The Next Big Step in Robotics: Machines That Adapt to People
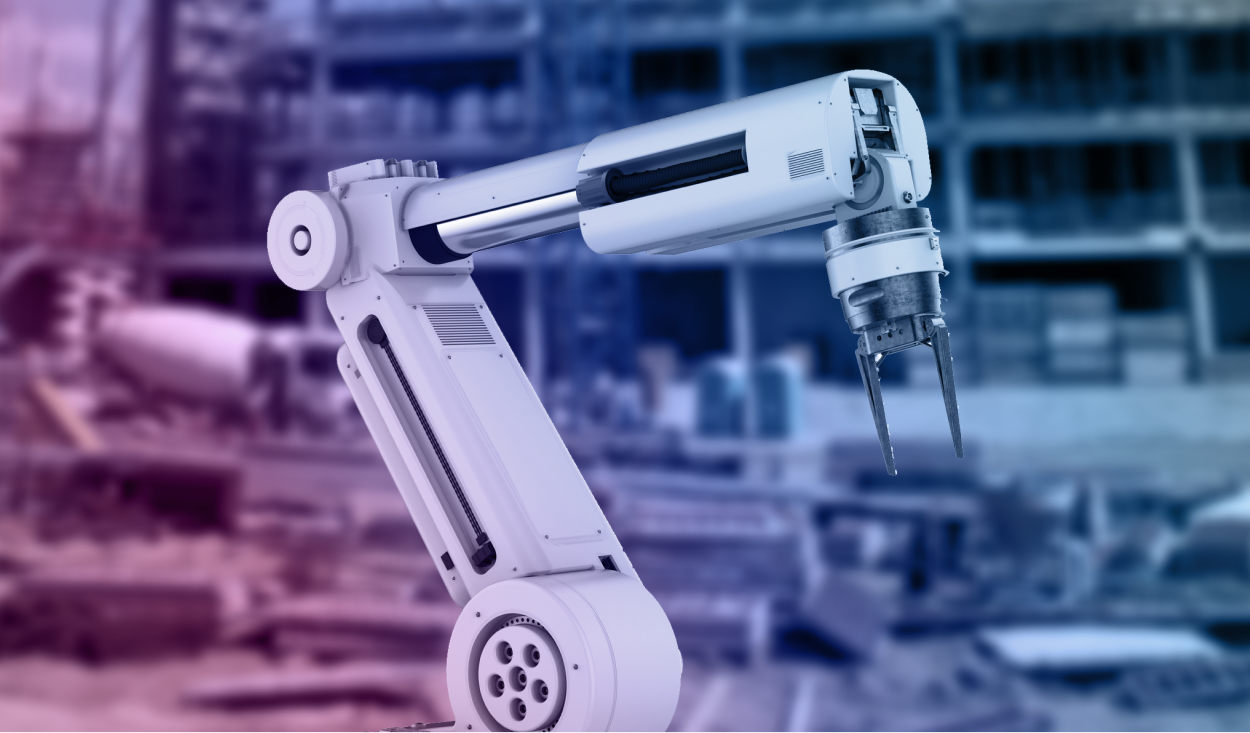
Bringing robotics into to widespread use through AI will require imagination, inspiration—and rethinking assumptions.

