The Week in AI: Quantum ML, Graphcore’s Wow Factor, Machine Peripheral Vision, SEER 10B
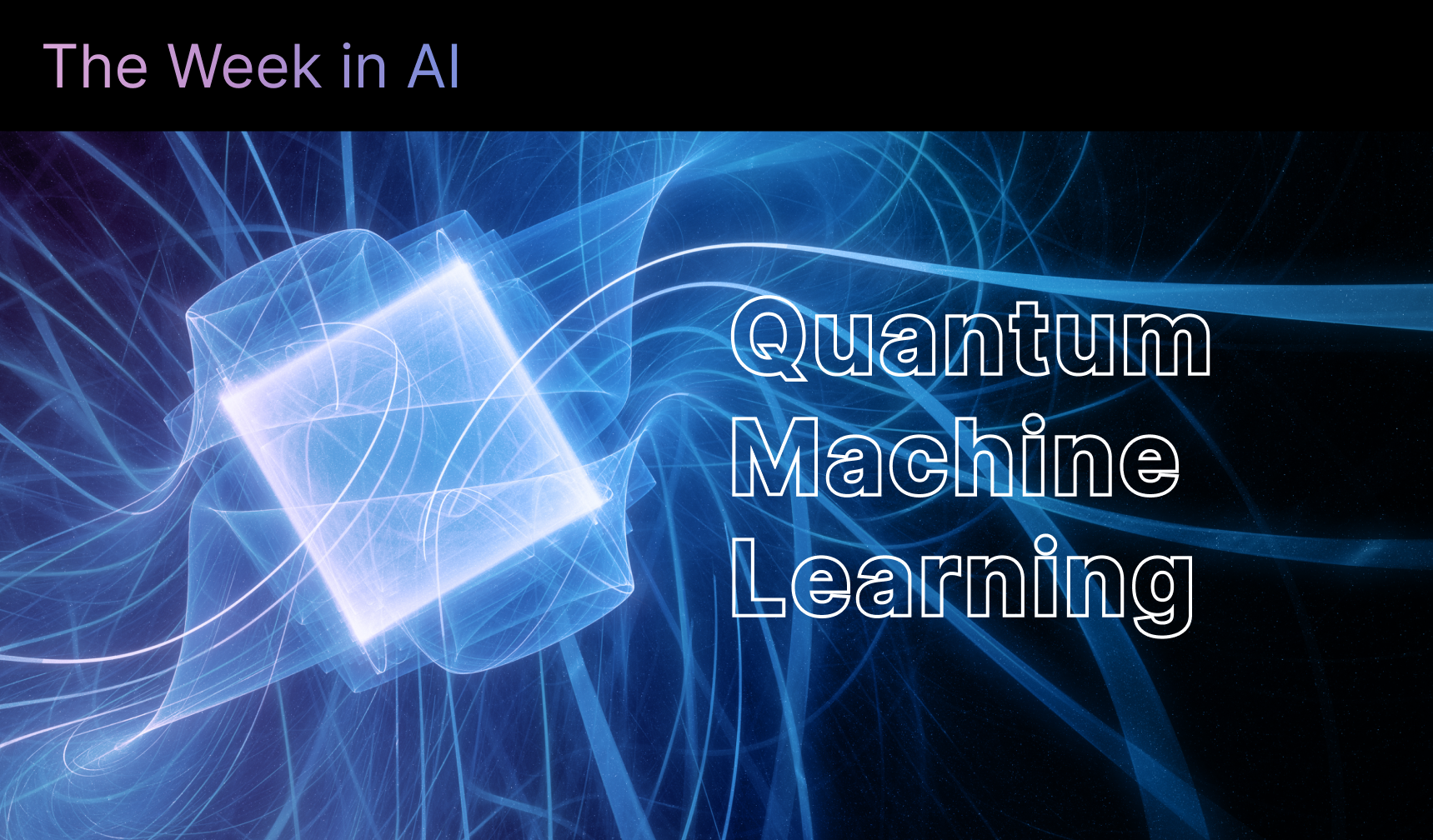
Learn about machine learning’s quantum speed-up, discover Graphcore’s AI superchip, look into peripheral vision to improve machines’ defensive abilities, and deep-dive into SEER’s journey toward fairness.

