Explore Online Debates with the DEBAGREEMENT Dataset
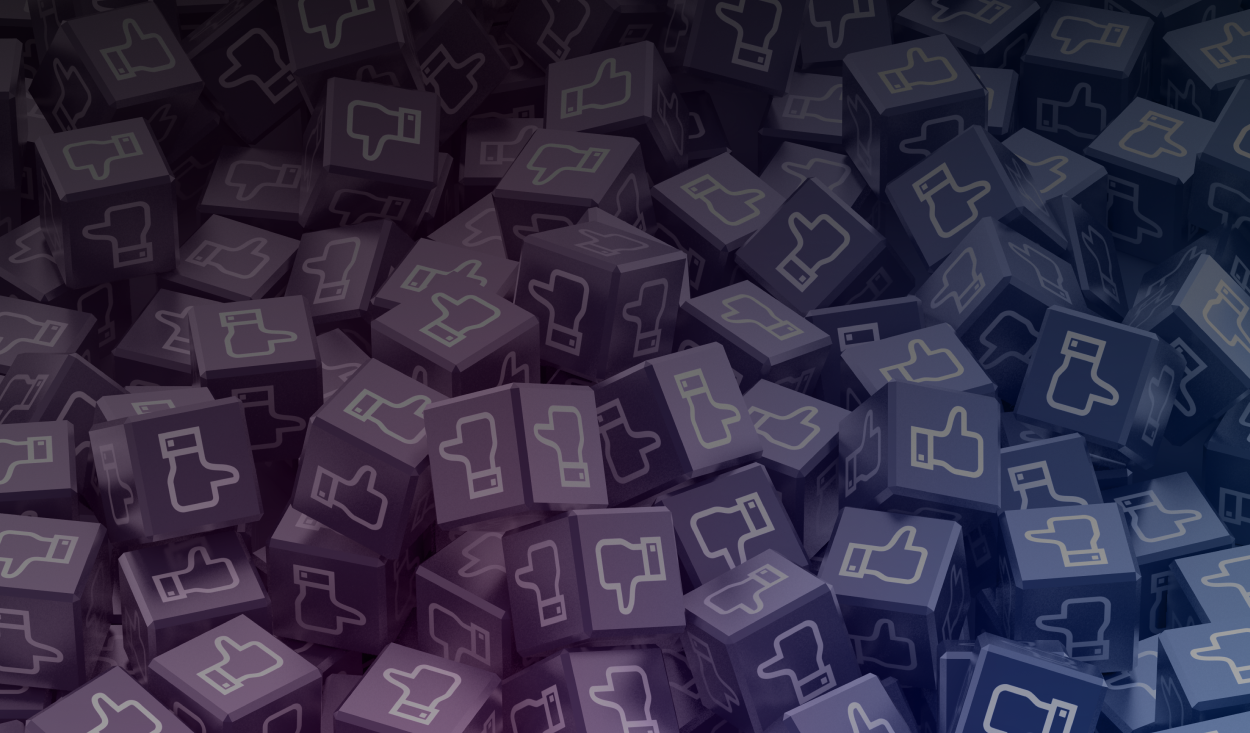
Here's how this new dataset can help with the exploration of online disagreement and polarization.

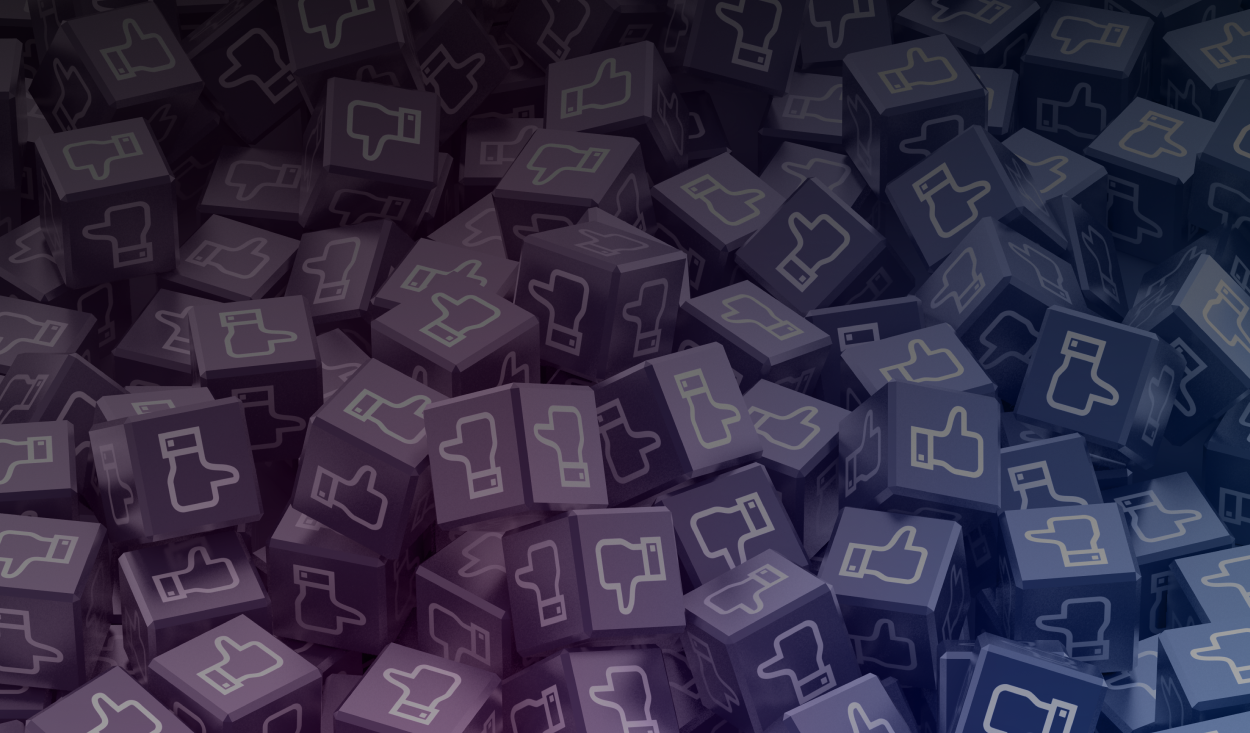
Social media sites have become the center of online communication—and polarization. By examining sentiment and disagreement in the posts on those sites, researchers can investigate sources of polarization online as well as how people express and change their views on social media over time. But identifying whether online commenters agree or disagree is easier said than done. Sites such as Reddit are filled with slang, sarcasm, and inside jokes that can be difficult for typical detection algorithms to parse.
