The Governance of Artificial Intelligence
speaker
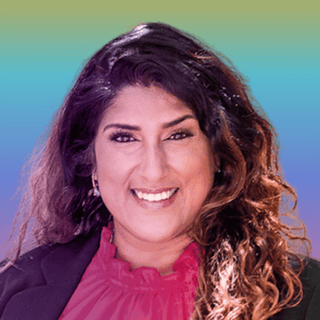
Navrina Singh is the Founder and CEO of Credo AI, a governance platform empowering enterprises to deliver Responsible AI. A technology leader with over 18+ years experience in Enterprise SaaS, AI and Mobile, Navrina has held multiple product and business leadership roles at Microsoft and Qualcomm. Navrina is a member of the U.S. Department of Commerce National Artificial Intelligence Advisory Committee (NAIAC), which advises the President and the National AI Initiative Office. Navrina is an executive board member of Mozilla guiding their trustworthy AI charter. Navrina is also a young global leader with the World Economic Forum and was on their future council for AI guiding policies & regulations in responsible AI. Navrina Holds a Masters in Electrical and Computer Engineering from University of Wisconsin-Madison, an MBA from University of Southern California and a Bachelors in Electronics and Telecommunication Engineering from India.
SUMMARY
Navrina Singh is the Founder & CEO of Credo AI which helps organizations build Artificial Intelligence with higher ethical standards. She discusses why AI governance is critical to the scaling of AI across and highlights the risks of not governing AI effectively. She shares how organizations can adopt AI governance practices effectively and continuously to build trust with internal and external stakeholders.
TRANSCRIPT
Nika Carlson (00:18): Next up, we're delighted to welcome Navrina Singh. Navrina is the founder and CEO of Credo AI, which helps organizations to build AI with high ethical standards. She is a technology leader with over 18 years of experience in enterprise SaaS, AI, and mobile. Navrina has held multiple product and business leadership roles at Microsoft and Qualcomm, and is an executive board member of Mozilla guiding their trustworthy AI charter. Navrina is also a young global leader with the World Economic Forum and was a member of their future council for AI, guiding policies and regulations in responsible AI. Navrina take it away.
Navrina Singh (01:01): Hello, everyone. So excited to be here at Scale's TransformX. Over the next 30 minutes, my hope is that I can convince you about the need for governance of artificial intelligence, especially why it is needed now. And why it is needed to ensure that you can scale your AI deployments effectively across multiple use cases, multiple regions. I'm Navrina Singh. I am the founder and CEO of Credo AI. We are an early-stage startup, which is bringing continuous and comprehensive governance and compliance of artificial intelligence to different enterprises ranging from financial services to high tech to government to HR technology and multiple other sectors. So I'm really looking forward to the next 30 minutes with you, where I think really diving in into what is AI governance, why the time is now, how you as an organization can start to adopt good governance practices, and more importantly, what is the benefits of doing this right now look like.
Navrina Singh (02:15): So let's dive in. I don't have to sell this audience on the importance or the pervasiveness of artificial intelligence. In the past decade, we've seen AI show up in pretty much every use case you can imagine whether it is in drug discovery, whether it's in hiring decision, facial recognition systems, supply chain optimization, and multitude of other use cases and industry. But I'm sure that all of you are thinking about the risks that these technologies are going to present to your enterprises as well as to your customers. So what we have seen in Credo AI over the past couple of years is when it comes to artificial intelligence, the unintended consequences of this technology can sort of span a spectrum of core areas.
Navrina Singh (03:05): So everything from system performance, is this system performing as expected? Is it trained on a representative data set? Have we ensured that there's no bias in the training dataset which might get propagated down the line? How is this unwanted bias potentially showing up in these AI systems? Is the machine learning application fair across different demographics? Is it easy to understand, not only by the executive stakeholders, the non-technical stakeholders, but by the regulators, the policymakers? Are you making the right disclosures to your consumers about how these algorithms are basically impacting their life?
