A Framework to Assess Your AI/ML Maturity with Chu-Cheng Hsieh of Etsy
speaker
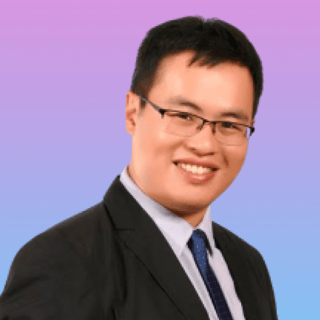
Chu-Cheng manages the data org across Etsy globally, including engineering, data science, and machine learning. Partnering with Etsy’s product and business executives, he develops the data strategy, represents data science, and drives high-impact decisions. He is specialized in search engine technology, recommendation systems, and machine learning technology. His primary responsibility is to deliver strategic and creative data science approaches that help achieve Etsy’s mission and goals. Chu-Cheng received PhD in computer science from UCLA. In his leisure time, he enjoys innovating and collaborating with academic researchers. He has brought cutting-edge research into products. He publishes papers in top-tier conferences and enjoys participating at a variety of academic or industrial conferences on information retrieval, recommendation systems, and data mining.
SUMMARY
Dr. Hsieh discusses how we should assess a company’s AI/ML maturity from zero to five from both a top-down (executive’s) perspective and a bottom-up (scientist’s) perspective.
TRANSCRIPT
Brad Porter: Our next speaker is Chu-Cheng Hsieh. Chu-Cheng is the Chief Data Officer at Etsy where he manages Etsy’s global data organization, including engineering, data science, and machine learning. Chu-Cheng was previously the head of Alexa voice recognition. He specializes in search engine recommendation systems and machine learning technology. He joins us today to provide us with a framework for assessing a company’s artificial intelligence and machine learning maturity.
Brad Porter: Chu-Cheng, welcome. Please take it away.
Chu-Cheng Hsieh: I’m the Chief Data Officer at Etsy. I’m very glad today to be here to talk to you all about how one person could assess the company’s AI machine learning maturity of another company. I’m going to present to you a five-level framework. In this five level framework, it will serve as a guide to help you to understand, if you are a data scientist, what kind of problem you are going to solve when you join a company. In the meantime, if you are an executive, I’m going to talk to you about how you can use the same framework, this five level framework, to measure the quality of a company you are thinking about acquiring, or to tell you what kind of scientist you should hire. Without further ado, let’s jump to these five frameworks.
Chu-Cheng Hsieh: In this five-level framework, I roughly describe the spectrum of AI machine learning into five stages. A lot of times when you are trying to build AI machine learning capability, you think about, “I want to do AI,” or, “I don’t want to do any AI.” The reality is that AI and machine learning is more like a progress, a spectrum, instead of an on and an off.
Chu-Cheng Hsieh: I describe these five stages using five different roles, from conductor, practitioner, craftsman, adventurist, to all the way to pioneers. I’m going to talk to you about each stage, and at the end, I’m going to tell you how you can quickly understand the stage of a company using three simple questions when you interview with a company, or when you are trying to talk and evaluate another companu. Let’s jump to level one, conductor stage.
Chu-Cheng Hsieh: In the conductor stage, products are powered by the AI machine learning service offered by other vendors. One could see a conductor as being a big orchestra. If you see each individual playing in an orchestra, that’s the service, then machine learning is one specialist in your team. How so? Let’s start with examples.
Chu-Cheng Hsieh: If you are going to build a product and you need AI/machine learning capability, like say, machine translations, you want to convert from English to Japanese. Certainly this is a solution, you can find many vendors that offer some kind of solution online. In this example, I just picked Microsoft translators. By using this translation service, your product will now be able to automatically support multiple different languages.
