Sign in or Join the community to continue
Reverse Engineering of Generative Models
Posted Jan 31, 2023 | Views 1.9K
Share
speaker
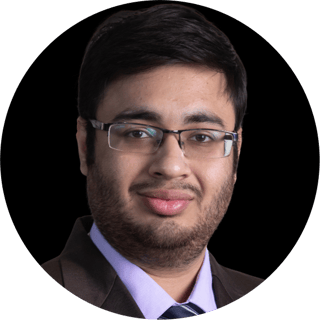
Vishal Asnani
Ph.D. In Computer Science and Engineering @ Michigan State University
+ Read More
SUMMARY
+ Read More
Watch More
OpenAI's Greg Brockman: The Future of Large Language (LLMs) and Generative Models
Posted Oct 20, 2022 | Views 5.9K
# TransformX 2022
# Fireside Chat
# Natural Language Processing (NLP)
# Foundation Models
# Large Language Models (LLMs)
# Generative Models
Emad Mostaque (Stability AI): Democratizing AI, Stable Diffusion & Generative Models
Posted Oct 23, 2022 | Views 6.5K
# TransformX 2022
# Fireside Chat
What's Next for AI Systems & Language Models With Ilya Sutskever of OpenAI
Posted Oct 06, 2021 | Views 23K
# TransformX 2021
# Fireside Chat