Human-Centered AI with Fei-Fei Li
speakers
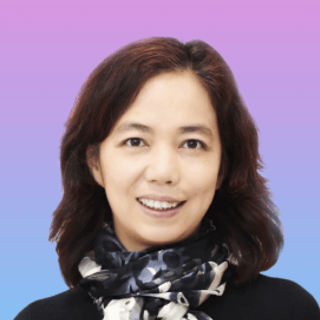
Dr. Fei-Fei Li is the Sequoia Professor of Computer Science at Stanford University and Denning Co-Director of the Stanford Institute for Human-Centered AI (HAI). Her research includes cognitively inspired AI, machine learning, deep learning, computer vision and AI+healthcare. Before co-founding HAI, she served as Director of Stanford’s AI Lab. During her Stanford sabbatical from 2017 - 2018, Dr. Li was a Vice President at Google and Chief Scientist of AI/ML at Google Cloud. Prior to joining Stanford, she was on faculty at Princeton University and University of Illinois Urbana-Champaign. Dr. Li is co-founder and chairperson of the national non-profit AI4ALL, which is increasing inclusion and diversity in AI education. She is an elected member of the National Academy of Engineering, among other distinctions. She holds a B.A. degree in physics from Princeton with High Honors, and a PhD degree in electrical engineering from California Institute of Technology.
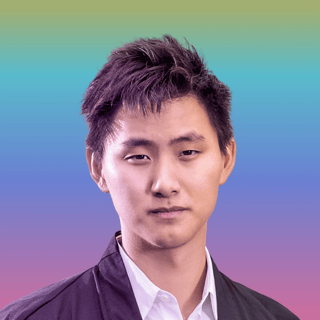
Alexandr Wang is the founder and CEO of Scale AI, the data platform accelerating the development of artificial intelligence. Alex founded Scale as a student at MIT at the age of 19 to help companies build long-term AI strategies with the right data and infrastructure. Under Alex's leadership, Scale has grown to a $7bn valuation serving hundreds of customers across industries from finance to e-commerce to U.S. government agencies.
SUMMARY
Dr. Fei-Fei Li discusses AI inspired by human intelligence and developed to augment human capabilities, and the importance of diversity in the field of AI.
TRANSCRIPT
Aerin Kim: I am pleased to introduce our final speaker for Transform, Dr. Fei-Fei Li. Dr.Li is a Professor of Computer Science at Stanford University and also a co-director of Stanford's Human Center AI Institute. Dr. Li's research interests include cognitively inspired AI, machine learning, deep learning, computer vision and AI healthcare, especially ambient intelligence systems for healthcare delivery. In the past, she has also worked on computational neuroscience.
Aerin Kim: Dr. Li is the inventor of ImageNet a large scale dataset that contains over 14 million labeled images that really kicked off the deep learning craze. On top of all these technical contributions, she is a national leading voice for advocating diversity and inclusion in tech. She's a co-founder and chairperson of the non-profit AI4All. Dr. Li, Thank you so much for joining us.
Alexandr Wang: I am very excited to have you and thank you so much for taking the time. We wanted to start off by just talking through some of your very recent work. And one of the things that you've been very involved in is standing up the Stanford Institute for Human Centered Artificial Intelligence. So, kind of just to start off, what do you mean by this?
Dr.Fei-Fei Li: Thank you, Alex. First of all, thanks for inviting me to this exciting event. And I have been so impressed by what Scale AI has been doing in the past few years. I feel like I watched you grow as a company since the beginning. So, about two years ago, my colleagues and I started Stanford Institute for Human-Centered AI. We just call HAI for a shorter name. And it was a really important thing that many of us wanted.
Dr.Fei-Fei Li: We started this in the end of 2018, beginning of 2019. And if you look back, we have hit a historical moment where AI as a technology was no longer a niche computer science discipline tucked in the corner of a computer science department. In a very, very short period of time, a matter of just less than a decade, it has grown into a full industrial force that is part of the fourth industrial revolution about to transform businesses and the human lives in every way possible.
Dr.Fei-Fei Li: And we're still on that journey, right? What you do, what we do as technologists. And it was really important for me that as the generation that brought AI into this transformative technology that we not only care about what the technology can do but really think through the angle of human lives and communities and society, because as every tool humanity has ever made in our civilization, it can be a double edged sword and that can bring tremendous hope, potential, productivity and improve human wellbeing, but it can harm people, communities, societies sometimes potentially intended if it's in the hands of bad actors, but a lot of times unintended consequences can truly bring harm.