Navrina Singh (03:49): Some of the consequences we are seeing is around adversarial attacks. Who tampered with your AI? How did they tamper with it? How you as an enterprise can be proactive about preventing those adversarial attacks from happening. And then at the end of the day, certainly thinking about not only the use of this technology but how it was built. And when you start thinking about accountability, who is responsible for when the systems don't perform as expected in the field and especially when they are in production for a long time and unfortunate incidents like pandemic happen, and your algorithm is completely making different predictions than what it was set out to do.
Navrina Singh (04:33): So, as you can imagine, all these unintended consequences are really bringing new category of risks to enterprises. Primarily, lot of companies that we work with, our focus extensively on increased regulatory scrutiny. Obviously, if you think about, the sectors, the highly regulated sectors like finance and banking, healthcare, government, there is a big question mark about when I'm bringing in a machine learning technology into my enterprise, or if I'm deploying it at scale, what are the things we have to be thinking about from compliance perspective? How should we be managing the risk and controls that we are putting in place?
Navrina Singh (05:17): And obviously there are established practices coming from governance risk and compliance space that a lot of these enterprises are adept at dealing with. But when it comes to machine learning, we are seeing new set of challenges emerge. As I mentioned, fairness being top of mind, how do you explain the outcomes? Who is accountable for it, et cetera? But as you dive deeper into these risks, one of the core risks that enterprises are grappling with, especially the ones that we are working with, there's a massive focus on potential reputational damage that these technologies can bring.
Navrina Singh (05:55): If last year is any indication of why accountability and oversight is needed now for machine learning, we've seen cases from facial recognition systems not performing as expected on the field all the way to the bias which is being built into underwriting models. All the way to bias showing up in algorithms which are data mining whether our kids are going to get accepted into a particular school. To advertisements and recommender systems really targeting and leaving out certain demographics. So as you can imagine this, we are at a very transformational point in the AI journey where these risks, if unmanaged not only regulatory risk and brand risk, if unmanaged could block a great opportunity that these artificial intelligence systems can bring to our walls and unlock $17 trillion in new market growth for different sectors. So as we speak to C-suite leaders in all these different sectors, the top of mind question right now is not only how can I manage, monitor, and mitigate the risk that artificial intelligence is potentially exposing my organization to.
Navrina Singh (07:17): But more importantly, how can I build trust? Not only with my employees, with my external stakeholders, with my customers, and internally with my board, and also with the regulators and policy makers. So in the past 17 months of building Credo AI are we've extensively worked across 120 partners, customers, certification bodies, regulators. And what has been fascinating to see is this emergence of AI governance as a core requirement to ensure that there is right oversight and accountability of the systems. Not just in development, but from the time you were actually thinking about building the system and deploying this at scale. So let's take a moment to really define what AI governance is because as you can imagine, there's been a lot of noise around responsible AI, trustworthy AI, ethical AI, ML Ops, AI governance. So I'm going to take the next couple of minutes really to define what AI governance means, especially for Credo AI and for our customers.
Navrina Singh (08:23): So AI governance really is a discipline to steer the development of machine learning technologies by providing continuous and comprehensive oversight and accountability to deliver responsible AI at scale. And I'm sure many of you attending today are thinking about responsibility and are thinking about trustworthiness of artificial intelligence technologies. The way we think about responsible AI within Credo is really putting a focus on, is it auditable? Is there a way to independently test these machine learning system? Can we not only understand what's happening from fairness perspective, but could we also present mechanisms to mitigate fairness and bias issues? Can we ensure that it is compliant to regulatory frameworks? And can we explain the outcomes? So as you can imagine, there's a spectrum of areas that come with managing accountability, as well as oversight of the systems. I won't spend too much time on this slide but wanted to give you a view into the AI-first organizations that we are working with.
