Autonomous Delivery: Overcoming the Technical Challenges with Jiajun Zhu of Nuro
speaker
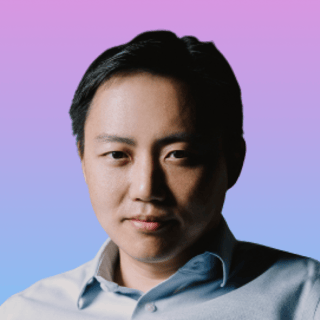
Jiajun Zhu is the CEO and co-founder of Nuro. Prior to founding Nuro, Jiajun was a principal software engineer of Google and one of the founding team members of the company’s self-driving car project (now known as Waymo). In addition to leading the project’s perception team, he also helped build and lead their simulation efforts. Jiajun earned both his Master's and Bachelor’s degrees in computer science from the University of Virginia and Fudan University respectively.
SUMMARY
Jiajun Zhu is the CEO and Co-Founder of Nuro. Prior to founding Nuro, Jiajun was a principal software engineer of Google and one of the founding team members of the company’s self-driving car project (now known as Waymo). He joins Scale AI CEO, Alexandr Wang, in a fireside chat to discuss the technical challenges of building autonomous delivery vehicles at scale. Together Jaijun and Alexandr explore why it's vital for society to automate the nearly 100 billion personal shopping trips we make each year in the US. Jaijun shares the key reasons autonomous goods delivery represents 'one of the hardest engineering challenges in history'. In particular, what are the challenges in managing data to optimize the learning process? How do you optimize for the 'long tail' of edge cases rather than solving the most common driving scenarios? How do you build a sensor strategy in conjunction with an AI strategy? Join this session to hear key lessons learned from the first autonomous vehicle company licensed to operate a driverless delivery service in the state of California.
TRANSCRIPT
Alexandr Wang (00:22): I'm excited to welcome our next speaker Jiajun Zhu. Jiajun is the CEO and co founder of Nuro. Prior to founding Nuro, Jiajun was a principal software engineer of Google and one of the founding team members of the company's self driving car project, now known as Waymo. In addition to leading the projects perception team, he also helped build and lead their simulation app.
Alexandr Wang (00:45): Jiajun received both his master's and bachelor's degrees in computer science and from the University of Virginia and Fudan University respectively. Welcome Jiajun, excited to have you here.
Jiajun Zhu (00:56): Thank you, Alex. Thank you for having me.
Alexandr Wang (00:59): To start out with, for audience members who may not be familiar with Nuro, you all produce autonomous vehicles, specifically designed for delivery. Can you share a little bit about why even started in Nuro in the first place and why you chose this particular use case to start out with?
Jiajun Zhu (01:17): Alright, Nuro is a robotics company. Our mission is to better everyday life through robotics. So my co founder and I, Dave and I, we actually spend a lot of time, most of our career on robotics and self driving before starting Nuro. I was one of the founding member of the Google self driving car project. And Dave was a planning lead on the CMU team in the DARPA Urban Challenge. And then he and I worked together very closely at Google before we started in Nuro.
Jiajun Zhu (01:52): So we both had this vision that in the next 10, 15 years, we really believe that robotics, especially truly autonomous intelligent robots will have a huge impact on society. So that's the reason why we want to start a robotics company, we want Nuro to play a role in accelerating that future. If you look at the last 30 years, many technologies have changed the way that we live our lives today. But if I have to pick three, that is the most impactful technology, I'll probably pick PC, Internet, and the smartphones. And they completely change how we produce and consume information today. But if you think about it, they haven't really changed how we interact and live in the physical world.
Jiajun Zhu (02:45): If you think about transportation, think about logistics, retail, manufacturing, and even how we spend time at home these days, it's very much similar to our parents generation or even our grandparents generation. So that's one observation and one key insight. Now, when we think about the next 10 years, we believe that robotics will, for the first time in history, to become capable enough to really solve problems and help people live a better life. So we want to create a product that could really give time back to people. And we want to create a product that gives people more access to goods and services. Now, thinking about the local goods transportation, which is the first product that we build. At the early stage of the company, in the first days of the company, we spent a lot of time thinking about, okay, what is the first product, what is the first robot that we want to build.
