fast.ai: The Why, How, and Future of Democratizing ML
speakers

Jeremy Howard is a data scientist, researcher, developer, educator, and entrepreneur. Jeremy is a founding researcher at fast.ai, a research institute dedicated to making deep learning more accessible. He is also a Distinguished Research Scientist at the University of San Francisco, the chair of WAMRI, and is Chief Scientist at platform.ai. Previously, Jeremy was the founding CEO Enlitic, which was the first company to apply deep learning to medicine, and was selected as one of the world’s top 50 smartest companies by MIT Tech Review two years running. He was the President and Chief Scientist of the data science platform Kaggle, where he was the top ranked participant in international machine learning competitions 2 years running. He was the founding CEO of two successful Australian startups (FastMail, and Optimal Decisions Group–purchased by Lexis-Nexis). Before that, he spent 8 years in management consulting, at McKinsey & Co, and AT Kearney. Jeremy has invested in, mentored, and advised many startups, and contributed to many open source projects.
He has many media appearances, including writing for the Guardian, USA Today, and the Washington Post, appearing on ABC (Good Morning America), MSNBC (Joy Reid), CNN, Fox News, BBC, and was a regular guest on Australia’s highest-rated breakfast news program. His talk on TED.com, “The wonderful and terrifying implications of computers that can learn”, has over 2.5 million views. He is a co-founder of the global Masks4All movement.
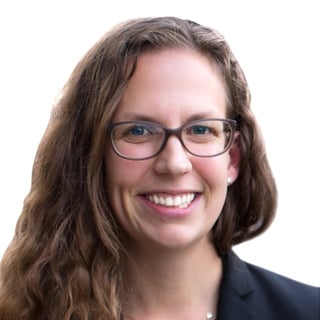
Rachel Thomas is director of the USF Center for Applied Data Ethics and co-founder of fast.ai, where she helped create the most popular free online course on deep learning, bringing people from around the world with diverse and nontraditional backgrounds into AI. Rachel earned her PhD in mathematics at Duke University and previously worked as a data scientist and software engineer. She was selected by Forbes as one of 20 Incredible Women in AI and was profiled in the book Women Tech Founders on the Rise. She wrote chapters for the books 97 Things About Ethics Everyone in Data Science Should Know and Deep Learning for Coders with fastai and PyTorch.
Rachel’s writing has been read by nearly a million people; has been translated into Chinese, Spanish, Korean, & Portuguese; and has made the front page of Hacker News 9x. Some of her most popular articles include:
- The problem with metrics is a big problem for AI
- If you think women in tech is just a pipeline issue, you haven’t been paying attention
- The real reason women quit tech, and how to address it
- Google’s AutoML: Cutting Through the Hype
- An Introduction to Deep Learning for Tabular Data
Rachel’s talks include:
- AI, Medicine, and Bias: Diversifying Your Dataset is Not Enough (Stanford AI in Medicine & Imaging Symposium)
- Getting Specific About Algorithmic Bias (featured talk at PyBay)
- The Barriers to AI are Lower than You Think (MIT Technology Review conference)
- The New Era in NLP (keynote at SciPy)
- Some Healthy Principles About Ethics & Bias In AI (keynote at PyBay)
SUMMARY
Jeremy Howard and Rachel Thomas sit down for a fireside chat explaining why they started fast.ai, how it progressed from classes to a software platform, the importance of community, and where they see the future direction of fast.ai.
TRANSCRIPT
Aerin Kim: Thank you Francois. Up next, we have Rachel Thomas and Jeremy Howard, the founders of fast.ai joining us from Australia. We are thrilled to have Rachel and Jeremy sit down for a fireside chat to discuss why they started fast.ai, how it progressed from classes to a software platform, the importance of the community and the future direction of fast.ai. Rachel and Jeremy, thank you for joining us and please take it away.
Jeremy Howard: Hi, my name is Jeremy Howard and I’m a co-founder of fast.ai.
Rachel Thomas: And I am Rachel Thomas and I’m the other co-founder of fast.ai.