Navrina Singh (09:35): On the AI maturity cycle, what we've seen in the past five years is organizations that have been built on AI, are deploying AI at scale. We basically call them AI-first organization. And then we have this massive middle, the back forward companies that are still experimenting and playing with artificial intelligence. And haven't got it to the scale that the AI-first companies have got. So the view that I'm sharing with you on this slide is really focused on those AI first enterprises that are not only building machine learning applications within their enterprise, but are actively purchasing ML systems and algorithms from third-party vendors. So if you think about such a setup, the top of mind for these enterprises is what does good look like in terms of standards when we have to manage risk? What are those standards and how we should be thinking about it?
Navrina Singh (10:33): How can we not only stay compliant to our internal policies, but also potentially existing regulations and new regulations that are showing up on the horizon? Very important. How can I provide social proof of good governance to my customers and clients so that we can unlock more sales opportunities, go through faster procurement cycles, unlock more new markets? And as you can imagine, scaling AI is now really dependent on how good you've done in terms of governing these technologies from end to end. And now this brings me to a really critical point here because in our very fast moving AI ecosystem, we've seen the emergence of a category called ML Ops. And in ML Ops, as you can imagine, there is a huge focus on how is the model management happening? How are you building these systems? How are they getting deployed?
Navrina Singh (11:33): How are you serving these models? And most of the ML Ops systems are really targeted for the technical stakeholders coming from data science, machine learning, engineers, and product functions. So one of the things we spend a lot of time on is really thinking about, how does AI governance really fit in this picture of ML Ops? But the most important thing that I think want to communicate here is AI governance is not ML Ops, and I'll share why, but also oversight and accountability within ML Ops is super critical. As you are thinking about bringing in different data sets for training your algorithms, to the kind of techniques that you might be using for building these models to how you're doing validation, certainly there's a huge component around how you bring that accountability. But AI governance is a layer that sits on top of ML Ops and integrates really well with it.
Navrina Singh (12:36): So to demonstrate that point, the way we think about AI governance is basically this multi-stakeholder alignment platform that is helping bridge that gap between the technical stakeholders and the oversight professionals coming from compliance, risk, audit, and other functions. As you can imagine with the breakneck speed of AI development, we've created this AI governance chasm, and it becomes really critical for us to bridge that gap for oversight so that we can build trust. So this layer that basically is creating a translation between the statistical view that ML Ops presents, obviously very, very conducive to the data scientists and the technical stakeholders. But how do you take that technical view, those statistical metrics, and translate that into business-relevant risk objectives as well as opportunity objectives? And then lastly, beyond this multi-stakeholder alignment, beyond this translation between technical and business objectives, how do you really integrate across the existing infrastructure that an enterprise has to ensure that you're staying relevant to the upcoming regulations as well as upcoming business rules that might be getting crafted?
Navrina Singh (14:02): So hopefully that should give you a sense into, ML Ops is a super critical category, really enabling the technical stakeholders. But AI governance is this great opportunity for companies to bring in multi-stakeholders, bringing in folks from risk oversight to exercise their expertise in putting the right guard rails around these technologies and enabling the technical stakeholders to bring in the right evidence as proof of these governance methodologies as well as frameworks that have been put out. I do want to spend a couple of minutes when we go and speak to our customers. We hear a lot about why they are not tackling AI governance right now. AI governance in some organization is an afterthought. And honestly, those organizations right now are finding themselves lagging in scaling AI quickly, as well as in a very compliant manner. So want to share some of the most cited reasons and then sort of be the Myth Buster here because why I think they are not true and those are just excuses.
Navrina Singh (15:14): So a lot of times when we go and speak to our customers and then folks who are just starting on this journey, they're thinking is we don't have to do governance of our machine learning technologies just yet because there's no regulation. And that is absolutely incorrect because there's already existing regulation. Whether you think about the financial space, SR 11-7, or whether you think about article seven in EEOC's domain talking about discrimination for hiring algorithms. There are already existing standards and regulations that actually do restrict how you can build and deploy machine learning systems at scale. And I'm sure all of you are following what's happening with the European commission's AI artificial intelligence act that is going to really inform the next wave of regulations and standards that we are going to see emerge.