Chu-Cheng Hsieh: This kind of machine learning capabilities is exactly like your conductors. Most of the time, if you are a scientist that joins such a company, you have to know machine learning. You need to know the limitation of machine learning translation. You need to know the parameters that you are going to set up when you are using this kind of service. Just like when you are an engineer, you need the library to sort a list of numbers, you are using a sorting function which is written by someone else, but you have to know which sorting function fits your need. Is that a memory intensive? Is that CPU intensive? Is this computable? You need to understand all these limitations and different choices.
Chu-Cheng Hsieh: Machine learning is the same thing. You have to know machine learning to pick the right solution, to evaluate the different pros and cons of different solutions. Oftentimes when you join a company at this stage as a data scientist, you are actually a hybrid of engineer and scientist. New machine learning in technology is very critical in this stage. In the meantime, a lot of your time is working with engineers, or sometimes to be an engineer, to integrate these machine learning solutions. That should … what you expected if you are a data scientist.
Chu-Cheng Hsieh: How about if I’m an executive? When I talk about acquiring a company, what does that mean when acquiring a company with level one stage of machine learning maturities? That is exactly the ten acquisitions steps. A lot of time when you are trying to acquire a company, the easy way of thinking about machine learning capability at this stage is that machine learning is coming from third party vendors. Their main competitive advantage is the engineering talents, a group of engineers who actually know machine learning and know how to pick the right machine learning solution, and integrate these machine learning solutions into a product. That’s what you acquire for.
Chu-Cheng Hsieh: At this stage, if you acquired a company for AI machine learning, what you will actually get is a lot of engineering talents with a machine learning background, which can sometimes be a very good choice if you want to quickly integrate these vendors into your ecosystems. They will tell you about different vendors, there are pros and cons, and then provide you a quick boost on your talent pools. That is the stage one.
Chu-Cheng Hsieh: Now let’s move to level two practitioner. Practitioner stage products are powered by prevalent AI machine learning solutions. Often you can find a single solution in a book, or you can get this kind of solution on some training materials you can find in your online course. What do you mean by prevalent AI machine learning solutions? What I’m trying to refer to is that these solutions are pretty common. They’re using the same algorithm to solve different problems. I’m going to first talk about one problem that we have to solve at Etsy, to help you understand how practitioners play such a role in a lot of products.
Chu-Cheng Hsieh: At Etsy, a lot of items are one of the kind. They are handmade. Once they are sold, it’s gone. For items that only exist once in the whole world, you cannot show a review because apparently once it gets bought, then it is gone. The best alternative is to find a review for similar items of the seller, they look the same or similar to these items, but not exactly identical.
Chu-Cheng Hsieh: How do you find reviews that are similar to the item which is one of the kind? Currently, this is a machine learning problem to find a similar item, and then sort a review based on the similarity between the item you are going to sell on your website, and the previous review for an item that is sold in the past. In order to find such similar reviews, you have to build a machine learning model to compare the similarity between two items.
Chu-Cheng Hsieh: Currently there are a lot of things you can do. You can look at the picture. You can read the language. You can even analyze whether they come from the same producers. That is how machine learning can help us to do, is to read the tons of transaction history and identify items that are sold, and identify the most likely review which is relevant to the new item, which is one of the kind.
Chu-Cheng Hsieh: If you Google, you will find that there are many popular libraries, like this Vowpal Wabbit. They are cited to learn. They have tons of different libraries that people can find online. All this library, they produce the easy-to-access models or functions, allowing scientists to input data and build projections on top of this data you produce. At this stage, we often partner with a company like Scale AI, and because they can help us to give the labels, like when two items are similar, such that we can feed this data into our machine learning pipeline and build a projection service.
Chu-Cheng Hsieh: Unlike the stage one, machine learning translation is a popular practice you can find a lot of vendors. The things I just described to you, like finding most relevant reviews through history, is unique to Etsy. Currently, many companies also have this unique problem they have to solve, and that is, using stage two. You need to work out a solution from a library which … or a solution you can find on the textbook, but then you have to be creative and figure out a way how to translate your problem into a solution.
