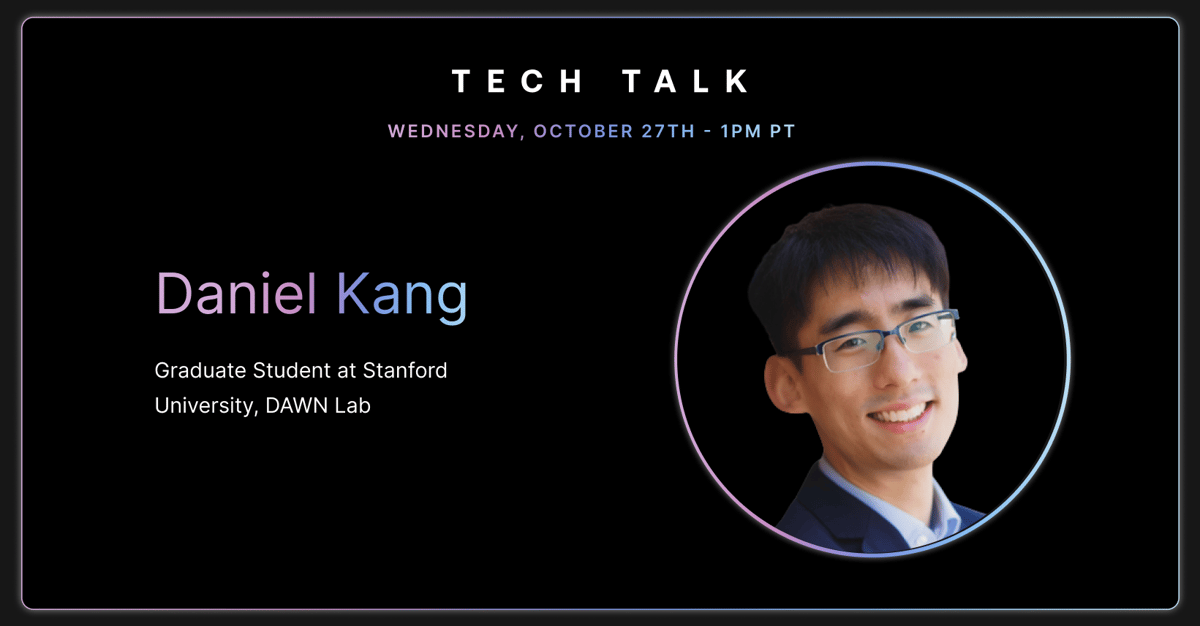
**Session Abstract: ** Machine Learning (ML) is increasingly being deployed in complex situations by teams. While much research effort has focused on the training and validation stages, other parts have been neglected by the research community. In this talk, Daniel Kang will describe two abstractions (model assertions and learned observation assertions) that allow users to input domain knowledge to find errors at deployment time and in labeling pipelines. He will show real-world errors in labels and ML models deployed in autonomous vehicles, visual analytics, and ECG classification that these abstractions can find. I'll further describe how they can be used to improve model quality by up to 2x at a fixed labeling budget. This work is being conducted jointly with researchers from Stanford University and Toyota Research Institute.
** Speaker Bio: **
Daniel Kang is a sixth-year PhD student in the Stanford DAWN lab, co-advised by Professors Peter Bailis and Matei Zaharia. His research focuses on systems approaches for deploying unreliable and expensive machine learning methods efficiently and reliably. In particular, he focuses on using cheap approximations to accelerate query processing algorithms and new programming models for ML data management. Daniel is collaborating with autonomous vehicle companies and ecologists to deploy his research. His work is supported in part by the NSF GRFP and the Google PhD fellowship.
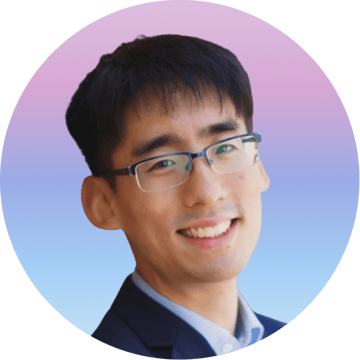
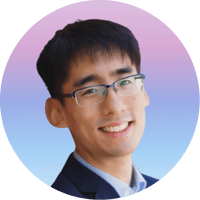