Opening Remarks
speaker
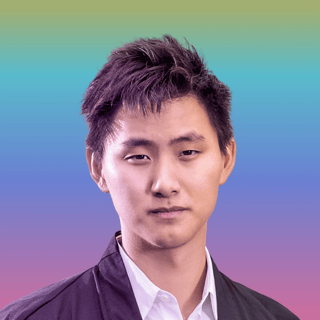
Alexandr Wang is the founder and CEO of Scale AI, the data platform accelerating the development of artificial intelligence. Alex founded Scale as a student at MIT at the age of 19 to help companies build long-term AI strategies with the right data and infrastructure. Under Alex's leadership, Scale has grown to a $7bn valuation serving hundreds of customers across industries from finance to e-commerce to U.S. government agencies.
SUMMARY
Opening Remarks with Alexandr Wang, CEO and Founder of Scale AI
TRANSCRIPT
Alexandr Wang: Thank you everyone for joining us today at our inaugural Scale Transform Conference. We have an incredible group of speakers who are going to speak to the opportunities and challenges for the future of AI. I couldn’t be more thankful for everyone who’s helped make this conference possible, and I hope you learn something new through our conversations today. As we reflect on the future of AI, the macro inputs are clear. We believe the next decade of machine learning will be defined by continued exponential scaling of compute, data, and algorithms. A consistent finding in machine learning research is that performance continues scaling seemingly infinitely with larger data sets, more compute, and larger models. Model performance increases logarithmically as a function of compute and dataset size. We can expect to see exponential growth in the amount of compute and data used to train state-of-the-art machine learning models this decade.
Industry is tackling the challenge of increasing compute needs head-on. There’s a boom of AI chip development, and experiments can be scaled up to massive compute sizes on public clouds. The recent Nvidia A100 chip is a strong example of this trend. Another interesting trend here is the parallels between new deep learning chips and the human brain. The new chips by Cerebras and Graphcore have interspersed compute and memory, which is more reflective of how the human brain actually works. It’s exciting to see hardware trends approach biological observations, as we continue to optimize machine learning workloads.
This leaves the creation of massive scale high-quality datasets as the largest remaining bottleneck. At Scale, our goal is to actualize Moore’s Law for annotation, so that data can grow in tandem with exponentially growing compute and model sizes. Recent research from OpenAI has shown how data set size model size and compute must be scaled in tandem for optimal performance. Through this research, we can actually predict exactly how much data we’ll need in the future as a function of compute and model sizes that are available. The short answer is, it’s a lot. There’s an incredible need and necessity for us as a community to figure out how to continue scaling our machine learning systems with larger data sets, and more efficient methods of annotation and human feedback. We’re excited to tackle these problems at Scale to ensure that we can all continue riding these exponential curves and scaling machine learning systems.
In machine learning, the evidence is clear: quantity has a quality of its own. We’re observing qualitatively new behaviors from similar algorithmic methods, simply by increasing the scale of compute and data. There’s a number of examples of this. It all started in the early 2012 experiments where neural networks finally started working once data finally scaled with the advent of the image net dataset. Later on today, we’ll be speaking with Fei-Fei Li on her seminal work in starting image net. GPD 3 wowed the world last year, where it demonstrated incredible results in few shot learning, generalization, and an impressive ability to distill human knowledge through text.
More recently, additional research from OpenAI has shown multimodal neurons, AKA Halle Berry neurons, emerging in clip training on 300 million image language pairs. These neurons show an ability for neural networks to learn complex concepts jointly in multiple domains, such as image and language. We’ll be speaking with Sam Altman of OpenAI later today on their breakthrough results. Lastly, Gabor-like filters emerging naturally from stochastic gradient descent in the early layers of convolutional neural networks, mirroring evidence we have of how the visual cortex works in mammalian brains from experiments as early as Hubel and Wiesel.
Overall, AI continues to surprise us with its new capabilities, primarily enabled through advancements in the core resources of data, compute, and algorithms. What types of new AI capabilities can we expect to observe when we reach the next orders of magnitude for data and compute? We may not be sure what these new emergent behaviors will be, but they will definitely exist, and they will be enabled by the next decade of data and compute scaling. Thank you everyone. I’m excited for our speakers to share their insights from the front lines of AI, and I hope you all enjoy the conference.