Jeremy Howard: Fast.ai is a self-funded research development and education organization, and we’re doing this first ever co-founder fire-side chat or lounge chat, looking back over the last five years, where we’ve come to, why we’ve got here, what we’re working on. So yeah, pretty excited to bring you this today. Just by way of background personally, unlike Rachel, I don’t have a technical background in terms of my education. I studied philosophy at university, but I spent 10 years in management consulting, 10 years running startups in Australia and 10 years running startups in America. So most recently, before fast.ai, I founded the first medical deep learning company, which is called Enlitic. Before that I was the founding president of Kaggle and Rachel, on the other hand, what’s your background?
Rachel Thomas: So I have a PhD in Math, and I had studied math and computer science as an undergrad. And I feel like I’ve done a little bit of everything. I worked in finance. I worked as a data scientist in the tech industry. I taught at coding bootcamp. I’ve taught in a Master’s of Data Science program.
Jeremy Howard: So I mean, another interesting point in time right now is that we’ve just moved to Australia. So it’s also interesting to reflect on the future of fast.ai now that we’re here.
Rachel Thomas: I believe this is our first talk from Brisbane, Queensland. Very excited to be here.
Jeremy Howard: So how’s your g’day? Can I hear a good day from you?
Rachel Thomas: Good day, mate.
Jeremy Howard: Oh, terrible. We’ll work on that. So let’s talk about why we started fast.ai. So could you talk a little bit about what made you enthusiastic about the opportunities of deep learning and artificial intelligence, but also concerned?
Rachel Thomas: Sure. So this really goes back kind of before, before fast.ai. I remember in 2013, I believe it was reading in the New York Times about Geoffrey Hinton’s team winning a drug discovery competition using deep learning.
Jeremy Howard: A Kaggle competition, in fact?
Rachel Thomas: A Kaggle, yes. And they had been a very last minute entry and it was just wow, this is really, really powerful. At the time I was interested in wanting to learn more deep learning and it was very, very hard to find practical information. Most of the materials were just kind of very, very theoretical math and they didn’t give you the kind of code or what you needed to implement solutions. And I felt like I have a PhD in math. I’m working as a data scientist in the tech industry. If it’s this hard for me to get into the field, what are most people experiencing?
Jeremy Howard: I will say I was very excited about deep learning as a technology. So I had worked with neural networks over 20 years ago and that was in a retail banking environment on about $2 million worth of hardware. And I thought, wow, this is fantastic what we can do. And this was all around marketing applications in financial services. And I thought this is the future, but also it’s not something everybody could use because A, it’s cost $2 million. We needed basically a whole retail bank’s worth of data to get anything useful. And so I kind of put it aside, but then at Kaggle in particular, I saw competition starting to be won by deep learning. And I thought, okay, it’s finally happening. I knew it would happen, and it’s finally happening. And I actually did a talk, which you can find on ted.com about both my enthusiasm and my worries. It was called something like the-
Rachel Thomas: It’s the Terrifying Implications of Computers.
Jeremy Howard: Yeah, or wonderful and terrifying implications. And much to my surprise, that’s now had over two and a half million views and I don’t feel any differently now to what I felt then just… What was that? 2012?
Rachel Thomas: I think that was 2014.
Jeremy Howard: Neural networks have a fundamental, theoretical reason why they’re special, which is they can kind of theoretically model any possible function. And with the development we saw GPU’s around the 2013, 2014 timeframe that became practically usable as well. I thought, well, this is great. There’s this incredibly powerful tool that we could use almost anywhere. I’m thinking back to kind of my management consulting days. I was thinking, look at all the opportunities in industry for this, but at the same time, I know the kinds of problems that get solved with a technology and how it’s used really depends on who’s doing the solving. Did you want to talk about that? Because I know that’s something you have a lot of opinions about. We talked about back at that time and why we started fast.ai. Rachel Thomas: Yeah, so at the same time, I was both thinking and interested in deep learning, as well as frustrated with how inaccessible the field was. I was also getting frustrated with the tech industry. I had kind of had this experience of experiencing a lot of sexism and toxicity during my PhD in mathematics and academia, and had initially been excited to switch into the tech industry and do something different and then becoming disillusioned with the tech industry and finding oh, a lot of these companies have very, very toxic work environments that drive a lot of people out and kind of appeal to a very homogeneous group. And so I was becoming increasingly concerned, seeing… Okay, deep learning is this really, really high impact technology that’s going to change the world, but we have a fairly homogeneous group that’s able to use it.
