Democratizing the Benefits of AI with Kevin Scott of Microsoft
speakers
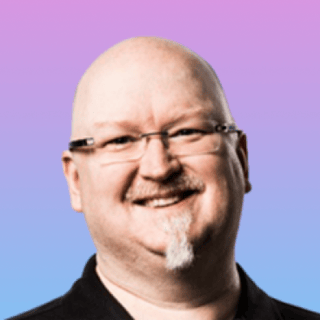
Kevin Scott is executive vice president of Technology & Research, and the chief technology officer of Microsoft. He is an innovative leader driving the technical vision to achieve Microsoft’s mission, and is passionate about creating technologies that benefit everyone. He focuses on helping make the company an exceptional place for engineers, developers and researchers to work and learn. Scott’s 20-year career in technology spans both academia and industry as researcher, engineer and leader. Prior to joining Microsoft, he was senior vice president of engineering and operations at LinkedIn, where he helped build the technology and engineering team and led the company through an IPO and six years of rapid growth. Earlier in his career, he oversaw mobile ads engineering at Google, including the integration of Google’s $750 million acquisition of AdMob. At AdMob, Scott was responsible for engineering and operations for the world’s leading platform for mobile monetization. Before joining AdMob, Scott held numerous leadership positions at Google in search and ads engineering and helped with the company’s early efforts establishing remote engineering centers. Scott is the host of the podcast Behind the Tech, which features interviews with technology heroes who have helped create the tech industry of today. He also authored the book “Reprogramming the American Dream”, which explores how artificial intelligence can be realistically used to serve the interests of everyone, not just the privileged few. As co-inventor on several patents around search and information extraction, he has also authored several publications on dynamic binary rewriting that collectively have been cited hundreds of times in other scholarly research. He has received a Google Founder’s Award, an Intel Ph.D. Fellowship and an ACM Recognition of Service Award. He is an adviser to several Silicon Valley startups, an active angel investor, the founder of the non-profit organization Behind the Tech, a member of the Anita Borg Institute’s board of trustees and a trustee of The Scott Foundation. He also serves on the Advisory Council for Stanford University’s Institute for Human-Centered Artificial Intelligence (Stanford HAI) and the Leadership Council for Harvard’s Technology for Public Purpose (TAPP) program. Scott holds an M.S. in computer science from Wake Forest University, a B.S. in computer science from Lynchburg College, and has completed most of his Ph.D. in computer science at the University of Virginia.
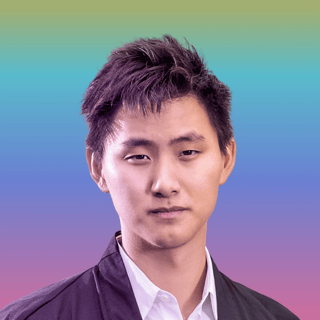
Alexandr Wang is the founder and CEO of Scale AI, the data platform accelerating the development of artificial intelligence. Alex founded Scale as a student at MIT at the age of 19 to help companies build long-term AI strategies with the right data and infrastructure. Under Alex's leadership, Scale has grown to a $7bn valuation serving hundreds of customers across industries from finance to e-commerce to U.S. government agencies.
SUMMARY
Kevin Scott, CTO of Microsoft, discusses recent drivers of progress for AI/ML, macro trends shaping the future, and how Microsoft is approaching the challenge of ethically and equitably scaling the benefits of AI.
TRANSCRIPT
Brad Porter: I’m very excited to welcome our next fireside chat guest. Kevin Scott. Kevin is the Executive Vice President of Technology and Research, and the Chief Technology Officer of Microsoft. Kevin is a prolific multihyphenate. He’s a builder, having previously led engineering at LinkedIn through an IPO, and overseeing mobile ads engineering at Google. He’s also an advisor to several Silicon Valley startups, and an active angel investor. He’s also the host of the podcast, Behind The Tech, which features interviews with technology heroes who have helped create the tech industry of today. Kevin is a published author whose book Reprogramming The American Dream, explores how AI can be realistically used to serve the interest of everyone. I could say much, much more, but with that, I will turn it over to Kevin. Welcome, and thank you so much for joining us today.
Alexandr Wang: Thank you so much for joining us Kevin, really excited to be chatting.
Kevin Scott: Thanks for having me.