Jiajun Zhu (03:50): And we have four principles when we look at the first product. The first one, it has to be massive impact, a lot of people can use it on a regular basis. And that the second one is something that we could uniquely contribute and have a potential to build a really, really good product. The third one is there is a timeline constraint, right? We don't want to build something that will take 10, 15 years to realize. So ideally, the timeline for us is we want to create something from prototype to large scale commercialization in about five to seven years. And the last one is of course, it has to be a business that will have a lasting impact to the world. So with these principles, we very quickly selected autonomous delivery for local commerce as the first product. The reason being, actually the more we looked into it, the more excited we are about it. If you look at all the personal trips that people take in the US, people take about 200 billion trips, every year in the US, these are personal trips.
Jiajun Zhu (05:02): And research show that over 45% of all of these personal trips are related to shopping or running errands. So about 100 billion trips, these are for moving goods, not necessarily for moving people. So that is a really interesting discovery that led us to think a lot about sort of scratch this.
Jiajun Zhu (05:33): So 100 billion personal trips about running errands and shopping, right. And that is a very, very large portion of the trips and the driving that we do. And this led us to the idea of why don't we just automate that instead of moving people, why don't we move goods, and also people spend a ton of time on this activities. If you look at all Americans, we spend a million years every year in grocery stores. And this is just a ton of time that we spend doing things that we don't necessarily want to do.
Jiajun Zhu (06:11): So that led to the first idea of creating a vehicle, a custom built vehicle, that completely drives by itself, can deliver lunch, can deliver food, grocery packages, pharmaceutical products, all of these things to us, rather than having people go to the stores by themselves.
Alexandr Wang (06:36): Yeah, super cool. I mean, I really like that, kind of what you'd mentioned at the beginning around, hey, you know the physical world hasn't changed very much. I think that Peter Thiel has this quote, which is like, Hey, we were over the past, I think he looked at say, 30 or 40 years, if you look in the average home, not very much has changed, we're promised the Star Trek computer, but really, everything just is the same technology with different finishes. So I think that, it's a very noble pursuit, how do we change the physical world? How do we bring technology to the physical world? What do you think is kind of, you alluded to this a little bit, which is like all the time save, all the benefits to the economy, but what do you think is the resulting impact on society when autonomous vehicles are kind of everywhere serving everything from passenger delivery to goods delivery, to local delivery? How do you think that improves the world?
Jiajun Zhu (07:30): I think it will be huge, the impact will be really, really big. And autonomous vehicles will definitely make the roads a lot safer, right? If you just look at the US 40,000 people die from car accidents every year. And I believe that we will be able to eliminate all of these accidents, once we have autonomous vehicles everywhere.
Jiajun Zhu (07:55): And I also believe that autonomous vehicles will change how people spend their time, will change how people choose to live, and where they choose to live, and how cities are built, many, many aspects of our daily life in the future.
Jiajun Zhu (08:13): Now for Nuro, obviously, we think a lot about safety, and other social, economic and environmental impact that we could make as a company. So on the safety side, I'll give you some examples. On the safety side, in addition to the benefits and the impact that autonomous vehicles can make, the general autonomous vehicles, by replacing a lot of the human transport with goods transport. By replacing these passenger vehicles with goods only autonomous vehicles, which are a bit smaller, narrower and also less harmful vehicles, we will be able to achieve even more safety benefits than just using the regular passenger autonomous vehicles. And one concrete example is that we actually have external facing airbags at the front end of the vehicle. And this is something that is very hard to do for passenger vehicles. But because we don't have passengers inside we could actually be more innovative in terms of what safety features that we could add to the vehicle platform that would further reduce fatality rate in a car accidents.
Jiajun Zhu (09:32): And in terms of environmental impact. In the scenario that I would like to think about a lot is people today drive their two tons ... re-do this. In terms of environmental impact, the scenario that I would like to think about is, a lot of people in the US they drive their SUV pickup trucks to go to the nearest grocery store, just to pick up, say, a gallon of milk, right? These are very heavy gasoline vehicles. All the Nuro robots are full electric vehicles, when we deploy them at scale, we will be able to reduce CO2 emissions by a huge amount.