Jeremy Howard: And we saw that, right? Like a lot of our friends were in those groups. And so they’re people we know and like that at the same time we can say they were all working on this small set of problems around-
Rachel Thomas: You had all studied with the same handful of PhD advisors at a small… This tiny handful of schools.
Jeremy Howard: And then we’re working on social networks, advertising, photo sharing. And like we were thinking, where are the people working on disaster resilience or water access or global education?
Rachel Thomas: And as well, the people with the deep domain expertise in those fields. And so not kind of techies applying this to fieldsvthat they don’t have the background in, but also kind of where the people who have the background in those fields.
Jeremy Howard: Yeah, because using deep learning effectively, like any data driven tool, requires data and a deep understanding of the problem to solve it to. The constraints for solving it and the opportunities. And so we both found that the people that were best able to drive the development of data products tended to be domain experts rather than kind of data scientists. So we thought, okay, how do we make the most of this? How do we help make the most of this technology by helping enable domain experts? The other thing that was driving us at the time was kind of looking at the history when there’s a step change in technology, it can often lead to a lot of hardship on other problems, a lot of issues around society. So for example, if you look at the history of the Industrial Revolution, that was not at all a smooth change, there were many decades or possibly even a hundred years of a decrease in the median income of a lot of people going hungry.
Rachel Thomas: Children working in factories, 12 hours a day in very terrible working conditions, lack of workers’ rights that made many people’s lives, materially, worse.
Jeremy Howard: And there’s fundamental reasons why that happens. When a new technology comes along, generally the people that can access that technology are people with capital. And so then they can spend that capital to harness that technology that’s getting more capital. And so, it at least for a while increases inequality.
Rachel Thomas: Yeah. It can kind of create a centralization of power and decentralization of capital.
Jeremy Howard: Which is not to say don’t have technology. You know, what we wanted to do is to think oh, can we find a way to help make a smoother transition? Everybody’s able to harness the technology and everybody’s able to benefit from the technology. So we decided together to start fast.ai. Did you want to talk about our ideas about how we might be able to make a dent in this problem or this potential problem?
Rachel Thomas: Yeah. And so our approach kind of had several prongs. We decided to start by teaching a course, and that was kind of a test bed for us to find what are the pain points? What are the things that can be improved and what are the hardest questions when it comes to kind of the practical and implementing things. And so we-
Jeremy Howard: So that’s not to say we decided to focus on education as an organization, but it was rather if our goal is to make deep learning more accessible, because by making it more accessible, it means that domain experts can harness search, including domain experts who have expertise in these kinds of societally important issues. So if we’re going to make it more accessible, then it felt like step one was figure out what’s already available, what we can already do and show people how to do that. But that was just the first step.
Rachel Thomas: And ultimately in this already has involved a lot of research and software development. So we kind of see the four prongs of fast.ai as research, software, education, and community. But I think even to motivate, we wanted our research to be high impact. And so in order for that to be the case, we had to see what are the pain points in the wild? You know, what are the things that are keeping this technology from being accessible? What’s the low hanging fruit here? And so that the research offered in development has really been driven by the information that we get by teaching the courses and by having a kind of this community of practitioners all around the world, working on a variety of applications.
Jeremy Howard: So let’s step that for a moment and think about what this end goal might look like. So what would it look like for deep learning to be truly accessible? Well, It’d mean that everybody can use deep learning, who can take advantage of it in their jobs, in their hobbies and their passions. Now, most of the world by far doesn’t code, so if it’s going to be accessible, then it can’t rely on code. Most of the world doesn’t have access to data centers full of GPU’s. So it can’t rely on too much compute. Most of the world doesn’t have access to hundreds of millions of rows of data. So it can’t rely on that. So, and most of the world doesn’t have a PhD in math, also some do so it can’t rely on that.
Jeremy Howard: So, so we kind of came up with this idea of developing an iterative loop where the loop, it’s kind of a spiral that eventually hopefully ends up in a situation like where we are now for the internet, where my 80 year-old mom uses the internet every day, but she doesn’t know how to set up TCP, IP subnets, masks, and she doesn’t have to know how to set up PPP or whatever. She just does stuff on the internet. So that’s kind of what, where we went on to eventually get to.