Dr.Fei-Fei Li: So, in that framework, Stanford believes we really must reconsider this technology through a human centered lens and seize that historical opportunity and also frankly, responsibility to advocate for advancing AI research, education, policy, and outreach to better human conditions. So, that was the beginning and inception of Stanford's HAI.
Alexandr Wang: That's super, super exciting with HAI and the work that you all are doing. What are some of the research focuses of the institute?
Dr.Fei-Fei Li: That's a great question. We actually do not only research, but research, education and policy. We built all of our work around three major pillars or principles of human-centered AI. And there are the following three. First of all, just as we were saying, we recognize AI is no longer a niche computer science discipline, it's actually extremely interdisciplinary. So, we invite social scientists and humanists to come and conduct deep research with us to understand, forecast and hopefully guide the human impact of AI. This means we work with economists on the future of work, we work with legal scholars on AI and governance, we work with political scientists on international and national security of the technology, we work with ethicists and philosophers on the ethics of the technology and many, many more. So, it's an extremely human and society focused multidisciplinary approach.
Dr.Fei-Fei Li: So, that is the first principle we based our research on. And the second principle is that there is a verb in the public collective consciousness of AI and that is: replaced. You think about AI, you think about the truck drivers being replaced, the human workers being replaced, but we actually believe there's a different, much more important verb associated with AI and that's to augment, augment human capabilities and augment and enhance our humanity.
Dr.Fei-Fei Li: And here we do research for example, we'll probably talk later about in healthcare, how can we become assistive technology for our clinicians and patients? In education, how can we have a colleague who calls, how can we superpower our teachers to improve education? In manufacturing, how can we put workers outside of harm's way while helping everyone to increase productivity, but respect human dignity and well-being?
Dr.Fei-Fei Li: So, there are many opportunities for this technology to augment humanity. And that's the second focus of HAI's research and education. Last but not least, these are high hopes for AI as you and I know, while there's a lot of excitement for AI, the technology is still very nascent. There's a lot of unsolved problems. For example, again, back to healthcare, if you want AI to truly provide a kind of empathetic assistance to clinicians and patients, it has to have a nuanced understanding of human emotion, intention and be able to collaborate with humans, that requires the technology to be more flexible, to be more robust, to learn under conditions that doesn't have massive amount of labeled data and all this requires better technology that is more human-inspired.
Dr.Fei-Fei Li: So, we work with neuroscientists, psychologists and cognitive scientists to invent or develop the next generation of AI that is human-inspired. So, I think these three principles cover the beliefs we have for tomorrow's human-centered AI technologies.
Alexandr Wang: Well, I think that's super exciting. And I think the three topics you just discussed are some of the biggest topics as a community, the AI community needs to really address, thinking about the full societal impact of AI in particular. There's a lot of technologists who are very focused on the technology, but it will deeply impact our society in ways that you just mentioned like replacement versus augmentation or with improvements in the technology to enable these new use cases.
ImageNet and the Deep Learning Boom
Alexandr Wang: Well, I wanted to take a step back in time to talk through some of the very early beginnings of the recent deep learning boom, which you were very intimately a part of in the beginning with the ImageNet dataset. So, the first question is, what led you to originally develop the ImageNet dataset which really kicked off a lot of what we see today in terms of the deep learning revolution?
Dr.Fei-Fei Li: I cannot believe this, more than almost 15 years has passed since the inception of ImageNet. I think the time was back in 2006. And at that time I think two things were driving me and my students and collaborators to come up with the ImageNet dataset. One thing was seeking the North star of computer vision, what was the fundamental problem of computer vision and how can we solve it? And second is about a refreshed way of looking at the way we can solve the problem. So, about the North star, computer vision is as old as AI field itself. At that time it was about 50 years old. And as a field, we have tested a lot of different problems from image matching to stereo 3D reconstruction to a lot of exciting problems, but a neighboring field called cognitive neuroscience, especially psychologists studying human mind and human brain around the '70s and '80s and '90s in the last century really started to tell us there is one fundamental functionality of human brain for human visual intelligence that is so critical.
Dr.Fei-Fei Li: And as machine vision scientists, we ought to see it as one of the holy grails of visual intelligence. And that's our ability to recognize objects or everyday objects, thousands, and tens of thousands of it. Kids at age six can recognize tens of thousands of objects and can use it, manipulate it, communicate it. So, ImageNet was a product that recognized this is a fundamental holy grail, a North star of our field. And in order to make true progress built upon the previous work of many researchers, we wanted to create a much, much larger benchmark to push the field forward to seek this North star.