Macro Trends in the field of AI
Alexandr Wang: One of the things that’s always great when I talk to you is that you’re able to bridge this massive divide between, knowing the technical details very well, while also being able to have a very clear macro picture on how that technology is affecting the world. So the first question I have for you is, what do you think are the macro trends that are going to define the future of AI over the course of the next decade?
Kevin Scott: I think there are things that we all are relatively familiar with, so AI is certainly getting more powerful over time. I think the interesting thing about the macro is that we have an interesting set of challenges that are facing us as a society where AI, I think is an important part of the solution. So you think about things like climate change, like the thing that we just experienced, which is going through a viral pandemic. AI I think is and will play a huge role in helping us tackle these things. And if you think more broadly about how you provide healthcare, and just access to high quality medical services across the board, like that gets much more important over time. I n most of the Western world, we’re about to experience a demographic inversion.
Kevin Scott: So we will very shortly have far, far more elderly people who are retired and out of the workforce, and who have different medical needs than younger people, than we have working age folks. And so how to take care of that aging population, how to make up for the productivity that they represent as they leave the workforce in retirement. Again, all of those things I think are going to be places where AI is critically needed. So I’m just looking at a world where, if we don’t invest super heavily in AI and figure out how to deploy things as quickly as possible, to solve some of these big societal challenges we’re going to struggle.
Advancements in the Field of AI
Alexandr Wang: It’s a very interesting, macro view looking at the other end to the things that are happening on the technical ground. What are some of the exciting advancements you’ve seen in the field of AI, that you don’t think people are talking enough about or excited about?
Kevin Scott: The thing that we’ve seen over the past couple of years in particular, is these large self supervised language models have really revolutionized how natural language processing is working. So this started with Google’s BERT models. And this whole notion of models that have attention or self attention, and this transformer architecture for deep neural networks is proving to be an extremely powerful thing, and we’re already starting to see the same basic set of ideas applied to different domains. So we’re quickly going from language to, visual representation models that you can pre-train and then, use in a whole bunch of different applications. The two properties of these models that are just fascinating is one, because they’re self supervised, you get into this mode where you’re primarily constrained by the amount of compute that you can throw at building the models.
Kevin Scott: So if you have a big enough supply of data, and it’s like unlabeled data for the pre-training set of tasks, and you can throw lots and lots of compute, you can build bigger models and the models are getting more powerful with that scale, so that’s super exciting. And then the other exciting thing is these models as they are getting bigger, transfer learning is actually working really well, and so you can train one model and then with either no additional training, or some amount of fine tuning with supervised learning, you can use them in a huge range of applications. And so you can start to think about the bottles themselves as platforms, so like they’re reusable, you can compose them. I think that is just an incredibly exciting thing. I know all of us like probably the people attending this conference have internalized that, but I don’t think the tech industry at large has really internalized what a profound change that is.
Alexandr Wang: Is a super interesting notion this thing you mentioned around models as platforms. Because I think historically, models have always been this very fragile thing, they’re almost anti platforms they are very specific. The idea of models being so generalizable, so composable, so reusable that they become platforms themselves, is very powerful and there’s a lot of economic implications. One thing that’s related to what you just mentioned was that, we’re in this regime as you described, where for the state-of-the-art results or the best possible algorithms, you have to keep throwing more and more compute at them, keep throwing more and more data at them, such that the economic constraints of actually creating these very large algorithms, has gone from, millions to tens of millions of dollars to train these like very, very large algorithms. Where do you think this goes long-term, and what do you think the implications of that are on AI development and the industry of AI?
Kevin Scott: We certainly have this really interesting crank, that we are able to turn right now with these large models where I don’t think we’re at the point of even diminishing marginal returns yet. So we just know that you can use more data and more compute to create bigger models that have increasing amounts of power, but it’s really striking to think about, the difference in the amount of energy consumed by training one of these models, and the energy that a human brain consumes, which is in the tens of Watts for a human brain. And these big model training runs consume enormous amounts of energy. And so I think that is a clear opportunity for us to be thinking about more efficient algorithms, more efficient ways to train these models. The thing that I would say to everyone is, I don’t think we’re at the point now where all of AI is solved. Every time anybody has said that AI is solved, they’ve figured out the architectural approach or the technological approach to solving a set AI problems, they’ve been wrong.
