basically identified the family that is doing business, and leaked privacy. And there's also other signals hidden within the data that might be correlations that could, again, sort of erode the privacy a little bit.
Chris Hazard (17:31): What's an appropriate epsilon value? There's a lot of about that. And many companies have applied different values, sometimes to applause or lack thereof. And how do you deal with sensitivity and scale? If one currency is 1000 times larger than another, as we showed in the previous example, with one value being basically 100 times larger than the next highest value, it can really offset all the data. So you have to apply smoothening techniques. How do you decide utility versus privacy? If you're administering drugs that if you over administer could kill a person, but if you get it just under or just right you might save that person's life, your loss function might be very sensitive. So how do you make sure that you're adding noise in the right way?
Chris Hazard (18:14): And further, with differential privacy, if you're performing all of these queries, you need to throw away your database or your data set once it's exhausted, once you've applied all of that privacy to it, or you've exhausted your privacy budget. You can't decide after the fact, "We should have queried this," because if you did you would've been yourself a feedback loop. It's sort of like back testing the wrong way. If you try a bunch of models and you find out what works, "Oh, this works. Let's now rerun it through," you've actually short circuited that and eroded your privacy.
Chris Hazard (18:47): There's also many other attacks on differential privacy, such as timing attacks. If I can determine how long it takes to [inaudible 00:18:55] return, I might determine whether that data was in the data set or not even if the noise is added. I can use techniques based on floating point values and how noise is added. I can perform different cyber attacks on a distributed differential privacy system, distributed differentially private database, and you basically increase your attack surface. So there's a lot of different attacks on here. And regardless of what single type of privacy measure you use, if you only use one, there're attacks against it. None of them are perfect by themself. So you have to think about privacy as a whole, and privacy versus utility.
Chris Hazard (19:31): Now let's get a little more private with our data set. And now we're not just going to privatize or anonymize the secret, we're also going to add privacy to the identifiers that lead us to that, like all of the different identifiers. So what happens when we add that type of Laplacian noise or privacy or local differential privacy to all the features? Well, what happens is, in our original data there's a nice perfect correlation, again, in our toy data set, between the secret and the house number. That's great. That's the insight we'd like to share. We'd like to maintain some privacy to make sure that individuals have the right behavior that we would like to encourage from our customers. But if we add the privacy also to their identifiers, now we've entirely lost that correlation. The analytics here are pretty meaningless.
Chris Hazard (20:24): What we want to get is we want to have distributions that have the same analytic outcome. If I want to express the idea there's a perfect linear relationship between these points, I can express that same idea by putting data points just at the end points here. I don't need to put every point on the way. Now yes, that will give different density, different analytic artifacts. So how can we address those? Well, as you get to higher and higher dimensions, you have basically more freedom. It becomes easier to create data that maintains the same distribution, but doesn't affect privacy. Basically the whole data set, when you get beyond six or seven dimensions or features, the data set is basically holes for most real world data sets. And if you apply joins, you apply it to a relational database, it's mostly sparse. So it becomes easier and easier to get good accuracy, or real close to the accuracy of the original data, oftentimes less than 1% difference between the original and synthetic data, without leaking privacy.
Chris Hazard (21:28): Now I mentioned synthetic data. The idea here is we're going to try to obtain new samples from the same underlying distribution without giving the original data. There's many techniques to apply, to create synthetic data, everything from Baeysian Networks to GANS, Variational Autoencoders, Diveplane GeminAI, one of the products that I work on. And they all have many different pluses and minuses. And I'll talk about that in a little bit. One of the issues that some of these techniques have is you can apply noise twice. So let's say that you're using a GAN, and you're using differential privacy. So your neural network is looking at the data. And you say, "Neural network, you have this much privacy budget for your training." And so every time the neural network is looking at a subset of the data to train, it's exhausting part of its privacy budget.
Chris Hazard (22:18): This is great because you're applying differential privacy. But as I mentioned before, some of the correlations and the data, the neural network may apply privacy sort of in a different way if it has any guidance over the training, or even if it doesn't. If there are certain artifacts that, due to statistical chance or likelihood, depending on how much data you push through this, the neural network may find those. And folks have found, and I will mention briefly in a little bit, that there are ways to extract and have a probability of detecting whether the data was in there. So by adding the noise twice, the first time with differential privacy and the second time with the GAN and how it generates, it's a little bit harder to characterize.
Chris Hazard (22:58): Similarly, Baeysian Networks often take handcrafting. Monte Carlo Markov chains, [inaudible 00:23:04] sampling, can be performance intensive. So there's a lot of pluses and minuses of these.
Chris Hazard (23:10): Now, what does a synthetic data set look like? Well, here's a nice toy example of a four dimensional data set. And from the original synthetic, you can see that they're remarkably similar. The trends are all there. You can see the insights. But the data isn't. It's a new set of points.
Chris Hazard (23:26): And we can even go one step further. And we can say, when we synthesize the data, let's make sure that we're never too close to any original case. Now, if you're dealing with a small one dimensional data set, let's say it's a social security number, well, about over half of the social security numbers have already been spoken for, they're already exhausted. So therefore by generating only ones that you don't have in your database, or that don't exist, you might actually be leaking a little bit of privacy there. So you have to be thoughtful about what is your sample? You're better off generating a nine digit number, a random nine digit number, unless you have to abide by certain laws that say that you can never reveal one that you already have. So now there's sort of a little bit of a conflict here between what is privacy maximizing and what is necessarily the most private, depending on your data set, depending on your situation.