Dr.Fei-Fei Li: So, that's one aspect of what was inspiring me to work on the problem of object recognition, but the recognition of big data comes from a different angle is, how do we work on algorithms to recognize objects? My PhD was all about Bayesian machine learning, creating models that are highly expressive, to try to express an object. In my Ted talk, I talked about assembling cats with different geometric shapes, and that method required a lot of parameter tuning of the models. And it was very unsatisfying for me. And we run into machine learning problems like over-fitting.
Dr.Fei-Fei Li: So, it dawned on me and my students in a kind of epiphany moment in late 2006 that children don't learn it that way. Humans and children and animals, they have incredible visual intelligence, but they learn by just experiencing the world, keep seeing so many things, even though we don't articulate what we see, but our eyes are capturing that data constantly. And from a mathematical point of view, we were pondering at that time, if that kind of big data approach will unleash motor abilities in a way that we've never seen before.
Dr.Fei-Fei Li: It was a little bit of a leap of faith because the memories and chips of that time were so small that thinking about 15 million images or a million images was just unthinkable in 22,000 classes. But I guess, we had faith in Moore's Law, and we had faith in math. So, we just nevertheless started that project. And after three years, we put together the dataset and benchmark of ImageNet and ImageNet challenge and I guess the rest is history. In a way, our assumption was proven right. But it was a little bit of as many things in science, it was a leap of faith and a bet that we took.
Alexandr Wang: Yeah. I mean, this thing that you just described, which is this parallel improvement of dataset size, computational power, and the algorithms, that's really been the story of deep learning for the past decade plus. And I think that the willingness to take these leaps of faith on each front has been very critical to progress. And so, I'm really curious when I look back and think about the progress of deep learning, I think ImageNet was an incredibly important milestone because it enabled these methods that used a lot of data to actually happen and actually be researched on. You obviously were playing a very central role and watched it all happen in sort of, as it was all happening. What role do you think that ImageNet played in enabling the leap forward with deep learning in AI?
Dr.Fei-Fei Li: Well, that question, I almost feel we should let history answer itself. It's hard for me to assess and claim a role, but like I said, I think our faith at that time was first believing in the North star. We need to seek, establishing that problem of object recognition. I think even to this day, there are many problems, important problems to solve in computer vision and machine learning, or if you look at natural language processing, but identifying that important problem of large-scale object recognition built upon the research by cognitive scientists was an important North star that we established.
Dr.Fei-Fei Li: Again, I don't claim credit all to myself. There were generations of work in that, but ImageNet as the largest dataset benchmarking that important problem put a stake in the ground and called the field to solve that important problem. Second is the attention to data. I think, prior to ImageNet a lot of machine learning efforts in algorithm was much smaller scale algorithms with the kind of almost manual parameter tweaking and ImageNet unleash the power of high capacity models like a neural network, which again has been around for 30, 40 years and to the heroic effort of Geoff Hinton and his colleagues they also maintain the faith.
Dr.Fei-Fei Li: It's a very powerful algorithm, but it was lacking data and it was lacking the computing chips to enable that kind of powerful high capacity models. So, I think together ImageNet played a fairly big role in rejuvenating the importance of neural network, the family of algorithms.
Importance of Datasets
Alexandr Wang: It's really incredible, data has played this really large part in the development of AI, particularly over the past decade. One question is, from today looking forward, how much investment do you think that organizations today such as research institutes or enterprises should be making in developing datasets?
Dr.Fei-Fei Li: Very important. I think datasets are here to stay. But I also think datasets are a means to an end. Maybe I speak more like a scientist, for me, my own scientific quest are the North star problems of a field in this case, visual intelligence and AI. And datasets play a huge role in terms of establishing the problems as well as giving the fuel for algorithms. In the business world, I also think datasets are a means to an end because the business world needs to answer the needs of customers and hopefully human-centered needs of customers and communities. We want to solve pain points, for example, in medicine, like helping radiologists to rapidly triage patients is the actual goal, but creating critical datasets to train algorithms of x-ray scans or CT scans that can improve the speed and reduce, let's say a false alarms or misses of the decision-making of radiology assessment is important so that means datasets play a huge role.