Navrina Singh (16:14): Many of them talk about it's too soon and I don't think good governance is too soon. Good governance is good business. And we will share with you some of the metrics we are seeing across our customer base that is really critical. No one else is doing it is another excuse that we hear a lot. But again, the brands that are trying to build their differentiation using artificial intelligence, they're already starting to implement good governance practices, are starting to build in tools for good governance. It's interesting. We, as humans, tend to sometimes put responsibility on others. So we don't understand who is the accountable leader is an excuse that many enterprises give in not tackling AI governance, but this is a multi-stakeholder sport and really critical for all of us to get involved. And we can't afford it because as you can imagine, similar to cybersecurity, similar to good Dev Ops, there is costs associated with good governance.
Navrina Singh (17:16): But I think the framing that I would encourage all of you to think about is that you can't afford not to do it. You can't afford not to have good governance to scale AI. You can't afford not to do it right now to ensure that you don't run into reputational damage, that you don't run into regulatory scrutiny because your machine learning systems are not performing as expected. And then the last one we hear a lot is we don't know how, and let's dive a little bit deeper into it because this is where Credo AI comes in. A lot of time the how is dependent on there's a lack of gold standards and policies in what fairness means, as an example, in financial systems versus what fairness means in facial recognition. Who is defining it? And who's pushing for it?
Navrina Singh (18:00): Now, lack of compliant data. This is something that is top of mind for companies that if they need to really have comprehensive bias testing, they need access to protected attributes and at scale. So lack of compliant data for testing and assessment is a critical need that we hear across our customers. Not all the customers have the centers of excellence around AI. So large expertise gap in artificial intelligence and machine learning, especially across diverse stakeholders, is a big problematic area. And then lack of tools. And this is where we come in and I'll share a little bit more about what we are building at Credo. But currently, most of the organizations can't do good governance at scale because they don't have the tools for continuous governance. And lastly, misalignment of incentives. This is something that I've seen in my 20 years career building product across companies like Microsoft and Qualcomm. As technology stakeholders, we are incentivized to build the highest performing model deployed very quickly in market.
Navrina Singh (19:07): But our risk stakeholders coming from compliance and audit are incentivized to manage risk. And unfortunately, because of the misalignment of incentives and no glue that is filling that gap, many organizations are trying to figure out how do you do AI governance at scale. So over the next five minutes, I'll give you a quick view into Credo AI and what we are building, but we are industry's first multi-stakeholder platform that is enabling continuous and comprehensive governance of artificial intelligence technologies. And we do that not only where we help you understand the risk, monitor and manage it, but also help you build compliant AI systems across different regions, as well as across different use cases. So quickly just to walk you through how we've built our product and what we are excited about bringing to this market is really starting with trust. We've built these three layers of trust.
Navrina Singh (20:09): The first layer of trust is really enabling a multi-stakeholder alignment to ensure that all the ethical decisions you are making as an organization actually move into action through a comprehensive governance workflow. The second layer of trust, which is the trust with AI solutions and machine learning models. This is where not only we help you align on what the right metrics are, whether they should be precision or recall or false positive rate, et cetera, really aligning on what good looks like. And then enabling that through our ethical assessment modules to really help you manage risk from fairness risk all the way up to robustness, explainability, security, and other risks. And lastly, we are big believers that once you've deployed these systems in the environment, how do you ensure that they're going to stay compliant to the regulatory standards that are emerging? And this is where Credo AI has an extensibility platform that not only pulls the right regulations and standards within Credo AI, but we help you think through how your system is going to be performing. What are you monitoring for, for compliance and production environment and do that at scale.
Navrina Singh (21:27): So again, going back to scaling your AI systems and thinking intentionally about how you build a system with a compliant viewpoint, it's really critical to sort of land these three layers of trust, which is around people and processes, around your AI models, and around the environment that you're going to be deploying your machine learning systems in. As we think about, what are the benefits and why should I, as an enterprise, invest in AI governance now, some of the core benefits that we are seeing of people deploying Credo AI, but also just AI governance in general, being very intentional about building these machine learning systems. One is obviously increased return on investment on artificial intelligence. Where companies now, because they have more confidence in deployment because they can handle the risk as well as the compliance much more better, they're seeing this uptick in their machine learning systems actually yielding the outcomes for the organizations that they are setting out to do.