Kevin Scott: I think there’s just enormous amounts of opportunity for innovation going forward. It is exciting right now to think about how we can put the power of these big models into lots of hands. So while it’s expensive to train a big representation model for a particular domain, what we’re seeing internally is that, it may actually be cheaper than what we were doing before because of these platform effects due to transfer learning, you train the model once, and then you’re able to just deploy it on a whole bunch of different scenarios, where each of those scenarios before might’ve required a team of data scientists, accumulating a whole bunch of data, training a model, and you’ve got a bunch of separate models that each require their own training regime to do each one of those tasks independently.
Kevin Scott: We don’t have conclusive data yet right now, but it could be that the platform effect makes it net cheaper. And then, if that is true, then it becomes mostly a question of access. Like how do we package these models up in a way where everybody can use them? Like we figured out how to do it inside of Microsoft in a reasonable way. We have not figured out a great way yet, how to package these things up so that we can put them in the hands safely for third-party developers. And that’s the thing that we’re focusing an intense amount of energy on right now, to try to figure out how to both empower people, but to do it in a safe and responsible way.
Alexandr Wang: The things that you just mentioned that I think is super interesting is how the technology affects the economics of what it means to build amazing products. The parallel I’ll draw is, the incredible thing that happened with software is that, while it takes an incredible amount of upfront investment to build software, the cost to reproduce software is very, very low. And that change in the physics of economics, had large implications for how obviously technology and the economy has developed in the time since then. You mentioned one of them which is that, there’s these platform effects of these large algorithms, in terms of making it so that the marginal development cost is actually low. Are there any other changes in the physics of the economics of technology that you think are caused by AI?
Kevin Scott: They’re a bunch of things that probably are going to change. You alluded to it in what you just said which is, for the entire history of computing and software development, harnessing the power of a computer was about programming it, which is a trained programmer trying to come to a human understanding of how to solve a problem, and then translating that human understanding into, a set of step-by-step instructions for telling your computer how to go solve the problem. And so it’s not the easiest thing in the world. It’s easier now than when I started programming when I was 12, because we’ve figured out very cleverly how to layer abstractions, and you just have more power now than ever in a single line of code just does more. The really interesting thing that I think is happening right now is, big chunks of software development is going from programming to teaching. I think this is something that you all very, very deeply get.
Kevin Scott: So you’re now able to harness the power of the computer to solve problems for people by, teaching it how to solve a problem, which I think is a much more accessible way of harnessing that power than programming. Like even a two year old child can teach other human beings how to do something. So teaching as part of what we all understand how to do. I’m really hopeful that that will be one of the things that really changes, the laws of physics as you put it of how software development happens, that we just have more people who are able to do a richer set of things with technology as a consequence of machine learning.
Ethical AI
Alexandr Wang: Definitely. Well, I wanted to focus in on one of the things that you mentioned earlier around responsible and ethical AI. You published this book last year called Reprogramming The American Dream, in which you talked about your own journey from growing up in rural West Virginia, to becoming a leader in technology and AI, how that shaped a lot of your beliefs. You also talked about how you believe that AI could be a massive source of good for the general public. What are some of the ways in which you think that AI is going to positively impact people outside of the tech industry?
Kevin Scott: Let’s just pick healthcare, and how we’re solving major health crises, like the COVID-19 pandemic. What we’ve seen is that as we partner with companies in developing therapeutics and vaccines, increasingly that development process is about harnessing like very, very powerful simulations of how molecular systems behave, and using increasingly sophisticated data analytics in all parts of the therapeutic and vaccine development life cycle. One of the really exciting things to me is that we’ve seen it first in diagnostics.
Kevin Scott: AI as a diagnostic tool is getting really quite powerful. What I’ve seen over the past 12 months is AI as a way to help accelerate the development of medicines is becoming increasingly powerful. I talk about this in the book, in the '50s and 60s, we had this interesting set of developments in aerospace. And at one point we decided as a society that we were going to create the Apollo program that we were going to build all of the technology required to send a person to the moon.
Kevin Scott: In a certain sense that moonshot was arbitrary. We didn’t need to go to the moon. What we needed was to get everyone focused on this audacious goal and to build an entire ecosystem of technologies that would help with defense, would help with travel and transportation and even change our mindset of how technology plays a role in shaping society.
Kevin Scott: The whole notion that we’re going to the moon. We say, moonshot right now to be a euphemism for an ambitious, like a really ambitious thing. That effort shaped our vernacular how we think about the world. I think we have an opportunity right now with these technologies where you could pick a thing like healthcare. We could say, we want to figure out how to give every person access to cheap, super high quality healthcare, where they had a better life from the time they’re born until the time that they pass away and that we are going to take technologies like AI, which I think are going to be very important to providing that.