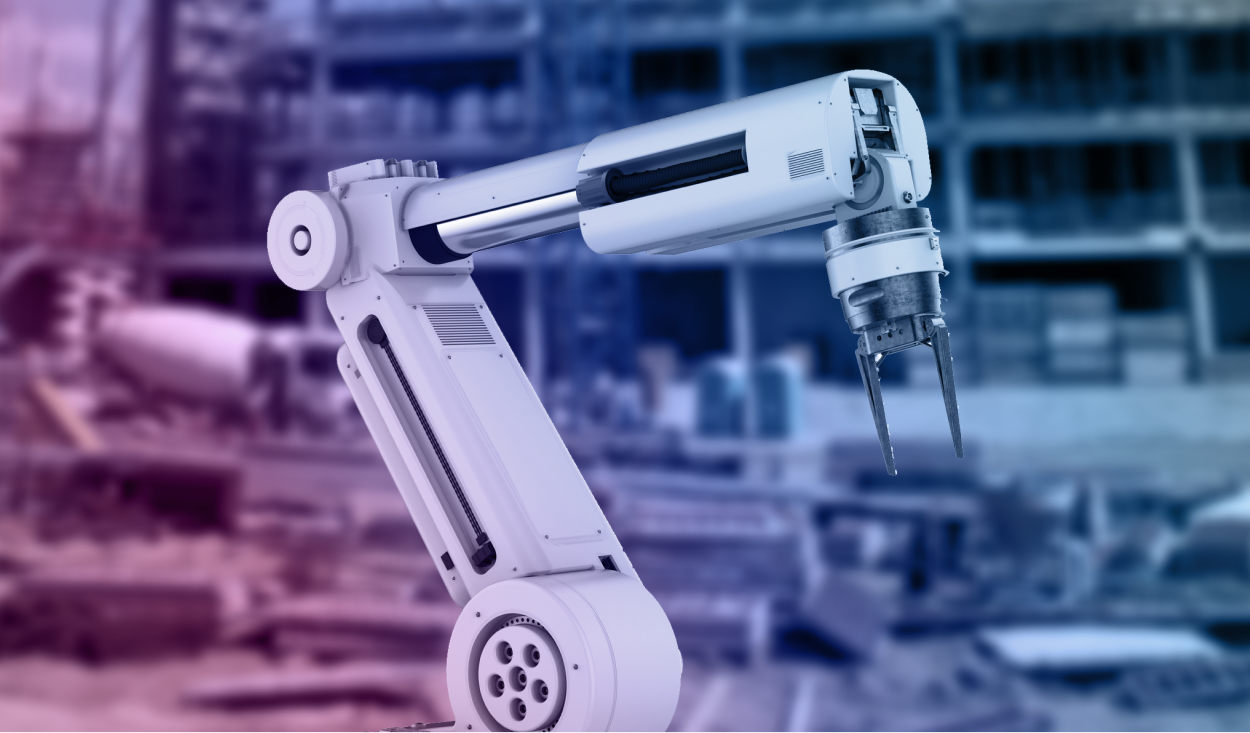
Until now, people who use robots in industrial applications have had to adapt to them, but for artificial intelligence and robotics technologies to permeate society, the industry must start building machines that adapt to people. That's what Daniela Rus, director of the Computer Science and Artificial Intelligence Laboratory (CSAIL) and deputy dean of research for Schwarzman College of Computing at MIT said during a panel discussion on AI and the Physical World at the Index AI Summit. Joining Rus in the discussion was Pieter Abbeel, co-founder of Covariant, director of the Berkeley AI Research Lab, and co-director of the Robot Learning Lab at the University of California, Berkeley.
It is particularly important that robots be able to handle variable tasks and not just the repetitive motions they’re currently engaged in at factories and other limited environments, Abbeel added.
For her part, Rus said her work was inspired by the possibility of developing machines “that give people superpowers and help people with physical work.” She imagines a future in which AI and robots support people by doing cognitive and physical work, with the same pervasiveness that smartphones support us now.
Robots Remain Isolated—for Now
The first industrial robot, the Unimate, introduced in 1961, was invented to do industrial “pick-and-place” operations. Sixty years later, there are tens of millions of robots that can do much more than people can, yet these robots remain isolated from the factory floor where humans work, because they’re too large, heavy, and dangerous to be around the more “soft and compliant” and more dexterous and intelligent humans.
Rus wants to build robots with the same kind of compliance, intelligence, and dexterousness, so much of her recent work focuses on soft robotics. More broadly, she is also wondering about how the industry will move from an era of physically isolated industrial robots to one of robots in human-centric environments helping people with physical tasks.