Jiajun Zhu (10:15): And in terms of economic impact, two of my favorite examples is one, research show that a lot of American families, they actually live in food desert. So there's like 20 million people in the US who live in food desert, they don't have reliable and low cost transportation, it's very hard for them to get fresh produce and healthy food. And I really believe that by solving this goods delivery problem and provide very low cost goods delivery service to the society, we will be able to really solve that problem.
Jiajun Zhu (10:57): Another example is, research actually showed that in the next 10 to 15 years, autonomous goods delivery will be able to create three million jobs in the US alone. That is also a really, really big economic contribution to the society.
Alexandr Wang (11:15): What is the driver of those jobs, is that just from basically the pure economic growth opportunities or is that because there's other downstream parts of the goods delivery problem, where those jobs come from?
Jiajun Zhu (11:27): So imagine a lot of these jobs will be created in the making of these robots, right? The manufacturing, the engineering jobs, the high tech jobs that we will create, and as well as, once these robots are deployed, in working with all the retailers, a lot of jobs will be created at the retailer location. So for example, grocery is one example. Another example is picking the packing at retailers. So think about groceries, there will be even the higher demand for grocery picking and packing once deliveries are a lot more popular. Because the cost of deliveries become a lot more affordable.
Alexandr Wang (12:19): Yeah. No, it's really cool, I think was almost as effective, like, how do you ... I think Uber created this as a level one step. But how do you bring these geographic distances to be less relevant to people's lives? For example, if you live in a food desert, that's irrelevant, because you have scalable goods delivery. Or how do you effectively make physical distance less relevant than sort of conceptual distances? I think it's super exciting.
Jiajun Zhu (12:46): Yeah, I totally agree with you. And I do think that, one way to describe this feature is that at that point we do have something like a teleportation machine. You don't need to really think about distance, in a way that we think about distance today. And I think that's a really exciting future.
Alexandr Wang (13:07): Yeah, totally. So Nuro is the first to market for autonomous delivery vehicles, you have a bunch of large scale partnerships with dominance, for example. And you're licensed in California with sort of a spotless safety record, as we've kind of discussed very top of mind and very important. To what do you attribute your sort of like speed, success and ultimately, a lot of the incredible advancements you all have made?
Jiajun Zhu (13:35): Yeah, well, as a company, safety is always a top priority for us and it influences how we design the hardware and the software and how we think about deployment, when we are ready to deploy things, and how we operate these vehicles on the road on a regular daily basis. Now, we're very, very proud to be the first company ever to get autonomous vehicle exemption from the US federal government, we actually have vehicles on the road, they're road legal today, and they don't have steering wheels and brake and pedals.
Jiajun Zhu (14:11): And we are also the first company to get commercial deployment licensed in California. And I would say that our focus on goods and our focus on zero occupant vehicle, and our approach to safety was also agreed by regulators and our partners, and they really see the value in the benefit of removing passengers completely from the vehicle platform. And that really help us in regulation and also in commercialization.
Alexandr Wang (14:47): Super cool. And then, from a technology perspective, I know that we've had this conversation before. Definitely there's a lot of ways in which you can innovate that are very differently from if you're a ride hailing company, you mentioned the airbags, but some may mistakenly assume, hey, from a technologies perspective, building autonomous delivery vehicles that don't have to carry humans ends up being way easier of an AI problem. Is that true? Or what are some of the nuances of that of goods delivery?
Jiajun Zhu (15:28): Yeah, that's a great question, Alex. And compared to passenger AVs, I would say that goods only autonomous vehicles are simpler. If you think about passenger autonomous vehicles, it essentially solving three problems, right. You're building a vehicle that is safe for passengers, and it is comfortable for passengers, and it has to be safe for other road users. So you're solving three problems at the same time. And to make this more challenging, the first two problems are actually conflicting goals, right? Making the passenger safer, doesn't necessarily make it more comfortable. So I think this is a very, very hard problem.
Jiajun Zhu (16:13): Now, if you do not have passengers, and you're building an occupant-less vehicle, then you're eliminating the first two problems right away. So you don't have passenger safety or passenger comfort, you're just focusing on the safety of other users of the road, right? Now, it is still a very, very hard problem. No one has solved it at scale yet, and our vehicles still need to navigate busy streets, deal with all the dense traffic in urban and suburban scenarios, and obey traffic rules. So it is still very, very hard, challenging engineering problem.