Kevin Scott: But we look at it all up and say we’re going to do whatever it takes to use technology to make that vision a reality. So I think there’s that opportunity for AI. In a sense you don’t have to pick healthcare, we could pick climate change, you could pick other problems, but I think we could pick better than the moon, right? Because these things are not arbitrary. They are real, tangible things that we struggle with that if we were able to solve them, no one would have any argument whatsoever that they would make the world a legitimately better place.
Alexandr Wang: Yeah. What are that set? You mentioned a few of them, healthcare, climate change. What are that set of AI moonshots so to speak that you think that for people watching, or people excited about the industry of AI that we should be focused on?
Kevin Scott: I think those are maybe the two most important ones, but you do have another couple of ones that are tangentially related. One of them is trying to figure out how we can take care of an aging population and partially that’s about healthcare, but partially it’s also about how we balance out this changing nature of how do you deal with the productive output of society? At the same time that you’re compassionately caring for the aging population ensuring them that they have dignity throughout the full course of their life.
Kevin Scott: There’s a whole bunch of interesting things that are not necessarily about medicines and diagnostics that AI, I think could help with there. And then you also have, this is related to climate change a little bit, but we have slowing population growth, which is going to result in this demographic inversion that we’ve talked about in most of the developed world.
Kevin Scott: So for sure in China, Japan, Korea in Europe and in the United States ex immigration. But that doesn’t mean that we’ve hit peak population yet. We probably still have another two and a half, three billion people who are going to be added to the population. And a lot of that population growth is going to be in Southeast Asia and in Africa. And so if you look at that, another three billion human beings that are coming into the world at the same time, that climate change is making agriculture more difficult.
Kevin Scott: I think we’re going to have to look at technologies like AI to figure out how we can optimize our agriculture so that we can feed everybody. That’s the bucket of things that I’m thinking about, and I’m sure we had all of the smart people in the world thinking about what could you do if you had 2% of your GDP like the United States GDP to invest in technology whose benefits accrued entirely to the good of the whole, other interesting things would come up as well.
Role of Datasets in Ethical AI
Alexandr Wang: Yeah, I think what you just described in terms of AI for agriculture is super insightful and something that I actually think not that many people are focused on, because I think that those set of macro trends are maybe not as obvious to most people. Stepping back or stepping into the weeds and thinking about ethical and responsible AI, what role do you think the data sets and the algorithms play in an ethical AI today and over the next few years?
Kevin Scott: I think we have an enormous amount of work to do. And I think the landscape is evolving very, very rapidly. I think we are learning a lot of things right now about biases and data sets. We’re learning a ton of stuff about how to manage potential harms that come from uses of AI. One of the things that we have at Microsoft is this thing called Office of Responsible AI. And we have a set of training and a framework to look at sensitive uses of AI. And it requires people to think about the harms that can come from applications of these AI systems.
Kevin Scott: I think we have an inclusivity challenge right now. The thing that we mentioned that these big models are becoming more expensive to train means that it’s harder to have a huge number of researchers participating in the development and figuring out how to get that inclusive set of voices in the development of the models and the algorithms and the data handling practices, I think is super important and something that we’ve got to think a lot about.
Kevin Scott: So, yeah, it’s just a bunch of stuff that we’re going to have to do. I mean we’ve done some really interesting work on Explainable AI. You imagine AI is going to be so useful in so many different contexts. In some contexts you’re always going to need to have a human in the loop where AI is recommending things. And then those recommendations even are going to have to be explainable so that a human can look at the output, understand why the AI made the recommendation that it made. And then the human is going to make an informed decision in other applications, you’re going to have things that are fully autonomous where the AI is making decisions at a superhuman rate like what you need in a self-driving car where they’re probably thousands or tens of thousands of decisions, a second are happening to keep everyone in the car and on the road safe.
Kevin Scott: And so we just have to understand this full continuum of things and make sure that we have tools for every one of the applications that make sure that the way that the AI is being used is being used in a safe and responsible manner as humanly possible. The corollary that I will draw is we had 75 years or so from the beginning of modern software development and high level languages happened in the '50s until now to figure out how to develop a set of practices that help make sure that the software that we’re writing is safe. And even then there’s an interesting set of theoretical results in computer science that bound what it is that you can say about a piece of code before you deploy it.