Dr.Fei-Fei Li: So, I do think datasets are a critical part of this AI revolution. But it's important to also remember what roles and goals they serve. And also one thing to point out if we keep in mind this, it also helps us to think about mitigating potential unintended consequences of datasets such as bias because the goal is not dataset, the goal is serving people well, and we do not want to introduce unintended adverse information into datasets.
Alexandr Wang: Actually, I was just about to ask you about that. Datasets and data bring interesting questions about bias, unfairness in machine learning, and this is a very important research topic for many organizations today. What do you think we as technologists can do to ensure that AI has positive benefits and no unintended consequences like you had mentioned?
Dr.Fei-Fei Li: I think this is exactly the goal of Stanford HAI. These are complex problems and issues, as we create technologies as a species, as a civilization, we are constantly learning. So, in this case, like you said there's so much awareness now already about technology's unintended consequences, especially if we focus on data, we can focus on the bias problem. So, there are many things I think, as technologists we should do. First of all, the awareness, we are trained as computer scientists and engineers and it's not in the traditional curriculum of our students and our engineering and computer science students to learn about the ethics and fairness issues of data and algorithms, but this is rapidly changing. So, here at Stanford, we are in a course of embedding ethics into as many computer science courses as possible.
Dr.Fei-Fei Li: Our colleagues at Harvard had done this starting a few years ago, led by professor Barbara Gross. And we have incredible classes at Stanford, such as the one led by Professor Rob Reesh, Mehran Sahami and Jeremy Weinstein on tech and ethics. So, education is a huge part of it. It raises awareness and the crazier generation of technologists who have that bilingual capability to think tech, but think ethics. Now, we also need to invest in the algorithm development and data development technology itself to debias or to avoid bias. And there, we have seen a lot of creative work coming up around the research community to look at how to assess data bias, to debias data, to debias algorithms, to debias decision-making and inference. And I think we need to continue to assess that, oh, sorry, invest in that. Also continue to advocate the interdisciplinary inclusion of ethicists and philosophers into the design of the algorithm.
Dr.Fei-Fei Li: At Stanford in my own healthcare team, we have ethicists to sit in the same room literally and Zoom wise when we develop our algorithm, because we need that voice from the design time. Last but not the least is the governance piece. We need much deeper research on how data governance and algorithm governance can be implemented, actually can be formulated and implemented, and also inspected. So, we have, for example, at Stanford HAI, we have legal scholars studying how laws can be modernized or re-thought in the light of algorithms making decisions? And how the laws can be applied to better governance. And also how to put in guardrails should there be an FDA of AI algorithms?
Dr.Fei-Fei Li: This is a public debate that's unfolding right now. And how do we mitigate the risk of algorithm decision making? Who is accountable? Who's responsible for the decisions? What does it mean to be transparent and explainable when we apply these algorithms? So, the governance issue is another piece that is critically needed to summarize education, research investment, and governance.
North Stars of AI Research Today
Alexandr Wang: Yeah, I couldn't agree more. I mean, what you described as this bilingual multidisciplinary understanding is absolutely critical. We need people who understand the technology deeply, as well as understand issues around policy or ethics or laws or these sort of governing decisions to be able to make as a society, the right decisions. So, you referenced earlier this idea of North stars in research, and you spoke about some of the North stars in building the ImageNet dataset, which they proved to be very true and almost precious looking back on them today. What do you think of are some of the North stars in AI research that you're really focused on today that you think are more researchers should really view as sort of important directional mile markers?
Dr.Fei-Fei Li: So first of all, I think AI has flowered in a way that I could not have predicted. So, 15, 20 years ago it was a much narrower field, whether we're talking about computer vision or AI at large. So, it was actually, I felt lucky. We were able to identify a major North star and fast forward to today the field is so vast and the opportunities are so broad. I really do agree with you that we need pretty large plural to even describe the potential. For example, in an unrelated field that I don't know much about, which is natural language processing, look at the large language models, right? It has brought tremendous progress in NLP in a way we could not have imagined a couple of years ago.
Dr.Fei-Fei Li: Of course, it also brings ethical questions, but that is a highly vibrant area of research, bringing home closer to my own interest. Here's what I have come to realize. Much of my earlier career, I was looking at vision as if I was a bystander. Even if assuming the child's angle of looking at the visual world, I'm still standing there watching the field, we're labeling objects. We're trying to understand, tell a story of the thing as if we are a third party looking from outside.
