Sign in or Join the community to continue
Challenges of Developing Models for Gigapixel-Scale Pathology
Posted Oct 19, 2022 | Views 1.1K
# TransformX 2022
# Breakout Session
Share
speaker
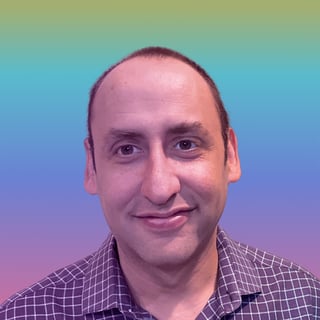
Nathan Silberman
Formerly, VP of AI at PathAI, Butterfly @ ML Executive
+ Read More
SUMMARY
+ Read More
Watch More
What's Next for AI Systems & Language Models With Ilya Sutskever of OpenAI
Posted Oct 06, 2021 | Views 23K
# TransformX 2021
# Fireside Chat
Designing for Autonomous Vehicles at Scale With Hussein Mehanna of Cruise
Posted Oct 06, 2021 | Views 5.7K
# TransformX 2021
# Fireside Chat