Kevin Scott: And so you just never know for sure if the code that you write is going to behave exactly as you have intended. And as a consequence, we built up all of this mechanism to help us try to find as many possible failure modes of the software as possible before we deploy it. And then after we deploy it, we’ve got a set of tools for monitoring the behavior of the software, identifying failure cases, and then controlling the impact when we do find those failure cases. And we’re going to have to work out a similar framework with AI. I think the good thing is the work is starting. And we’ve got lots of smart people with a lot of passion and working on it right now.
Alexandr Wang: I think what you described is super interesting in particular this concept of what are the methods and the mechanisms and the technology that you’ve built to allow us to feel confident of the code that we’re releasing and feel like that we’re doing that in a responsible and ethical way. And what are the parallels of that to AI? Because they think that AI is not the first technology that we’re going to be rolling out at large scale.
Strategic AI Initiatives at Microsoft
Alexandr Wang: I know one thing that you and the Microsoft Team is really focused on is what are the platforms and technologies that are going to enable developers to be able to actually utilize the benefits of AI and make it more democratized and more accessible? What are some of the strategic initiatives that you and the Microsoft Team are considering to enable this goal?
Kevin Scott: There’s a bunch of stuff that we’re doing. And I think you have to be fully cognizant that people are going to come to AI in a bunch of different ways. We are plugging fairly sophisticated flavors of machine learning into tools that people use Power BI and Microsoft Excel, where you don’t have to know much at all about machine learning to be able to solve classification or regression problems. In the back end we’re using some very sophisticated auto ML tools to look at your data set and the thing that you’re trying to do, the objective function that you’re trying to optimize so to speak and yeah, helping you to not just automatically in the background, set the hyper parameters for a training process, but to select the algorithm and the neural architecture for the type of machine learning that ought to be applied to the particular problem that you’re solving.
Kevin Scott: You just can come to it with relatively minimal expertise. We bought this company a few years ago and this is now released to the public called “Lobe” where we wanted to make it really, really, really easy for people to build AI tools. I’ve told this story a bunch of times, one of the designers, founder of Lobe lives in an off-grid house. He’s a designer by training and wanted to be able to write this little web app that would show how much water is in this cistern that they use for drinking. The cistern gets filled up by a well during the day, powered by solar panels. And at night when you’ve got no solar panels, gravity feeds the house. And so it’s important to make sure you’ve got enough water in this thing before the sun sets.
Kevin Scott: The way that I would solve this problem, maybe you would solve this problem is you put a bunch of sensors in the tank, get a little raspberry PI or Arduino and take the sensor outputs and translate them to water levels. You’d wire it up to the internet, you’d write some code for this embedded control bore, you write some more code to move the data to the web app, blah, blah, blah. Right? The way that he wound up solving the problem with Lobe is he put what amounted to a toilet float in the water with a piece of rope tied over it, slung over a pulley. And he had a marker, a little piece of wood that would float up and down on the side of the cistern.
Kevin Scott: When it was high it meant that the float was low and that the water level in the tank was low when the marker was low on the side of the tank, that meant the float was high. And you had a high water level. He took a bunch of pictures of the position of the float and notated how much water was in the tank with every one of those pictures, pointed a webcam at this thing, and then trained a model with Lobe that translated the pictures of that float into water levels, pressed a button. It publishes a nice little JSON:API. And then he wrote some code in his web app to build a nice little interface that showed the water levels. That, again, going back to this notion of teaching versus programming, that is a very, very different, but powerful way to solve problems.
Kevin Scott: We’re thinking about all of those ways that we can give people tools to get new people thinking about these different ways to solve problems with these tools. We’ve got things all the way on the other end, we’re building interesting infrastructure for our teams that are building these large models for OpenAI that we’re partnering with, that’s really just way out on the frontier of what’s possible technologically. And so I think our approach is just full spectrum. It’s like, let’s think about every developer and give them what they need, where they’re at to use these tools.
Alexandr Wang: Kevin, that was incredible. Thank you so much for joining us for Scale Transform. It’s always great to hear your thoughts, not only about the long-term future of AI, but also the exciting work that’s being done technically today. So thank you again. It was really great.
Kevin Scott: Well, thank you so much for having me, I always enjoy chatting with you and I think you’ve built an incredible community and it’s just great to see things evolve. So thank you for all the work that you’re doing.