Dr.Fei-Fei Li: But the real excitement, in my opinion, in vision and intelligence is the immersion and the embodiment is that animal kingdom developed more and more intelligent species because we're constantly part of it, a part of the world that we need to survive, we need to seek food, we need to hide away from predators, we need to communicate, socialize by the time it becomes human, that we use our intelligence, including visual intelligence in everything we do.
Dr.Fei-Fei Li: So, from that point of view my only interest had turned from a more passive static way of looking at visual intelligence to a much more embodied and active way of looking at visual intelligence. And I'm super excited by the much more intimate interaction between robotics vision and just learning agents. So, whether it's through simulated virtual environments or actual robots, I feel like I'm restarting a PhD and focusing my research in embodied agents and combining visual intelligence with planning and learning, and to just study how complex generalizable, multitask learning agents emerge from interacting with the real world. So, that's one of my personal North stars recently that keeps me very excited, especially feeling like redoing a PhD and learning so many new things.
Human-in-the-Loop and Embodied Intelligence
Alexandr Wang: Yeah.100%. And one of the things that's very interesting about this idea of embodied intelligence is the evolution in how these algorithms learn. And one simple example that we think about a lot at Scale is what is the evolution of human feedback to the algorithms? In the current paradigm data annotation is as you mentioned, a relatively static or third-party way to provide feedback to the algorithms, but as we know, humans learn in a much more dynamic way from other humans.
Alexandr Wang: And so, what do you think about the evolution and what are some paradigms that you're excited about in terms of how algorithms can learn from humans in more dynamic ways?
Dr.Fei-Fei Li: I think humans in the loop and constant collaborative learning is definitely something that's receiving attention and very interesting. We had a recent project in our own lab, looking at how we can engage humans in active conversation with an AI agent who is trying to learn visual concepts. But again we let the agent be much more proactive in seeking meaningful conversations with humans so that the agent is learning what it wants to learn rather than being very passive and a researcher designing the concept space.
Dr.Fei-Fei Li: But that poses a new problem of how to engage with a human? And what kind of conversation can you have? And also eventually the research will go into how could you help a human accomplish the task? How do you dynamically update the learning dataset? And your knowledge base, so that both of the human and agent can both engage in a win-win collaboration? There are definitely emerging ideas like reinforcement learning algorithms with engagement rewards and all that. So, it's not of course, active learning, online learning. All those are very interesting areas of research.
Alexandr Wang: And one of the things that has been super interesting for me to read about from the outside is at the Stanford Vision Lab and Stanford HAI, the researchers have always been very inspired by cognitive science and an understanding of how humans learn. And I'm curious, you've always been super engaged in this multidisciplinary combination of the fields. What do you think in the next few years are some concepts from cognitive science or neuroscience that you think will be very applicable or interesting to see how they develop in AI?
Dr.Fei-Fei Li: We actually see it as a two-way road that AI definitely can play a huge role in neuroscience and cognitive science as a tool. But in the meantime every time I look into the brain I just find myself in awe, “How did nature create this incredible machinery that is just, like less than a half a kilo of weight, burns about 20 to 40 watts when it works, yet has this incredible capacity of learning and creativity and empathy and compassion?” And that just continues to just put me in such a wondrous world and there's so much to learn, for example, on the robustness and energy side. How do we create algorithms that require so little energy, and yet be so flexible and robust in learning?
Dr.Fei-Fei Li: How do we get inspired by cognitive science and especially developmental science and see how children through exploration and curiosity acquire the kind of world knowledge and the skills that carries them for life of complex tasks? Is that something we can translate into machines? Or down the road like I said, our technology needs to become more empathetic in order to collaborate with humans well. Then I don't even know where to begin to think about the mathematical formulation of empathy and that we could instill in machines. So, there's so much more research in terms of cognitive science, as well as AI to figure that piece out. So, there's so much more to be done down the road.
Diversity in the Field of AI
Alexandr Wang: That's so cool. I wanted to change topics a little bit to something that I know you're very passionate about, which is the topic of diversity in AI. Back in 2015, you co-founded AI4All with Dr. Olga Russakovsky. What was the thinking behind creating this non-profit?
Dr.Fei-Fei Li: This month, March is actually our fourth year birthday because 2015, we had a different name and then 2017 we changed it to AI4All, but anyway, I've been doing this for six plus years. So, what was the thinking? Well, the thinking, it was an interesting one around 2014 with AlphaGo, that really energized the world about AI and also self driving cars was no longer a scifi dream it's really being industrialized. And the world, the public conversation of AI was heating up and I was still Stanford AI Lab Director, at that time, we didn't have HAI Institute yet.