Chu-Cheng Hsieh: If you are a scientist joining such a company, you can assume that you are actually using a lot of machine learning libraries, and use them to solve business problems. In my examples, if you are a scientist, you need to first be creative, understand the problem is that every items only exist once, and your job is using natural] language processing, image understanding, or all this library you can find online and build a solution to predict what is the most relevant reviews to show.
Chu-Cheng Hsieh: This is your job if you join their companies. A lot of time, you are not going to like stage one to connect all the machine learning services. You will be responsible for identifying which data you are going to use to build a model. You need to work with a company to get label data in this example, like a total machine learning, which to review are relevant and why. Then eventually, you build machine learning on top of that. That will be what your day-to-day job looks like.
Chu-Cheng Hsieh: How about if you are an executive? If you acquire a company or you decide to work with the company that provides this level two stage of maturities, what you will often see is that the other company will have a lot of AI machine learning generalists. When I say generalist, it means they are actually an expert. That they are, to some extent, understand many, many machine learning solutions. They understand supervised learning and unsupervised learning. They understand different types of machine learning problems like a class three classification. They even can tell you the difference between, what is the tree model? What is the linear model? They’ve brought us understanding about machine learning, will be able to work with your engineer and product managers and understand and translate the problem into solutions, and then build up a pipeline to generalize these solutions in productions.
Chu-Cheng Hsieh: That is the person you are going to acquire, there are a lot of people who are, I often call them, they are applied scientists. They have a strong understanding of a broad spectrum of different machine learning, and they have patience to help the company to build a solution based on this data, and then help you to deliver value to your customers.
Chu-Cheng Hsieh: Now, we are going to talk about level three craftsmen. A craftsman stage product is powered by customized AI machine learning solutions, which often come from latest papers or conferences. What is level three and why is it very different from level two? If you think about level two, is something you can go to Ikea and buy the furniture. Then for three, what you want is talk to some specialist, and customize a solution to fit your unique need, and you want them to be the best you could find. Often for a company like Etsy, we want our solution to be not just unique, but also best in the class. That’s why level three is very critical for our success.
Chu-Cheng Hsieh: What does level three mean? Let me give you an example of how we get ideas and also how the solution was built. A lot of time, the breadth of what exists on a textbook, this solution is usually published at a conference, or they are reading papers that you can download from the internet, or there will be a lot of open source solutions that implement these papers.
Chu-Cheng Hsieh: Say we want to detect whether a review is positive or negative. Well, you certainly can be the level two stage solutions. You can find some popular library and solve that, but as you can imagine, the human language is very difficult to understand. In order to really do and understand the sentiment of the review, there are a lot of diverse new neural network solutions, or today, people call it deep learning, you can use to make a much better solution which can understand the unique need. A language written on an e-commerce website like Etsy would be very different from the language that you can find on a newspaper website.
Chu-Cheng Hsieh: Think of these examples, the solution, the breakthrough coming from the academic society, is actually a cornerstone of many solutions that you can find online. If you are a scientist joining these companies, what you actually do is that you actually read a lot of papers. You understand what is the latest state of the art solutions, and then use these technology breakthroughs to build a unique experience for customers.
Chu-Cheng Hsieh: Unlike level two, which is spending a lot of time understanding the problem, forming problem machine learning, and then solving that with a popular library you can download online. Then with three, you have to figure a way to convert a theory into a production line solution. If you are a scientist joining a level three company, you should expect that you have to read a lot of papers, and you should expect that you are going to build a lot of customized solutions that are unique to the company. Often they are even based on the latest technological breakthrough like a lot of deep learning solutions these days.
Chu-Cheng Hsieh: What does this mean for the executive? Unlike level two when you acquire a company, you usually acquire a lot of generalists. At level three when you acquire a company, you often acquire their technology. For example, if your company needs to hire a lot of people who understand fraud detection, then you should understand that the technology used to solve fraud and detect fraud is very different from image understanding. These are A and B, they are totally different. Of course, you can find the generalist of which you can find the solution to solve both problems easily, but the last problem also has their own unique perspective that people need to really understand the domain, and also understand the breakthrough in that domain to build a good solution in level three. Of course, one could argue level two and level three is generalist to specialist.