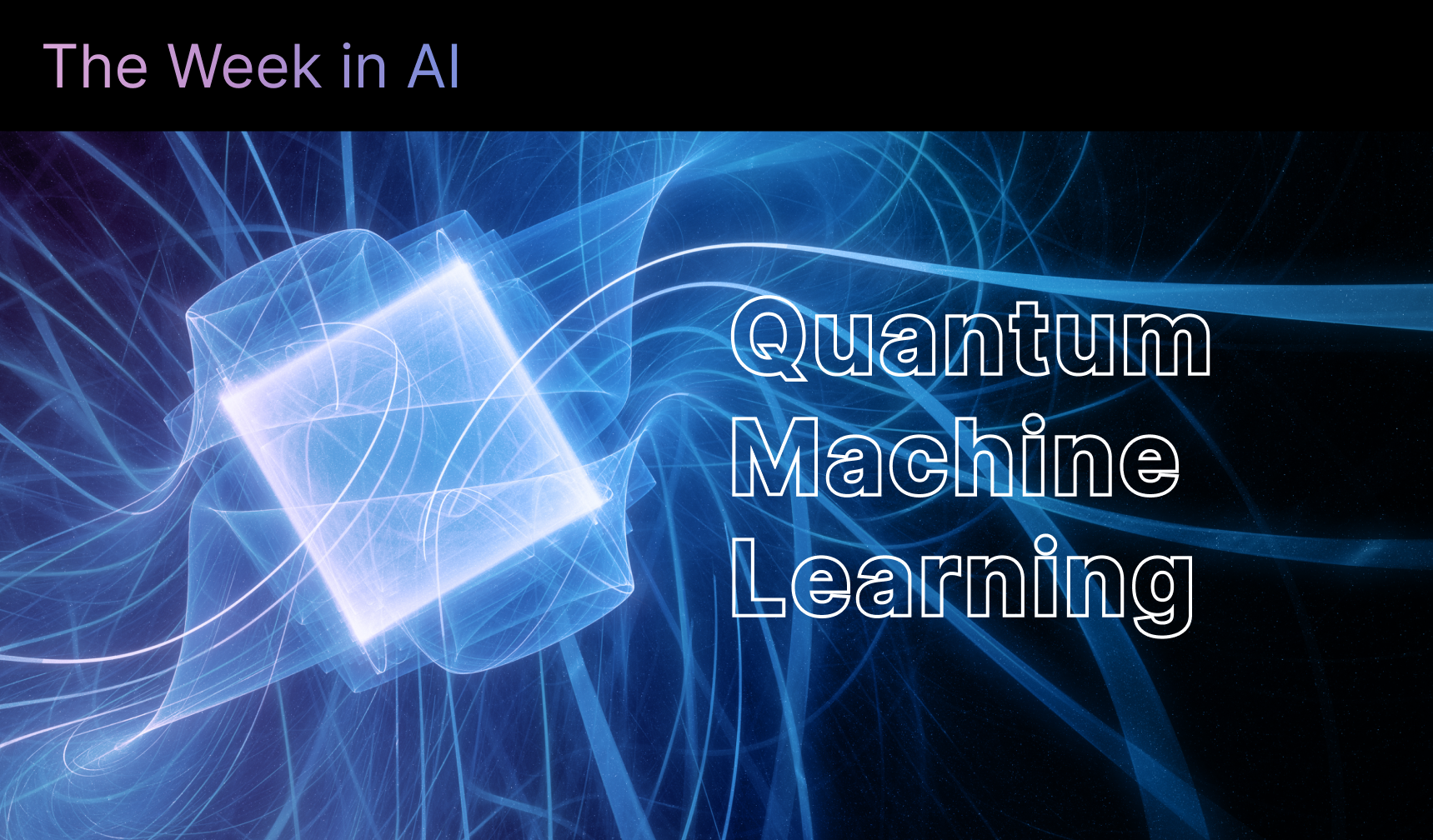
The Week in AI is a roundup of key AI/ML research and news to keep you informed in the age of high-tech velocity. This week, quantum physics outwits classical computing, AI powers up with the world’s first 3D wafer-on-wafer processor, adversarial attacks meet machine peripheral vision, and SEER aims to be better and fairer using 10 billion parameters.
Quantum Mechanics Approach Gives Machine Learning a Boost
Two teams from MIT and Sorbonne University set out to prove that machine learning can leverage quantum mechanics to solve problems faster than classical computers, bringing physics and computer science closer together.
The researchers ran multiple experiments in a quantum lab to demonstrate how a quantum search algorithm helps a computer navigate an optical “maze” more quickly and in fewer steps than a classical algorithm. By pairing classical reinforcement learning, which rewards right choices, with the time-saving power of quantum superpositions, the researchers proved that machine learning can benefit from the use of quantum computing.
Two recent studies, one demonstrating that quantum mechanics can help reinforcement learning agents learn faster and the other proving that quantum computers can learn to classify data in tasks that are infeasible for a classical computer, reveal a common point: Given the right circumstances, quantum machine learning can outmaneuver classical algorithms.