Rachel Thomas: I think another good analogy is spreadsheets, like Excel. And that’s something where people are using Excel across a ton of different domains and while there are Excel power users or experts, you largely though, a lot of people are proficient in Excel, but their expertise is in whatever domain that they apply it to. But it’s a tool that’s in a lot of people’s toolbox.
Jeremy Howard: And they don’t have to know about assembly, language, micro code architectures and whatever, exactly. So, the spiral that we hope to get to that point involves initially starting with education, which is to say, okay, here’s the software that exists right now. Here’s the data that you can find right now, here are the techniques that are available right now. Here’s what you can do with it. Here’s what you can’t really do with them. Here are where the opportunities are right now, and take advantage of this. Here are the pain points. And so that became the first version, of course, fast.ai, which, I mean, it was a huge experiment because a lot of people didn’t think this would be possible. I mean, they, it wasn’t like there were any other general deep learning MOOCs for a kind of non-math, non-graduate audience, right?
Rachel Thomas: Right, right. Yeah. And it can be hard because things have changed so much since 2016, when we ran the first version of the course, that can be hard to remember how few resources there were and that the resources out there were primarily kind of theoretical math, building from first principles, what do you need to just understand the math theory behind deep learning, but less so about implementations, much less cutting edge state-of-the-art implementations, which was always our goal and was always part of the course that we didn’t… We never wanted to just be using toy examples or things that were kind of not good enough performance to actually use in production, but to really be taking people to the state of the art.
Jeremy Howard: Also, I remember as we started out that first course, there was a lot of push back from folks in the deep learning community around… this is giving people ideas above their station. You know, people aren’t going to be able to handle this. If they don’t have a graduate math background, don’t give them the impression that they can do anything useful because frankly they can’t. Do you remember that conversation?
Rachel Thomas: Yes, I do. I do. And I also remember seeing a lot of advice from someone who would say they were interested in deep learning and see us on sites like Hacker News. And then someone else would post, these are all the textbooks you need to buy. And you need to start with kind of learning all these areas of theoretical math and coding and CUDA, and writing your own compiler and things before you can actually start learning deep learning.
Jeremy Howard: So anyway, we were delighted to find that this experiment, which honestly, as when you’re doing something new like this, it’s hard not to listen to all the negativity. And we were certainly concerned, maybe we would fail. That would give it a go. And yeah, we were amazed that this totally new, unknown, unfunded organization, the very first course we put out had hundreds of thousands of people taking it and kind of instantly transformed almost the way people talked about deep learning education. And within a year of us putting out this course, I feel like the phrase democratizing deep learning went from an unknown, weird, impossible dream to almost like something that everybody was talking about.
Rachel Thomas: The other thing is most education tends to be bottom up and we’ve talked a lot. Most technical education tends to be bottom up.
Jeremy Howard: So starting with the math-
Rachel Thomas: Yes, and you have to learn each building block you’re going to use. And then in a few years you’ll be able to stack these building blocks into something interesting. And so we wanted to use this top-down approach. And while we had read about it, like David Perkins and other fields, I had-
Jeremy Howard: Paul Lockhart.
Rachel Thomas: Paul Lockhart. I had never heard anyone apply top-down education to deep learning before fast.ai. And that’s something that we got a lot of skepticism about as well, but the idea that we’re going to get people-
Jeremy Howard: Well, not just skepticism, but almost this kind of angry… Okay, you’re showing people how to do stuff in less than one to like run some pre-canned model, but this is dumbing it down, you’ll never be able to do anything useful.
Rachel Thomas: Yeah, they don’t understand all the details and the dumbing it down accusation. Yeah. A lot of people said that, but really the idea was that in each subsequent lesson we were digging into more and more low level details and getting lower and lower levels. And-
Jeremy Howard: And we ended up being the only ones actually, or were some of the very few that actually taught, like how does automatic differentiation actually work? How does back propagation actually get done on a computer in a performant way?
Rachel Thomas: And by part to us, our first year of the course was spring 2017. We were implementing papers as they were coming out during the course and-
Jeremy Howard: I remember we did the 100-layer tiramisu.
Rachel Thomas: 100-layer tiramisu, neural style transfer was relatively new then. And our goal was to teach people, how do you read a new paper and implement it yourself?