Chu-Cheng Hsieh: What I usually tell my peers and tell other CEOs, is that when I acquire a level three company, I always pay attention to the technology they have. Do they really have something that is very unique? They can bring a value that you cannot easily produce by general solutions? That’s quite often when you want to acquire a level three company, you pay attention to the domain they have specialties.
Chu-Cheng Hsieh: Now we are going to talk about level four, adventurist. At this stage, new companies should be the first mover who have experienced that power by AI machine learning. Solutions at this level usually are unique to your business and oftentimes, they are actually your secret sauce, is keeping your trade secrets, or if you decide to disclose that. It’s become a patent.
Chu-Cheng Hsieh: If you go to USPTO patent search, you can find that the many, many companies these days share their innovations first by patent, and then by papers. It is very important to protect your IP at this stage of the innovation because oftentimes, you are the first person to come up with a solution that hadn’t been solved before. This becomes a very strong, competitive advantage for technology companies because in a world like that, very often when you come up with some solution, someone is working on a similar problem. If you don’t patent your solutions and someone else submits the patent first, in the future, they will be a legal liability when you try to build a solution on top of your innovations.
Chu-Cheng Hsieh: At this stage, I often call this more a patent first, paper next, and product the last. Of course, this means that you are a scientist in such a company, you will produce a solution that is very unique, that is very innovative. In a sense that you will even be protected and sign an NDA and you cannot talk about your work. If you’re a data scientist joining such a company in level four, expect the solution you are going to build is a company confidential that you cannot share with your family and friends, because they become a trade secret of many companies at this stage.
Chu-Cheng Hsieh: What if you are an executive? What does it even mean to acquire a company at level four? First, level four doesn’t mean they are a very big company. They could be a spinoff from a school lab, a professor and a few students who created a company that publishes a lot of patents and papers. Even if they don’t have a concrete product, if you find that they have a solution that is really important to your future product, now you can acquire a level four company for intellectual properties. You acquire these IPs to protect your company, and also prevent other competitors from entering the space.
Chu-Cheng Hsieh: That’s the level four stage of AI machine learning. It doesn’t mean that the size of your team is the biggest …But it does mean that the focus is different. At this stage, whether something is immediately become a product is less critical than an originality and innovation over solutions. We are talking about the last stage in level five, pioneer stage. At this stage, your companies push the boundaries of innovation to the next levels. And you are often recognized as the leaders in a space, which you choose to become the best in the business. So what does pioneers mean? If you take a look at the recent machine learning conference, like ICML, one of the best conferences. You will see many researchers or chairs actually from industry. And how so? Because oftentimes the industry has tons of data and unique problems they have to solve. And at these stages of the companies, what they are trying to do is to make sure that they put enough resources to make sure that whenever there is a breakthrough, they will be the first to move at this stage.
Chu-Cheng Hsieh: Of course, a patent is still important at this stage, but that’s not the whole goal. The goal is to make sure that the company was perceived as the leader, and the best of the class among all the competitors. And you can see a lot of publications found in industrial companies these days in all these academic conference for these reasons. Oftentimes people working in these locations, or these offices classify themselves as researchers or a research scientist. So if you are starting to join a level five, like a group or level five companies, then you are mostly doing applied research. And remember that very few companies actually are on level five. They actually have solutions for one, two, three, four, all the way to five. And they usually create a lab, a research lab sometimes, for innovation there but depending on how you call it. But if you are a scientist joining this lab, you are actually doing very similar work.
Chu-Cheng Hsieh: Just like when you are up for 10 professors with one difference is that your research area is constrained by the field or the domain you choose to compete with. And you are expecting to publish papers, and your KPIs open how many papers you’ve published, and patents you’ve published, and the most important thing is how can you convert these innovations into a product. You’re a consultant for other companies, you’re a consultant for your groups. And this is very common especially for companies that are choosing to be technology leaders in the field. How about if you’re an executive, how does this mean to acquire level five companies?