Alexandr Wang (16:55): Yeah, I love to like dig a little deeper here, right. So as you mentioned, you share some challenges with autonomous passenger vehicles, right, like dealing with complex urban scenarios, crowds of people, et cetera. So what are some of these challenges that you share? And then conversely, what are the things that are unique to the goods delivery problem that are harder, interesting in their own way, but are sort of independent?
Jiajun Zhu (17:28): Yeah. So there are a ton of challenges that we share with passenger autonomous vehicles. So A, is to be able to navigate the busy dense traffic, right, you have to know where you are, you have to detect all the other road users, right? Pedestrians, cyclists, vehicles, you have to know what they are. And not just these high level types, but also emergency vehicles, such as school buses, fire trucks, right. And when you look at scenarios or scenes, like construction zones, or accident scenes, you understand this, and then you can still navigate through these scenarios.
Jiajun Zhu (18:24): And if we're moving objects, you need to know how fast they are moving, you need to be able to predict in the next few seconds, where they will be, this is also a pretty challenging problem. And you need to deal with bad weather, like heavy rains or heavy snow. And at the end of the autonomy stack, you have to figure out how to safely navigate around all of these dynamic and static objects in the world, and then get to your destination. And on top of all of these software challenges, you also have hardware challenges, like building the sensors, building the compute, building the vehicles, integrate all of these things together, so that they are very reliable. And you can build them at large quantity and also meet your cost and performance targets to build a viable business. So all of these challenges that we share with passenger autonomous vehicles.
Jiajun Zhu (19:29): Now in terms of unique challenges, I would say that we do have unique opportunities and that also creates interesting and challenging engineering problems for us. So for example, the external facing airbags, we have to know when to deploy them. That is also an interesting problem. And we also have this really cool technology which we internally called super stop, which is a mechanical system that could really stop the vehicle, really, really quickly at very, very high deceleration. Now, if you have a passenger in the vehicle, it would not be comfortable for the passenger to stop that quickly. But because we don't have passengers, we can stop the vehicle from full speed driving all the way to stop maybe within a couple of meters. And that would really create a better, even more safe road for other road users. But that itself is a really, really big hardware challenge and technical challenge that our engineers spend a lot of time on.
Alexandr Wang (20:44): Yeah, one of the things that and you just touched on it, but I think it's very interesting, and we've talked about this before, obviously, one of the things that Nuro by focusing on goods delivery, kind of enables Nuro to innovate on or utilize is, is experiment more with comfort, if that makes sense, like comfort is irrelevant to the goods in a Nuro vehicle. Whereas for a lot of passenger vehicle companies, they have this dimension they have to optimize, which is comfort, like how do you have rides that don't make you nauseous, but because of that, that allows you to have a lot more flexibility. And ultimately, that's one of the differences between sort of like the moon shot versus the Mars shot analogy that you mentioned before?
Jiajun Zhu (21:29): Exactly.
Alexandr Wang (21:31): One of the things that I think is interesting about Nuro is just sort of the A, the fact that you guys are scaling up pretty quickly. And scaling for your fleets pretty quickly creates a lot of interesting challenges, maybe very early on in Nuro's sort of company lifecycle, and one of those I think is really around data. So your fleets are producing incredible amounts of data. How do you think about prioritizing it, mining it, focusing on what matters? And so you're learning new and interesting things? And then ultimately, using that to approach kind of this long tail problem of machine learning?
Jiajun Zhu (22:12): Yeah, great question, Alex. And we do have a pretty large fleet collecting data every day now. So making use of that data is definitely a challenge and a really important task for us.
Jiajun Zhu (22:30): And also, using this large amount of data to validate our system performance is an important part of our safety case. So this is really, really important here at our US team in the company. And there are a few stages of this entire process, right, in terms of collecting and then processing the data. It is mostly machine jobs, and fully automated. And we want this to be as automated and as reliable, as much as possible. So the machines can punch through a lot of data very, very quickly.