Jeremy Howard: Yeah. So, I mean, at the same time the outcome of that first course, I mean, it did show us the key thing we were trying to figure out. Well, two things we really wanted to know. One was, is there any point doing this at all? Is it possible for people without a math PhD to do useful work? And the answer was, oh my God, yes it is. Lots and lots of students came out of that course doing breakthrough work, getting published in academic papers, getting patents, building startups. But the second thing they wanted to know was yeah, where are the hard edges? And we found a lot of hard edges. In fact, pretty much anything except computer vision, object recognition was almost impossible to do in practice, outside of academia. So from that, after that first course, we went fully into research mode.
Jeremy Howard: So that was kind of the second part of our spiral. So education research, and one of the big wins there was, we thought, well, how do we turn these great computer vision results, into natural language processing results? And out of that research came the ULMFiT algorithm, which basically said, you know what? You can just use the computer vision ideas directly in NLP and they’ll work. And so that’s what ULMFiT was. I coded it up in like four hours and it worked the first time. One of the challenges for the idea there was that I spoke to lots of NLP researchers before I tried this. And all of them said, it’s not going to work. All of them said, NLP is different. It’s special. You know, forget it.
Rachel Thomas: And the core idea Jeremy’s talking about is using transfer learning. So for instance, training on texts from Wikipedia first, and then applying that to a different corpus of text for kind of fine tuning your model.
Jeremy Howard: Yeah. So like that was a great example of the research that we had to do. And then, so it was really cool because I presented the research actually in a class, in a part one class actually, and then a great PhD student called Sebastian Ruder, saw the video and said that’s publishable. You know? And I was like, “I don’t even… I don’t know how to publish this.” I don’t know anything about publishing. I’m just a philosophy graduate. And he was like, “All right, I’ll help make it happen.” And so him and I wrote it up and he helped with lots of experiments and wrote most of the paper and got published in the top computational linguistics conference, which was amazing and kind of helped kick off this modern era in NLP. And so that was also a nice kind of tick for our idea, which was like doing the course, finding a hard edge, which is like NLPs doesn’t really work. Everybody doing NLP at that time, almost everybody was doing pretty academic stuff, like entity recognition or kind of passing.
Jeremy Howard: They were like sub-problems that you couldn’t hand it to somebody in an organization and say, “Here’s how this solves your problem.” So I just wanted to do something very directly applicable, let’s just get classification working. So it was cool to be able to say okay, this approach of taking out learnings from the course and using it to solve a problem in research. And then the next thing we did was software development was we… so this was built very heavily on top of Stephen Merity’s work LSTM, which was research code, but code, but research code. And so it was nice to be able to then take that along with some code that James Bradbury developed and start to build a library around it. And so then we were able to put that out on the internet and say, okay, there’s now this thing called the fast.ai library. And there’s a thing called fast.ai dot text. And you can install that and use your ULMFiT yourself. And we had this script that was a dozen lines of code that will give you the same results that we got in the paper. And so this was getting up to step three, the course, the research, and then the software to implement that research.
Jeremy Howard: So then the fourth pillar is community, which you want to talk about that event?
Rachel Thomas: So the courses actually, I should probably give us some background, the courses prior to the pandemic, we used to teach in-person at the University of San Francisco. It was an evening course, kind of open to anyone and you didn’t have to be enrolled in a degree program at USF. We had from the very, very start, we have diversity fellowships. We had international fellowships to have people from all over the world, participating in real time to really try to build a kind of diverse and varied group of people taking the course. And we also have something that was amazing to us on the kind of unexpected, is people from the later courses, we would have people come from around the world because they wanted to take it in person and be a part of that community. But we also had a very active online community forums on fast.ai.
Jeremy Howard: And the reason we built that up is because we thought, okay, like a lot of these people going through the course and using our software we’re as we hoped, domain experts, but often they would be the only people at their companies to be using deep learning or interested in deep learning. And that would be pretty isolating and pretty difficult. So by providing an online community, that was really helpful because people could find each other, and could help each other. A lot of people were trying to break into jobs. So people helped each other with interviewing, people helped each other with reviewing their projects. Without that community, we would be missing the critical piece around people helping each other.