Chu-Cheng Hsieh: First, level five companies are often a group or a department inside big companies and then could be sold separately, but most of the time, it’s very hard to acquire such a level five company. With one exception, sometimes the university will have a spinoff with a professor, who creates a small company who focuses on applying so they can research and build the stop. But suppose that you acquire an AI machine learning company at this stage. Which you actually try to acquire, it’s not just the technology and the people you acquired for their brand. There’s a Google expert who joined together and created a brand in the communities where you acquire such a company. Whether you are trying to compete, it’s the lecture that will be you using your brand to either bring business or to recruit. And that’s how executives should see if they are thinking about acquiring a level five company, and obviously level five company is not in the color.
Chu-Cheng Hsieh: So usually this is not a popular scenario where you will be encountered doing MMA discussions. Now you know the five stages framework, from one conductor all the way to five pioneers. Now most often the question I get from my mentees and other peers is, I don’t know how to tell, how can I tell whether companies are level one, two, three, four, and five. There are two important things to remember here. The first thing you should remember is if you’re talking about a stock, the property in one stage or in between two stages. But if you’re talking about big companies, depending on which team you join, it can be level one all the way to level five. So how can you tell? I will be showing you three powerful questions you can use. Number one, how do you interview your scientist? Your first introduction cores, your hiring managers ask these questions. If you are an executive, you are trying to acquire a company, ask their leaders. How do you interview your scientist?
Chu-Cheng Hsieh: If they focused on mathematical skill, research skill problem and solving skill, they are likely to be level three and above. If they focus more on engineering skills, they are likely to be level one to for three. So the number one thing you can roughly get, whether they are in the front or in the back, is by asking how they interview their scientists. How many technical interviews, what question will get asked in technical interviews. Then if you are a scientist after you’ve done all your interviews, you should roughly know which station they are. Number two, you can ask them, how do you measure your success in AI/ML? Again, if they say we really want to be a competitive advantage, it’s very important for us to come up with innovations, and then patent these innovations, how you got the keywords patent, you probably can guess they are level four and above. On the other hand, they say your success is based on the product you build, and we really want to build something that is very unique to the industry. Likely level three.
Chu-Cheng Hsieh: So by asking how they measure their success, you can roughly guess, which state they are. And my favorite is the third one, because if number one and number two is most subjective and trying to guess where they are, then number three is very powerful, but often difficult to answer is how are you going to define your KPIs? Apparently if your KPIs is the number of papers that you publish in a year, you shouldn’t expect that the row is at least the number level of four or level five. Because level four and level five innovation and sheer loss innovation is very critical. On the other hand, if your solution is mostly business within the matrix like revenues volumes, then likely it’s level two or level three. And if they say, Oh, we don’t have KPIs. I just need someone who knows machine learning and come to help me to use machine learning. It is possible they are level one. They need someone to understand how machine learning solutions can be used in their product.
Chu-Cheng Hsieh: And so let’s do a quick conclusion from level one to level five. At level one, your job is trying to coordinate different solutions. Level two, your job is to make sure you can scale a solution to productions. At level three, your job is to make sure you build customer solutions. At level four, they should make sure that your solution is one of the best, and you help the coming to be the first, the mover to deliver a solution. At level five, you are a pioneer, your job is to push the boundary of one domain. And that’s what I want to use, these five level framework to help me to assess the AI machine learning maturity of a company, or to be more precise, the part of the team you are going to work for. So thank you so much.
Chu-Cheng Hsieh: If you want to learn more of our current work in data science and machine learning, you can come to our website, dsml.etsy.com. I want to thank you all for joining the talk today. It has been a pleasure to share my learning with some of these five Stages, and I am looking forward to hearing questions from you. Thank you.