Jiajun Zhu (23:07): When it comes to analyzing and labeling the data, I'm sure this is the part that you have a lot of expertise. And we're very, very happy in our partnership to solve this problem together. Now we have a hybrid approach, which relies on both the software and the machines and as well as human laborers. Now we spend a lot of effort making the software very smart, as smart as possible. So that we could have very high recall at detecting all these interesting events. And at the same time, very low false positive rate. So that amount of data that we have to verify in a review by human is still manageable.
Jiajun Zhu (23:54): And you asked good questions about AI and the machine learning. Yeah, so we use machine learning and train AI models all the time. And we pretty much now have a automated process to so that every day, whenever we have new data collected, all of these data will go through this pipeline, and at the end of the day, they will help contribute to improving our AI models. We do have quite a lot of AI models. A large portion of our autonomy stack today is relying on machine learning now. And that is quite different from 13 years ago, when I started working on self driving, it was a lot of heuristics, a lot of engineer the rules, but today, most portion of the autonomy stack is the learnable.
Alexandr Wang (24:52): And what do you think about that? Oftentimes, I think one of the interesting things about the autonomy problem, right, is that this problem of the long tail, right? And there's just a sort of a general problem, machine learning algorithms aren't great in general dealing with long tail, how do you think about balancing the sort of performance on that sort of head or average use cases versus the long tail use cases and designing the kind of like an approach to that into your sort of technology stack?
Jiajun Zhu (25:21): Yeah, well, so the long tail edge cases are always super interesting. And I would say that the more long tail edge cases that we see, really help make the overall technology and the overall stack even more robust. I'll give you some examples. After driving a lot of miles, many unusual things are no longer that uncommon. And so for example, we see people drive on the wrong side of the roads all the time. And in some more dangerous scenarios, for example, which are really hard cases for autonomous driving are like, sudden review, for example, like a vehicle or a pedestrian just reviewed themselves very quickly, maybe previously were occluded, right? And other vehicles, very reckless drivers were violating right of ways. Or jaywalkers were not paying attention.
Jiajun Zhu (26:21): These cases, I would say, they still are edge cases that are pretty challenging for autonomous vehicles. And I think the more data that we see, like this, we go through our entire data labeling training process, and help our machine learning models see more of these examples so that they can do a better job and make these models more robust over time.
Alexandr Wang (26:53): Yeah, totally. One thing I love to chat a little bit about is, your sort of like sensor strategy, right? I'd love to hear how you think about your sensor platform, the managing that challenges that sensor platform, how you intend to improve and iterate on that, because this is sort of like a general problem that you'll need to think about or platforms like yourself need to be thinking about over a multi year, multi decade time horizon. How do you think about that? What's your strategy to iterate and improve on your sensors? And then how does that play back to your AI strategy?
Jiajun Zhu (27:30): Yeah, I that's a great question, Alex. And Nuro, we use a lot of different types of sensors, so that we can have different sensory modalities when we perceive the world. And we are now on our third generation sensor platforms. So a lot of iterations and improvements over the last five years. And I would say that you always, like by designing the sensor platform, you always start with your product specs. And then you translate that to system requirements, and subsystem requirements, and the sensor requirements. And you put these sensors together, right? Cameras, lasers, radars, we do have some in house sensor development, as well, that we're very, very excited about.
Jiajun Zhu (28:21): And you put these sensors together on the test platform, you collect a lot of miles, try to understand the data collected back, and then compare that to a spec and really make sure that you are building these according to spec. But even when you build these things according to spec sometimes you will discover edge cases, as we talked about earlier. And then you will take these new information into account when you design the next generation of platforms. Now, one thing that is, I think, particularly important is, how you deal with this upgrade, right, like transition from the previous generation of sensor platform to the next generation. Sometimes they have a lot of overlap, sometime they differ quite a bit. And how do you deal with two versions of this sensor platform coexist at the same time, and how much old label data, how much old training data you could reuse, and how much you would have to collect in a label again, so these are all very interesting problems, especially when you get to this scale.
Jiajun Zhu (29:34): Thinking about how to optimize the process for solving this problem become really interesting. And one example is imagine you have a brand new sensor system that has like 90% overlap with the old system, but the 10% is really, really new. Then you have to figure out how do you validate that 10% by collecting new data, but do all the other data that you can still retain and to make sure that they can be still useful for the new sensor system. So I think that is a very interesting kind of operation problem. Is a very interesting machine learning problem. Is a very interesting kind of an infrastructure data pipeline problem.