Reinforcement learning boils down to one question: How will the computer explore its possible choices? A classical computer can go through the options sequentially, but superpositions allow a quantum computer to amplify the promising paths. In the study, a tiny quantum computing processor learned about 63% faster than a classical computer. With the rise of proven benefits from quantum mechanics, researchers are now eager to see more experiments that highlight how quantum reinforcement learning can help with anything from chess and natural language algorithms to decoding brain signals in neural interfaces and personalizing complex treatment plans for cancer.
Graphcore’s Wow Factor and Its Road Map to an Ultra-Intelligence AI Supercomputer
The British semiconductor company Graphcore made two recent announcements that promise an exciting future for AI computing. First, it unveiled the Bow Intelligence Processing Unit (IPU), the world's first 3D wafer-on-wafer processor. The Bow IPU will power the next generation of Bow Pod AI computer systems, delivering up to 40% higher performance and 16% better power efficiency for real-world AI applications than its predecessors, all for the same price and without any changes to existing software.
Bow Pods set out to deliver real-world performance for a wide range of AI applications, including GPT and BERT for natural language processing, EfficientNet and ResNET for computer vision, graphing neural networks, and many more use cases. Graphcore worked closely with Taiwan Semiconductor Manufacturing Company (TSMC) to prepare the Bow IPU, which contains a 3D die enhanced by 900MB of in-processor memory.
Second, the AI and machine learning accelerator developer announced a road map to an ultra intelligence AI supercomputer destined to surpass the parametric capacity of the human brain. The GoodTM Computer is named after Jack Good, the computer scientist who first described such a computer in a 1965 paper. Notable capabilities include over 10 exaflops of AI floating-point compute, up to 4 petabytes of memory with bandwidth of over 10 PB/sec., and support for AI model sizes of 500 trillion parameters. It is clear that this ultra-intelligence machine is set to help AI innovators develop the next breakthrough in AI.
Machines Acquire Peripheral Vision to Deflect Adversarial Attacks
The MIT Center for Brains, Minds, and Machines and Lockheed Martin supported research that found similarities between how some computer vision (CV) systems process images and how humans see out the corners of their eyes. These types of CV models, called “adversarially robust models,” are designed to overcome subtle bits of noise that have been added to image data by perceiving visual representations in the same way that humans do using peripheral vision.
MIT researchers say little work has been done on the CV peripheral processing model. And because, like humans, CV systems use foveal vision to scrutinize highly detailed objects, these systems are vulnerable to or unaware of adversarial attacks, where malicious agents may subtly modify pixel images.
For example, an image affected by adversarial noise might look like a car to a human but be misclassified by a CV model as a cake. You can imagine how serious the implications are for an autonomous vehicle.
Researchers jumped on the opportunity to design a peripheral-processing ML model that can automatically learn visual representations that are sensitive to indistinct manipulations of image data. This work could also help shed some light on the little-understood goals of peripheral processing in humans. This research will be presented at the 2022 International Conference on Learning Representations.
SEER’s 10B Parameters Make It a Better, Fairer Computer Vision Model
Meta AI Research is publicly releasing its groundbreaking, self-supervised computer vision model, SEER, following the announcement of new advances that allow it to achieve better, fairer performance through learning on diverse datasets. SEER gained popularity when it proved that it can learn directly from any random collection of images on the Internet without the need for the data curation and labeling that go into conventional computer vision training, and then output an image embedding.
The model, formerly with 1 billion-dense parameters, has scaled to 10 billion-dense parameters in order to extract better-quality visual features and important information present in real world, large-scale datasets that have trillions of random, uncurated images worldwide.
Traditional computer vision systems are trained primarily on examples from the United States and wealthy countries in Europe, so they often don’t work well for images from other places with different socioeconomic characteristics. And because SEER does not rely on labeled datasets, it was trained on a set of examples that are much more geographically diverse than ImageNet. That’s why it delivered superior results for images all around the globe, including non-U.S. and non-European regions with a wide range of income levels, outperforming other models on important fairness benchmarks.
The self-supervised model can also handle challenging tasks such as classifying images contaminated by blur, camouflage, or unusual perspectives. A system that can accurately geo-localize images from across the world is a system that is on the right path to building trust with its users by meeting the requirements for privacy and fairness.
Why These Stories Matter
Quantum computers are not meant to get rid of classical computers, but to collaborate in tackling the hard-to-solve problems of our world, such as rapid drug discovery. Moreover, transistor innovations in computer chips give a glimpse into a post-Moore’s law world, accelerating new breakthroughs with AI. We still need to remain vigilant regarding adversarial attacks to prevent catastrophic incidents in real-world use cases. However, an enhanced focus on AI fairness and explainability is a step in the right direction. Until next time, stay informed and get involved!