Navrina Singh (22:35): The other amazing benefit that we are seeing beyond risk management is unlocking sales. When you can build that trust with the customer, because you can show a proof of good governance or who provided oversight, what were the rules you were following, what were the standards you had set internally, what were the metrics you were looking at, how have you done testing across different vectors for fairness, for explainability, how are you disclosing outcomes to your customers, all that builds trust. And what we are seeing is that's really enabling unlock more key sales opportunities and faster procurement cycles. And obviously all this is possible because, with good governance, you can get more confidence in your AI deployments, which now are showing up in the market and all the different use cases that your company is operating and the different sectors your companies operate in. On the risk side, improve time to compliance.
Navrina Singh (23:30): As you can imagine right now, there's a lack of understanding in terms of what does compliance metric really look like for machine learning systems. And then through that alignment, what we are seeing, one of our customers spending $50 million on manual compliance checks of these machine learning systems. But because of Credo, they're able to not only improve and remove all the manual components, the tedious tasks, but really create a central repository of trust, which then lends to a very informed risk management. Now you can proactively tackle the brand risk, the financial risk, the regulatory risk, or in case of our government clients, the mission impact risks. And as you can imagine, that is a big value proposition.
Navrina Singh (24:15): So just in the closing, as we think about AI governance, we are really encouraging enterprises to start thinking about what can you do today? And especially to scale AI. Know where AI is being used within your enterprise. It is surprising that really having a comprehensive model repository is a big challenge for many companies. But knowing where you are actually using AI versus you're not using AI where maybe you're using old statistical methods and models is really critical for not only the executive stakeholder so that they can manage risks, but also for the technical stakeholders. So you can actually do a good inventory of where the risk priorities should be. And that brings us to the second, which is identifying and prioritizing high-risk AI use cases. In the customers that we are working in, whether it is retail side, recommender systems which have inherent bias, or underwriting models that potentially could not give you loan, or facial recognition systems which don't do a good job in recognizing Asian brown person like myself, it becomes really critical to figure out what are those high impact risk scenarios.
Navrina Singh (25:31): And once you have that model inventory, that's where it will become pertinent for you to start estimating that risk and then establishing accountability structures. Just like we've seen with the cybersecurity wave, the same thing that we saw with Cloud wave, companies are going to go through this change management with artificial intelligence. We are seeing new kind of rules emerge, whether it is chief AI officer, whether it is chief ethicist. But new accountability structures are getting created where it's going to become very clear in terms of how do you provide oversight and how do you make sure that the right people are involved. And lastly, to really make sure that your AI governance actually yields the outcomes that you are setting out to accomplish the need for continuous governance is super critical. It is not once and done snapshot of your compliant. But more importantly, once you've deployed these systems in the market, how do you ensure that in the production environment you're monitoring for the right metrics. Especially monitoring for the compliance changes that your production environment might be lending to your machine learning systems.
Navrina Singh (26:49): Just to close it out, we are big believers that AI governance is going to be the bedrock of building trust and managing risk. And right now the companies that are developing and scaling AI in a massive way are going to become the front runners for trusted brands in this AI evolution. And then the most important component of that is going to be AI governance done not only comprehensively, but also continuously. And would love to just share with you that we are at a very interesting point in this AI revolution, where to deliver responsible AI at scale, it is going to be critical for companies to take an early bet on governance and compliance of these systems. And if you have any questions, we'll be happy to reach out to Credo AI because we are on this mission to help organizations create AI with the highest ethical standards so you as an enterprise can deliver responsible AI at scale.
Navrina Singh (27:58): Well, thank you so much. And I really hope that today you got a good understanding of what AI governance is, why the time is now if you're thinking about either starting or scaling your artificial intelligence initiatives within your company, betting on AI governance is going to reap benefits as well as help you manage risks and help you establish as the next differentiated brand in the coming years. Well, thank you so much.