Alexandr Wang (30:19): Yeah, and I want to pull the thread to the limit, right? Like, imagine the world that obviously we're all building towards where, let's say there's Nuros in every single city across the world. And there's just like tons and tons of data coming off your robots. I think a lot of these things that we're talking about become especially important, A, you're likely to have multiple different sensor platforms operational at any one moment. And you have to be thinking about that. B, they're going to constantly encounter new edge cases, new scenarios, and you want to figure out how to integrate those learnings in a scalable way, while you're just getting way too much data that you possibly imagine. So how do you think about in a scaled way, or the architectures today that will scale the designing the sort of like fleet learning system that's able to identify problematic situations, learn from them, apply that learning back to the fleet, and do so across multiple sensor platforms, multiple software packages, multiple levels of maintenance, et cetera?
Jiajun Zhu (31:25): Yeah, I think I think the nice thing about the world is actually that there are a lot of similarities in the common elements in the world that we live in, right? So it's not like you deploy a million robots and all the sudden you discover a million new things. So I think as we scale our operation, and expand our commercialization, when the more, we will invest more in the technologies to discover and find really rare and the really interesting cases. And I think 99% of the data, they're probably not adding a lot more new information to the existing system. And I think, how can we detect these rare cases and how can we detect these really new and useful piece of data, will be the focus.
Jiajun Zhu (32:31): And, of course, all of these vehicles, the large fleet, uploading a ton of data all the time, that also presents some engineering and technical challenges on building that very reliable, large scale infrastructure. And this is also something that we're investing.
Alexandr Wang (32:51): What are the problems? I think, a lot of times as you scale up, as we think about Nuro scaling for into the future, a lot of these problems, there's new problems that emerge, this problems that seem to get harder, what problems you get easier as Nuro scales, and there's more and more Nuro robots in every city around the world?
Jiajun Zhu (33:12): Well, so I would like to think that in building autonomous vehicle company is a brand new problem, right? This is like, there's no manual of how to build a company, how to build a product that drives by itself, that deliver things to many customers every day, go pick up these goods from retailers every day, and you operate this at large scale. How do you maintain the fleet? How do you make sure that they have very high uptime, very high utilization, you can operate all these things in a very efficient way. And what happens when you have a soft regulation, what do you do? Like this entire process, I think it is a super, super interesting problem for the industry to solve. And once you solve that, once you build that playbook, once you have that process, I think a lot of things will become easier. And it will look more like the traditional industry in the past. So I think that's one part. And the second part is, I also think about a lot of the investment that we make in data processing, how you update the AI models through all of these new data that gets uploaded every day. Like once you build that pipeline, the technology will eventually get to a point where you just have more data and it will automatically improve by itself. And I also think that at that point, the R&D process will also look a bit simpler.
Jiajun Zhu (34:58): Of course, as we expand to new geographic areas, we have to solve new problems like, different weather conditions and different road signs and different driving behaviors to get to the local markets. I think all of these problems that we have to address in the future. But I also do believe that as we grow the business, as we have more data and more robots on the road, a lot of things will become a lot easier.
Alexandr Wang (35:27): Yeah. You have a unique vantage point because as we've talked about, you were working on in the very early days, the Google self driving car project. And so my question for you is, obviously the entire industry has really developed over the course of the past, you mentioned 13 years, what are problems that have kind of surprised you at how difficult they've been? Or maybe new challenges that have popped up that have been really interesting? And what were problems that like you thought maybe 13 years ago would have been very, very hard, but then ended up becoming being very easier or being not bottlenecks for the technology?
Jiajun Zhu (36:09): So I think 13 years ago, when I started working on this problem, there was no, like, really reliable sensor at a very reasonable cost. And there was no deep learning at that time, it was more kind of a classic computer vision technique to solve some of the hardest perception problems. And I think fast forward 13 years later, we see a ton of progress on machine learning and using machine learning to solve these really, really hard and challenging perception problems, prediction problems and behavior problems.
Jiajun Zhu (36:53): So I think, very, very glad to see the progress. I think perception used to be one of the really, really hard problems a decade ago. And now with all the innovation and progress in machine learning and compute power, and the sensors, I think we see a ton of progress in AI. I'm very, very confident that this is a very, very solvable problem now.
