From6:52 PM, GMT
To8:23 PM, GMT
Tags:
Stage 1
Presentation
NeurIPS: Are We Learning Yet? A Meta-Review of Evaluation Failures Across Machine Learning
+ Read More
Speakers: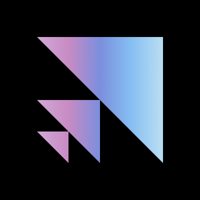
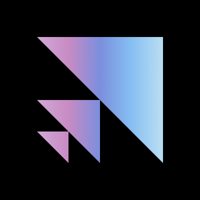
Evaluation issues often undermine the validity of results in machine learning research. In collaboration with researchers from Stanford University, the University of California, Berkeley, and the University of Washington, we conducted a meta-review of 100+ survey papers to identify common benchmark evaluation problems across subfields. In some cases, several years’ worth of progress in certain fields may be misstated. Our meta-review surveys evaluation papers reporting on a broad range of subfields, ranging from computer vision to deep reinforcement learning, to recommender systems and natural language processing, and more. We found a consistent set of failure modes, which we organized into a systematic taxonomy.