Rachel Thomas: Yes. And we’ve seen that with so many fast.ai alums writing blog posts to answering each other’s questions, starting podcasts. There have been examples of a fast.ai students live coding together while they’re in the course, often kind of remotely. We have a study group going during the course, but that component of helping each other and learning together is so important. I have to say one other thing that we emphasize and have from the start is ethics, and kind of the importance of really thinking about the impact of our work and the ethical implications of it. And that has been a part of the course and really including it as a core part, something I love is in the book, which is that deep learning for coders with fast.ai and PyTorch libraries.
Jeremy Howard: Deep Learning for Coders.
Rachel Thomas: Deep Learning for Coders, chapter three is on ethics. And we really try to keep that front and center that ethics is not an add-on elective and it’s not tucked in an appendix at the end, but to really have that as an integral part of the course in the book to really think about what are the risks and harms of a potential misuse, and what should we be doing to very proactively prevent and address those.
Jeremy Howard: That’s kind of even more general than that. It’s like throughout the course and throughout the book, it’s all about practical. So it’s all about like, what does it take? How do you get the data? How do you deploy the models? Chapter two of the book is all about deployment.
Rachel Thomas: Also, I think it is great to start with deployment. Cause that’s also something that tends to kind of be forgotten or just tacked on at the end in a glossary way.
Jeremy Howard: Right? A lot of the kind of academic folk who teach would know how to properly segue. I can’t talk about that. And throughout that, it’s all about well, how do you avoid feedback loops? Where do you need a human involved? And so the ethics component is very tightly knit with just the practical development of data products. Now having said all that, I feel like we’re just scratching the surface towards our goal. You know, deep learning is still very exclusive because as it stands, you still need to know how to code.
Rachel Thomas: It’s also still an overly homogeneous field, we do not have the diversity that we need yet.
Jeremy Howard: Yeah. I’m sure as you do our course, although you only need high school math to start it, we teach you a lot of additional math on the way, which is, we kind of made, yeah. I mean the vast majority of people in the world are still not able to harness deep learning effectively, which is fine. We didn’t expect to solve that problem in five years. I think we’re very happy with how far we’ve got, thus far. But you know, from here, what’s next for fast.ai? Our focus has been moving gradually from education as being the initial thing. We were working on more and more then towards research and then more and more towards software development. And I think we’re going to keep heading in that direction because software development is the thing that… It allows us to actually remove the barriers entirely. If you can get to a point that the software is so capable, and so easy to use, that you don’t need a course. You don’t need prerequisites. Then that feels like the only way we’re actually going to really solve this problem. Especially if that software will run on any computer and we’ll work out of the bumps with small datasets, this feels like the way we eventually remove the barriers entirely.
Rachel Thomas: Yes. And I would say we are planning to teach an in-person course in Brisbane and that this goal of software development is still in… I mean, the goal of software being kind of all you need and no education being necessary is still-
Jeremy Howard: Probably many years away.
Rachel Thomas: Many years away.
Jeremy Howard: So we’re still doing the loop?
Rachel Thomas: We’re still doing the loop, yeah. Do you want to say more about our move to Australia?
Jeremy Howard: Yeah. I mean, I’m not sure it means much. We’re still doing the same four things and we’re still doing it in the same iterative way. Hopefully the course will probably stay the same length, 14 lessons, two parts of seven each. But what happens is each year the amount we pack in gets bigger because thanks to the software and the research, there’s less to teach and we can do more with less. So hopefully, once you get in this process of each year, at the end of the 14 lessons, you’ll be able to do more and more and more. And also we also kind of hope that, and refining this, is working the more we do the course, the fewer lessons most people need.
Jeremy Howard: You know, we don’t want you to do all 14 lessons. We actually want you to ideally to do, have to do no lessons, which is not where we’re at now, but where we’re at now, I think a lot of people just need three lessons to make a reasonably good start, but hopefully we’ll keep making it so that you can do more with less. The answer to what now that we’re in Australia is things will continue to look as much as they do now. I think Rachel actually, you’ve been involved… We’ve been focusing on ethics for the last couple of years now.
Rachel Thomas: Yes, and particularly the last two years, I was founding director of the Center for Applied Data Ethics at the University of San Francisco, so that was my full-time focus.
Jeremy Howard: But hopefully now you might have a bit more time to…
Rachel Thomas: Get back into fast.ai more. I’m excited for it.
Jeremy Howard: Yeah. So thanks everybody for joining us. And I hope you enjoy the rest of your conference.