Chris Hazard (24:17): But, as we get to these higher and higher dimensions, it becomes easier and easier. Supposedly at 50% of the social security numbers covered, some other percentage of something else, some other percentage of something else, those multiply and your data becomes very sparse. So again, we're able to create data that is never too close, based on the uncertainty, based on the smooth sensitivity of the original data, so that no one could ever find themself in the data even by chance. Not exact, but not even close.
Chris Hazard (24:45): And what does that look like when we're doing anonymity preservation exclusion is, if you look at the original data set on the left and the one on the right, you can't find a spot that matches up. You can't find a circle of a similar size and similar color that matches on both sides.
Chris Hazard (25:01): So this is all technology that is available today. As I mentioned before, you have to be very careful with regard to privacy and social graphs and time series. Even if you say that the lifetime privacy budget of a particular customer is one thing, what about that one customer who really uses a lot of your services? What if you're trying to maintain the privacy of contractual records between a manufacturer and different large sellers? Let's say that you've got Walmart and Amazon do, let's say, 40% each of their sales. And let's say there's a long tail of smaller resellers and sellers who sell the rest of it. Well, maybe let's just say Walmart was their first big customer, and they negotiated a certain deal which will expire in some number of years. And they would rather not leak that private information to other folks, because of NDAs or whatever other contracts they have.
Chris Hazard (25:58): And so if you've got this lopsided data where 40% of it is one customer, how do you make sure that that customer, that their privacy is protected as well? This applies no matter whether you're talking about individuals, if you're talking about organizations, corporations, governments. This applies across everything. In finance, there are different trades that might have certain curves, and there are different patterns of trading known as executions, some of which are proprietary. So how do you make sure that if a fund would like to share some insights with another fund or another analytics firm, how do they make sure that their proprietary trades are not compromised? Or let's say a medical device manufacturer who's looking at some sort of data, some sort of maybe imagery data, and they want to make sure that their patients are not re-identifiable.
Chris Hazard (26:52): When you start talking about unstructured data or images or text, we're just beginning to be able to push into those spaces with privacy. But it's also very important that we do so correctly today because of things that could bite us in the future. We see that for every new advancement in synthetic data that we create every, every new GAN that we create, there's a way of attacking it. So let's make sure that when we synthesize data, that it's as future proof as we can, so that we're protecting privacy for all of our customers in the future.
Chris Hazard (27:23): And there's many value in privacy trade-offs that we can make here. If you want to get the most value, the best way is to use the original data. If you want to get the best labels, if you want to get the best outcomes, use that, but you're also potentially incurring the biggest liability. Is there going to be a data exfiltration event where you're very careful with it, but not quite careful enough, and someone pulled that data out? Versus if you kept the production data in a smaller environment, synthesized data and pulled it out, and now worked with the synthesized data, regardless of whether it's analytics or data labeling, you can be more free with that synthetic data. If you just mask it, there's again, still different ways of extracting the private data out of there, or re-identifying folks.
Chris Hazard (28:10): If we apply GANS or we apply differential privacy with differentially private databases, there's still a lot of dragons there. There's still a lot of challenges, that if you apply it wrong you could lead yourself to problems in the future. So this is something that we at Diveplane really focus on a lot with our products. And on the other extreme there's just deleting the data. And we know many companies that are kind of afraid to unlock the value of their data, because it is scary if you do it wrong. So we encourage them to look at the state of the art what is possible with regard to this so they can unlock that value and start to do things with it.
Chris Hazard (28:45): So in conclusion, we see here that privacy affects behavior. It's not just about the laws. It is about what kind of customers do we want to have in the next five years, the next 10 years? What is the value? What is the trust in our brand? And the attacks against privacy are numerous. There's the traditional cyber attacks, to different attacks on differentiated private databases, to re-identification. And we see in the news, often again, the different ways of re-identifying people.
Chris Hazard (29:15): And differential privacy, that notion is very mathematically powerful. And any solution that you use should use that. But if you just use that, it's easy to misapply or apply wrongly. So you want to make sure that you're applying other privacy techniques, and applying checks and balances in applying differential privacy in a sound fashion. And synthetic data, which again properly should use differential privacy as a piece of it. When integrated tightly with the production systems and applied with the right other types of anonymity preservation and the right types of privacy measures, can be used to unlock that value of your data while being safe. And you can even synthesize data multiple times. You can synthesize it from the synthesized data. You could apply a privacy budget and have a sort of a higher fidelity and lower fidelity, as long as you stay within that budget. It unlocks a lot of different use cases. And you can use it almost as you could the original data.
Chris Hazard (30:14): But as always, you have to validate privacy and value. If you're trying to find that little squiggle, that little tiny signal in the noise, maybe it's hard to get that while maintaining privacy. Or maybe it turns out that you only need a small amount of data, 5% of your data, to be able to unlock 90% of the value, and make sure that you maintain privacy as well.
Chris Hazard (30:36): So in conclusion, synthetic data is a very powerful technology that can be used in many ways to enhance privacy, and can be used in conjunction with what I believe is problems that everyone here faces. So thank you for your time.
Chris Hazard (30:50): (music).