To address the limitations of current stance-detection datasets, researchers at Scale AI, in partnership with Oxford University, built the open-source DEBAGREEMENT dataset, which contains 42,894 comment-reply pairings from a selection of Reddit forums, including r/BlackLivesMatter, r/Brexit, r/Climate, r/Democrats, and r/Republican. Each of those comment-reply pairs is labeled with “agree,” “neutral,” and “disagree” labels.
After creating the dataset, our team explored the performance of state-of-the-art language models on sentiment-detection tasks. Here are the key highlights of our study, DEBAGREEMENT: A comment-reply dataset for (disagreement) in online debates.
Models Trained on Existing Datasets Underperform on Real-World Data
When we evaluated the performance of four pretrained language models—BERT, RoBERTa, DeBERTa, and XLNet—on our DEBAGREEMENT dataset, we found that these models achieved an average accuracy of 62% to 64%. Models were generally more successful at identifying positive and negative sentiments than neutral interactions.
Because existing stance-detection datasets typically either focus on a single topic or contain formal discussions, we wanted to see if these interactions would map to the more informal discussions from Reddit.
To explore this, we trained the BERT model on the Perspectrum dataset, a formal detection dataset that focuses on structured conversations. This model achieved an accuracy of 90.5% on the Perspectrum data, but the BERT model’s accuracy fell to 57.7% when tested on the DEBAGREEMENT subreddits.
This sharp decrease in performance suggests that disagreement in online settings is a different problem compared to the more formal disagreements captured in existing stance-detection datasets. Messier data, such as that found in Reddit forums, requires a different approach for successful sentiment analysis.
DEBAGREEMENT Presents New Opportunities to Explore Online Interactions
Rather than analyzing the sentiment of individual online statements, DEBAGREEMENT uses a graph structure to describe the relationship between a comment and its reply. This structure allows researchers to model complex online interactions by incorporating both the text and its context into the models.
The dataset contains not only the text of the comment and its reply, but also the user IDs of each comment and its associated timestamp. By structuring data in this way, we can apply a combination of text-based machine learning and graph representation approaches to create more robust models that incorporate conversation context.
DEBAGREEMENT Allows Us to Explore User Interactions over Time
The DEBAGREEMENT dataset provides temporal and user-level data that can be useful for exploring interactions over time. Because comments and replies are linked as graphs, researchers can frame their analysis as a sign link prediction task.
With this approach, models incorporate the context between two comments when predicting the agreement or disagreement between them. These models can then generate more complex predictions of agreement or disagreement by considering past user comments and interactions as well as their timestamps.
Researchers can also use this temporal data to explore shifts in sentiment and polarization over time. Sentiment, especially in political spaces, is not static and is often affected by external events. By using the temporal information encoded for each of these comments, researchers can explore the shifts in sentiment over time.
For example, when we examined sentiment over time in r/Brexit, we saw changes in polarization in response to current events. As shown in the graph below, polarization in the forum increased after the initial draft withdrawal agreement was published and when Boris Johnson became prime minister.
With the temporal information included in the DEBAGREEMENT dataset, we can examine trends in polarization over time and even infer events that have sparked division.
Where Will DEBAGREEMENT Go from Here?
With the DEBAGREEMENT dataset, researchers can investigate how people’s views shift over time and explore sources of polarization online. There is still plenty of room to expand this dataset, however. Researchers may want to explore annotating interactions across full comment threads, rather than focusing on comment-to-comment interactions, or expand the dataset to include even more subreddits.
Using the existing dataset, researchers can also explore ways to build better stance-detection models for online communities. For example, they could train socially aware language models that produce better predictions by incorporating more contextual information. These models could include information such as the relationships between commenters across different threads to further enhance data annotation and modeling.
The ancient proverb “The enemy of my enemy is my friend” may be a useful line of reasoning when exploring agreement and disagreement in online communities.
Get Started with DEBAGREEMENT
Social media is a huge source of online communication, and the DEBAGREEMENT dataset provides researchers with the ability to explore trends in sentiment on platforms such as Reddit. Our study found that models trained on existing stance-detection datasets underperform on real-world social media data, and our DEBAGREEMENT dataset presents a potential solution to this problem. With this dataset, researchers can unlock opportunities to explore how users interact online.
For more information about how the researchers built and validated the DEBAGREEMENT dataset, watch Aerin's Tech Talk on DEBAGREEMENT on AI Exchange, and check out the full paper, DEBAGREEMENT: A comment-reply dataset for (disagreement) in online debates.