Dr.Fei-Fei Li: So, I'm starting to hear more and more a public concern about the Machine Overlords coming, the Terminator Next Door, and this extreme warning of this technology, which is fair, right? With a powerful technology like that, there should be this kind of warning, but that crisis talk was juxtapositioned with another crisis that is much more real, but yet much more silent in my world and that crisis, the lack of diversity and representation, I looked around in 2014, I was the only woman faculty in Stanford AI Lab of almost two dozen researcher faculty.
Dr.Fei-Fei Li: And if you look at our undergrads, our graduate students, our young generation of faculty and scholars, the percentage of women was constantly below 15%. And the percentage of underrepresented racial minorities was way worse and still worse. So, how do I reconcile this two crises in my world? I was thinking, and it really dawned on me. They're actually deeply connected if we as a society are going to worry about what the technology is going to bring us, is it a machine overlord or it's a Baymax, a benevolent robot, we should be much more worried about who's creating this technology. Who is going to lead the research, the development, the business, the deployment?
Dr.Fei-Fei Li: So, the real connection of these two crises to me was, who is at the steering wheel of tomorrow's AI? And luckily my former student Olga, who was finishing her last year of PhD also was thinking about that. She walked into my office one day, late 2014, and started talking about her desire of increased inclusion and diversity in AI. So we just hit off like, it was meant to be that moment. So, we invited also another educator at Stanford called Dr. Rick Summer, and three of us spent, I think the week before Christmas in 2014, talking about building up a program at Stanford that invites high schoolers to join our AI study and research in the summer, but really show them this technology through a human lens, it's human mission and inspire the diverse group of students to come join us in AI because these are extremely talented students. They can go to other fields, nothing wrong with the other field, but we need them in AI.
Dr.Fei-Fei Li: So, that was really the inception of AI4All our motto was still is AI will change the world who will change AI? And that's truly what I believe. I believe that by creating tomorrow's generation of diverse AI leaders, we have a much better chance of creating the kind of human centered technology we want and to avoid the so-called machine overlord terminator scenario that will get us into without the diverse leadership.
Alexandr Wang: Yeah. It's such an important issue and definitely one that I think has really been heightened as AI has become more and more important. It matters so much more exactly, as you're saying, who's developing this technology? A question that I have and I think a question that everyone in the community likely is what can we all be doing to help? So, what would you say to all members of the AI community? What are the ways in which we can all help?
Dr.Fei-Fei Li: Just even raising the awareness of this issue is an important first step, especially for impactful business leaders like you. So, I think starting from awareness, I think we have simultaneously multiple fronts we need to work on. We definitely have a pipeline issue, from K-12 education, how do we include underrepresented and underserved communities is a big thing. And that's what AI4All is focusing on. We want to put a lot more focus on our gender and racial minority on our first generation for high school, zero generation college students, family, low income family, rural family, deep south, center of America.
Dr.Fei-Fei Li: This is building that pipeline and encouraging that diversity, but there's also a culture issue. Once we invite these students to join the field of AI, to study in the computer science department, to intern in technology companies, to become a part of the workforce, how do we ensure the culture is becoming more and more welcoming and inclusive in the workforce, especially for a business leader?
Dr.Fei-Fei Li: I think that I would definitely appreciate and encourage you to think about within your company and in your community, how do we ensure that inclusion and also work with the civil society and government to incentivize those kinds of programs, whether it's by helping education programs or calling out the importance of culture in the workforce environment. And just from all angles create a new way of advocating for this issue.
Alexandr Wang: I think it's going to be a perennial issue. There will never be a point at which we're going to say, “This is not important.” Because like you're saying, we all need to be a part of making sure that AI is developed by a representative and diverse group of people and thoughts. So, thank you so much for taking on that initiative and creating AI for All. It's so important.
Dr.Fei-Fei Li: Thank you. It's everybody's effort. We're definitely part of this. Yeah.
Alexandr Wang: All the work you're doing across healthcare, diversity in AI and around human-centered artificial intelligence are so incredibly impactful, so important. And thank you again for all the work that you're doing.
Dr.Fei-Fei Li: Thank you, Alex. This is fun and good luck to the rest of the program and good luck to your business.
Alexandr Wang: Thank you so much.