In the coming decades, machines will be available in a broader diversity of shapes and will “be made out of all the materials available to us,’’ including woods, plastic, engineered materials, paper, ice, and even food, Rus said.
Her lab is not only developing the computational approaches for designing robots from a variety of materials, but is also working on the brains that will allow robots to do what the user wishes the body to do. For example, among the applications her lab is building are robots “that swim like fish and move like turtles, robots that brush your hair, robots that pack your groceries and can reason that you shouldn’t put milk on top of lettuce,’’ she said.
Robotic Challenges: Novelty Versus Reliability
It’s more important to achieve very high reliability than it is to to show a robot doing something for the first time, Abbeel said.
But high reliability becomes especially difficult once you move into environments with variability. The millions of robots doing useful things today tend to be in confined environments and are preprogrammed to do certain repetitive motions.
While that is a productive way to build a car or electronics, robots in more open-ended spaces such as warehouses, farms, and construction sites must be able to operate in semi-structured environments where they will always be faced with something new, he said.
The robot will have to understand what it’s looking at and then react and learn from that. The robot will have to face many variables at the same time and react appropriately and reliably, or else it’s not creating any value, Abbeel said.
“Covariance” refers to working on how to achieve many lines of reliability for whatever the robot is supposed to be doing, even when the robot is constantly faced with different items in the warehouse with different configurations. Abbeel called that “a very different kind of challenge oriented around reliability” compared to what he does at Berkeley, which is academic work focused on novelty.
Showing the feasibility of a solution for a new type of problem is one thing, but taking that solution from the research lab into the physical world is a huge step that involves resolving a lot of issues around robustness and use cases, he said.
Approaching the Nebulous Nature of Research
Sometimes, research projects pan out and sometimes they don’t, said Rus. When they don’t, you have to ask why. Regardless of a project’s success, there is always some value—something to be learned.
At that point, researchers can ask what assumptions need to be introduced in order to find a solution for the problem they’re trying to solve. Even though that may sound abstract, Rus said, the approach works.
Abbeel agreed, saying there are definite ups and downs in research, and sometimes things move fast. Ultimately, the idea is to ask yourself a question you don’t know the answer to; you typically hope for the answer to go one way rather than the other. Often, one way allows you to build something impressive, and the other allows you to realize that you cannot yet build that impressive thing, he said.
“The secret to research progress on the academic side is really to ask the right questions,’’ which can be hard to do, and have a fast turnaround in getting answers, Abbeel said. The biggest research lesson he learned as a Ph.D. student and later as a professor is that you can often learn the same lesson by running a smaller experiment instead of a large one.
A good life lesson when something doesn’t pan out is to be open-minded and check your assumptions rather than having a picture in your mind of how you want things to go, Abbeel said.
What the Future Holds
It’s useful to have people understand what technology can and can’t do and have a discussion around what it should and shouldn’t do—and what technology must do for the greater good, Rus said.
Over the next five years, Rus said, robotics companies need to allay people’s fears about robots taking over and stealing jobs. Everyone needs to understand that machine learning robots are simply tools for people to use as they choose , that they're not intrinsically good or bad, and that they're not interchangeable with people. “Machines have speed, but humans have wisdom,’’ Rus said.
It’s important for people to understand that AI and robotics can make our lives better, Abbeel added. The he motivation for him to work on robot brains is that it’s an opportunity for people to learn about all the things that AI and robotics can do and are already doing in our world, as well as learn about the people who are building those robots and AI systems.
Within a year, Abbeel expects to see more deployment of AI in the software world than in robotics. That said, robots are moving beyond car factories, where things are extremely structured, into warehouses for order fulfillment.
Rus cited three areas where she feels AI needs to be enhanced. The first is coming up with breakthrough ideas and applications to manage the major technical challenges facing AI. This includes the computational infrastructure needed for progress. The second is sustainable AI to help with climate change and the planet by generating better insights. The third area is to get more serious about AI and privacy.