Jiajun Zhu (37:22): The one area that I think is still going to be challenging, and it will still require a lot of innovation to solve is more on the behavior side. And I think we're seeing a lot of good progress in the last few years. But I think in terms of how we can reliably predict other agents behavior, and reliably coming up with a plan, or trajectory of how our robots will navigate the roads, given a super complex, dense traffic around them. I think that is still a very, very interesting technical problem for us to solve.
Alexandr Wang (38:02): Yeah. And then, maybe one question is like, do you think it's going to be solved through new breakthroughs that are yet to be found in the research community, almost like the next Nuro networks or whatnot? Or do you think that there's real promise to the existing approaches and it's just sort of a matter of whittling away at the machine learning problem.
Jiajun Zhu (38:27): I think we have a very good foundation for how to solve this problem. And I think we're making a ton of progress into both Nuro and also, if you look at the industry, the research community was seeing a ton of progress. I do think that we have the core technology and the fundamental framework to solve these problems. I think it just takes a bit time to really refine it to the point where it is fully solved. So I remain very, very confident that this problem can be solved. And it doesn't require like a scientific breakthrough at this point. I think it's mostly, more time, more engineering. There will be some new innovation required in AI and the algorithms, but not something that is like fundamentally different than what we're doing today.
Alexandr Wang (39:33): Awesome. Well, to kind of close off the last few minutes left here, as Nuro continues of expansion into new markets and your use cases, what are the future challenges that you expect that Nuros yet to face?
Jiajun Zhu (39:50): Yeah, well, as I mentioned earlier, we're still working on the autonomy tech, we're making good progress. But I think there's still more work to do before we can deploy this in more markets, and in the future when we expand to more geographic areas, one challenge as I mentioned earlier is different traffic behaviors and different weather scenarios like heavy rains and snows. And in terms of our use cases, we will expand from one vertical to multiple verticals, which we are working on right now. So from groceries to meals, right, from pizzas to packages, and we want to focus on how to further improve the customer experience. How can we improve the drop off and pickup experience, how many orders that we can batch? And what is the best way to create a product that really delight our customers? I think a lot of things that we can do to build even better product.
Alexandr Wang (40:59): Yep. Awesome. And then as you scale your AI across in the future, like first different locations and later future use cases, what are you seeing is like the exciting challenges yet to solve there, in scaling across different geographies, different use cases, different operating zones over time?
Jiajun Zhu (41:25): Yeah, so I think fortunately, there are a lot of similarities, right, and a common things seen in the world. So I'm confident that our AI will be very generalizable in dealing with different environments. One example is that we are already operating and testing our robots in three different states today, so California, Texas and Arizona. And that shows how generalizable the technology is. And as we expand to more areas, we will always collect more data and do some testing in new locations. But we expect that the amount of data and testing that we collect will decrease over time. For every new city that we deploy, I think the key there is really to find that last remaining 1% or point 0.1%, of new information, new edge cases. And really feed all this same information, new information to the AI model so that it can become even more generalizable. And I think there's a ton of interesting ideas that we're working on, how to improve this process. And I'm personally really, really excited about the time when everyone, the industry has crossed the zero to one moment, right. The autonomous vehicle is already deployed at one city, it's already a city scale operation, and now we're just scaling to more cities.
Alexandr Wang (43:04): Yeah. Awesome. And just last question, when will we see Nuros in every single city? And I'll be able to get my burrito from a Nuro vehicle, within five minutes.
Jiajun Zhu (43:15): San Francisco will be earlier than New York City, for sure. Every city will take a while. But it's definitely the long term aspiration. It's very hard to predict the timeline. But I'm very, very confident that autonomous robots will be a common object in the next five years. So for all of us who dreamed about building robots, when we grow up, right, I really think that this is the best time in history to do it. And I hope that 10 years from now, if we were talking about longer term, Nuro will be not only offering robots on the roads in the cities, but also building robots for people's homes.
Alexandr Wang (44:03): Thank you so much for sitting down and chatting with us today, JZ. This is a lot of fun. And good luck with deploying Nuros to every city.
Jiajun Zhu (44:11): Thank you, Alex. Thank you.